The Rise of Augmented Analytics
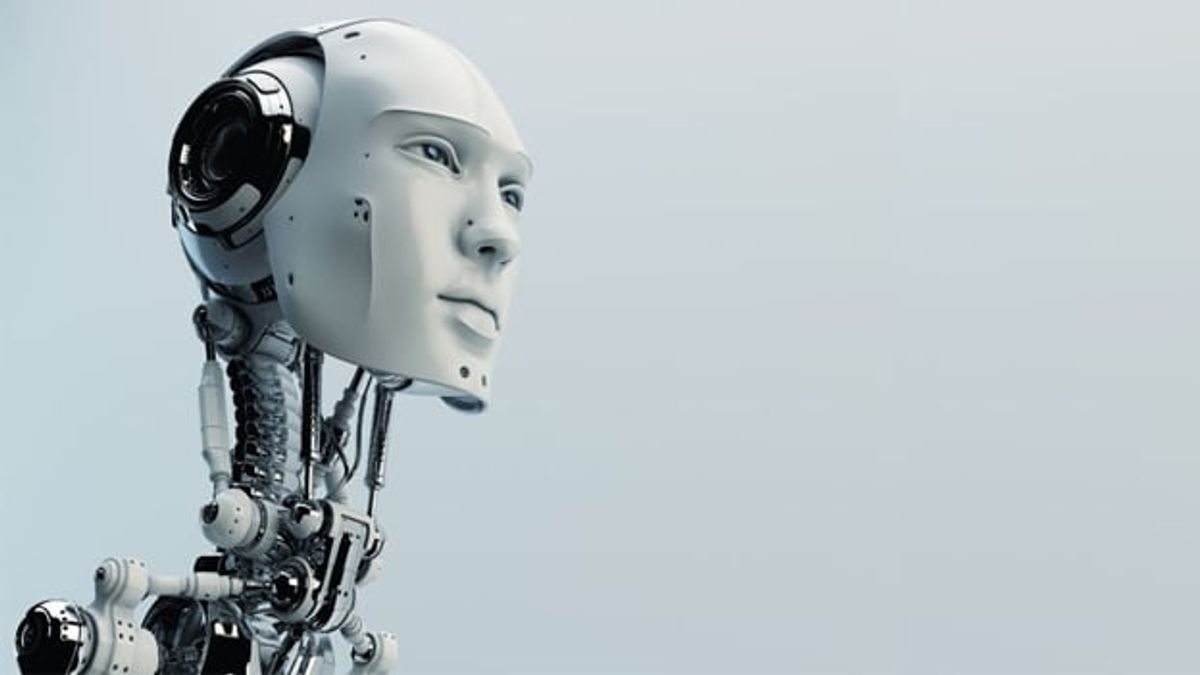
Defining Augmented Analytics
Augmented analytics represents a paradigm shift in how data is analyzed and utilized within organizations. It leverages advanced technologies such as machine learning, artificial intelligence (AI), and natural language processing (NLP) to extend the capabilities of traditional analytics. This innovative approach automates the labor-intensive aspects of data analysis, including data preparation and insight discovery, making it more accessible to a broader range of users.
Augmented analytics democratizes the data analysis process, enabling users without deep technical expertise to generate meaningful insights.
The key benefits of augmented analytics include:
- Automation of routine data tasks, freeing up human analysts for more complex analysis
- Enhanced data sharing and collaboration across departments
- Accelerated insight discovery, leading to faster decision-making
By simplifying the interaction with data, augmented analytics tools empower businesses of all sizes to harness the power of their data without the need for extensive data science knowledge.
Impact on Decision-Making Processes
Augmented analytics is revolutionizing the way organizations approach decision-making. By harnessing AI to automate the discovery of insights, businesses can now focus more on strategic initiatives and less on the manual aspects of data analysis. The automation of these processes frees up valuable time for decision-makers, allowing for swift, well-informed decisions that optimize business outcomes.
In the realm of data-driven decision-making, the ability to quickly interpret vast amounts of structured and unstructured data is crucial. Augmented analytics facilitates this by enabling:
- Collection and processing of data
- Analysis to identify patterns and trends
- Interpretation to inform strategic initiatives
The incorporation of NLP enhances the decision-making process by simplifying the extraction of insights, thus expediting strategy formulation.
As a result, organizations are empowered at all levels to make informed decisions that are aligned with their strategic goals, driving business success in today's competitive landscape.
Case Studies: Augmented Analytics in Practice
The advent of augmented analytics has democratized data analysis, enabling businesses of all sizes to harness the power of AI and NLP for data-driven insights. Businesses are now empowered to automate data preparation and analysis, making strategic decisions more accessible to non-technical users.
- Retail companies leverage real-time analytics to adjust inventory and pricing dynamically.
- Healthcare providers utilize predictive analytics to improve patient care and operational efficiency.
- Manufacturers integrate edge computing to optimize production processes.
- Financial institutions analyze large datasets for risk assessment and fraud detection.
Augmented analytics tools are not only transforming how data is analyzed but also reshaping entire industries by providing immediate action and informed decisions.
SQL's role in structuring and querying data remains crucial, as it underpins the ability to extract meaningful insights from vast amounts of information. The following table illustrates the impact of augmented analytics across various sectors:
Sector | Benefit |
---|---|
Retail | Dynamic inventory management |
Healthcare | Enhanced patient outcomes |
Manufacturing | Increased production efficiency |
Finance | Improved risk and fraud analysis |
The Evolution of Business Analytics
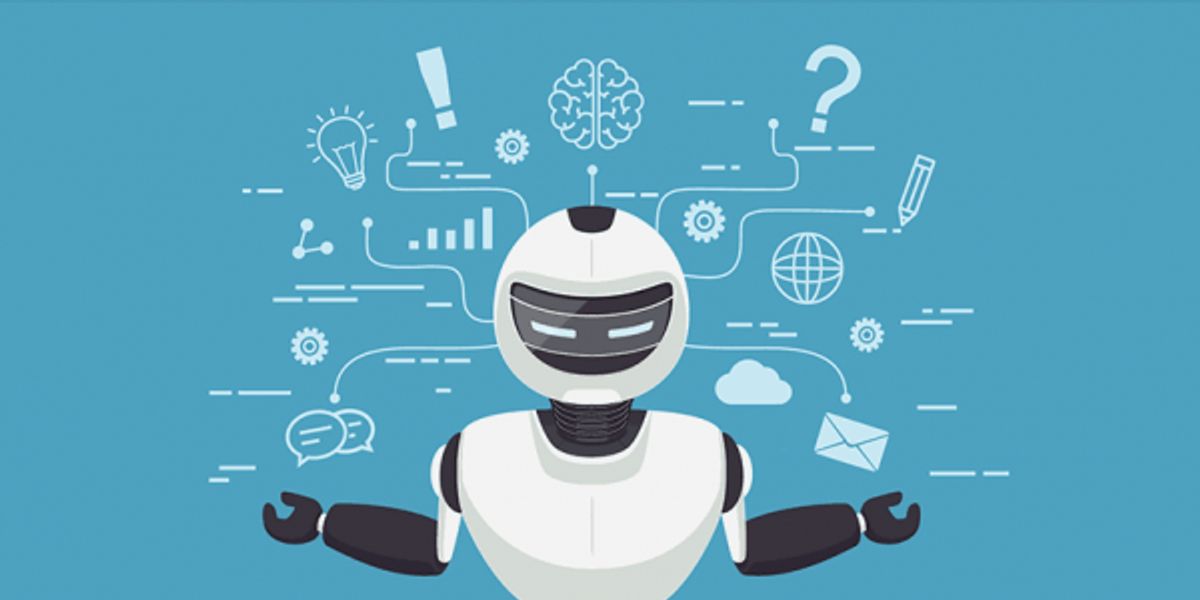
From Descriptive to Predictive Analytics
The transition from descriptive to predictive analytics marks a significant shift in the business intelligence landscape. Descriptive analytics, which focuses on summarizing past data to understand what has happened, is now being augmented by predictive analytics. This newer form of analytics harnesses statistical models and machine learning algorithms to forecast future trends and behaviors.
Predictive analytics has become increasingly sophisticated, integrating technologies such as deep learning to provide more accurate forecasts. By analyzing both current and historical datasets, businesses can anticipate future outcomes, enabling proactive decision-making. For example, a retail executive might use predictive analytics to determine the potential sales of a product line, based on past performance and market trends.
Predictive analytics not only answers the question of "What might happen?" but also empowers organizations to prepare for those possibilities, turning insights into actionable strategies.
The synergy between descriptive and predictive analytics is pivotal for driving business success. It enhances informed decision-making by providing a more comprehensive view of data. Below are the key characteristics of predictive analytics:
- Statistical models and machine learning algorithms
- Identification of patterns and trends in historical data
- Forecasting of future events or behaviors
The Shift to Real-Time Data Analysis
The landscape of business analytics is undergoing a significant transformation with the shift towards real-time data analysis. This evolution marks a departure from traditional methods that relied heavily on historical data, moving towards an approach that emphasizes the immediacy and dynamism of real-time insights.
Real-time analytics is poised to become a fundamental aspect for businesses aiming to secure a strategic edge. The capability to analyze data as it unfolds enables organizations to make prompt decisions, a vital advantage in sectors like finance, e-commerce, and healthcare.
The integration of AI and automation with real-time data access is revolutionizing decision-making processes, making BI tools indispensable for data democratization.
The following table illustrates the contrast between traditional and real-time analytics:
Aspect | Traditional Analytics | Real-Time Analytics |
---|---|---|
Data Timeliness | Historical | Immediate |
Decision Speed | Delayed | Instant |
Industry Application | Static Industries | Dynamic Industries |
Embracing real-time analytics allows for a more agile and responsive business model, where decisions are informed by the latest data streams, rather than outdated reports.
The Integration of AI and Machine Learning
The integration of Artificial Intelligence (AI) and Machine Learning (ML) into business analytics heralds a new era of insight discovery. Organizations are now capable of analyzing complex datasets with unprecedented precision and speed. This integration is not just about automating tasks; it's about enhancing the cognitive capabilities of analytics tools to provide deeper, actionable insights.
- AI algorithms are becoming increasingly sophisticated, enabling predictive analytics that can forecast trends and behaviors.
- ML models learn from data over time, improving their accuracy and the quality of insights derived.
- The automation of decision-making processes is becoming more prevalent, allowing businesses to respond to market changes swiftly.
The synergy between AI and ML is transforming data analytics into a more intelligent, adaptive, and automated discipline, which is crucial for maintaining competitive advantage in today's fast-paced business environment.
Data-Driven Digital Transformation
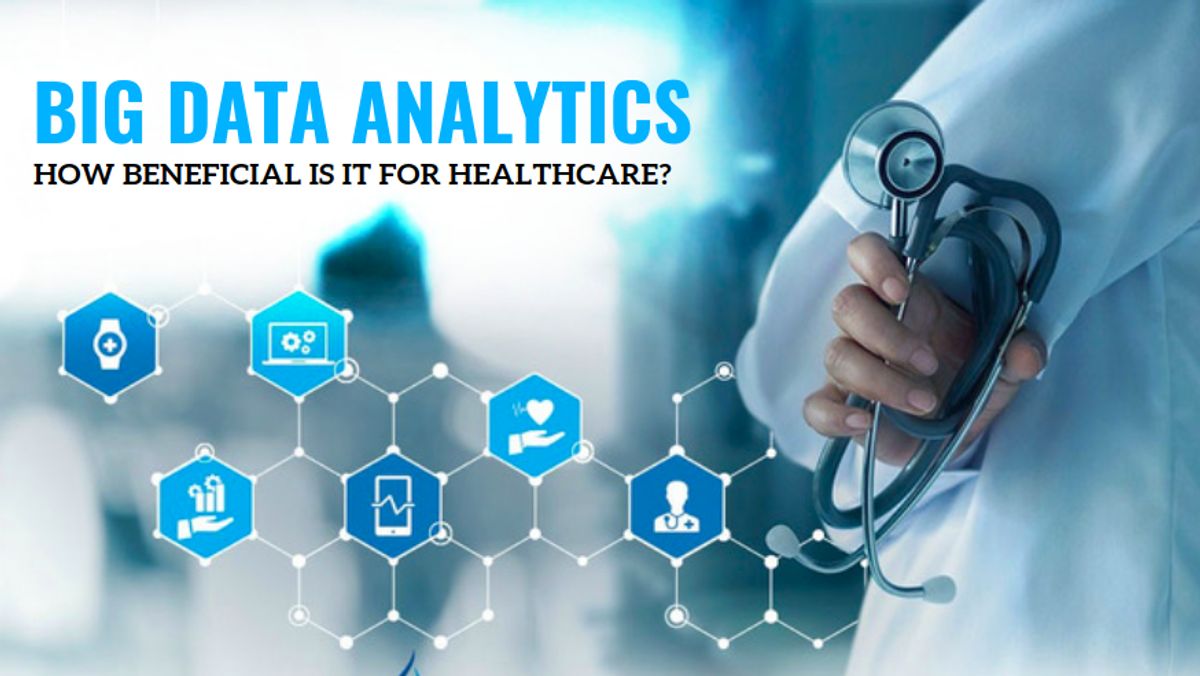
The Role of Data in Process Optimization
In the realm of business process management (BPM), data is the cornerstone of process optimization. By harnessing the insights gleaned from data analytics, organizations can refine their operations, leading to enhanced efficiency and achieving their business goals. The journey to optimization involves a strategic blend of high-quality data and robust analytical methods.
Consider the transformational impact of data analytics on a retail business. Initially, descriptive analytics might be used to review historical sales data, identifying trends in product popularity. However, with the advent of prescriptive analytics, the same data can guide decisions on inventory management during peak seasons, ensuring optimal stock levels.
The integration of automation and machine learning is revolutionizing the way businesses handle and interpret vast amounts of data. This evolution is not just about managing data more effectively; it's about leveraging it to gain a competitive edge and enhance customer experiences. As we look to the future, technologies like augmented reality will further empower workers with real-time data visualization, driving innovation and productivity in sectors such as manufacturing.
The ultimate goal is to transform raw data into actionable insights that propel business forward, turning challenges into opportunities for growth and innovation.
Predictive Modeling and Business Strategy
Predictive modeling has become a cornerstone of modern business strategy, enabling organizations to anticipate market trends and customer behavior with remarkable accuracy. By leveraging historical data and advanced analytics, companies can forecast future scenarios and make strategic decisions that are both informed and proactive.
Statistical models and machine learning algorithms form the backbone of predictive analytics, transforming raw data into actionable insights. This analytical prowess is not just about predicting outcomes; it's about shaping them to align with business objectives.
- Improved Sales Forecasting: Predictive models offer a robust method for forecasting sales based on historical data, market conditions, and marketing inputs.
- Scenario Planning: Businesses can evaluate various future scenarios and their potential impacts, allowing for strategic adjustments in real time.
- Risk Mitigation: Identifying potential risks before they materialize helps companies to devise contingency plans and reduce vulnerabilities.
Predictive analytics is not a crystal ball, but it provides the next best thing: a data-driven glimpse into the future, empowering businesses to act rather than react.
Automation in Data Management
The integration of automation in data management is a game-changer for businesses. Big data utilization and automation advancements are transforming business operations, enabling data-driven decisions and enhancing productivity. By automating routine tasks, companies can allocate more resources to strategic analysis and innovation.
Automation paired with advanced analytics is streamlining the data lifecycle from collection to insight generation. This shift not only improves accuracy but also significantly reduces the time taken to derive valuable business insights.
Here are some ways automation is revolutionizing data management:
- Automated data cleansing and preparation
- Real-time data monitoring and alerts
- Advanced systems for predictive analytics
- Self-service data platforms for non-technical users
The automation of data processes is not just about efficiency; it represents a paradigm shift in strategic business management. Decision-makers are now equipped with quicker, more accurate insights, allowing for swift and well-informed decisions that optimize business outcomes.
The Expanding Universe of Business Analytics
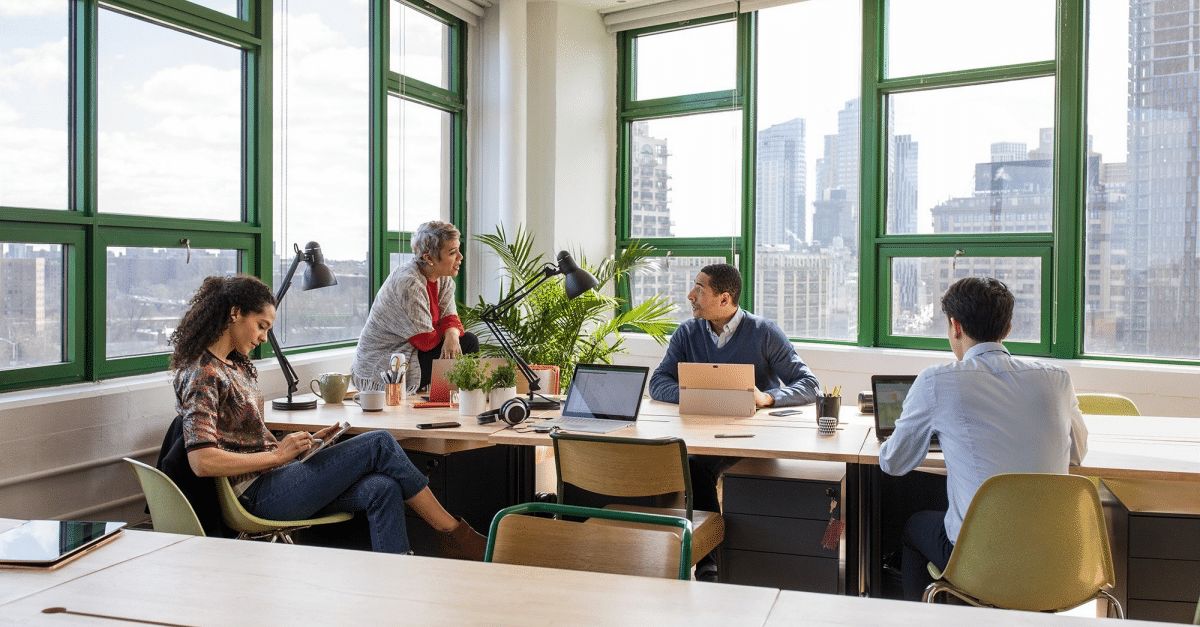
Descriptive to Prescriptive: The Analytics Spectrum
The journey from descriptive to prescriptive analytics represents a significant leap in the capability of businesses to not just understand, but to shape their future. Descriptive analytics, the cornerstone of Data Analytics, provides a historical view of what has happened in the business. It's akin to reading a diary of past events, allowing companies to identify patterns and trends from their historical data.
Moving up the spectrum, prescriptive analytics takes the insights gained from descriptive analytics and predictive models to recommend actions that can lead to desired outcomes. It's about answering the question, "What should we do next?" to optimize decision-making and achieve specific goals. This advanced form of analytics incorporates techniques like graph analysis, simulation, and machine learning.
Prescriptive analytics represents the pinnacle of the analytics evolution, enabling businesses to not only predict the future but also to influence it with informed, strategic decisions.
Here are some key characteristics of prescriptive analytics:
- Optimization: Aiming to recommend the most beneficial actions.
- Decision support: Providing insights into the potential impact of different actions.
In contrast, descriptive analytics focuses on data summarization, which condenses extensive datasets into a concise representation, using statistical summaries such as mean, median, and mode.
Data Democratization and Employee Empowerment
In the landscape of modern business, data democratization is a pivotal trend that is reshaping how insights are accessed and utilized across organizations. By equipping employees with user-friendly analytics tools, businesses are fostering an environment where data can be leveraged for strategic decision-making at all levels. This empowerment leads to enhanced collaboration and drives innovation, as individuals are no longer dependent on specialized data teams to interpret complex datasets.
Data governance and privacy are integral to the successful implementation of data democratization. As employees gain greater access to data, it is imperative that businesses establish robust frameworks to ensure ethical usage, security, and compliance. This includes practices such as access control, data de-identification, and stringent data security measures to safeguard against cyber threats.
By embracing data democratization, companies are not only accelerating their growth and competitiveness but are also cultivating a data-driven culture that permeates every facet of their operations.
To illustrate the impact of data democratization, consider the following points:
- Encourages independent data analysis and informed decision-making.
- Reduces bottlenecks by allowing direct access to data for all employees.
- Promotes a culture of continuous learning and improvement.
- Nurtures transparency and accountability in data handling.
Ensuring Data Accuracy and Reliability
In the realm of business analytics, the quality of data underpins the integrity of insights derived. High-quality data is the cornerstone of any analytics strategy, ensuring that the forecasts and patterns identified are reflective of true market conditions. Advanced analytics might forecast future sales based on these patterns, but such insights are only valuable when built on high-quality data.
To maintain data accuracy and reliability, businesses must adopt robust data management practices. These include standardization, validation, and de-duplication, which are essential to avoid the risk of misinformed decisions. Real-time data can provide instant feedback on a marketing campaign's success, but without accurate, clean, and structured data, such analysis might lead astray.
Moving to the cloud is a strategic business decision for scalability and cost-effectiveness. Data analytics advancements offer valuable insights for decision-making and reshaping strategies in various industries.
Ensuring data security and compliance is also critical, especially when dealing with sensitive information. The analytics tools must adhere to data security standards and compliance regulations relevant to your industry. Here are some key practices to enhance data accuracy and reliability:
- Recruit and retain skilled professionals with expertise in data science, analytics, and data engineering.
- Implement strong data governance practices to ensure data quality, security, and compliance.
- Establish clear policies and procedures for data management, access control, and data privacy.
- Emphasize data ethics and privacy, including access control, data de-identification, and robust data security measures.
The Future Landscape of Data Analytics
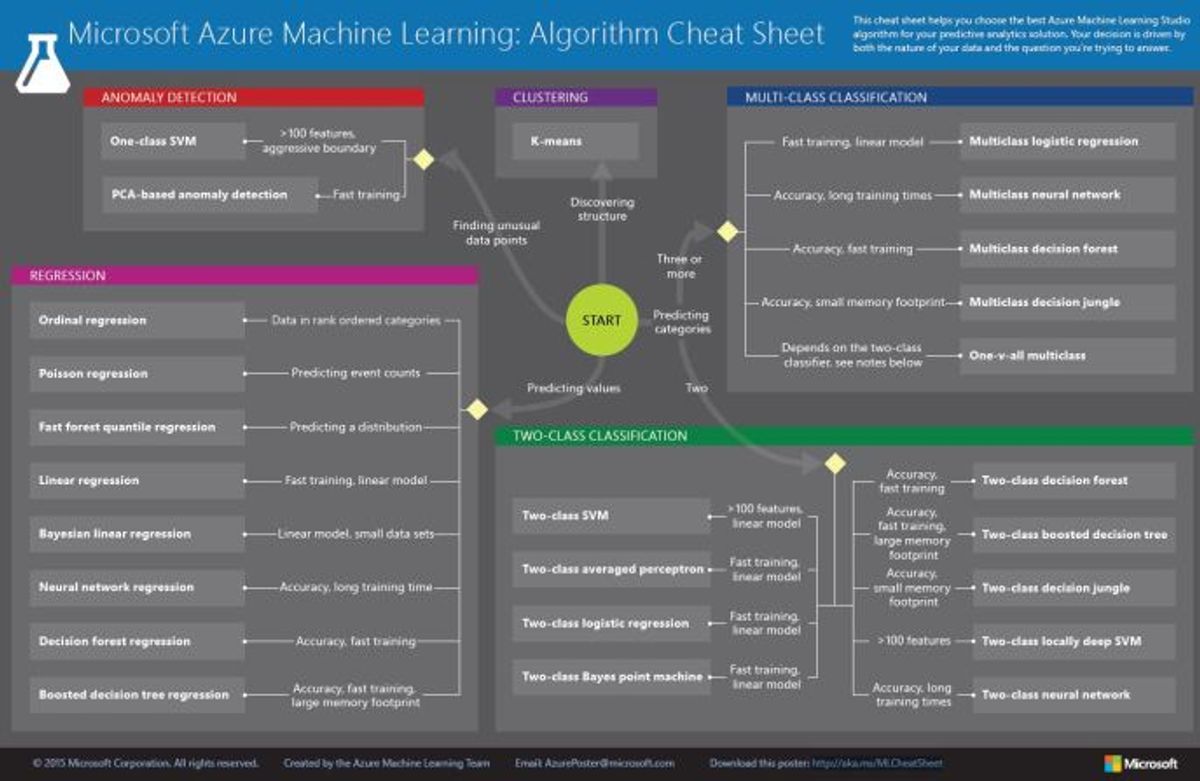
Market Growth Projections and Industry Impact
The trajectory of the data analytics market is nothing short of remarkable. According to Allied Market Research, the sector valued at $225.3 billion in 2023 is on a steep climb, projected to hit a staggering $665.7 billion by 2033. This growth is not just numerical; it reflects a deeper integration of analytics into the fabric of modern business.
Key trends driving this expansion include the democratization of data, advancements in natural language processing (NLP), Data as a Service (DaaS), and deeper AI integration. These trends are setting the stage for a future where predictive modeling, machine learning (ML), and data-driven decision making become the cornerstones of innovation and competitive advantage.
The surge in market value is paralleled by the diversification of industries embracing analytics. From startups to healthcare, and from retail to public sectors, the application of data analytics is becoming ubiquitous, signaling a transformative era in business intelligence.
The implications for industry are profound. As businesses harness these trends, they are reshaping their strategies, operations, and customer engagements, ensuring that data analytics remains a critical tool for achieving strategic advantage.
Emerging Technologies in Data Analytics
The landscape of data analytics is continuously evolving, with new technologies emerging to transform how businesses harness data for strategic insights. Augmented analytics is at the forefront, utilizing AI and natural language processing to simplify data analysis for a broader user base. This democratization of data empowers employees across various departments to engage with data-driven decision-making.
Real-time analytics is another significant trend, enabling organizations to react swiftly to market changes and customer behaviors. By leveraging the latest in cloud technology, businesses can process and analyze data as it's generated, leading to more agile and informed strategies.
The integration of advanced SQL techniques and AI into analytics tools is enhancing the precision and speed of data-driven insights, which is essential for maintaining a competitive edge in today's fast-paced business environment.
Data governance and privacy are also gaining prominence as critical components of a responsible analytics framework. With regulations like GDPR in place, companies are prioritizing the ethical use of data and the protection of consumer privacy.
The Increasing Importance of Data-Driven Cultures
In the modern business environment, the cultivation of a data-driven culture is not just a trend; it's a fundamental shift in how organizations operate. A data-driven culture emphasizes the strategic use of data at all levels, from executives to front-line employees, ensuring that decisions are backed by evidence rather than intuition.
Data governance and privacy are becoming increasingly important as the reliance on data grows. Organizations are implementing stricter regulations and enhancing security measures to protect sensitive information and maintain trust with stakeholders.
- Foster collaboration between data teams and business units
- Encourage continuous learning and improvement
- Prioritize ethical considerations and compliance with regulations like GDPR
The integration of data analytics into business strategies has become a cornerstone for achieving competitive advantage and driving innovation. As businesses continue to democratize data access, employees are empowered to leverage insights for tactical and strategic decision-making.
Harnessing Big Data for Strategic Advantage
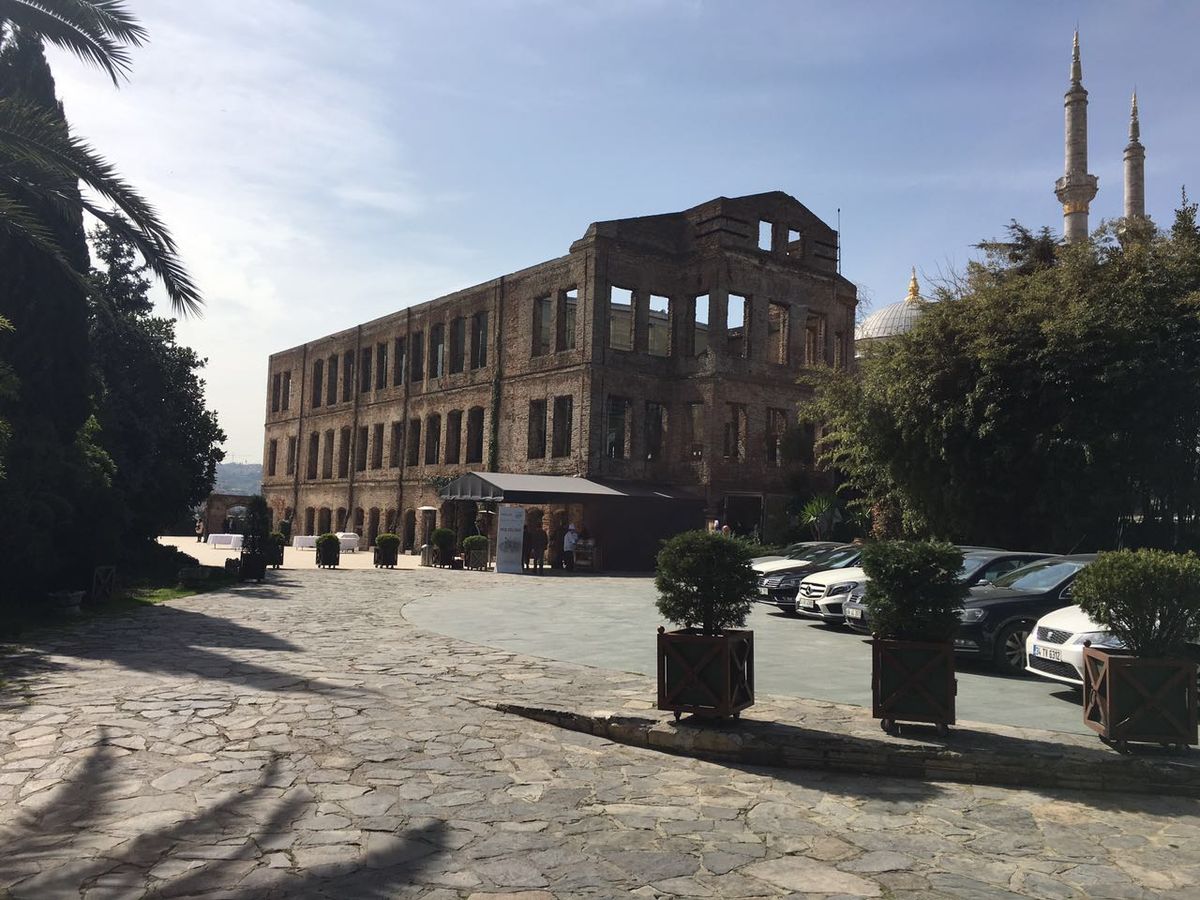
Applications of Big Data in Various Industries
In the digital era, Big Data is a pivotal asset across various industries, driving innovation and competitive advantage. The applications of Big Data are as diverse as the industries it permeates, from marketing to supply chain management.
- In marketing and sales, Big Data enables precise customer segmentation and personalized campaigns, leveraging behavioral and demographic insights.
- Operations benefit from predictive analytics, enhancing supply chain efficiency and forecasting.
Big Data's transformative impact is evident in its ability to optimize operations and facilitate data-driven decision-making.
The following table illustrates the role of Big Data in different sectors:
Industry | Application |
---|---|
Retail | Customer segmentation and demand forecasting |
Healthcare | Patient data analysis for improved outcomes |
Entertainment | Content tailoring and marketing strategies |
Each sector harnesses the power of Big Data to not only understand current trends but also to predict future patterns, ensuring strategic agility and resilience.
Overcoming Challenges in Data-Driven Decision Making
In the realm of data-driven decision making, organizations face a myriad of challenges that can hinder their ability to harness the full potential of Big Data. Key among these challenges is ensuring data quality and integrity, which is critical for generating reliable insights. To overcome these obstacles, businesses must adopt a multifaceted approach:
- Foster a Data-Driven Culture: Cultivate an environment where data is valued across all organizational levels. Encourage collaboration between data professionals and business stakeholders to translate insights into strategic actions.
- Continuous Learning and Improvement: Embrace a philosophy of ongoing education to keep pace with evolving data analytics techniques and technologies.
- Root Cause Analysis: Implement systematic approaches to identify the underlying causes of data issues, enabling more effective resolutions.
By addressing these challenges head-on, companies can refine their data analysis processes, leading to more accurate and actionable business insights. The journey to optimizing data analysis is not just about the data itself, but also about the methodologies and the people involved in the process.
The Benefits of Big Data in Business Insights
The advent of Big Data analytics has been a game-changer for businesses across the globe. Harnessing the power of vast data sets can lead to significant competitive advantages, from cost reduction to the development of customer-centric products and services. Big Data analytics not only aids in identifying trends and patterns but also in making predictions that inform strategic business moves.
- Enhanced decision-making through predictive insights
- Cost savings by identifying inefficiencies
- Creation of personalized customer experiences
- Improved operational efficiency and risk management
The strategic application of Big Data is pivotal in transforming data into actionable insights that drive business success.
The utilization of Big Data is not without its challenges, but the potential rewards make it an indispensable tool for companies looking to thrive in the digital age. By turning data into intelligence, businesses can uncover opportunities for innovation and growth that were previously untapped.
Real-World Applications of Data Analytics
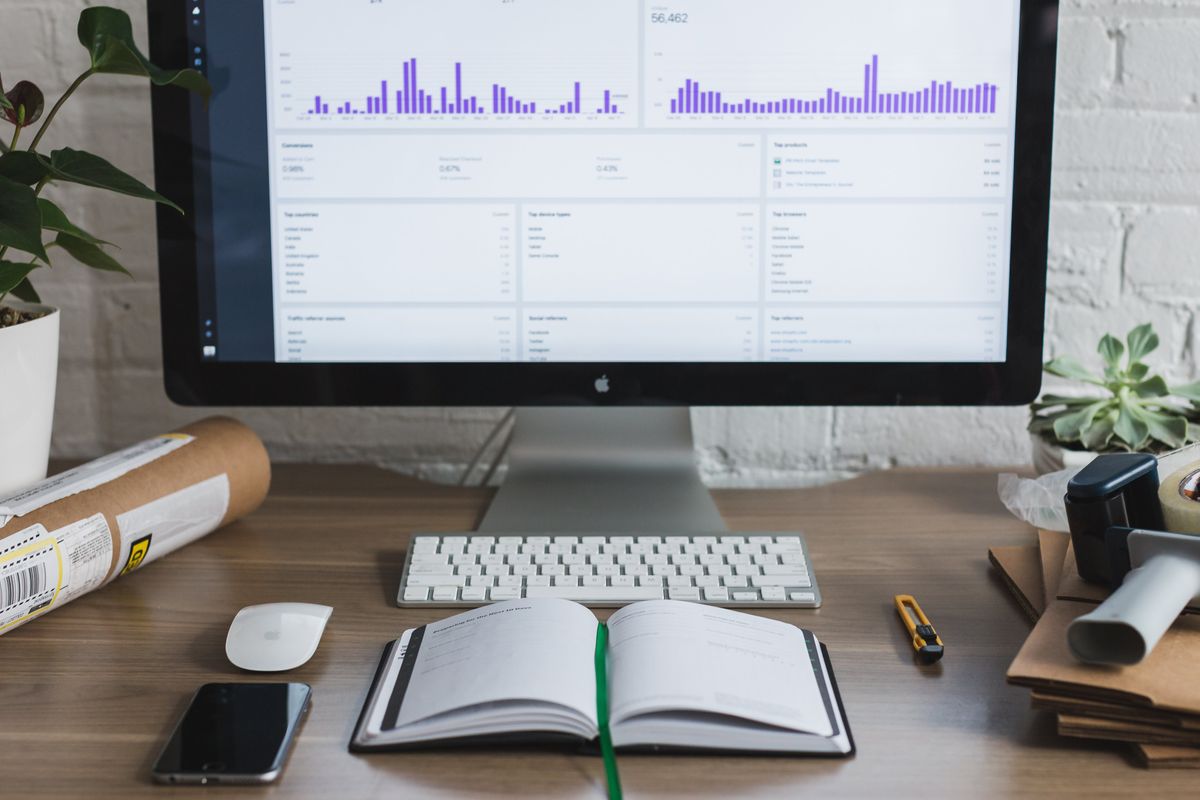
Healthcare: Improving Patient Outcomes
In the realm of healthcare, data-driven decision-making is pivotal for enhancing patient care and operational efficiency. By leveraging analytics, healthcare providers can forecast future trends and behaviors, leading to more proactive and personalized treatment plans. This approach not only improves patient outcomes but also optimizes resource allocation within healthcare facilities.
For instance, the use of predictive analytics allows for the anticipation of patient admission rates. Healthcare executives can analyze historical and current data on health trends and seasonal illnesses to better prepare for patient needs. This strategic planning is crucial for ensuring that resources, staff, and bed space are adequately allocated, thus maintaining high standards of service delivery.
The integration of wearable devices and genomic data into healthcare analytics further empowers providers to offer real-time monitoring and tailored healthcare interventions.
Additionally, programs like UnitedHealthcare's Advocate4Me demonstrate the transformative power of analytics in healthcare. By analyzing anonymized claims data, they identify members at risk and proactively address their health and social needs, showcasing a commitment to patient-centric care.
Retail: Personalizing Customer Experiences
In the competitive landscape of retail, Big Data Analytics plays a pivotal role in personalizing the customer journey. By leveraging customer data, retailers can create highly tailored experiences that resonate with individual preferences and behaviors. This not only fosters customer loyalty but also drives sales through targeted marketing strategies.
- Customer segmentation allows for more effective marketing campaigns.
- Demand forecasting helps in maintaining optimal inventory levels.
- Supply chain optimization ensures timely product availability.
The integration of AI and machine learning into retail systems has revolutionized the way businesses understand and cater to their customers. Personalization has become a cornerstone of customer satisfaction and loyalty.
The table below illustrates the impact of data analytics on key retail metrics:
Metric | Before Analytics | After Analytics |
---|---|---|
Customer Engagement | Low | High |
Marketing ROI | Uncertain | Improved |
Inventory Management | Inefficient | Streamlined |
As we look to the future, the retail industry is poised to embrace even more advanced technologies, such as virtual reality and predictive merchandising, further enhancing the shopping experience.
Entertainment: Tailoring Content and Marketing
In the entertainment industry, big data is revolutionizing the way content is created and marketed. By analyzing viewer preferences and behaviors, studios and networks can produce shows and movies that are more likely to resonate with their target audiences. This targeted approach extends to marketing, where data analytics enables personalized advertising campaigns that are more effective and cost-efficient.
- Data analytics in entertainment:
- Customer segmentation for targeted content
- Personalized marketing campaigns
- Social media engagement analysis
- Conversion rate and ROI assessment
Moreover, data analytics serves as a catalyst for enhanced profitability. By leveraging precise analytics, entertainment businesses can optimize their marketing strategies and create content that aligns with viewer demands, thus boosting revenue streams.
By employing predictive modeling, entertainment companies can anticipate trends and viewer preferences, allowing for proactive content strategy development.
Case Studies: Transformative Power of Data Analytics
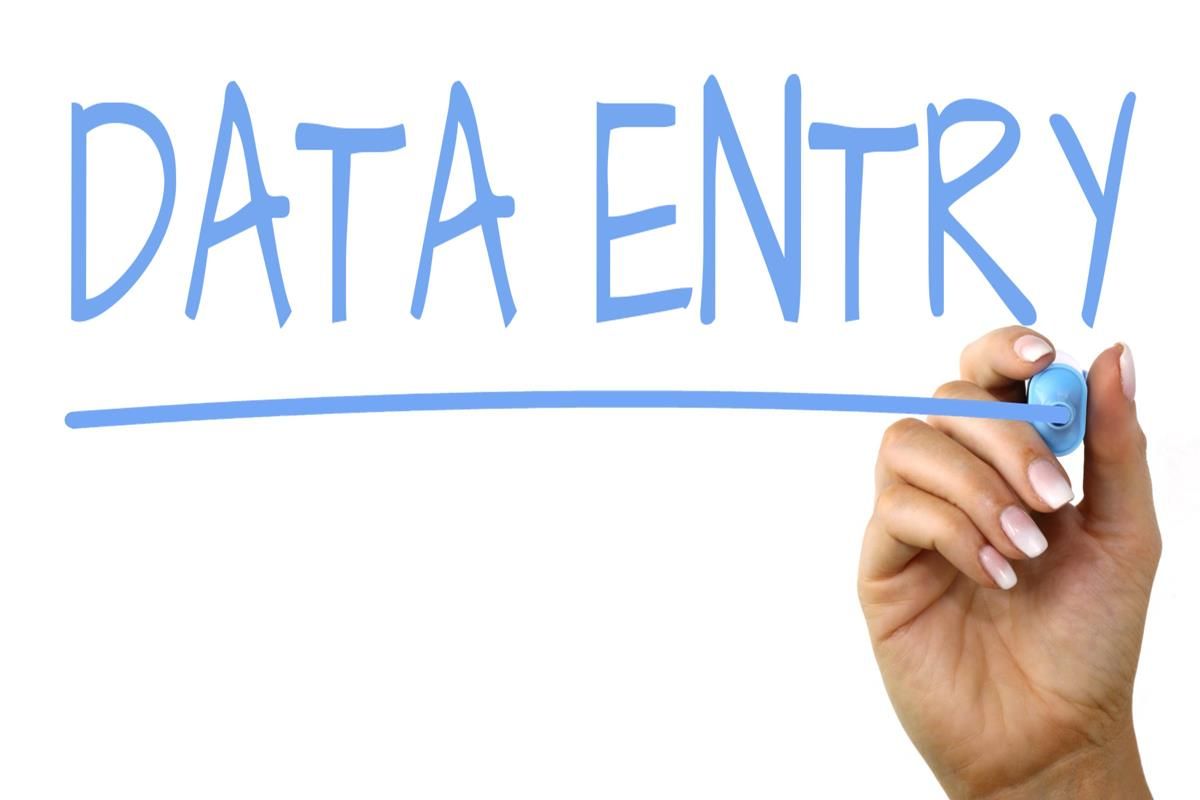
Revolutionizing Supply Chain Management
The recent global events, such as the COVID-19 pandemic, have underscored the critical role of supply chain management. Businesses are increasingly turning to analytics to enhance operational efficiency, manage risks, and build resilient supply chains. By leveraging predictive analytics, companies can anticipate disruptions and adapt their strategies accordingly.
- Predictive maintenance in manufacturing reduces downtime.
- Risk management through data analysis minimizes supply chain vulnerabilities.
- Enhanced resilience by anticipating and preparing for potential disruptions.
The transformative power of analytics in supply chain management is not just about responding to immediate challenges; it's about reimagining the entire ecosystem for long-term sustainability and success.
As industries continue to evolve, the integration of big data and advanced analytics tools is becoming a cornerstone for competitive advantage. The manufacturing sector, for instance, is witnessing a significant shift towards digitalization, with predictive maintenance and quality control being prime examples of data-driven optimization.
Adaptive Pricing Models in E-commerce
In the competitive landscape of e-commerce, adaptive pricing models have emerged as a game-changer for businesses. By leveraging analytics, companies can dynamically adjust prices in real-time, responding to market demands, inventory levels, and consumer behavior. This strategy not only maximizes profits but also enhances customer satisfaction by offering competitive pricing.
The essence of adaptive pricing lies in its ability to transform vast amounts of data into actionable insights, enabling businesses to stay ahead in a rapidly changing market.
For instance, during high-demand periods, prices may increase to capitalize on the willingness to pay, while during slower periods, discounts can stimulate sales. The table below illustrates a simplified example of how prices might fluctuate based on demand:
Time of Day | Demand Level | Price Adjustment |
---|---|---|
Morning | Low | -5% |
Afternoon | Medium | No Change |
Evening | High | +10% |
This approach is not without its challenges, however. It requires a robust technological infrastructure and sophisticated algorithms to ensure that price changes are both timely and appropriate, avoiding potential customer dissatisfaction or brand damage.
Data Analytics in Customer Relationship Management
In the realm of Customer Relationship Management (CRM), data analytics plays a pivotal role in enhancing customer engagement and optimizing marketing strategies. By dissecting customer interactions and feedback, businesses can tailor their approaches to meet individual customer needs, fostering loyalty and increasing retention rates.
Enhance customer satisfaction: Leveraging data analytics leads to a deeper understanding of customer preferences, which can be used to personalize products and services. This not only boosts customer satisfaction but also minimizes churn.
Gain a competitive advantage: Data-driven insights allow businesses to spot trends and potential risks, providing a strategic edge in the marketplace.
Data-driven organizations are significantly more likely to excel in customer acquisition and retention, underscoring the transformative impact of analytics on CRM.
The table below illustrates the benefits of data analytics in CRM through key performance indicators:
KPI | Impact of Data Analytics |
---|---|
Customer Lifetime Value (CLV) | Improved prediction and strategy alignment |
Sales Forecast Accuracy | Enhanced resource planning and allocation |
Marketing Campaign Effectiveness | Increased customer engagement and ROI |
In conclusion, the integration of data analytics into CRM systems is not just a trend but a fundamental shift towards a more nuanced and effective customer-centric business model.
Discover the transformative power of data analytics through our compelling case studies. At OptimizDBA, we pride ourselves on delivering faster data solutions and unparalleled database optimization consulting. Don't just take our word for it; our clients, like Stella Budrean from Radio-Canada Inc., have experienced the exceptional benefits of our services. Ready to unlock the full potential of your data? Visit our website to learn how our proprietary methodologies can revolutionize your transaction speeds and overall performance. Let's embark on this journey to data excellence together!
Conclusion
The landscape of business analytics is undergoing a remarkable transformation, driven by the relentless growth of big data and the continuous advancements in analytical technologies. As we have explored in this article, from the evolution of analytics to the emerging and future trends, it is evident that data analytics has become an indispensable tool for businesses seeking to harness the power of data for strategic decision-making. The integration of AI and machine learning into analytics is not just enhancing the accuracy of predictions but also enabling prescriptive insights that can guide businesses towards optimal outcomes. The case studies discussed underscore the practical applications and tangible benefits of data analytics across various industries. As organizations continue to embrace these evolving trends, the potential for innovation and competitive advantage is boundless. The era of data-driven business is here, and it promises to redefine the paradigms of success in the digital age.
Frequently Asked Questions
What is Augmented Analytics?
Augmented analytics refers to the use of enabling technologies such as machine learning and AI to assist with data preparation, insight generation, and insight explanation to augment how people explore and analyze data in analytics and BI platforms.
How has business analytics evolved over time?
Business analytics has evolved from traditional reporting and descriptive analytics to advanced predictive and prescriptive analytics, incorporating AI and machine learning to analyze real-time data and provide deeper insights for decision-making.
What role does data play in digital transformation?
Data is fundamental to digital transformation, providing the backbone for process optimization, predictive modeling, and strategic decision-making. It enables businesses to gain insights, improve customer experiences, and maintain a competitive edge.
How does data democratization empower employees?
Data democratization involves making data accessible to all employees within an organization, regardless of their technical expertise. This empowers them to make informed decisions, fosters a data-driven culture, and helps optimize operations.
What are the projected market growth and industry impacts for data analytics?
The global big data and business analytics market is projected to grow from $225.3 billion in 2023 to $665.7 billion by 2033. This growth highlights the increasing importance of data analytics in driving industry innovation and efficiency.
What are some real-world applications of data analytics?
Real-world applications of data analytics include improving patient outcomes in healthcare, personalizing customer experiences in retail, and tailoring content and marketing strategies in the entertainment industry.
What are the benefits of Big Data in business insights?
Big Data offers numerous benefits, including enhanced decision-making, more efficient operations, improved customer experiences, and the ability to identify and act on market trends and consumer behavior insights.
Can you provide examples of how data analytics has transformed business practices?
Data analytics has transformed business practices by revolutionizing supply chain management with predictive forecasting, enabling adaptive pricing models in e-commerce, and improving customer relationship management through data-driven strategies.