Driving Data-Driven Insights: Exploring the World of Analytics
Overview
What is Analytics?
Analytics is the process of analyzing and interpreting data to gain insights and make informed decisions. It involves the use of mathematical models and statistical techniques to uncover patterns, trends, and relationships within the data. Analytics helps organizations understand their customers, optimize their operations, and drive business growth. It encompasses various disciplines such as data mining, data visualization, and predictive analytics. By leveraging analytics, businesses can identify opportunities, mitigate risks, and improve their overall performance.
Importance of Analytics
Analytics plays a crucial role in driving business transformation. By leveraging data and applying advanced analytical techniques, organizations can gain valuable insights into their operations, customers, and market trends. These insights enable informed decision-making, identify opportunities for growth, and optimize business processes. Analytics also helps in improving operational efficiency, reducing costs, and mitigating risks. In today's data-driven world, organizations that embrace analytics have a competitive advantage and are better positioned to adapt to changing market dynamics.
Types of Analytics
There are various types of analytics that organizations can leverage to gain valuable insights from their data. These include descriptive analytics, which focuses on summarizing and interpreting historical data to understand past trends and patterns. DBMS analytics, on the other hand, involves analyzing data stored in a database management system to uncover relationships and make informed decisions. Another type is predictive analytics, which uses statistical models and algorithms to forecast future outcomes based on historical data. Lastly, prescriptive analytics goes beyond predictions and provides recommendations on the best course of action to achieve desired outcomes. Each type of analytics has its own unique benefits and applications, and organizations often use a combination of these approaches to drive data-driven insights.
Data Collection
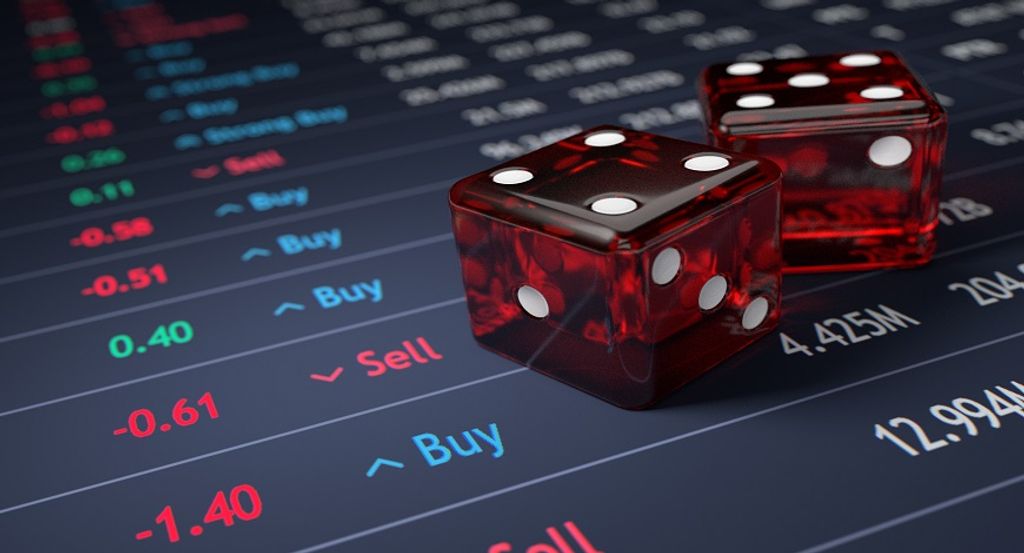
Sources of Data
There are various sources of data that organizations can collect and analyze. These sources include databases, websites, social media platforms, IoT devices, and third-party data providers. For example, organizations can collect data from their own MariaDB databases, which store structured data in a relational format. They can also gather data from websites and social media platforms through web scraping and API integration. Additionally, IoT devices such as sensors and smart devices generate vast amounts of data that can be utilized for analysis. Moreover, organizations can obtain external data from third-party providers to enrich their datasets.
Data Collection Methods
Data collection methods are the techniques and processes used to gather data for analysis. There are various methods available, including surveys, interviews, observations, and data mining. Each method has its strengths and weaknesses, and the choice of method depends on the nature of the data and the research objectives. Surveys involve collecting data through questionnaires or interviews, allowing researchers to gather information directly from individuals. Interviews provide more in-depth insights but are time-consuming and require skilled interviewers. Observations involve systematically watching and recording behavior in real-world settings. Data mining involves extracting patterns and insights from large datasets using computational algorithms. It is particularly useful in uncovering hidden patterns and relationships in complex data. Choosing the right data collection method is crucial to ensure the accuracy and reliability of the data collected.
Data Quality Assurance
Data quality assurance is a critical step in the data analysis process. It involves ensuring that the collected data is accurate, reliable, and consistent. Query optimization is an important aspect of data quality assurance, as it focuses on improving the performance and efficiency of database queries. By optimizing queries, organizations can reduce the time and resources required for data analysis, allowing for faster and more accurate insights. Additionally, query optimization helps to identify and resolve any potential issues or bottlenecks in the data collection and analysis process.
Data Analysis
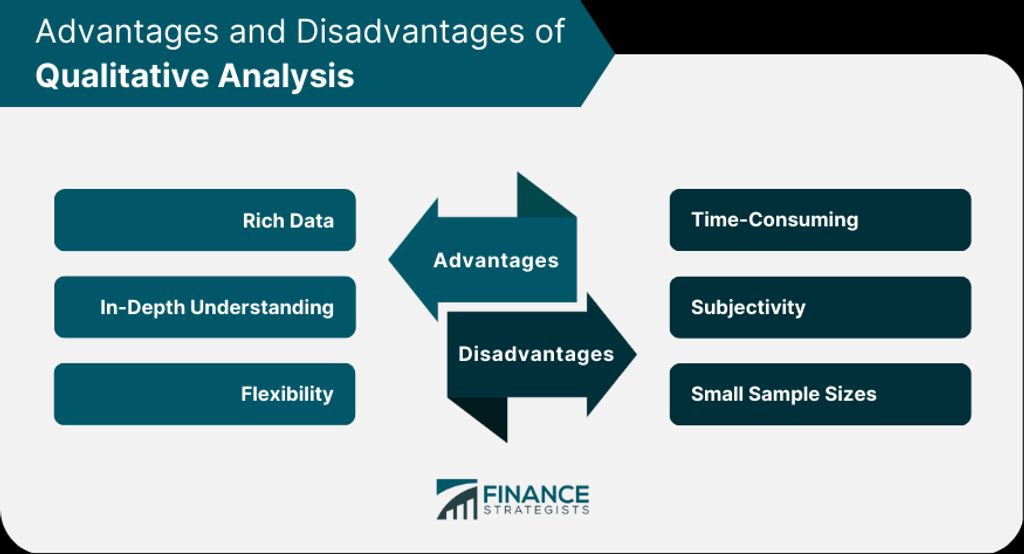
Exploratory Data Analysis
Exploratory Data Analysis (EDA) is a crucial step in the analytics process. It involves analyzing and visualizing data to gain insights and understand the underlying patterns. EDA helps in identifying trends, outliers, and relationships within the data. By using various techniques such as summary statistics, data visualization, and correlation analysis, analysts can uncover valuable information that can drive decision-making. EDA plays a significant role in identifying potential areas for further analysis and helps in formulating hypotheses for statistical testing. It provides a foundation for the subsequent stages of data analysis.
Statistical Analysis
Statistical analysis is a crucial step in the analytics process. It involves the use of statistical methods to analyze and interpret data. Data-driven insights are derived from statistical analysis, which allows organizations to make informed decisions based on the patterns and trends observed in the data. This analysis helps identify relationships between variables, uncover hidden patterns, and make predictions. Some common techniques used in statistical analysis include hypothesis testing, regression analysis, and analysis of variance (ANOVA). By applying these techniques, organizations can gain valuable insights into their data and drive data-driven decision-making.
Predictive Modeling
Predictive modeling is a powerful technique used in analytics to forecast future outcomes based on historical data. It involves the development of mathematical models that can predict trends and patterns in the data. This process utilizes various statistical algorithms and machine learning techniques to analyze the data and make predictions. By leveraging predictive modeling, organizations can make informed decisions and take proactive measures to optimize their operations and achieve their goals. It is important to note that predictive modeling relies heavily on accurate and reliable data. Therefore, it is crucial to follow database best practices to ensure data quality and integrity.
Conclusion
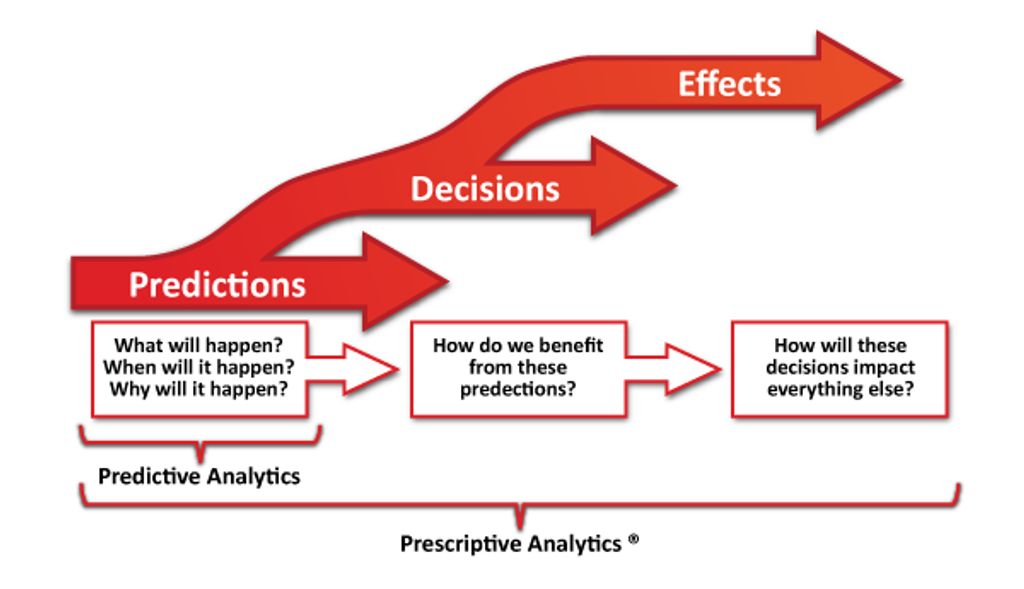
Benefits of Analytics
Analytics provides numerous benefits to organizations. Data management is one of the key advantages of analytics. It allows businesses to collect, store, and analyze large volumes of data efficiently. With effective data management, organizations can make informed decisions, identify patterns and trends, and gain valuable insights. By leveraging analytics, businesses can improve operational efficiency, optimize processes, and enhance customer experiences. Additionally, analytics enables organizations to identify and mitigate risks, detect fraud, and improve overall performance.
Challenges in Analytics
While analytics offers numerous benefits, it also presents several challenges that organizations need to address. One of the key challenges is data privacy and security. With the increasing amount of data being collected and analyzed, ensuring the privacy and security of this data becomes crucial. Organizations need to implement robust measures to protect sensitive information and comply with data protection regulations. Another challenge is data quality. Inaccurate or incomplete data can lead to flawed analysis and unreliable insights. Organizations must invest in data quality assurance processes to ensure the accuracy and reliability of their data. Additionally, the rapid pace of technological advancements poses a challenge in analytics. As technology continues to evolve, organizations need to keep up with the latest tools and techniques to effectively analyze and interpret the data. By overcoming these challenges, organizations can harness the power of analytics to drive informed decision-making and gain a competitive edge.
Future of Analytics
The future of analytics is promising, with the increasing emphasis on digital transformation. As businesses continue to adopt advanced technologies and digitize their operations, the amount of data generated is growing exponentially. This data provides valuable insights that can drive strategic decision-making and improve business performance. However, with the growing volume and complexity of data, there are also challenges to overcome. Organizations need to invest in data management and analytics capabilities to effectively harness the power of data. Additionally, advancements in artificial intelligence and machine learning are expected to further enhance analytics capabilities, enabling more accurate predictions and automated decision-making. The future of analytics holds great potential for organizations to gain a competitive edge and drive innovation.
In conclusion, OptimizDBA Database Optimization Consulting is the go-to solution for improving your database performance. With over 20 years of experience, we have helped over 500 clients achieve transaction speeds that are at least twice as fast as before. Our average speeds are often 100 times, 1000 times, or even higher! We guarantee a significant increase in performance. If you're looking to optimize your database and experience unparalleled speed, contact us today. Visit our website to learn more about our remote DBA services and how we can help you achieve optimal performance.