How to Elicit Business Insights through Data Analytics
Understanding the Importance of Data Analytics in Business
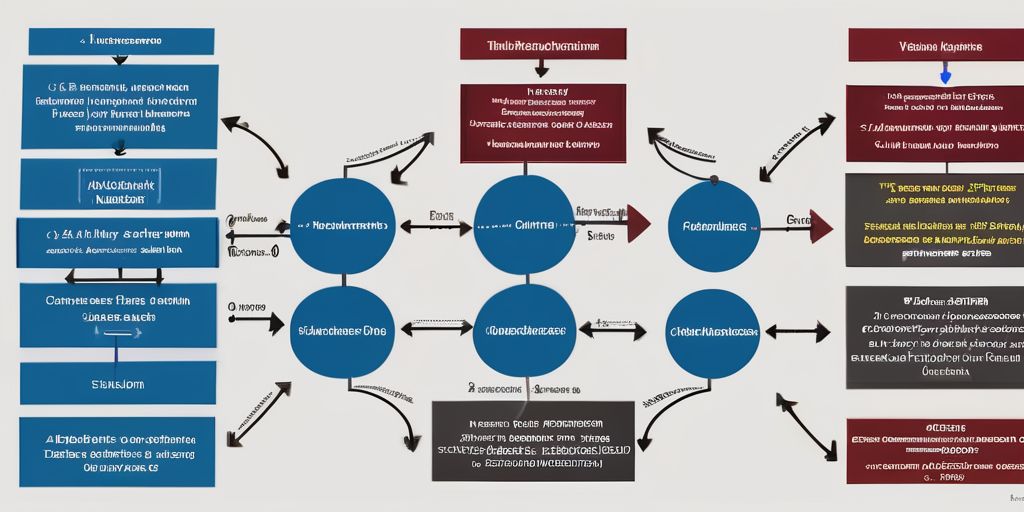
The Role of Data Analytics in Decision Making
Data analytics plays a crucial role in decision making for businesses. It involves the analysis and interpretation of data to extract valuable insights that can inform strategic choices. By leveraging data analytics, businesses can make more informed decisions based on solid evidence rather than intuition or assumption. This enables them to identify trends, patterns, and correlations in the data, which can guide their decision-making process.
One effective way to present structured, quantitative data is through a Markdown table. This allows for a clear and concise representation of data, making it easier to analyze and compare different variables. Another option is to use a bulleted or numbered list for presenting less structured content, such as steps or a series of related items.
Tip: When using data analytics for decision making, it is important to ensure the quality and reliability of the data. Data cleaning and data quality assurance processes should be implemented to remove any inconsistencies or errors that may affect the accuracy of the insights derived from the data.
How Data Analytics Drives Business Growth
Data analytics plays a dynamic role in driving business growth. By leveraging data-driven decision-making models, companies can make faster and well-informed business decisions. This empowers leaders to avoid wasting resources and capitalize on opportunities that lead to growth. Data analytics provides valuable insights into customer behavior, market trends, and competitive landscapes, enabling businesses to identify new revenue streams and optimize existing operations. With the ability to uncover hidden patterns and correlations in data, organizations can gain a competitive edge and stay ahead in today's rapidly evolving business landscape.
Benefits of Implementing Data Analytics in Business
Implementing data analytics in business offers several key benefits. One of the main advantages is the ability to identify patterns, trends, and consumer behaviors through the collection and analysis of business-relevant data[^ef78]. This valuable insight can inform decision-making processes and drive strategic initiatives. Additionally, data analytics tools provide actionable insights that turn unprocessed data into a valuable strategic resource for business decisions. These tools go beyond simply gathering and displaying data; they analyze it to offer illuminating advice[^ef78].
Collecting and Preparing Data for Analysis
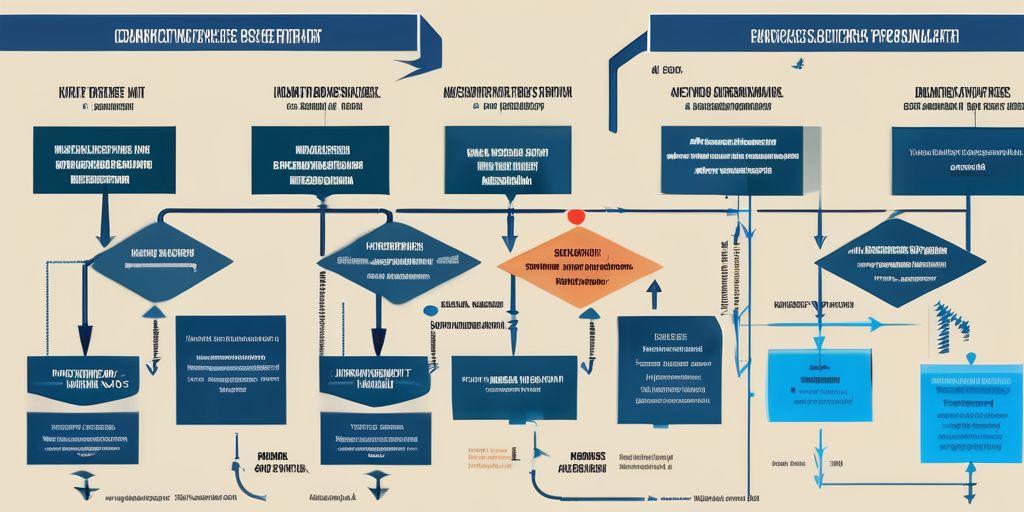
Identifying Relevant Data Sources
When it comes to identifying relevant data sources for analysis, there are several factors to consider. First, it is important to determine the specific information that is needed to answer the research question or address the business problem at hand. This will help narrow down the types of data sources that are most relevant.
Once the information needs are identified, the next step is to explore the available data sources. These can include internal sources such as company databases, CRM systems, and transaction records, as well as external sources such as government databases, industry reports, and social media data.
It is also important to consider the quality and reliability of the data sources. Data should be accurate, up-to-date, and relevant to the research question. Additionally, data privacy and security should be taken into account to ensure compliance with regulations and protect sensitive information.
In summary, identifying relevant data sources involves understanding the information needs, exploring internal and external sources, and considering data quality and privacy.
Data Cleaning and Data Quality Assurance
Data cleaning and data quality assurance are crucial steps in the data analytics process. Data cleaning involves identifying and correcting errors, inconsistencies, and inaccuracies in the dataset. It ensures that the data is reliable and accurate for analysis. Data quality assurance focuses on maintaining the integrity and quality of the data throughout the analytics process.
To ensure effective data cleaning and data quality assurance, consider the following:
- Use standardized data cleaning techniques to identify and correct errors, such as missing values, outliers, and duplicate entries.
- Validate the data against predefined rules and criteria to ensure accuracy and consistency.
- Implement data validation checks to identify and resolve data quality issues.
Tip: Regularly monitor and update data quality metrics to track the effectiveness of data cleaning and quality assurance efforts.
By following these best practices, organizations can ensure that the data used for analysis is reliable, accurate, and of high quality.
Data Integration and Data Transformation
Data integration and data transformation are crucial steps in the data analytics process. Integration involves combining data from different sources and formats into a unified dataset, while transformation involves cleaning, organizing, and structuring the data for analysis. These steps are essential for ensuring the accuracy and reliability of the data used in analytics.
To effectively integrate and transform data, organizations can follow these steps:
- Identify the relevant data sources: Determine which data sources are necessary for the analysis and ensure they are accessible and compatible.
- Clean and validate the data: Remove any inconsistencies, errors, or duplicates in the data to ensure its quality and reliability.
- Organize and structure the data: Arrange the data in a logical and consistent manner, making it easier to analyze and derive insights.
- Apply data transformation techniques: Use techniques such as aggregation, normalization, and feature engineering to enhance the data and make it suitable for analysis.
Tip: It is important to establish clear data integration and transformation processes to maintain data integrity and consistency throughout the analytics journey.
By effectively integrating and transforming data, organizations can unlock valuable insights and make data-driven decisions that drive business growth and success.
Exploratory Data Analysis Techniques
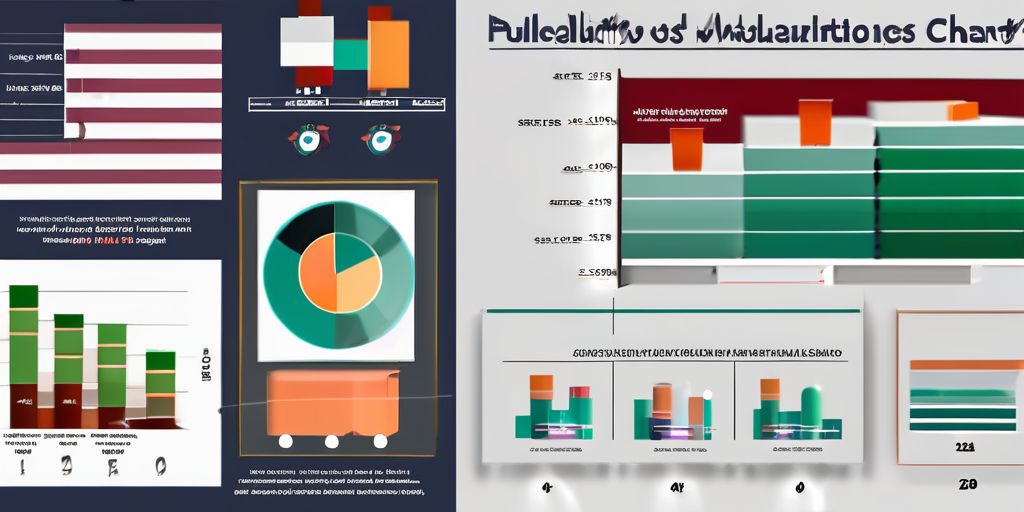
Descriptive Statistics and Data Visualization
Descriptive statistics and data visualization are essential techniques in data analytics. Descriptive statistics provide a summary of the main characteristics of a dataset, such as measures of central tendency and variability. These statistics help us understand the distribution and patterns in the data. Data visualization, on the other hand, allows us to represent the data visually through charts, graphs, and tables. It enhances our ability to identify trends, outliers, and relationships in the data.
When presenting structured, quantitative data, it is recommended to use a Markdown table. Tables provide a clear and concise way to present numerical information. Ensure that the table is properly formatted in Markdown to maintain readability.
For less structured content, like steps or qualitative points, a bulleted or numbered list can be used. Lists help organize information and make it easier to follow. They are particularly useful when presenting a series of related items or summarizing key points.
Remember to always consider the audience and purpose of your analysis when choosing the appropriate visualization techniques and formats for presenting your insights.
Identifying Patterns and Trends in Data
When analyzing data, it is important to focus on interpretation and identify key patterns and trends. This allows us to transform raw data into actionable insights that guide product development. There are several techniques that can be used to identify patterns and trends:
- Look for recurring themes in qualitative data
- Analyze statistical trends in quantitative data
- Identify outliers and anomalies for deeper exploration
By analyzing both qualitative and quantitative data, we can gain a comprehensive understanding of the patterns and trends present. This information can then be used to make actionable conclusions and prioritize changes based on user needs.
It is also important to link our findings directly to product improvements and develop effective measures for protecting communication security. By understanding the difference between simple and complex communication security scenarios, we can implement appropriate security measures.
In the world of data analytics, tools such as machine learning and AI can be used to identify patterns, predict user actions, and automate insights. Real-time analytics provide up-to-date information on user behaviors, while data visualization techniques such as charts, graphs, and tables make it easier to comprehend and analyze data.
Overall, identifying patterns and trends in data is a crucial step in data analytics. It allows us to uncover valuable insights that can drive business growth and guide decision-making processes.
Correlation and Regression Analysis
Correlation is the statistical technique that is used to describe the strength and direction of the relationship between two or more variables. It assesses the degree to which changes in one variable are associated with changes in another variable. Regression analysis, on the other hand, is a statistical method used to model the relationship between a dependent variable and one or more independent variables. It helps to understand how changes in the independent variables affect the dependent variable. Correlation and regression analysis are both important tools in data analytics, but they serve different purposes.
In correlation analysis, the relationship between variables is measured using a correlation coefficient, which ranges from -1 to 1. A positive correlation coefficient indicates a positive relationship, where an increase in one variable is associated with an increase in the other variable. A negative correlation coefficient indicates a negative relationship, where an increase in one variable is associated with a decrease in the other variable. A correlation coefficient close to 0 indicates no significant relationship between the variables.
Regression analysis, on the other hand, involves fitting a regression line to the data points to model the relationship between the dependent variable and the independent variables. The regression line represents the best fit to the data and can be used to make predictions about the dependent variable based on the values of the independent variables. Regression analysis also provides information about the significance of the relationship between the variables, as well as the strength and direction of the relationship.
In summary, correlation analysis is used to measure the strength and direction of the relationship between variables, while regression analysis is used to model the relationship between a dependent variable and one or more independent variables. Both techniques are valuable in data analytics and can provide insights into the relationships within a dataset.
Advanced Data Analytics Methods
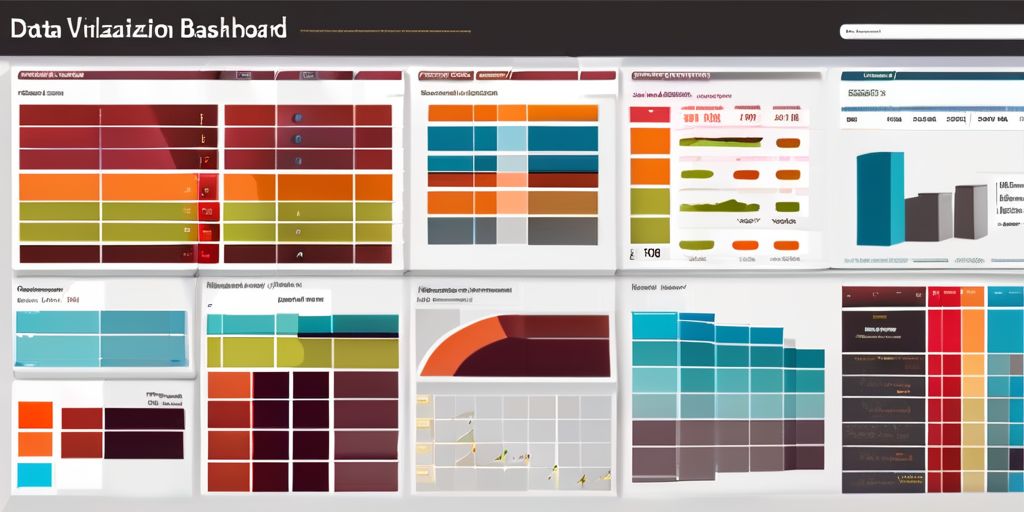
Predictive Analytics and Machine Learning
Predictive analytics is a powerful technique that leverages historical data and machine learning algorithms to forecast future outcomes. By analyzing patterns and trends in the data, predictive analytics can provide valuable insights and help businesses make informed decisions. This technique is particularly useful in industries such as finance, marketing, and healthcare, where accurate predictions can drive significant business growth.
One important aspect of predictive analytics is its ability to identify patterns and relationships in large datasets. Machine learning algorithms can analyze vast amounts of data and uncover hidden patterns that humans may not be able to detect. These patterns can then be used to make predictions and inform business strategies.
In addition to forecasting future outcomes, predictive analytics can also be used for anomaly detection. By comparing current data to historical patterns, businesses can identify unusual or unexpected events that may require immediate attention.
Implementing predictive analytics requires a combination of data analysis skills and the right tools and technologies. Businesses need to have access to high-quality data, as well as the expertise to interpret and apply the results of the analysis. It is also important to consider ethical and privacy considerations when using predictive analytics, as the use of personal data raises important ethical questions.
Overall, predictive analytics and machine learning are powerful tools that can help businesses gain valuable insights and make data-driven decisions. By leveraging historical data and advanced algorithms, businesses can forecast future outcomes, detect anomalies, and drive business growth.
Text Mining and Natural Language Processing
Text mining and natural language processing (NLP) are widely used in knowledge-driven organizations. Text mining is the process of examining large collections of documents to discover new information or help make data-driven decisions. NLP, on the other hand, focuses on the interaction between computers and human language, enabling machines to understand, interpret, and generate human language. These techniques have revolutionized various industries, including healthcare, finance, and customer service.
In text mining, structured, quantitative data can be presented in a table format to provide a clear overview of the extracted information. This allows analysts to identify patterns, trends, and relationships within the data. On the other hand, less structured content, such as steps or qualitative points, can be presented in a bulleted or numbered list format.
Tip: When implementing text mining and NLP techniques, it is important to consider the quality of the data being analyzed. Ensure that the data is clean, accurate, and relevant to the analysis objectives.
Social Network Analysis and Graph Analytics
Social Network Analysis and Graph Analytics is a powerful technique used in data analytics to analyze and visualize relationships between entities. It involves studying the structure and dynamics of networks, such as social media connections, customer interactions, or organizational hierarchies. By applying graph theory and algorithms, businesses can gain valuable insights into network patterns, identify key influencers, and detect communities or clusters within the network.
Some key applications of Social Network Analysis and Graph Analytics include:
- Identifying influential individuals or organizations in a network
- Understanding information flow and diffusion
- Recommender systems and personalized recommendations
- Fraud detection and anomaly detection
Tip: When conducting Social Network Analysis and Graph Analytics, it is important to consider the ethical implications of analyzing personal or sensitive data. Ensure compliance with data protection regulations and prioritize data privacy and security.
Data Visualization and Reporting
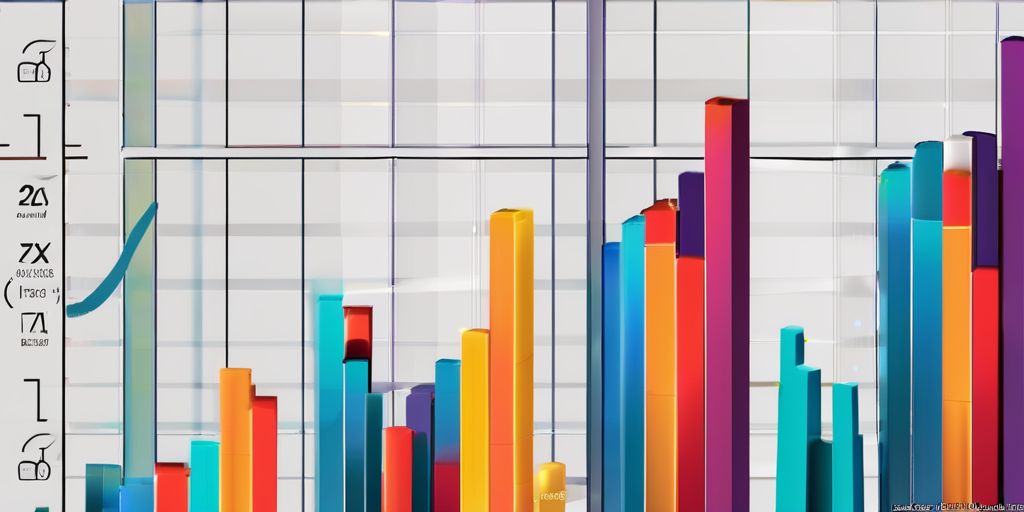
Choosing the Right Visualization Techniques
When it comes to choosing the right visualization techniques for your data analysis, there are several factors to consider. One important factor is the type of data you are working with. Structured, quantitative data can be effectively presented using a Markdown table. This allows for a clear and concise representation of the data, making it easy to compare and analyze.
Another factor to consider is the level of structure in your content. If you have less structured content, such as steps, qualitative points, or a series of related items, a bulleted or numbered list can be used to present the information in a clear and organized manner.
Additionally, it is important to keep in mind the audience you are presenting the data to. Different visualization techniques may be more effective for different audiences. For example, if you are presenting data to a non-technical audience, using charts and graphs that are easy to understand and visually appealing can help convey your insights effectively.
Lastly, it is worth noting that choosing the right visualization techniques is not just about the aesthetics. It is also about ensuring that the visualizations accurately represent the data and convey the intended message. It is important to select visualization techniques that best highlight the patterns and trends in your data, allowing for meaningful insights to be derived.
In summary, when choosing the right visualization techniques, consider the type of data, the level of structure in your content, the audience, and the accuracy and effectiveness of the visualizations in conveying insights.
Creating Interactive Dashboards
Creating interactive dashboards is an essential aspect of data visualization and reporting. Interactive dashboards allow users to explore and interact with data, enabling them to gain deeper insights and make informed decisions. One important step in creating interactive dashboards is to convert data into a Pivot table, which serves as the foundation for interactive charts. By organizing and summarizing data in a Pivot table, users can easily filter, sort, and analyze data in real-time. This enhances the user experience and facilitates data exploration.
To present structured, quantitative data in an interactive dashboard, it is recommended to use a Markdown table. A Markdown table provides a clear and concise representation of data, making it easy for users to compare and analyze different values. The table should be formatted correctly in Markdown, with appropriate headers and alignment.
In addition to tables, interactive dashboards can also include other visual elements such as charts, graphs, and maps. These visualizations help to convey complex information in a visually appealing and easily understandable manner. By incorporating interactive features like drill-down, filtering, and highlighting, users can further explore and analyze the data based on their specific needs.
Tip: When designing interactive dashboards, consider the target audience and their specific requirements. Focus on providing relevant and actionable insights that align with the business objectives. Keep the dashboard layout clean and intuitive, ensuring that users can easily navigate and interact with the data.
Creating interactive dashboards requires a combination of technical skills and a deep understanding of the data. It is important to select the right tools and technologies that support interactive data visualization and provide a seamless user experience. By investing time and effort in creating interactive dashboards, businesses can unlock valuable insights and drive data-driven decision making.
Effective Communication of Insights
Clear and concise communication is essential for effectively conveying insights to stakeholders. Summarize key findings in a concise manner and use visuals to enhance understanding. Tailor communication to the audience's expertise. It is important to ensure that your findings directly inform product decisions and development plans. To present structured, quantitative data, consider using a Markdown table that is succinct and formatted correctly. For less structured content, such as steps or qualitative points, use a bulleted or numbered list. Remember to keep the content clear and concise, and split it into multiple short paragraphs if needed.
Data Privacy and Ethical Considerations
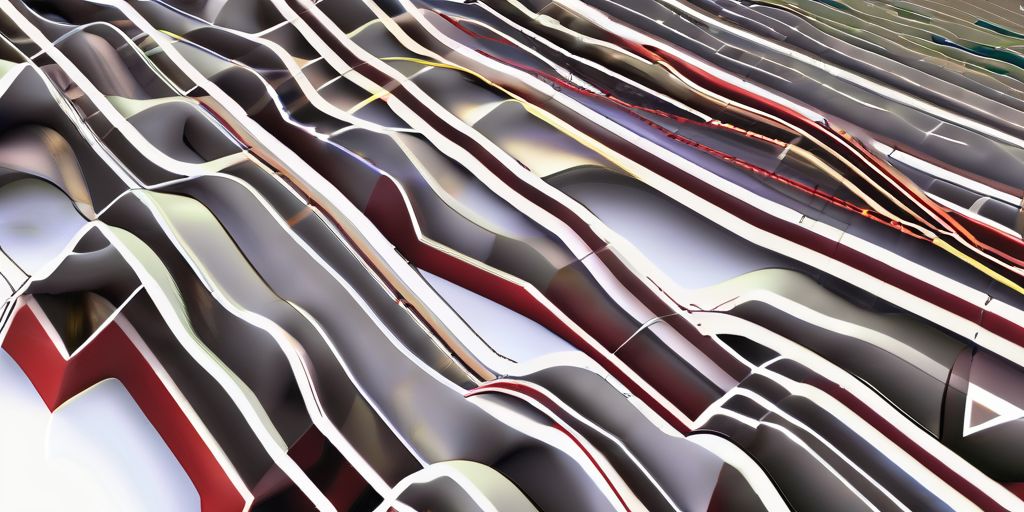
Ensuring Data Security and Privacy
In the world of data analytics, ensuring the security and privacy of data is of utmost importance. Organizations must take proactive measures to protect sensitive information from unauthorized access and breaches. This includes implementing robust data encryption methods and access controls to limit data exposure. Additionally, organizations should regularly conduct security audits and vulnerability assessments to identify and address any potential weaknesses in their data infrastructure. By prioritizing data security and privacy, businesses can build trust with their customers and stakeholders, and mitigate the risks associated with data breaches and regulatory non-compliance.
Ethical Use of Data Analytics
When it comes to data analytics, ethical considerations are of utmost importance. Organizations must ensure that they follow ethical guidelines and standards to protect participant confidentiality and maintain trust. Data collection is a critical step in the analytics process, and it is essential to ensure accurate and unbiased data gathering. This can be achieved by using reliable tools for surveys and interviews and maintaining consistency in data collection methods. Once the data is collected, the next step is to interpret the findings and draw insights. Identifying key patterns and trends is crucial in transforming raw data into actionable insights that guide product development. It is also important to combine behavior data with customer information to gain a comprehensive understanding of user preferences and needs. Responsible data analytics tools prioritize privacy and security, providing features such as real-time analytics, alerts and notifications, and data visualization for easy comprehension. By adhering to ethical practices and leveraging data analytics effectively, organizations can make informed decisions and drive business growth.
Compliance with Data Protection Regulations
Compliance with data protection regulations is crucial in the field of data analytics. Organizations must ensure that they adhere to laws and regulations regarding the collection, storage, and use of data. This includes implementing measures to protect the privacy and security of personal information, as well as obtaining necessary consent from individuals. Failure to comply with these regulations can result in severe penalties and damage to the organization's reputation.
To ensure compliance, organizations should:
- Conduct regular audits to assess data protection practices
- Implement robust data security measures, such as encryption and access controls
- Provide training to employees on data protection policies and procedures
- Establish clear procedures for handling data breaches
It is important for organizations to prioritize data protection and take proactive steps to mitigate risks and ensure compliance with regulations.
Challenges and Limitations of Data Analytics
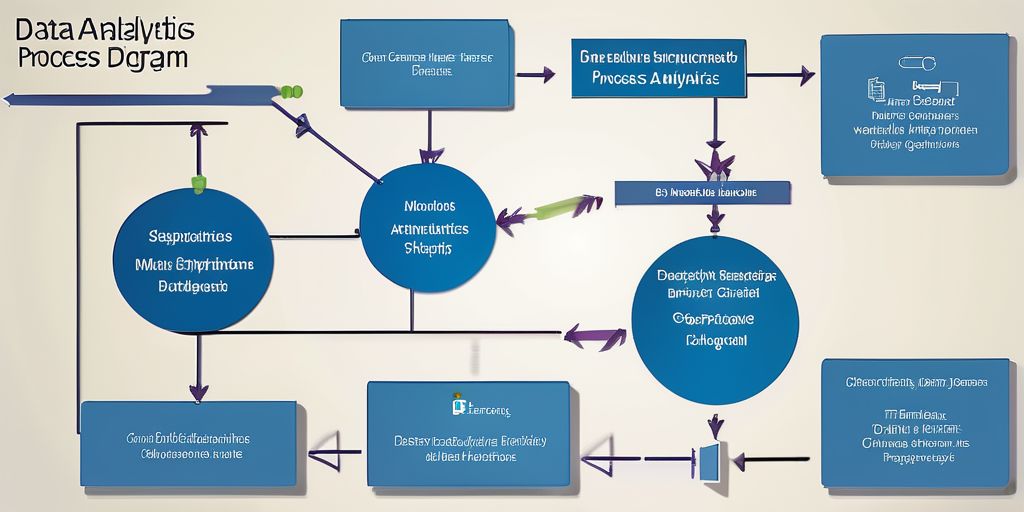
Data Quality and Data Integration Issues
Data quality and data integration are crucial aspects of data analytics. Ensuring the accuracy, completeness, and consistency of data is essential for obtaining reliable insights. However, organizations often face challenges in maintaining data quality and integrating data from various sources.
One common issue is the presence of duplicate or inconsistent data. This can occur when data is collected from multiple systems or sources without proper validation and cleansing processes. Inaccurate or incomplete data can lead to erroneous analysis and misleading conclusions.
Another challenge is data integration, which involves combining data from different sources to create a unified view. Incompatibility in data formats, structures, or naming conventions can make integration complex and time-consuming. It requires careful mapping, transformation, and validation to ensure the integrity and consistency of the integrated data.
To address these issues, organizations should establish data governance practices and implement data quality assurance measures. This includes defining data standards, conducting regular data audits, and implementing data cleansing and validation processes. Additionally, investing in data integration tools and technologies can streamline the integration process and improve data accuracy and consistency.
In summary, data quality and data integration are critical for effective data analytics. By addressing these challenges and implementing appropriate measures, organizations can ensure the reliability and usefulness of their data insights.
Lack of Skilled Data Analysts
The field of data analytics is rapidly growing, and there is a high demand for skilled data analysts. However, finding professionals with the right expertise can be a challenge. To address this issue, organizations can take several steps:
- Invest in Training and Development: Provide continuous learning opportunities for your business analysis team. Encourage them to update their knowledge about new technologies, tools, and best practices in data analytics.
- Collaborate with Educational Institutions: Build partnerships with universities and colleges to create internship programs or offer specialized courses in data analytics. This can help bridge the gap between academia and industry.
- Foster a Data-Driven Culture: Create an environment that values data-driven decision making. Encourage employees to develop data analysis skills and provide resources for them to enhance their expertise.
- Leverage Outsourcing and Consulting Services: If hiring skilled data analysts internally is not feasible, consider outsourcing or consulting services. This can provide access to specialized expertise and help meet short-term data analysis needs.
By implementing these strategies, organizations can overcome the challenge of a lack of skilled data analysts and leverage the power of data analytics to drive business insights and growth.
Ethical and Legal Challenges
Ethical dilemmas in data analytics refer to the complex moral and ethical issues that arise when organizations and individuals collect, process, analyze, and interpret data. These dilemmas can include concerns about privacy, consent, bias, transparency, and fairness. It is essential for businesses to navigate these challenges responsibly and ethically to maintain trust and credibility.
To address ethical and legal challenges in data analytics, organizations should:
- Obtain informed consent from participants
- Protect participant confidentiality
- Follow ethical guidelines and standards
Implementing these measures ensures that data collection and analysis are conducted in a responsible and ethical manner, respecting the rights and privacy of individuals involved. By prioritizing ethical considerations, businesses can build a strong foundation for their data analytics initiatives and foster trust with their stakeholders.
Implementing Data Analytics in Business
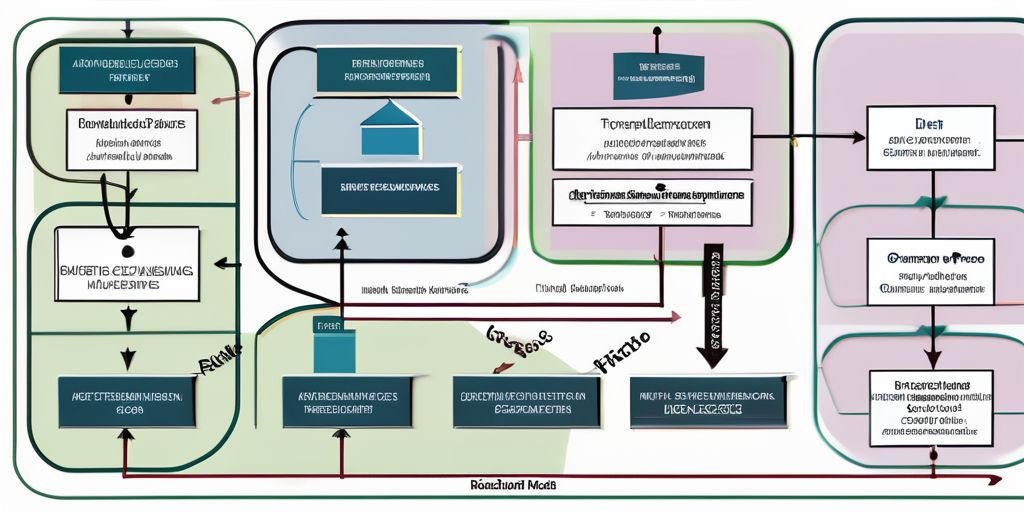
Building a Data-Driven Culture
Building a data-driven culture is essential for organizations looking to leverage the power of data analytics in their decision-making processes. It involves creating an environment where data is valued and used to drive insights and actions. Here are some key steps to building a data-driven culture:
- Promote Data Literacy: Ensure that employees have the necessary skills and knowledge to understand and interpret data. Provide training and resources to improve data literacy across the organization.
- Encourage Data-Backed Decision Making: Foster a culture where decisions are based on data and evidence rather than intuition or personal biases. Encourage employees to use data analytics to inform their decision-making processes.
- Establish Data Governance: Implement processes and policies to ensure the quality, integrity, and security of data. This includes defining data standards, establishing data ownership, and enforcing data privacy regulations.
- Create a Collaborative Environment: Encourage cross-functional collaboration and knowledge sharing to facilitate the use of data across different departments. Foster a culture of collaboration and teamwork to drive data-driven initiatives.
- Measure and Track Data-Driven Success: Establish metrics and key performance indicators (KPIs) to measure the impact of data-driven initiatives. Regularly track and evaluate the success of data-driven projects to identify areas for improvement.
By following these steps, organizations can create a data-driven culture that empowers employees to make informed decisions and drive business growth.
Selecting the Right Tools and Technologies
When it comes to selecting the right tools and technologies for data analytics, it is important to consider your specific business needs and objectives. There are a wide range of tools available in the market, each with its own strengths and capabilities. Here are some key factors to consider:
- Functionality: Ensure that the tools you choose have the necessary functionality to meet your data analytics requirements.
- Scalability: Consider the scalability of the tools, especially if you anticipate an increase in data volume in the future.
- Integration: Look for tools that can seamlessly integrate with your existing systems and technologies.
- Ease of use: Choose tools that are user-friendly and intuitive, as this will facilitate adoption and usage by your team.
It is also important to stay updated on the latest trends and advancements in data analytics tools. This will help you make informed decisions and ensure that you are leveraging the most effective tools for your business.
Overcoming Resistance to Change
To successfully implement data analytics in business, it is important to address and overcome resistance to change. Here are some practical tips for organizations:
- Encourage active communication: Clear, open communication is crucial for successful digital transformation. Encourage regular dialogue between business analysts, IT teams, and other stakeholders to ensure everyone understands the transformation efforts’ objectives and progress.
- Manage change and facilitate adoption: Digital transformation often involves significant change, and managing this change is another critical role for business analysts. They help to prepare stakeholders for the changes, facilitate training, and assist in overcoming resistance. They ensure that the digital transformation is technically successful, accepted, and adopted by the users.
- Measure success and continuous improvement: Once a digital transformation initiative is implemented, it is important to measure its success and continuously improve. Business analysts play a key role in defining success metrics, monitoring progress, and identifying areas for improvement.
- Implement agile practices: Adopting agile practices can make the implementation of data analytics more adaptable. Breaking down the process into manageable tasks and iterations allows for quick adjustments based on emerging findings and changing requirements.
- Invest in training and development: Continuous investment in the training and development of the team is essential. Staying updated on the latest research methodologies and tools ensures that the team remains well-equipped to leverage data analytics effectively.
Successfully overcoming resistance to change is crucial for the successful implementation of data analytics in business. By following these tips, organizations can navigate the challenges and drive positive change.
Implementing Data Analytics in Business is crucial for companies to stay competitive in today's data-driven world. By harnessing the power of data analytics, businesses can gain valuable insights and make informed decisions. At OptimizDBA Database Optimization Consulting, we specialize in helping businesses optimize their databases to improve performance and efficiency. With our expertise and experience, we can help you experience transaction speeds that are at least twice as fast as before. Our average speeds are often 100 times, 1000 times, or even higher! We guarantee a significant increase in performance. As a trusted industry leader in remote DBA services since 2001 with over 500 clients, we have the knowledge and skills to deliver exceptional results. Contact us today to learn how we can help your business thrive.
Conclusion
In conclusion, data analytics is a powerful tool for eliciting business insights. By leveraging behavioral analytics tools, businesses can collect and analyze data to uncover valuable insights that drive informed decision-making. Combining quantitative metrics with qualitative insights strengthens the credibility of findings and provides a comprehensive understanding of user behavior. Additionally, implementing a data-driven strategy and iterating based on feedback allows businesses to continuously improve and meet customer needs. With the right tools and approach, data analytics can transform raw data into actionable conclusions that guide product development and enhance business performance.
Frequently Asked Questions
What is the role of data analytics in decision making?
Data analytics helps businesses make informed decisions by analyzing data and extracting valuable insights.
How does data analytics drive business growth?
Data analytics enables businesses to identify opportunities, optimize processes, and make data-driven decisions that lead to growth.
What are the benefits of implementing data analytics in business?
Implementing data analytics in business can lead to improved efficiency, better decision making, increased competitiveness, and enhanced customer satisfaction.
What are the steps involved in collecting and preparing data for analysis?
The steps involved in collecting and preparing data for analysis include identifying relevant data sources, data cleaning and quality assurance, and data integration and transformation.
What are some exploratory data analysis techniques?
Some exploratory data analysis techniques include descriptive statistics and data visualization, identifying patterns and trends in data, and correlation and regression analysis.
What are some advanced data analytics methods?
Some advanced data analytics methods include predictive analytics and machine learning, text mining and natural language processing, and social network analysis and graph analytics.
How can data visualization and reporting help in data analytics?
Data visualization and reporting help in data analytics by presenting data in a visual and easily understandable format, enabling better interpretation and communication of insights.
What are the challenges and limitations of data analytics?
Some challenges and limitations of data analytics include data quality and integration issues, lack of skilled data analysts, and ethical and legal challenges.