Driving Business Decisions with Predictive Analytics
Overview
Introduction
Predictive analytics is a powerful tool that allows businesses to make data-driven decisions. By analyzing historical data and using statistical algorithms, businesses can forecast future outcomes and identify trends and patterns. This enables them to anticipate customer behavior, optimize operations, and gain a competitive edge in the market. One of the key aspects of predictive analytics is indexing, which involves organizing and structuring data in a way that allows for efficient retrieval and analysis. With proper indexing, businesses can quickly access and process large volumes of data, enabling faster decision-making and improved business performance.
Importance of Predictive Analytics
Predictive analytics is a powerful tool that enables businesses to make informed decisions based on data-driven insights. By analyzing historical data and using advanced algorithms, businesses can identify patterns, trends, and relationships that can help them predict future outcomes. This allows businesses to anticipate customer behavior, optimize operations, and mitigate risks. Analytics tools play a crucial role in the process, providing the necessary capabilities to collect, analyze, and visualize data. These tools enable businesses to uncover hidden patterns and insights that can drive strategic decision-making.
Key Components
The success of predictive analytics in driving business decisions relies on several key components. These components include data collection and preparation, model development and evaluation, and business impact and implementation. Data collection and preparation involves sourcing relevant data from various internal and external sources, cleaning the data to ensure accuracy and consistency, and feature engineering to create meaningful variables for analysis. Model development and evaluation focuses on selecting the most appropriate predictive models, training and testing these models using historical data, and evaluating their performance using various performance metrics. Finally, the business impact and implementation phase involves using predictive analytics to provide decision support for strategic and operational decision-making, integrating predictive models into day-to-day operations for operational integration, and ultimately unlocking the potential of predictive analytics to drive business growth and success.
Data Collection and Preparation
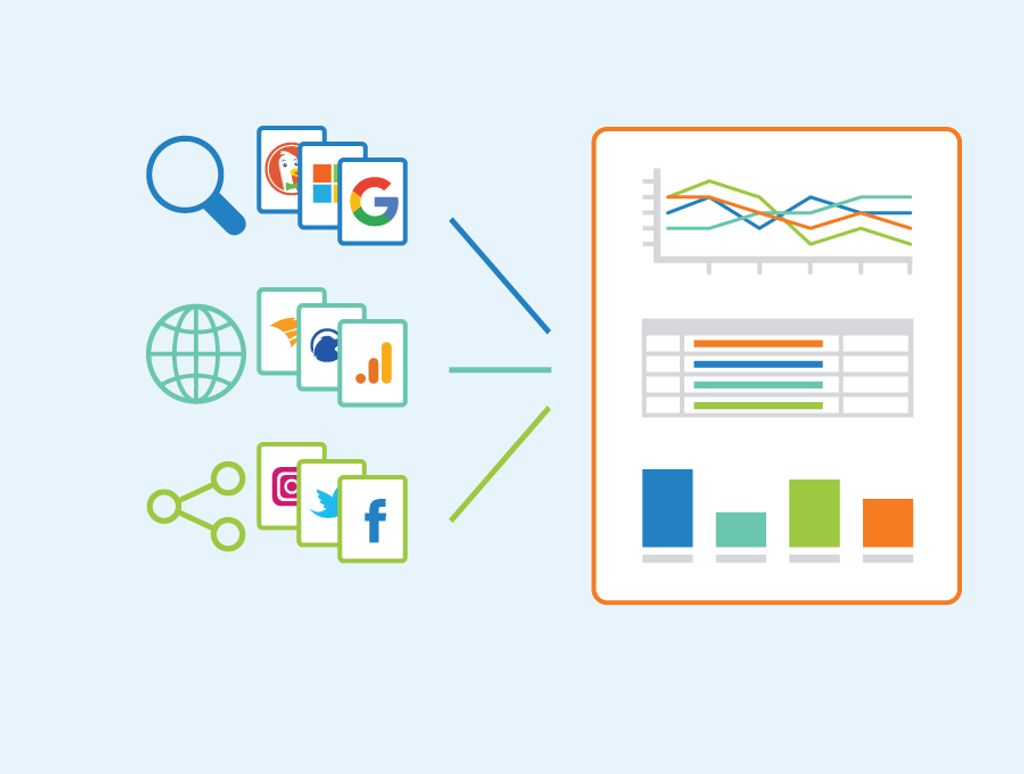
Data Sourcing
Data sourcing is the first step in the predictive analytics process. It involves identifying and collecting relevant data from various sources such as databases, APIs, and external data providers. This data is then used to build predictive models that can drive business decisions. In order to ensure the accuracy and reliability of the data, it is important to verify its quality, completeness, and consistency. This can be done through data profiling and data cleansing techniques. Data sourcing is a crucial component of the predictive analytics workflow as it lays the foundation for accurate and meaningful insights.
Data Cleaning
Data cleaning is a crucial step in the data preparation process. It involves identifying and correcting or removing inaccurate or incomplete data from the dataset. This ensures that the data used for analysis and modeling is of high quality and reliable. Data cleaning techniques include removing duplicates, handling missing values, and outlier detection. Additionally, query optimization is performed to improve the efficiency of data retrieval and processing. By cleaning the data, organizations can reduce the risk of making decisions based on faulty or misleading information.
Feature Engineering
Feature engineering is a crucial step in the predictive analytics process. It involves transforming raw data into a format that is suitable for model development. This step includes selecting relevant features, creating new features through mathematical transformations, and encoding categorical variables. Feature engineering plays a vital role in improving the performance of predictive models by enhancing the representation of the data. It helps in capturing important patterns and relationships that might not be apparent in the raw data. By leveraging feature engineering techniques, businesses can extract valuable insights from their databases and make more accurate predictions.
Model Development and Evaluation
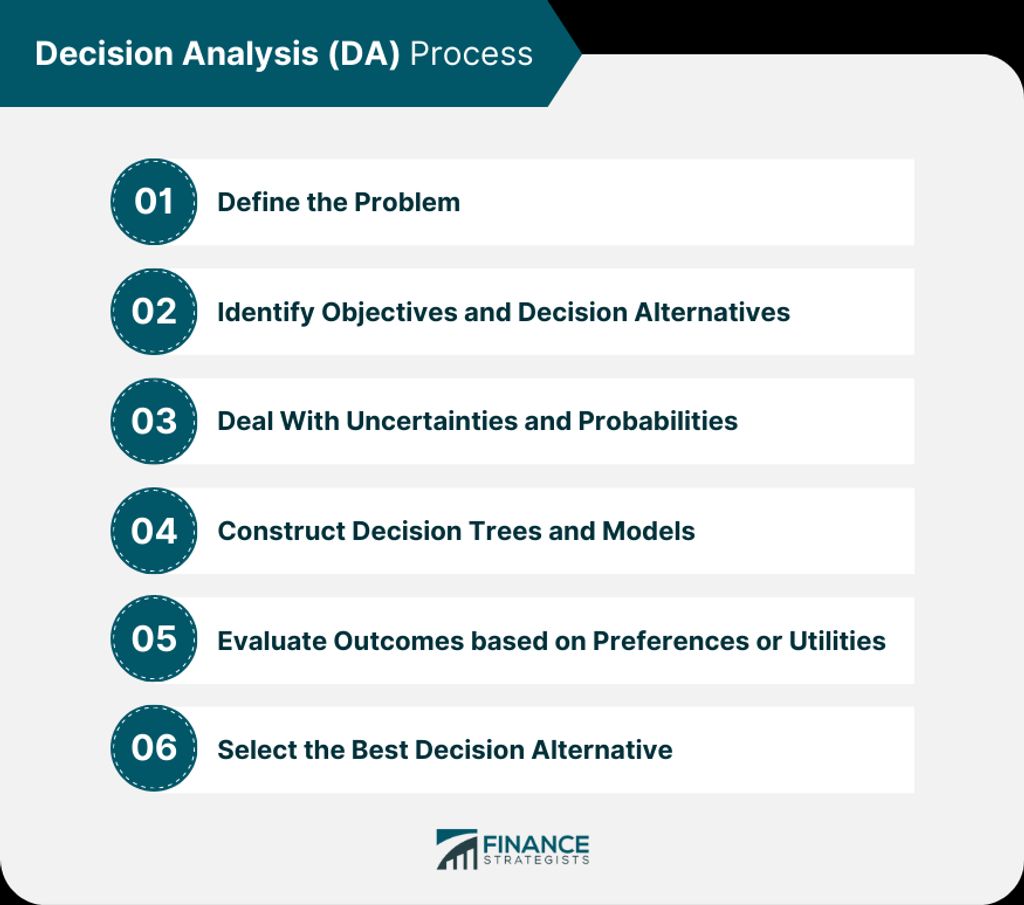
Model Selection
Once the data has been prepared, the next step in the predictive analytics process is model selection. Model selection involves choosing the most appropriate algorithm or technique to build the predictive model. There are various factors to consider when selecting a model, such as the type of data, the problem at hand, and the desired outcome. It is important to evaluate different models and compare their performance using various evaluation metrics. The chosen model should be able to accurately predict the target variable and provide valuable insights for driving business decisions. In the context of AI in software delivery, model selection plays a crucial role in optimizing software development processes and improving delivery efficiency.
Training and Testing
Once the predictive model has been selected, it is crucial to train and test it using appropriate data sets. Training involves feeding the model with historical data to learn patterns and relationships. This allows the model to make accurate predictions. Testing is done using a separate set of data to evaluate the model's performance and assess its ability to generalize to new data. The success of the model depends on how well it performs during testing, as it indicates its reliability and effectiveness in making predictions.
Performance Metrics
Once the predictive model has been trained and tested, it is crucial to evaluate its performance using various metrics. These metrics provide insights into how well the model is performing and help in understanding its strengths and weaknesses. Some commonly used performance metrics include accuracy, precision, recall, and F1 score. Accuracy measures the overall correctness of the model's predictions, while precision measures the proportion of true positives among all positive predictions. Recall, on the other hand, measures the proportion of true positives that are correctly identified by the model. The F1 score is a combination of precision and recall and provides a balanced measure of the model's performance. By analyzing these metrics, stakeholders can make informed decisions about the model's effectiveness and suitability for the business. It is important to note that the choice of performance metrics may vary depending on the specific problem and the business's objectives.
Business Impact and Implementation
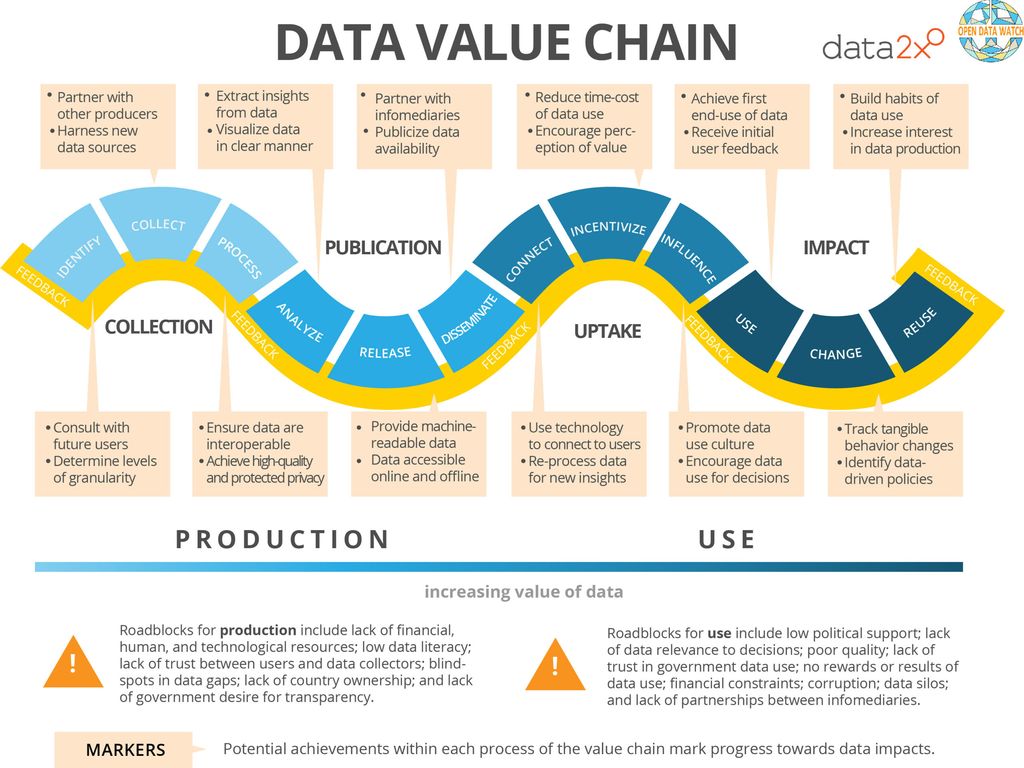
Decision Support
Once the predictive analytics model is developed and evaluated, it becomes a powerful tool for decision support. Innovation is a key aspect of driving business decisions, and predictive analytics provides the necessary insights to identify new opportunities and make informed choices. By leveraging the power of data and advanced algorithms, businesses can gain a competitive edge and stay ahead of the curve. The model's predictions and recommendations can guide strategic planning, resource allocation, and risk management. With the support of predictive analytics, businesses can make data-driven decisions that align with their goals and drive growth.
Operational Integration
Operational integration is a crucial step in the successful implementation of predictive analytics. It involves transforming the insights gained from the predictive models into actionable strategies and incorporating them into the day-to-day operations of the business. This requires close collaboration between data scientists, business analysts, and operational teams to ensure that the predictive analytics solutions are seamlessly integrated into existing systems and processes. By integrating predictive analytics into operations, businesses can make data-driven decisions in real-time, optimize resource allocation, and improve overall efficiency and profitability.
Conclusion
In conclusion, predictive analytics is a powerful tool that can drive business decisions and provide valuable insights into future trends and patterns. By leveraging data collection and preparation techniques, businesses can ensure the accuracy and reliability of their predictive models. Model development and evaluation allow businesses to select the most appropriate model and assess its performance. Finally, by integrating predictive analytics into decision-making processes, businesses can make informed choices that have a positive impact on their operations. The future holds immense potential for predictive analytics to revolutionize the way businesses operate and make decisions.
The Business Impact and Implementation section of OptimizDBA Database Optimization Consulting focuses on delivering significant performance improvements for your database systems. With our expertise and industry-leading remote DBA services, we guarantee transaction speeds that are at least twice as fast as before. In fact, our average speeds often reach 100 times, 1000 times, or even higher, ensuring a remarkable increase in performance. Since 2001, we have been a trusted leader in the industry, serving over 500 clients with our exceptional database optimization solutions. Experience the power of optimized databases with OptimizDBA today!