1. Data Mining
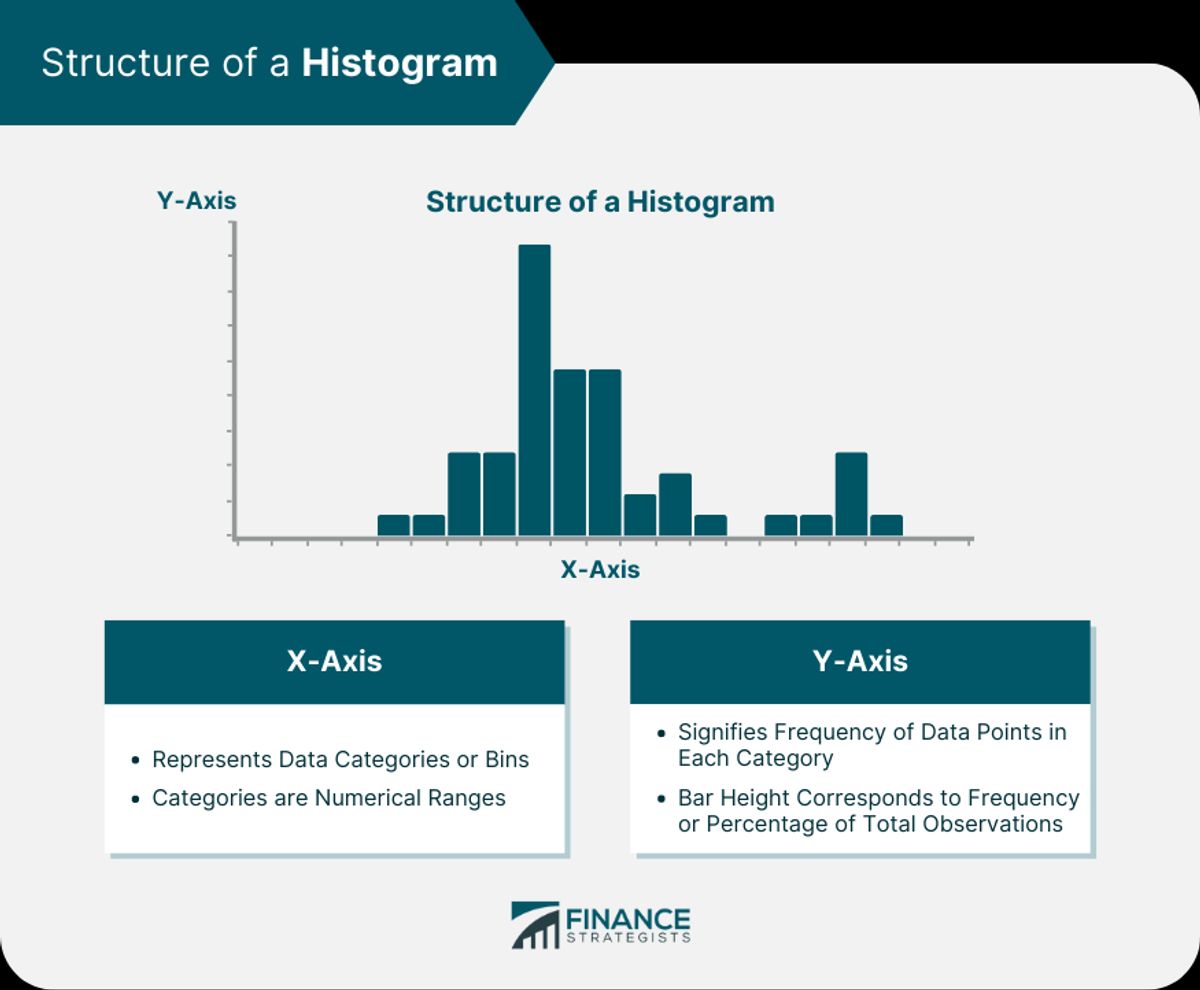
Data mining is a critical business intelligence technique that involves the extraction of useful information from large datasets. Utilize decision trees for decision-making to uncover patterns and relationships that can inform strategic business moves. By following systematic data analysis steps, organizations can transform raw data into meaningful insights.
Data mining is not just about processing data, but also about leveraging this information for a competitive edge. Companies integrate data sources for business intelligence, allowing them to gain a comprehensive view of their operations and market dynamics.
Data mining empowers businesses to predict future trends and behaviors, enabling proactive decision-making rather than reactive strategies.
Here are some key benefits of data mining:
- Identification of sales trends
- Effective marketing campaign planning
- Customer behavior analysis
- Fraud detection and prevention
Incorporating data mining into business processes ensures that decisions are data-driven and aligned with the company's strategic goals. Use data visualization techniques to present the findings in an accessible way, enhancing the decision-making process.
2. OLAP (Online Analytical Processing)
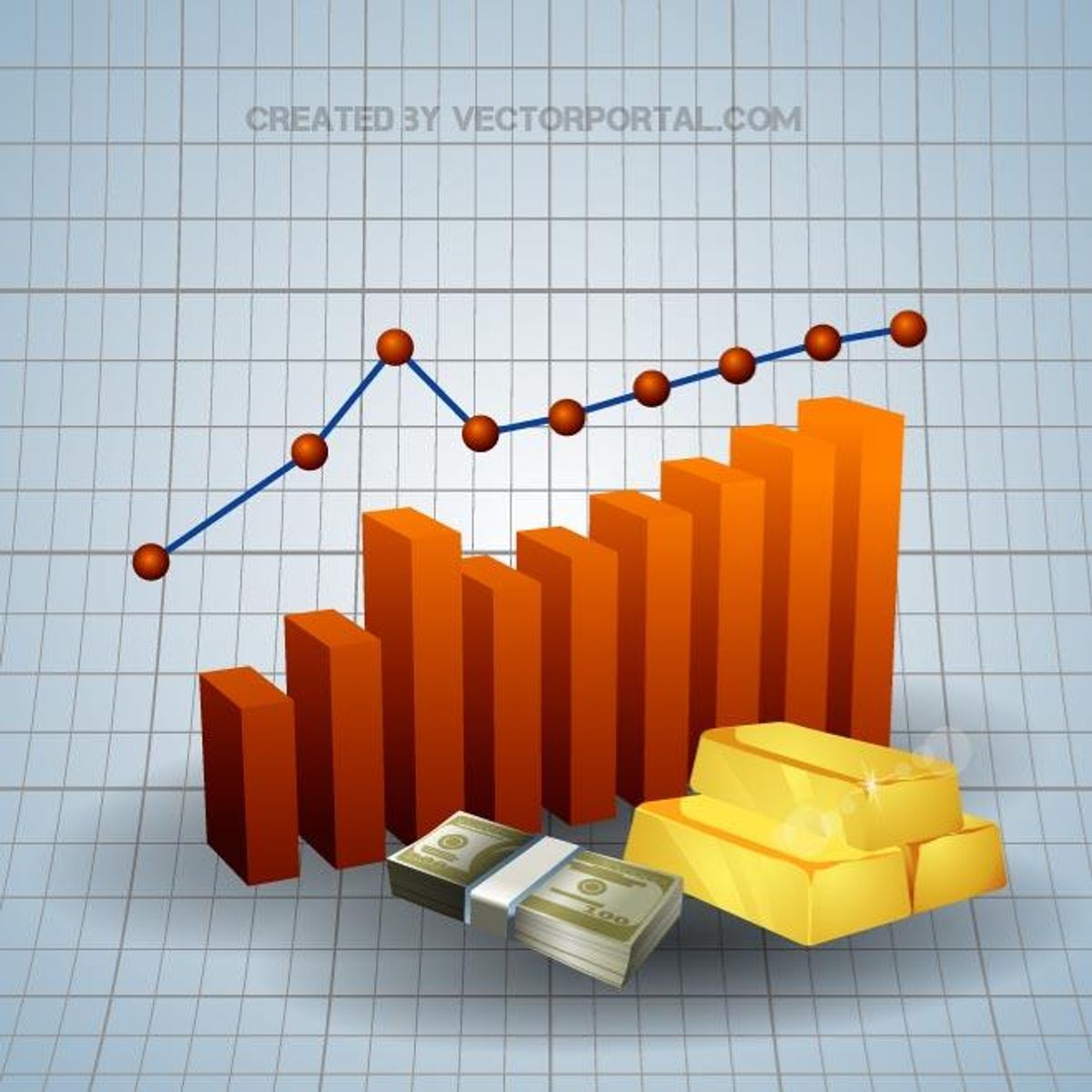
OLAP tools are pivotal in the realm of Business Intelligence (BI), enabling multidimensional analysis of data stored in databases. These tools facilitate complex calculations, trend analysis, and data modeling, essential for making informed business decisions.
OLAP systems are designed to optimize data retrieval for analytics, supporting complex queries and aggregations across large datasets. Unlike traditional databases, which focus on transactional processing, OLAP is tailored for analysis, making it a cornerstone for data-driven strategies.
OLAP's ability to quickly answer multi-faceted analytical queries makes it indispensable for businesses that require agility in decision-making.
The following table illustrates the contrast between OLAP and other data systems:
System Type | Designed For | Optimization Focus |
---|---|---|
Databases | Transactions | Speed, Efficiency |
Data Warehouses | Query, Analysis | Read Operations |
OLAP | Multidimensional Analysis | Complex Queries |
By leveraging OLAP, organizations can dissect and understand their data in ways that traditional reporting and querying cannot match. It is a key component in the suite of BI tools that support data analytics operations, including data mining, querying, and reporting.
3. Data Visualization
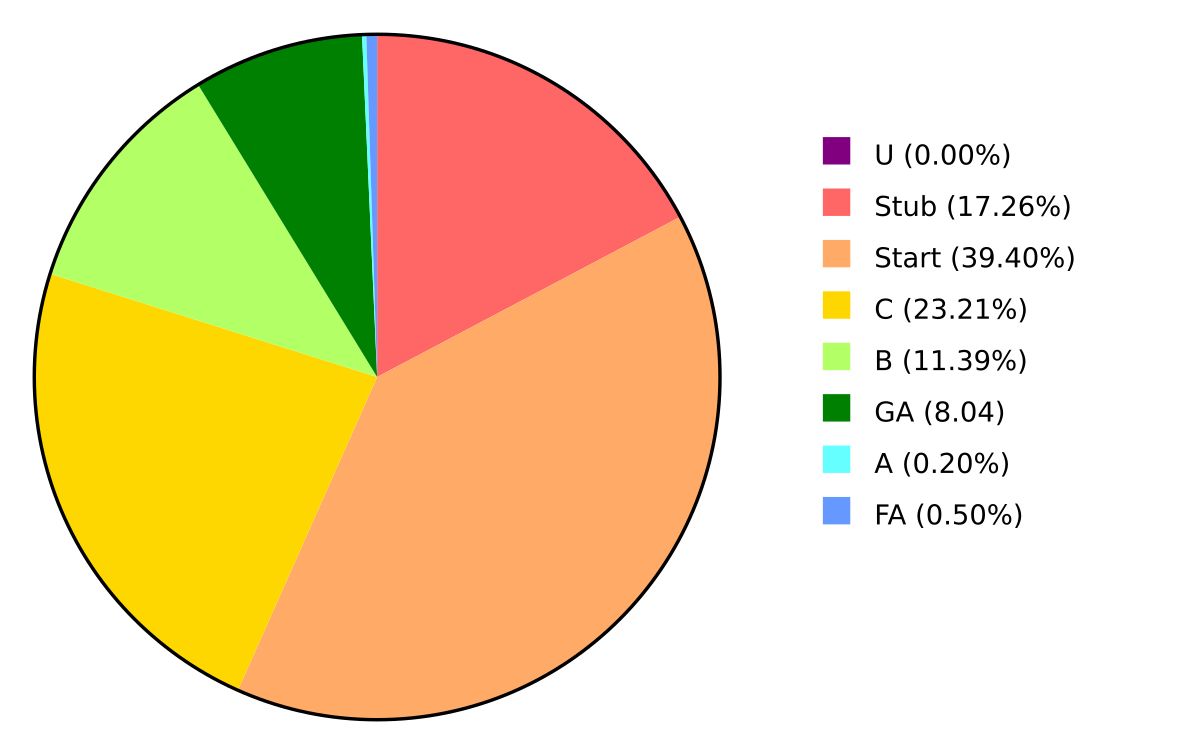
Effective data visualization is a cornerstone of Business Intelligence, transforming complex datasets into clear and actionable insights. Presenting data in a user-friendly design is not just about aesthetics; it's about making information accessible to all stakeholders, regardless of their technical background. Tools like Tableau and QlikSense lead the way in this domain, offering intuitive interfaces for creating interactive dashboards and reports.
Tableau, for instance, supports a wide range of data sources and provides live visual analytics that empower users to quickly identify trends through a simple drag-and-drop interface. Similarly, portfolio visualization tools are revolutionizing the way investors assess risk by providing interactive dashboards that illustrate risk metrics and potential outcomes under various scenarios.
Embracing data visualization is not just about adopting new tools; it's about fostering a data-driven culture that values the clarity and efficiency that good visualization provides.
For those looking to enhance their data visualization skills, numerous learning opportunities are available, such as:
- Data Visualization with Tableau specialization from the University of California Davis
- Data Visualization and Communication class with Tableau from Duke University
- Guided Project on Visualizing Citibike Trips with Tableau
These courses and projects are designed to help individuals leverage the power of data visualization to make more informed decisions.
4. Predictive Analytics
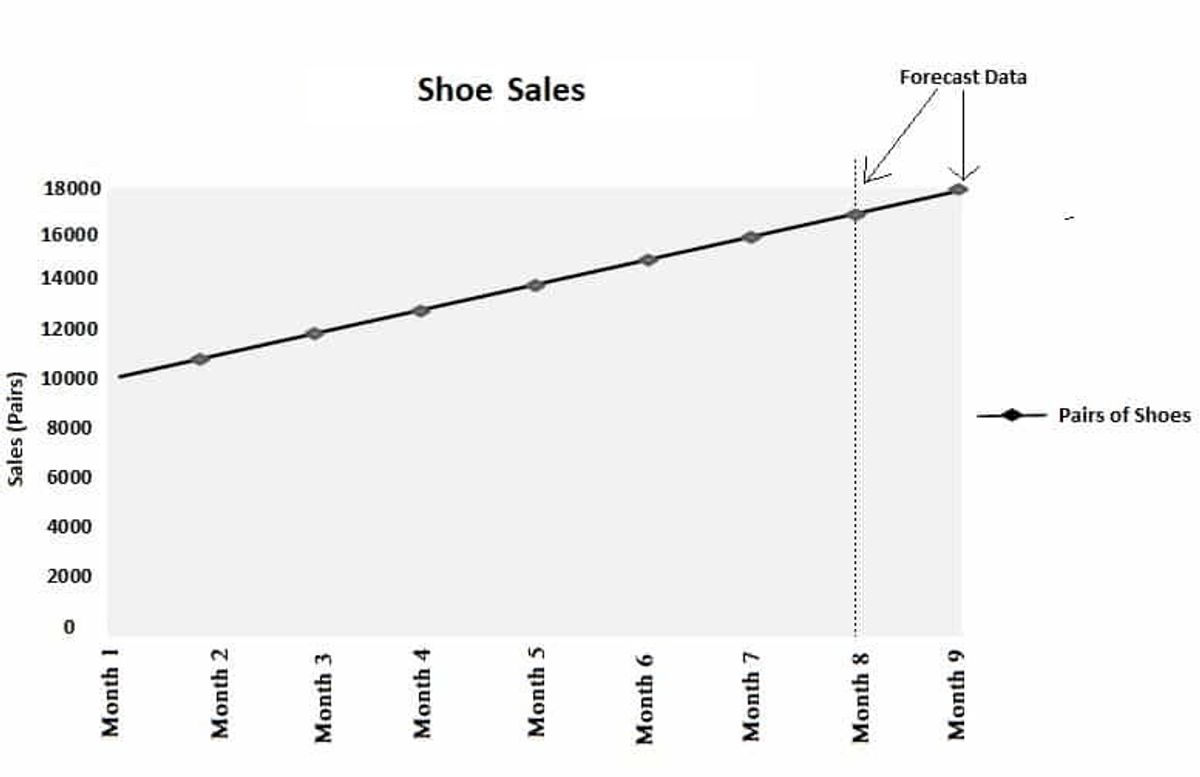
Predictive analytics harnesses historical data, statistical models, and machine learning algorithms to forecast future events and trends. This technique is pivotal for organizations aiming to identify risks, anticipate potential losses, and make data-driven decisions.
Key applications of predictive analytics include demand forecasting, fraud detection, and maintenance optimization. These applications enable businesses to proactively address challenges and seize opportunities by understanding what is likely to happen in the future.
Predictive analytics transforms raw data into actionable insights, allowing companies to strategize with confidence.
For instance, in fraud detection, predictive analytics scrutinizes patterns and trends that may indicate fraudulent activities. A sudden surge in high-value transactions by a customer could be flagged as potential fraud, prompting further investigation.
The integration of predictive analytics into business intelligence tools also empowers employees to make evidence-based decisions. With insights drawn from a product or service's performance history, businesses can adapt swiftly to changing conditions and address anomalies as they occur.
5. Real-Time Data Analytics
In the fast-paced business environment, real-time data analytics is crucial for staying ahead of the competition. This technique involves the immediate processing and analysis of data as it's collected, enabling organizations to make swift, informed decisions.
Real-time monitoring and reporting allow businesses to react to changes instantly, whether it's adjusting to consumer behavior, managing inventory, or responding to market trends. Here's how real-time data analytics can benefit a company:
- Immediate insight into financial performance
- Enhanced ability to forecast sales
- Optimization of pricing strategies
- Identification of cost-saving opportunities
Real-time data analytics transforms raw data into actionable intelligence, providing a competitive edge by allowing businesses to act quickly on emerging trends and insights.
By integrating real-time data analytics into their operations, companies can not only predict future outcomes but also adjust their strategies on the fly to maximize efficiency and profitability. The agility afforded by this technique is invaluable in today's data-driven business landscape.
In the fast-paced world of technology, staying ahead means leveraging the power of real-time data analytics. At OptimizDBA, we pride ourselves on delivering faster data solutions than anyone else, ensuring that your business stays at the forefront of innovation. Our proprietary methodology guarantees significant performance increases, making us the trusted industry leader since 2001. Don't let your data slow you down. Visit our website to learn how we can optimize your database performance and help you experience transaction speeds that will revolutionize your operations. Let's talk about your challenges and propel your business forward with OptimizDBA!
Conclusion
In conclusion, implementing the top 5 business intelligence techniques discussed in this article can significantly enhance data-driven decision making in organizations. By leveraging business intelligence tools and practices, companies can uncover valuable insights, optimize operations, and gain a competitive edge in today's data-driven landscape. It is essential for businesses to embrace the power of data and utilize BI tools to drive strategic decision-making processes. Embracing these techniques will empower organizations to make informed decisions, improve efficiency, and achieve their business goals effectively.
Frequently Asked Questions
What is Data Mining and how is it used in business intelligence?
Data Mining is the process of discovering patterns and trends in large datasets to extract valuable information. In business intelligence, Data Mining is used to identify insights that can help businesses make informed decisions based on historical data.
What is OLAP and how does it differ from traditional databases?
OLAP, or Online Analytical Processing, is a technology that allows users to interactively analyze multidimensional data from multiple perspectives. Unlike traditional databases that are optimized for transaction processing, OLAP is designed for complex queries and data analysis.
How does Data Visualization enhance data-driven decision making?
Data Visualization involves presenting data in graphical or visual formats to make complex information more accessible and understandable. By using charts, graphs, and dashboards, Data Visualization helps users identify trends, patterns, and outliers in data, leading to better decision making.
What is the role of Predictive Analytics in business intelligence?
Predictive Analytics uses statistical algorithms and machine learning techniques to analyze historical data and predict future outcomes. In business intelligence, Predictive Analytics helps organizations forecast trends, identify risks, and optimize strategies based on predictive insights.
How does Real-Time Data Analytics support agile decision making?
Real-Time Data Analytics processes data streams as they are generated, providing immediate insights for quick decision making. By analyzing data in real-time, businesses can respond to changing market conditions, customer preferences, and operational issues promptly.
What are the key benefits of using Business Intelligence tools in organizations?
Business Intelligence tools offer benefits such as improved decision-making, enhanced data visualization, increased operational efficiency, and better strategic planning. These tools enable organizations to gain valuable insights from data and drive business growth.
How can Business Intelligence tools help in reducing costs and increasing revenue?
Business Intelligence tools help in reducing costs and increasing revenue by optimizing processes, identifying inefficiencies, and uncovering new revenue opportunities. By analyzing data effectively, organizations can make data-driven decisions that lead to cost savings and revenue growth.
What are the common challenges faced when implementing Business Intelligence techniques?
Some common challenges when implementing Business Intelligence techniques include data quality issues, integration complexities, resistance to change from employees, and the need for continuous training and upskilling. Overcoming these challenges is essential for successful BI implementation.