Predictive Modeling in Business Analytics
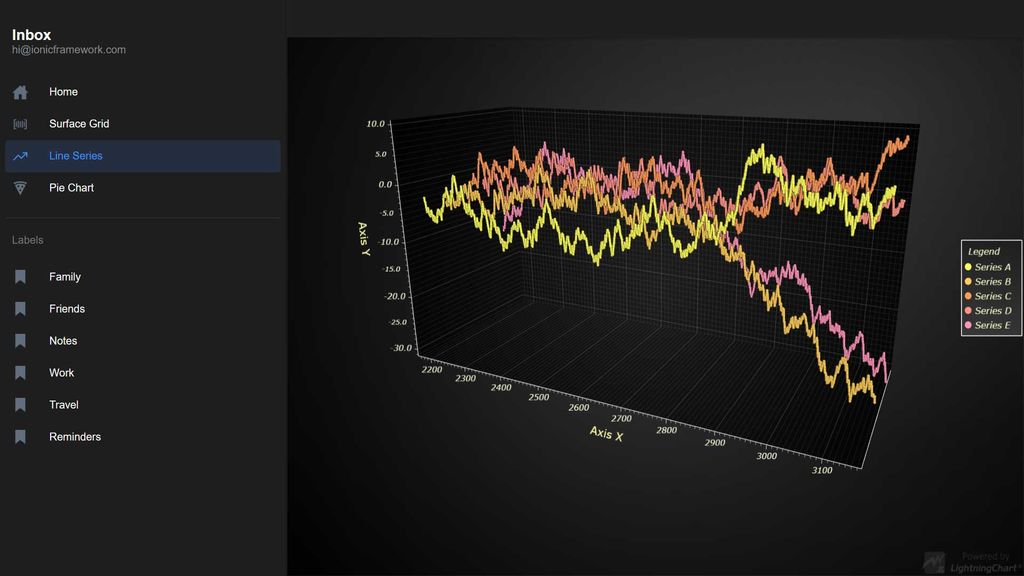
Applications of Predictive Modeling
Predictive modeling harnesses historical data, statistical algorithms, and machine learning to forecast future outcomes, transforming vast amounts of data into actionable insights. Businesses leverage predictive analytics to anticipate market demands, optimize operations, and personalize customer experiences. For instance:
- Customer Retention: Predictive models can forecast churn rates, helping businesses to implement retention strategies proactively.
- Demand Forecasting: By analyzing past sales and market conditions, companies can predict future demand and manage inventory accordingly.
- Fraud Detection: Utilizing patterns in data, predictive modeling aids in identifying potential fraudulent activities, enhancing risk management.
- Marketing Optimization: Insights from predictive analytics guide the allocation of marketing resources for maximum ROI.
Predictive modeling is not just about predicting the future; it's about making informed decisions today for a more successful tomorrow. The ability to accurately forecast and act upon these predictions gives businesses a substantial competitive edge.
Benefits of Predictive Modeling
Predictive modeling stands as a cornerstone in the realm of business intelligence, offering a suite of advantages that can transform data into strategic foresight. Accurate forecasting is perhaps the most significant benefit, allowing businesses to anticipate market trends and customer behavior with a high degree of precision. This leads to more informed decision-making and can significantly reduce risks associated with business planning.
Another key benefit is the operational efficiency gained through the identification of patterns and trends within data. By understanding these insights, companies can optimize processes, reduce waste, and enhance customer experiences. Predictive modeling also facilitates personalized marketing and customer segmentation, tailoring strategies to individual customer needs and thus improving engagement and retention.
The actionable insights provided by predictive modeling not only forecast future trends but also empower businesses to make proactive strategic decisions, leading to optimized marketing campaigns and a stronger competitive edge.
Here's a snapshot of the benefits that predictive modeling brings to businesses:
- Enhanced decision-making capabilities
- Improved operational efficiency
- Increased customer engagement through personalization
- Optimized inventory and demand planning
- Competitive advantage through data-driven strategies
Real-Time Business Intelligence and Decision Making
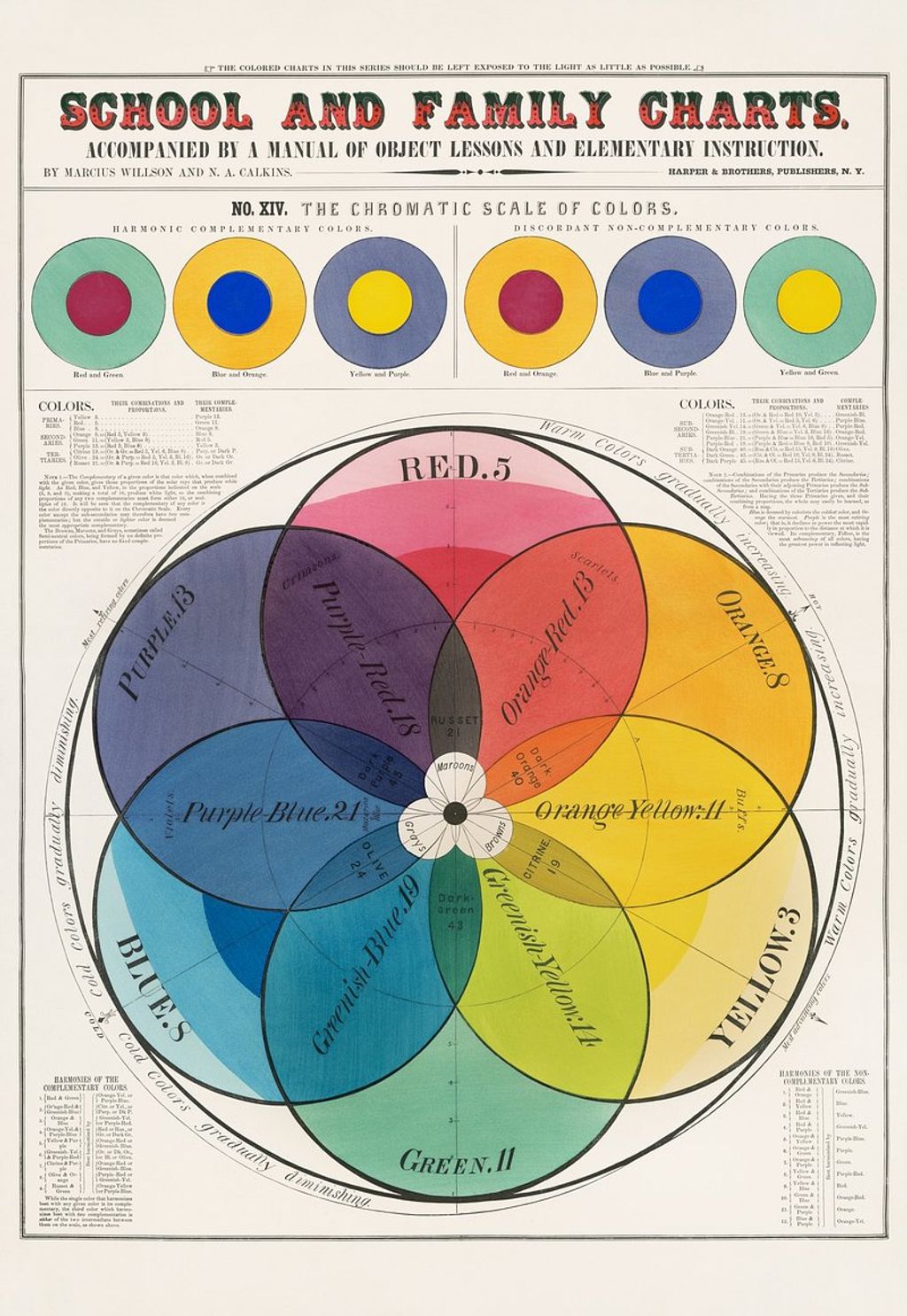
Role of Real-Time Business Intelligence
Real-time Business Intelligence (BI) is transforming the landscape of data-driven decision-making. By leveraging the power of immediate data analysis, organizations can respond to market changes with unprecedented speed. The agility afforded by real-time BI enables businesses to make informed decisions swiftly, often leading to a significant competitive advantage.
Real-time analytics processes data as it is generated, ensuring that the insights are current and actionable. This immediacy is crucial in environments where conditions change rapidly and the window for effective response is narrow.
- Immediate insights: Understand the impact of events as they happen.
- Faster response: Quickly adjust strategies to market dynamics.
- Enhanced customer experience: Provide timely and relevant interactions.
The integration of real-time BI into business operations is not just a technological upgrade but a strategic imperative that can dictate the success or failure of a company in today's fast-paced economy.
Impact on Decision Making
The advent of real-time business intelligence has significantly altered the landscape of decision making. Organizations can now make swift, informed decisions as data flows in, allowing for a more dynamic response to market changes. This immediacy is particularly beneficial in areas such as project management, where predictive analytics enable project managers to anticipate future resource needs and adjust plans accordingly.
Real-time insights also play a crucial role in risk management. AI algorithms enhance the ability to identify and quantify risks, providing a clear prioritization for mitigation efforts. This not only prevents negative outcomes but also fosters a culture of learning and rapid improvement in decision-making processes.
The integration of AI insights in decision making equips businesses with a deeper understanding of market dynamics and consumer behavior, leading to a strategic edge in a competitive market.
The table below illustrates the expected impact of AI on project management, as per a PMI survey:
AI's Impact on Project Management | Percentage of Leaders Agreeing |
---|---|
At least some impact on projects | 91% |
Dramatic improvement in outcomes | Significant majority |
Data Visualization for Enhanced Data Analysis
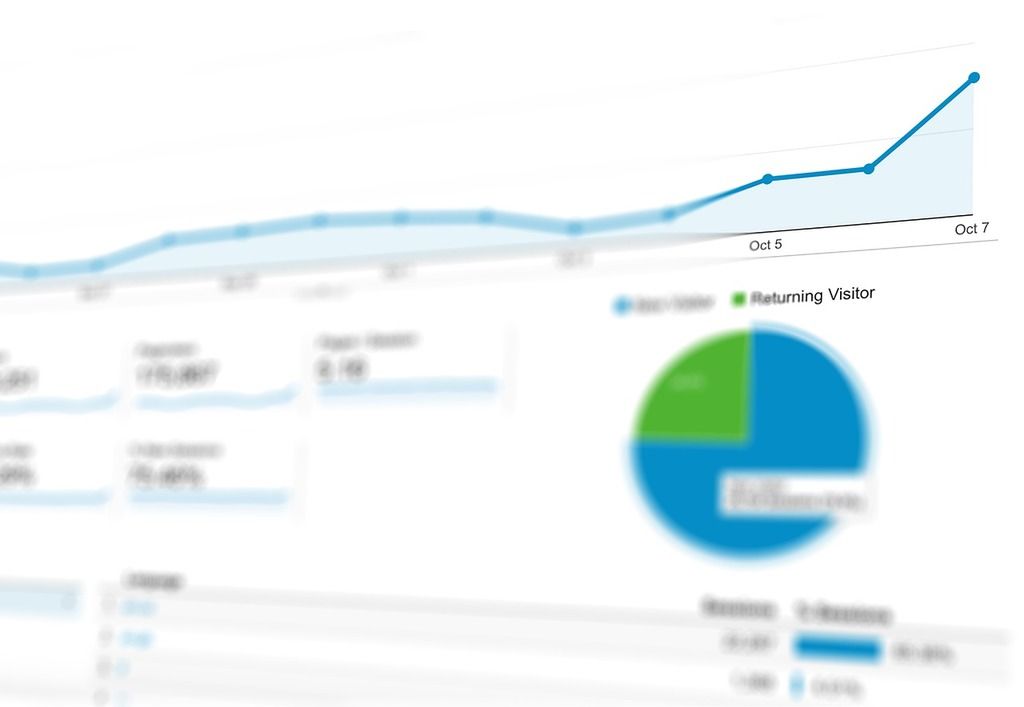
Importance of Data Visualization
In the realm of Business Intelligence (BI), data visualization is a critical component that transforms complex data sets into visual representations, making them easier to understand and analyze. By presenting data in the form of charts, graphs, and maps, businesses can quickly identify trends, outliers, and patterns that might go unnoticed in raw data.
Effective data visualization is not just about presenting data aesthetically; it's about enhancing the cognitive process. It allows stakeholders to grasp difficult concepts or identify new patterns. With the right visualization techniques, data can tell a story and provide insights that drive strategic business decisions.
The ability to visualize data effectively is now a fundamental skill in the toolkit of any data professional. It bridges the gap between data and decision-making, ensuring that insights are not just generated, but are also comprehensible and actionable.
Enhancing Data Patterns
The advent of data visualization tools has significantly improved the ability to enhance data patterns, making complex datasets more comprehensible and actionable. Bold visualization techniques enable businesses to quickly identify trends and anomalies that might otherwise remain hidden in raw data.
- Identification of trends and outliers
- Simplification of complex data for better understanding
- Facilitation of quicker decision-making processes
Data visualization acts as a bridge between raw data and actionable insights, allowing for a more intuitive grasp of the information presented.
While data visualization provides a clear advantage, it is crucial to remember that the effectiveness of these visual tools depends on the quality of the underlying data. Inaccurate or incomplete data sets can lead to misleading visual representations, emphasizing the need for robust data governance practices.
Key Technologies for Business Intelligence and Analytics
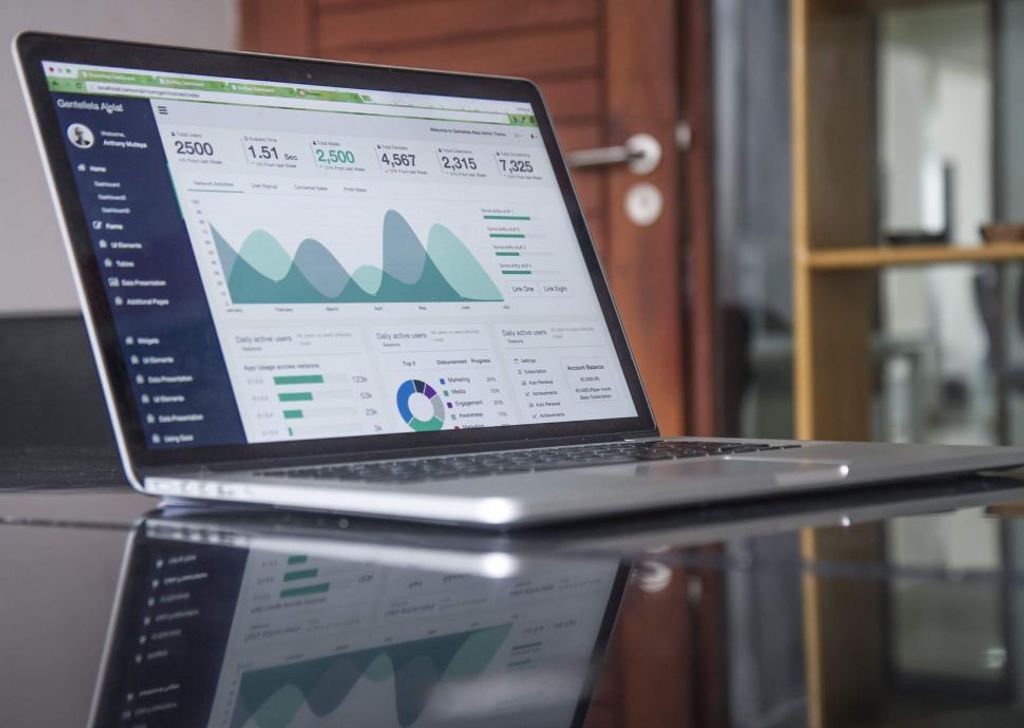
ETL (Extract, Transform, and Load)
ETL, standing for Extract, Transform, and Load, is a foundational process in the realm of business intelligence and analytics. It involves the extraction of data from various sources, transforming it into a format suitable for analysis, and loading it into a target system such as a data warehouse.
The efficiency of the ETL process is critical to ensuring that data is accurate, consistent, and readily available for business insights. ETL tools have evolved to handle complex data structures and large volumes of data, making them indispensable in today's data-driven environment.
- Extract: Data is collected from multiple sources, which can include databases, CRM systems, and cloud storage.
- Transform: Data is cleansed, aggregated, and reformatted to meet the target system's requirements.
- Load: The prepared data is then transferred to the data warehouse or another analytical database.
ETL processes are not just about moving data; they are about transforming raw data into meaningful information that can drive strategic business decisions.
CDC (Change Data Capture)
Change Data Capture (CDC) is an essential technique in the realm of data integration, playing a pivotal role in maintaining up-to-date and accurate data across systems. CDC is designed to identify and capture changes made to data in a source system so that other systems can synchronize and update accordingly. This process is crucial for businesses that rely on real-time data to make informed decisions.
CDC enables organizations to streamline their data processing by ensuring only the changes—inserts, updates, or deletes—are transferred to the target systems. This reduces the volume of data movement and minimizes the load on networks and databases, leading to improved performance and reduced costs.
- Benefits of CDC include:
- Minimized data transfer load
- Real-time data synchronization
- Reduced system downtime
- Enhanced data accuracy
- Improved decision-making capabilities
By leveraging CDC, businesses can achieve a more dynamic and responsive data management strategy, which is essential in today's fast-paced market environment.
Data Deduplication
Data deduplication is a critical process in the realm of business intelligence and analytics. It involves the elimination of redundant data, ensuring that only unique data instances are stored. This technique is not only about saving storage space; it's about maintaining data integrity and improving data quality.
Maximizing storage efficiency and data integrity, data deduplication plays a pivotal role in the overall data management strategy. By removing duplicates, businesses can achieve more accurate analytics and reporting. The process can be applied across various data storage systems, from databases to data warehouses.
Data deduplication is particularly beneficial in environments with significant data replication, such as backup and recovery systems. Here's how it adds value:
- Reduces storage costs by minimizing the amount of data stored
- Enhances data retrieval speeds by decreasing the volume of data to be processed
- Lowers bandwidth requirements for data replication across networks
- Improves data management by simplifying the data landscape
By streamlining data storage and management, organizations can focus their resources on deriving actionable insights from their data, rather than managing excessive data volumes.
Predictive Analytics and Forecasting
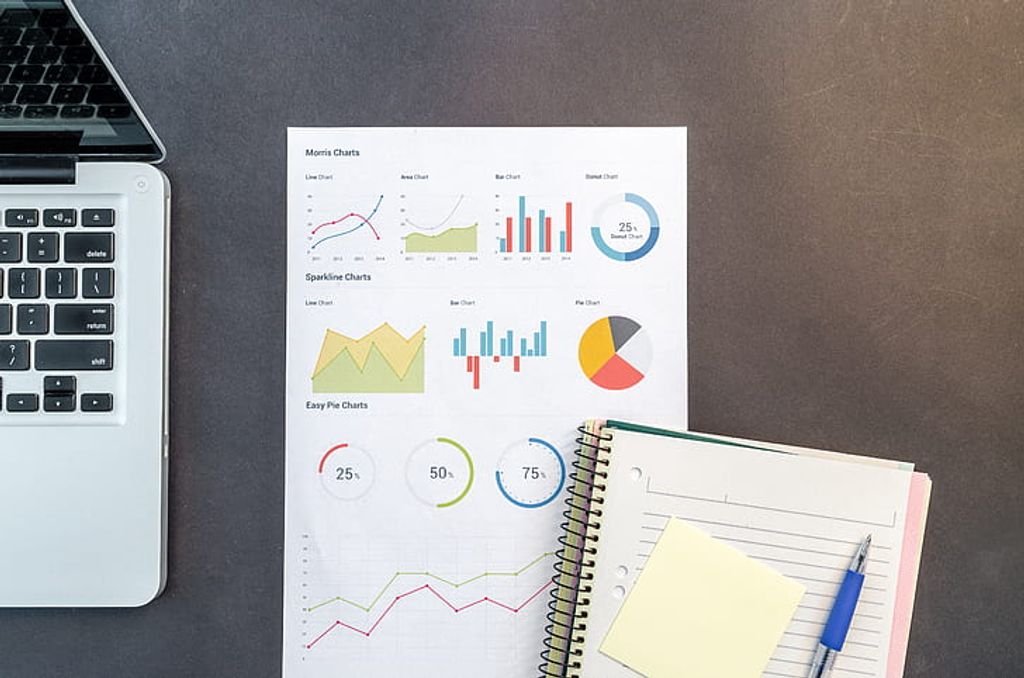
AI's Predictive Analytics Capabilities
Artificial Intelligence (AI) has dramatically transformed the landscape of predictive analytics. AI-powered models excel in analyzing past data, detecting intricate patterns, and forecasting future trends with remarkable precision. This capability is pivotal for businesses that aim to stay ahead of the curve by anticipating market shifts and consumer behavior.
One of the standout features of AI in predictive analytics is its ability to handle complex datasets that traditional statistical methods may struggle with. AI algorithms are adept at uncovering deep linkages and dependencies that can provide businesses with nuanced insights, which are essential for making informed strategic decisions.
- Revenue Estimation: AI predicts future sales based on historical data.
- Market Trend Analysis: Detects emerging patterns in market data.
- Consumer Behavior Prediction: Anticipates changes in consumer preferences.
By leveraging AI's predictive analytics, organizations can make confident strategic judgments, ensuring they are well-prepared for future challenges and opportunities.
Enhanced Decision-Making with AI Insights
In the realm of business intelligence, AI-generated insights are proving to be a game-changer for decision-making. By leveraging the predictive prowess of AI, companies can gain a deeper understanding of market trends, consumer behaviors, and internal operations. This leads to more informed and strategic decisions, ultimately granting businesses a competitive edge in the fast-paced market.
AI's analytical capabilities extend beyond traditional data analysis, offering a comprehensive view that can anticipate future scenarios and guide strategic planning.
The integration of AI into decision-making processes also facilitates the identification of potential blind spots and the evaluation of decisions through AI-driven simulations. These simulations provide a safe environment to test various strategies and avoid potential pitfalls before they impact the business.
Key Benefits of AI-Enhanced Decision-Making:
- Precise revenue forecasting
- Detection of emerging market trends
- Anticipation of consumer behavior
- Strategic planning based on predictive analytics
- Identification and mitigation of decision-making blind spots
As we continue to harness AI for business intelligence, it is imperative for data analysts, researchers, and decision-makers to stay abreast of the latest AI technologies to fully capitalize on these advancements.
Predictive Modeling Applications in Business
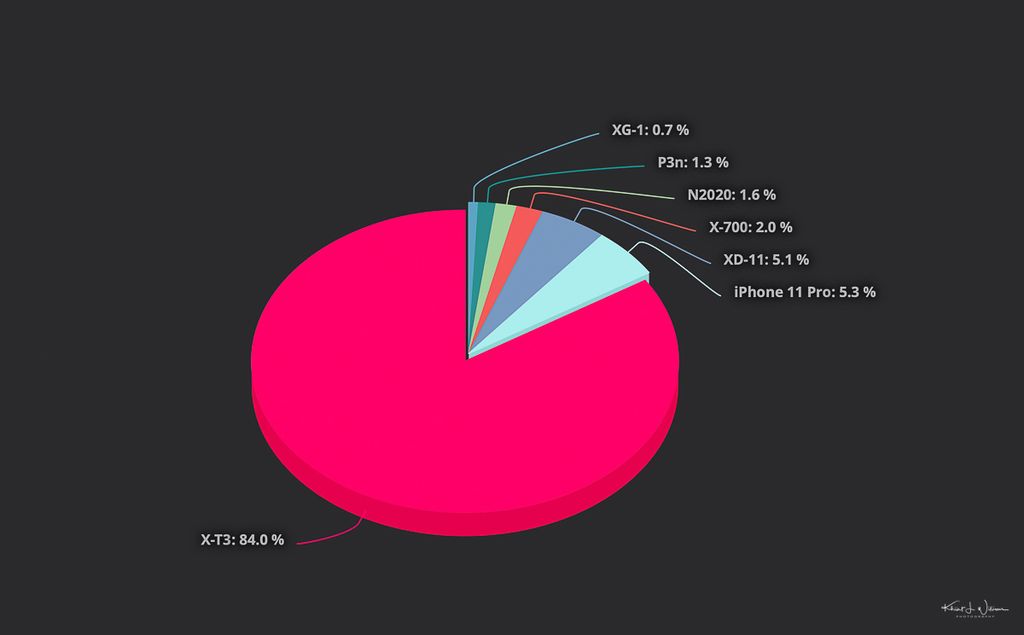
Domains of Predictive Modeling
Predictive modeling is a cornerstone of modern business intelligence, offering insights that drive strategic decisions across various sectors. The versatility of predictive modeling is evident in its wide range of applications, from marketing to finance, and from healthcare to manufacturing. Each domain leverages predictive modeling to enhance decision-making and optimize processes.
Marketing, for instance, uses predictive models to forecast sales trends and customer behaviors, thereby shaping effective strategies. In finance, risk assessment and credit scoring are revolutionized by predictive analytics. The healthcare sector employs these models for patient outcome predictions and resource allocation, while manufacturing benefits from predictive maintenance and demand forecasting.
Predictive modeling transforms raw data into a strategic asset, enabling businesses to anticipate market dynamics and consumer needs with remarkable accuracy.
Here is a brief overview of the domains where predictive modeling is making an impact:
- Marketing: Optimizing marketing mix, forecasting sales, and understanding customer behavior.
- Finance: Credit scoring, risk management, and fraud detection.
- Healthcare: Predicting patient outcomes, managing resources, and personalizing treatment plans.
- Manufacturing: Anticipating equipment failures and streamlining supply chain management.
Benefits of Predictive Modeling in Business
The strategic implementation of predictive modeling in business analytics yields a multitude of benefits. Enhanced decision-making emerges as a pivotal advantage, as predictive models provide a forward-looking perspective, crucial for informed strategic choices. This data-driven approach underpins proactive decision-making, steering businesses away from reliance on intuition or outdated trends.
Another significant benefit is the optimization of operational efficiency. By identifying patterns and trends within historical data, businesses can forecast demand, manage inventory more effectively, and allocate resources with greater precision. This leads to improved ROI and sustained business growth. Moreover, predictive modeling facilitates personalized marketing strategies and customer segmentation, allowing companies to engage with their audience in a more targeted and meaningful way.
Predictive analytics in business offers foresight for effective navigation, forecasting sales, market trends, and customer behavior. It optimizes inventory, pricing, and resource allocation, revolutionizing industries with increased accuracy and risk management.
In summary, the application of predictive modeling in business is not just about anticipating the future; it's about creating a dynamic environment where data informs every strategic move, ensuring that businesses remain agile and competitive in an ever-evolving marketplace.
Conclusion
In conclusion, predictive analytics techniques are revolutionizing business intelligence by enabling accurate forecasting, improved decision-making processes, and driving business growth. From customer analytics to predictive modeling, these technologies have the potential to enhance operational efficiency and provide a competitive advantage in the digital age. Embracing these technologies and leveraging their capabilities will position businesses for success in the evolving landscape of data analysis and business intelligence.
Frequently Asked Questions
What are some examples of predictive modeling in business?
Predictive modeling has various applications in business, including customer retention and churn prediction, demand forecasting and inventory optimization, fraud detection and risk management, personalized marketing and customer segmentation, and equipment maintenance and predictive maintenance.
What are the benefits of predictive modeling?
Predictive modeling offers benefits such as accurate forecasting, improved operational efficiency through identifying patterns and trends, enhanced customer insights for personalized experiences, and gaining a competitive advantage by making data-driven decisions.
What is the role of real-time Business Intelligence?
Real-time Business Intelligence enables faster decision making by providing up-to-date insights and data for immediate action, leading to more agile and responsive business operations.
How does data visualization enhance data patterns?
Data visualization enhances data patterns by presenting complex data in a visual format, making it easier to identify trends, correlations, and outliers, leading to better-informed decision making.
Where can predictive modeling be applied in business analytics?
Predictive modeling finds applications across various business domains, including marketing, finance, healthcare, and manufacturing. It helps businesses make informed decisions, optimize processes, enhance customer experiences, and mitigate risks.
What are the key technologies involved in preparing raw data for Business Intelligence?
The key technologies involved in preparing raw data for Business Intelligence include ETL (Extract, Transform, and Load), CDC (Change Data Capture), and Data Deduplication, which support a wide range of goals within organizations.
What are AI's predictive analytics capabilities?
AI's predictive analytics capabilities enable the analysis of past data, detection of trends, and precise predictions about future outcomes, enhancing decision-making processes by estimating revenue, identifying market trends, and anticipating consumer behavior.
What are the domains of predictive modeling in business?
Predictive modeling finds applications across various business domains, including marketing, finance, healthcare, and manufacturing. It helps businesses make informed decisions, optimize processes, enhance customer experiences, and mitigate risks.