Exploring Predictive Analytics for Business Decision-making
Overview
Introduction
Predictive analytics is a powerful tool that helps businesses make informed decisions by using historical data and statistical algorithms to predict future outcomes. It enables organizations to identify patterns, trends, and relationships in data, allowing them to gain valuable insights and make accurate forecasts. With the SQL industry growth and the increasing availability of data, predictive analytics has become essential for businesses looking to stay competitive in today's data-driven world.
Importance of Predictive Analytics
Predictive analytics plays a crucial role in data-driven decision making. By analyzing historical data and using advanced algorithms, businesses can gain valuable insights into future trends and outcomes. This allows them to make more informed decisions and take proactive measures to optimize their operations. Predictive analytics helps businesses identify patterns, detect anomalies, and predict customer behavior, enabling them to allocate resources effectively and improve overall performance. With the increasing availability of data and the advancements in technology, the importance of predictive analytics in business decision-making cannot be overstated.
Key Components
The key components of predictive analytics include data collection, data preprocessing, model development, and model evaluation. Data collection involves gathering relevant data from various sources such as databases, APIs, and external datasets. Data preprocessing is the process of cleaning, transforming, and preparing the data for analysis. Model development involves selecting and building the appropriate predictive models based on the problem at hand. This can include techniques such as regression, classification, and clustering. Model evaluation is the process of assessing the performance of the predictive models and fine-tuning them for better accuracy and reliability. By optimizing MySQL queries, businesses can improve the efficiency of data retrieval and processing, leading to faster and more accurate predictions.
Applications
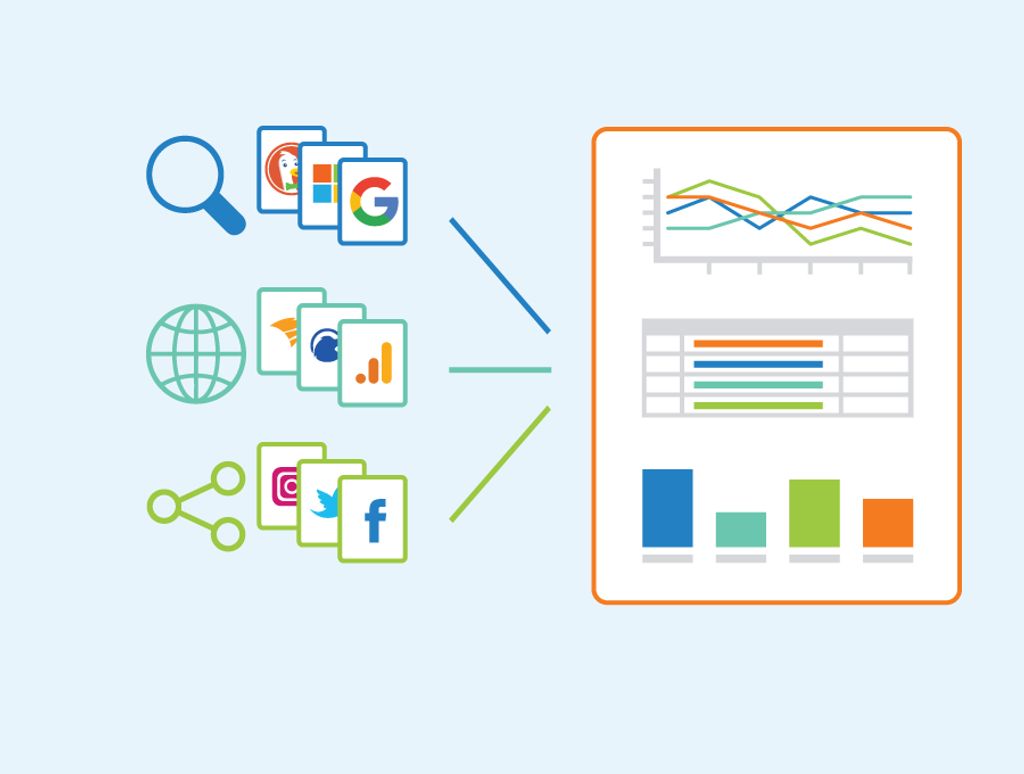
Sales Forecasting
Sales forecasting is a crucial aspect of business decision-making. It helps organizations predict future sales trends and make informed decisions about production, inventory, and resource allocation. By analyzing historical sales data, market trends, and customer behavior, businesses can identify patterns and factors that influence sales. Accurate sales forecasting enables companies to optimize their operations, minimize risks, and capitalize on opportunities. However, it is important to ensure data security when collecting and analyzing sensitive sales data.
Customer Segmentation
Customer segmentation is a crucial aspect of predictive analytics for businesses. By dividing customers into distinct groups based on their characteristics and behaviors, companies can gain valuable insights into their target audience and tailor their marketing strategies accordingly. Database optimization plays a significant role in customer segmentation, as it ensures that the data used for analysis is accurate, up-to-date, and easily accessible. Implementing effective database optimization techniques allows businesses to efficiently manage large volumes of customer data, leading to more accurate segmentation and better decision-making.
Risk Management
In today's rapidly changing business landscape, risk management plays a crucial role in ensuring the success and sustainability of organizations. Predictive analytics has emerged as a powerful tool for identifying and mitigating potential risks. By analyzing historical data and identifying patterns, predictive analytics enables businesses to make data-driven decisions when it comes to risk management. This allows organizations to proactively identify potential risks, assess their impact, and develop strategies to minimize their negative effects. Whether it's identifying fraudulent activities, predicting market trends, or assessing credit risk, predictive analytics empowers businesses to take proactive measures to protect their interests and make informed decisions.
Implementation
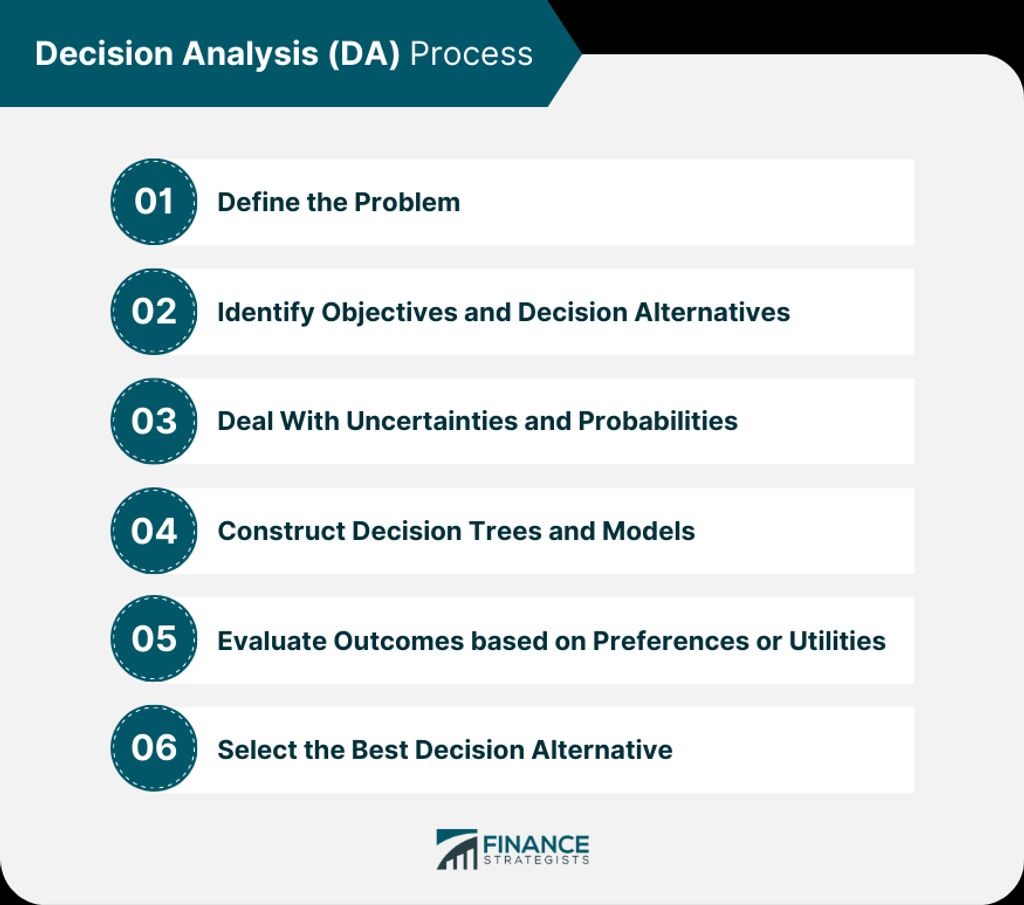
Data Collection and Preparation
In order to effectively use predictive analytics for business decision-making, it is crucial to collect and prepare the right data. Data collection involves gathering information from various sources such as internal databases, external sources, and third-party data providers. This data can include customer demographics, sales transactions, website interactions, and social media data. Once the data is collected, it needs to be cleaned, transformed, and organized for analysis. This process involves removing duplicates, handling missing values, and ensuring data consistency and accuracy. Additionally, data preparation may also involve feature engineering, which is the creation of new variables or features based on existing data. By carefully collecting and preparing the data, businesses can ensure that their predictive analytics models are accurate and reliable.
Model Development
Once the data has been collected and prepared, the next step in the predictive analytics process is model development. This involves the creation and training of machine learning models using the prepared data. The models are then evaluated and refined to ensure their accuracy and reliability. Model development is a crucial stage as it determines the effectiveness of the predictive analytics solution. It requires expertise in data science and statistical modeling techniques to develop models that can effectively predict future outcomes based on historical data.
Integration with Business Processes
Integration of predictive analytics with business processes is a crucial step in leveraging its benefits. Server Management is one area where predictive analytics can play a significant role. By analyzing historical data and patterns, businesses can predict server failures and proactively take necessary actions to prevent downtime. This not only improves efficiency but also reduces costs associated with server maintenance. Additionally, predictive analytics can help optimize resource allocation, ensuring that servers are utilized effectively and efficiently. By integrating predictive analytics into their business processes, organizations can make data-driven decisions and stay ahead of the competition.
Case Studies
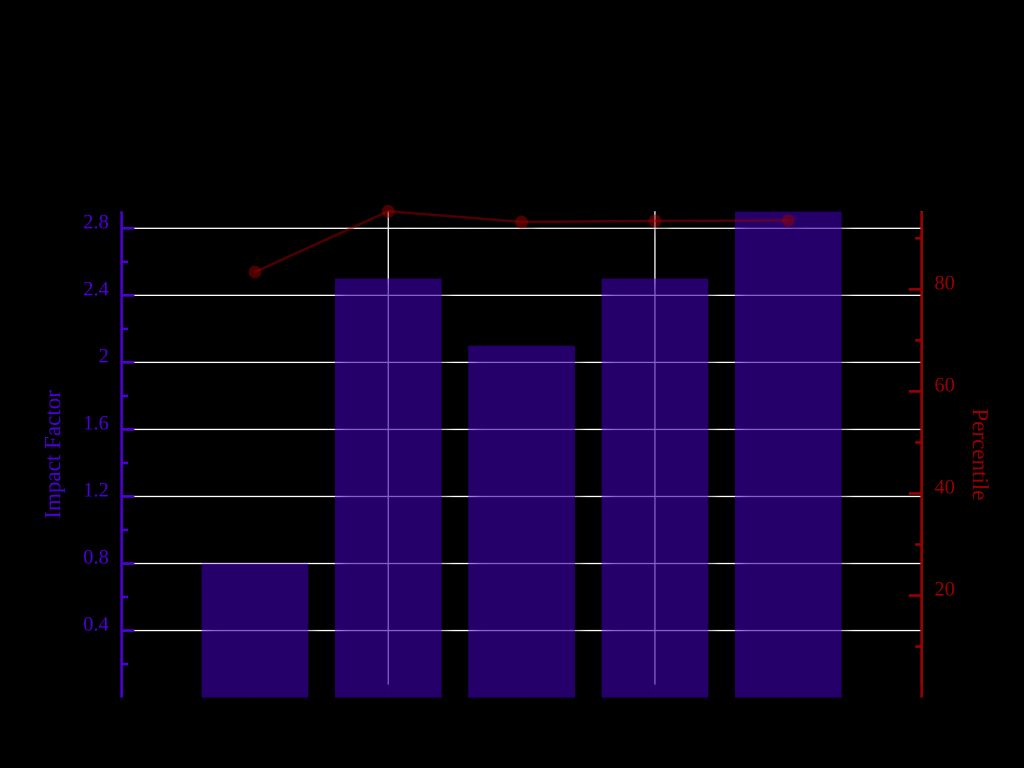
Retail Industry
The retail industry is one of the sectors that has greatly benefited from predictive analytics. By leveraging the power of data and advanced algorithms, retailers are able to make more informed decisions and improve their business outcomes. Predictive analytics allows retailers to forecast demand, optimize pricing strategies, and personalize customer experiences. For example, retailers can use predictive analytics to analyze historical sales data and identify trends and patterns that can help them predict future sales. This enables them to make accurate sales forecasts and ensure that they have the right amount of inventory at the right time. Additionally, predictive analytics can help retailers segment their customers based on their preferences and purchasing behavior, allowing them to tailor marketing campaigns and promotions to specific customer segments. Overall, predictive analytics is revolutionizing the retail industry by enabling retailers to make data-driven decisions and gain a competitive edge in the market.
Healthcare Sector
The healthcare sector is one of the industries that can greatly benefit from predictive analytics. By leveraging advanced analytics techniques, healthcare organizations can improve patient outcomes, optimize resource allocation, and enhance operational efficiency. Predictive analytics can be used in various areas of healthcare, such as patient diagnosis, treatment planning, and disease prevention. For example, predictive models can analyze patient data to identify individuals at high risk of developing certain conditions, allowing healthcare providers to intervene early and provide targeted interventions. Additionally, predictive analytics can help healthcare organizations in optimizing staffing levels and predicting patient demand, ensuring that resources are allocated efficiently. Overall, predictive analytics has the potential to revolutionize the healthcare sector by turning data into gold and enabling evidence-based decision-making.
Financial Services
In the financial services industry, predictive analytics has become an invaluable tool for unlocking hidden insights and driving informed decision-making. By analyzing vast amounts of historical data, financial institutions can identify patterns, trends, and potential risks, enabling them to make accurate predictions about market trends, customer behavior, and investment opportunities. This allows them to optimize their operations, improve risk management, and enhance customer satisfaction. For example, predictive analytics can help banks identify potential fraudulent activities by analyzing transaction patterns and detecting anomalies. Additionally, it can assist in predicting customer churn and developing targeted marketing strategies to retain valuable customers. Overall, predictive analytics has revolutionized the way financial services operate, providing them with a competitive edge in a rapidly changing landscape.
Conclusion
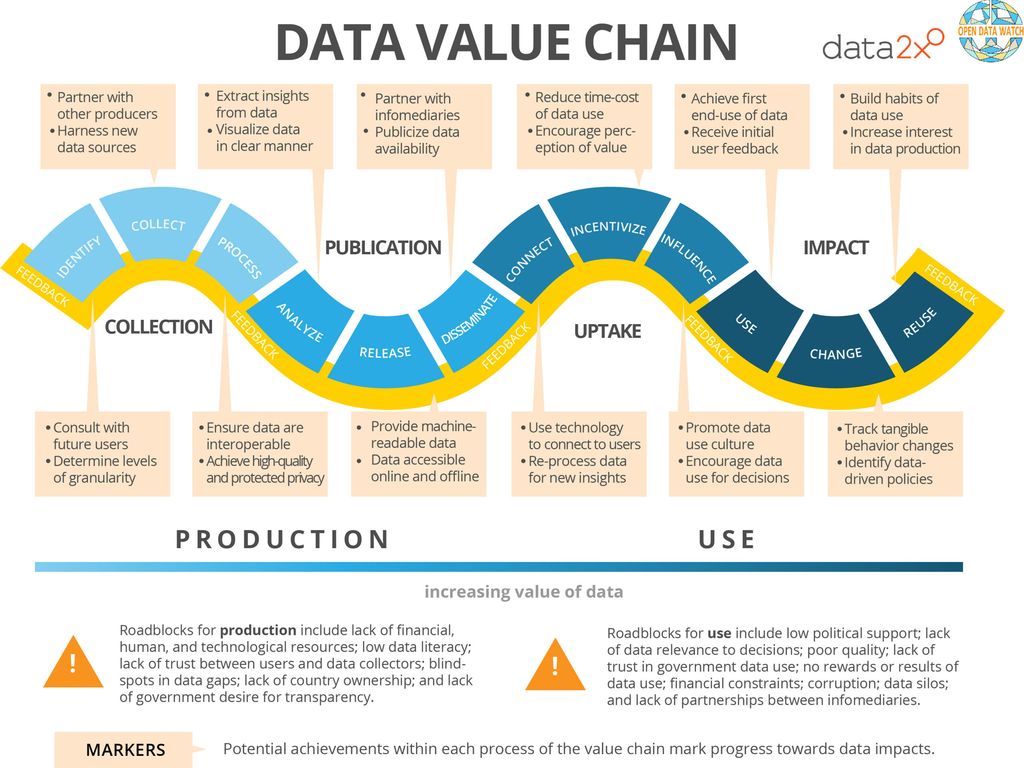
Key Takeaways
In conclusion, predictive analytics is a powerful tool for business decision-making. It allows organizations to make data-driven decisions and gain a competitive advantage. Database Optimization is a critical component of implementing predictive analytics, as it ensures that data is stored and retrieved efficiently. By optimizing databases, businesses can improve the performance and speed of their predictive models. Overall, predictive analytics has the potential to revolutionize the way businesses operate and make decisions.
Future Trends
As technology continues to advance, the future of predictive analytics looks promising. Best Cloud Database Softwares are expected to play a crucial role in enhancing data storage and processing capabilities. This will enable businesses to handle large volumes of data more efficiently and extract valuable insights. Additionally, there is a growing emphasis on real-time analytics, allowing organizations to make faster and more informed decisions. Machine learning algorithms are also evolving, becoming more sophisticated and accurate in predicting outcomes. With the increasing availability of data and advancements in technology, predictive analytics is poised to revolutionize business decision-making in the coming years.
Final Thoughts
In conclusion, predictive analytics is a powerful tool that can revolutionize business decision-making. By leveraging historical data and advanced algorithms, organizations can gain valuable insights into future trends and patterns. Indexing plays a crucial role in speeding up data retrieval and improving the efficiency of predictive models. It allows for quick access to specific data points, enabling faster analysis and decision-making. However, it is important to note that predictive analytics is not a magic solution. It requires careful data collection, model development, and integration with existing business processes to ensure accurate and actionable results. As technology continues to evolve, the future of predictive analytics holds great promise, with advancements in machine learning and artificial intelligence opening up new possibilities for businesses in various industries.
In conclusion, OptimizDBA Database Optimization Consulting is the trusted industry leader in remote DBA services. With over 500 clients and a track record of delivering transaction speeds that are at least twice as fast as before, we guarantee a significant increase in performance. Our average speeds are often 100 times, 1000 times, or even higher! If you're looking to optimize your database and experience unparalleled performance, contact OptimizDBA today. Visit our website to learn more about our services and how we can help you achieve optimal database performance.