Understanding the Importance of Data Analytics
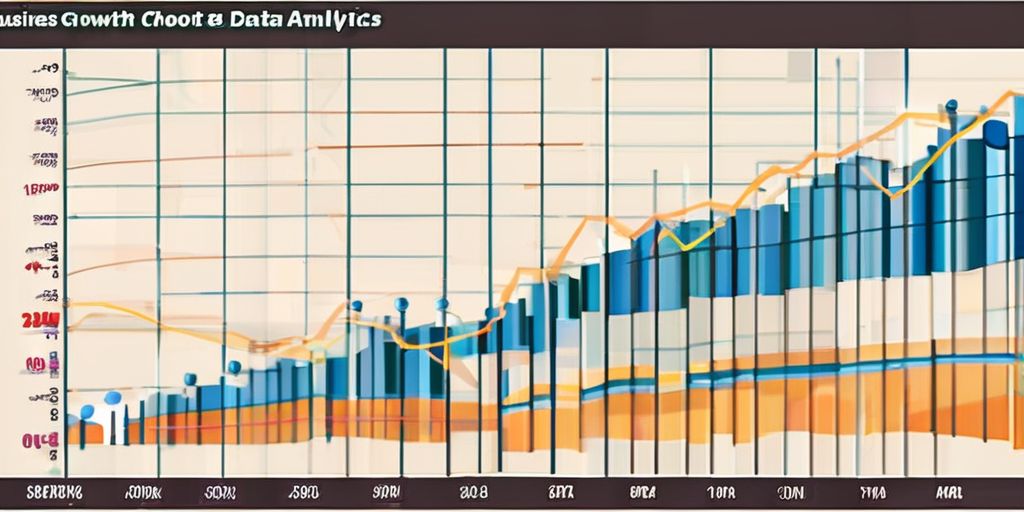
Why Data Analytics is Essential for Business Growth
Data-driven business development isn't just a fancy phrase--it's the compass that guides companies through the murky waters of the market. It’s the “why” to every strategic decision, from targeting new customers to finessing product offerings. Using data effectively means understanding not just where your business stands today, but where it has the potential to go. The real magic happens in the analysis—sifting through the numbers to spot patterns, opportunities, and even warning signs. This is where tools and technologies, like analytics software and customer relationship management systems, come into play. They're the sieves and magnifying glasses that help businesses dig into the details and make sense of the stats. But it's not just about having the right tools; it’s also about asking the right questions. What does this data point mean for our customer retention? How can we tweak our marketing strategy to tap into emerging trends? What does this mean for our overall business strategy? By leveraging data analytics, businesses can make informed decisions, drive growth, and stay ahead of the competition.
The Role of Data Analytics in Decision Making
Data analytics plays a crucial role in decision making for businesses. It provides valuable insights and helps companies make informed choices. By analyzing data, businesses can identify patterns, trends, and opportunities that may not be apparent at first glance. Data analytics software and tools act as sieves and magnifying glasses, allowing businesses to dig into the details and make sense of the stats. It's not just about having the right tools; it's also about asking the right questions. By understanding the meaning behind data points, businesses can make strategic decisions that drive growth and success.
Benefits of Implementing Data Analytics in Business
Implementing data analytics in business provides several key benefits. First, it allows companies to make data-driven decisions, using insights from the analysis to inform strategic choices. This leads to more informed and accurate decision-making, reducing the risk of making costly mistakes. Second, data analytics helps businesses identify trends and patterns in customer behavior, enabling them to personalize their marketing and sales strategies. By understanding customer preferences and needs, companies can tailor their offerings to better meet customer expectations. Third, data analytics improves operational efficiency by identifying areas for optimization and streamlining processes. By analyzing data on supply chain management, inventory levels, and logistics, businesses can make informed decisions to reduce costs and improve overall efficiency.
Collecting and Managing Data for Analysis
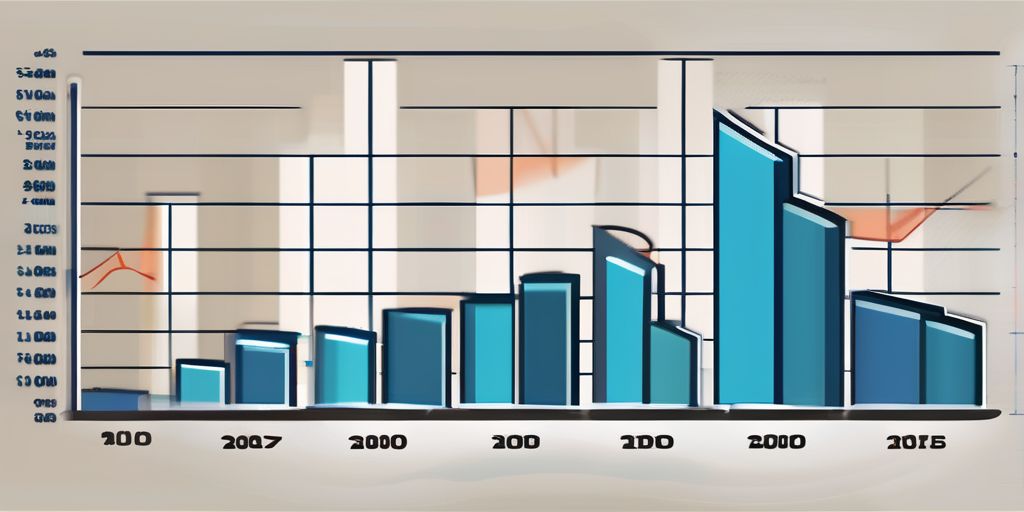
Choosing the Right Data Sources
When it comes to data analytics, choosing the right data sources is crucial for obtaining accurate and reliable insights. Accuracy is key in data analysis, as decisions and strategies are based on the information derived from the data. It is important to ensure that the data sources selected are relevant to the specific goals and objectives of the analysis. Additionally, considering the quality of the data sources is essential. Data sources should be reliable, up-to-date, and free from errors or biases.
To assist in the selection process, here are some factors to consider:
- Data Availability: Determine if the desired data is available and accessible for analysis.
- Data Relevance: Evaluate the relevance of the data to the specific analysis objectives.
- Data Reliability: Assess the reliability and credibility of the data sources.
- Data Compatibility: Ensure that the data sources are compatible with the analysis tools and techniques being used.
By carefully choosing the right data sources, businesses can ensure the accuracy and reliability of their data analytics efforts, leading to more informed decision-making and ultimately driving business growth.
Data Collection Methods and Tools
The real magic happens in the analysis—sifting through the numbers to spot patterns, opportunities, and even warning signs. This is where tools and technologies, like analytics software and customer relationship management systems, come into play. They're the sieves and magnifying glasses that help businesses dig into the details and make sense of the stats. But it's not just about having the right tools; it’s also about asking the right questions. What does this data point mean for our customer retention? How can we tweak our marketing strategy to tap into emerging trends?
Data Quality and Cleaning Techniques
Data quality and cleaning are crucial steps in the data analytics process. Ensuring that the data used for analysis is accurate, complete, and consistent is essential for obtaining reliable insights. There are several techniques that can be employed to improve data quality and clean the data, including:
- Data validation: Verifying the integrity and accuracy of the data by checking for errors, inconsistencies, and outliers.
- Data cleansing: Removing or correcting any errors, duplicates, or irrelevant data.
- Data transformation: Converting data into a standardized format or structure to facilitate analysis.
These techniques help to eliminate noise and ensure that the data is suitable for analysis, leading to more accurate and reliable insights.
Analyzing Data to Gain Insights
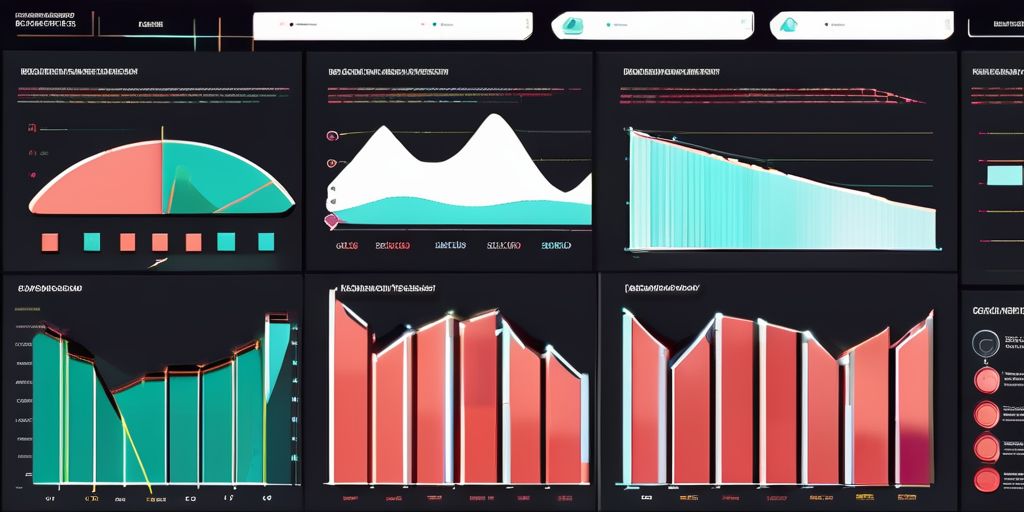
Exploratory Data Analysis Techniques
Exploratory Data Analysis (EDA) is a crucial step in the data analysis process. It involves examining and visualizing the data to uncover patterns, trends, and relationships. EDA helps in understanding the data distribution, identifying outliers, and gaining initial insights. Some common techniques used in EDA include:
- Histograms: A graphical representation of the data distribution.
- Scatter plots: Used to visualize the relationship between two variables.
- Box plots: Displaying the distribution of a variable and identifying outliers.
EDA provides a foundation for further analysis and decision-making.
Statistical Analysis for Business Insights
Statistical analysis plays a crucial role in gaining valuable insights for business growth. It involves examining and interpreting data to identify patterns, trends, and correlations. By applying statistical techniques, businesses can make informed decisions and develop effective strategies. Hypothesis testing is a common statistical analysis method used to determine the significance of relationships between variables. It helps businesses validate assumptions and draw meaningful conclusions.
In addition to hypothesis testing, businesses can also use regression analysis to understand the relationship between variables and make predictions. Regression analysis allows businesses to quantify the impact of different factors on business outcomes. For example, it can help determine the effect of marketing spend on sales revenue.
To present structured, quantitative data, businesses can use tables in their analysis. Tables provide a clear and concise way to present numerical information. They can be used to compare different variables, summarize data, and highlight key findings.
In summary, statistical analysis is a powerful tool for gaining insights and driving business growth. By leveraging statistical techniques and presenting data in a structured manner, businesses can make data-driven decisions and optimize their strategies.
Data Visualization for Effective Communication
Data visualization is a powerful tool for communicating complex information in a clear and concise manner. By representing data visually, businesses can easily identify trends, patterns, and insights that may not be apparent in raw data. Visualizing data allows for better understanding and interpretation, enabling effective decision-making. It also helps in presenting data to stakeholders and clients, making it easier for them to grasp key information. Whether it's through charts, graphs, or interactive dashboards, data visualization enhances communication and facilitates data-driven discussions.
Using Predictive Analytics for Business Forecasting
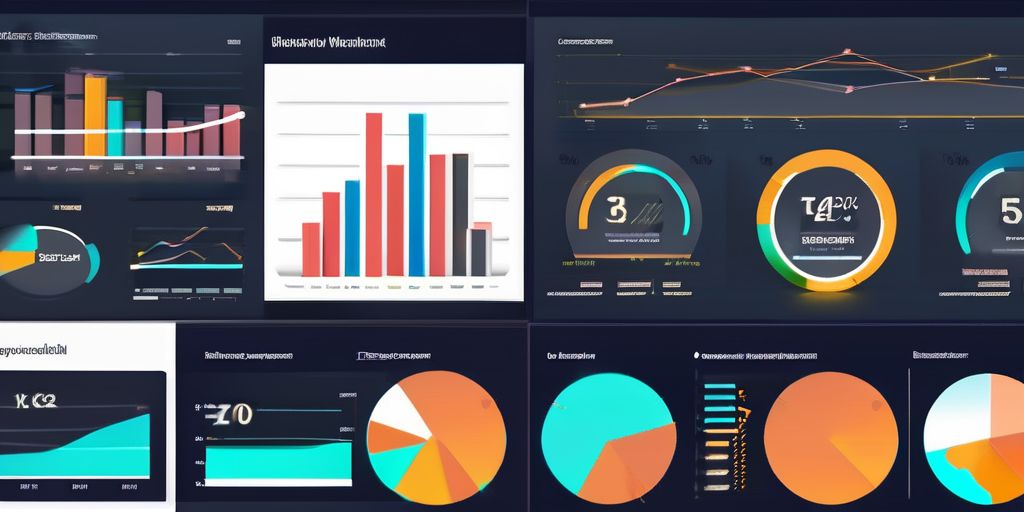
Understanding Predictive Modeling
Predictive modeling is a powerful machine learning technique that enables companies to forecast future outcomes for business growth. By analyzing historical data and identifying patterns, predictive modeling can provide valuable insights and help businesses make informed decisions. With the right algorithms and data, companies can accurately predict customer behavior, market trends, and other key factors that impact business success. Implementing predictive modeling can give businesses a competitive edge by enabling them to proactively respond to changes and optimize their strategies.
Applying Machine Learning Algorithms
Applying machine learning algorithms is a crucial step in leveraging data analytics for business growth. Machine learning algorithms enable businesses to uncover patterns and insights from large datasets, allowing for more accurate predictions and informed decision-making. These algorithms use statistical techniques to train models on historical data and make predictions on new data points. By implementing machine learning algorithms, businesses can automate processes, optimize operations, and improve the overall efficiency of their operations.
Evaluating and Improving Predictive Models
Evaluating and improving predictive models is a crucial step in leveraging data analytics for business growth. Once a predictive model has been developed, it is important to assess its performance and make necessary improvements. This involves analyzing the model's accuracy, precision, recall, and other relevant metrics to evaluate its effectiveness. Iterative testing and refinement are key to enhancing the predictive power of the model.
To improve predictive models, data scientists can employ various techniques such as feature engineering, ensemble methods, and hyperparameter tuning. These techniques help optimize the model's performance and ensure it is robust and reliable. Additionally, regular monitoring and updating of the model is essential to adapt to changing business dynamics and maintain its accuracy over time.
When evaluating and improving predictive models, it is important to consider the business context and align the model's objectives with the organization's goals. This ensures that the model provides actionable insights and drives business growth.
In summary, evaluating and improving predictive models is a continuous process that involves assessing performance, refining techniques, and aligning with business objectives. By investing in this process, businesses can harness the power of data analytics to make informed decisions and drive sustainable growth.
Driving Business Growth with Data-Driven Strategies
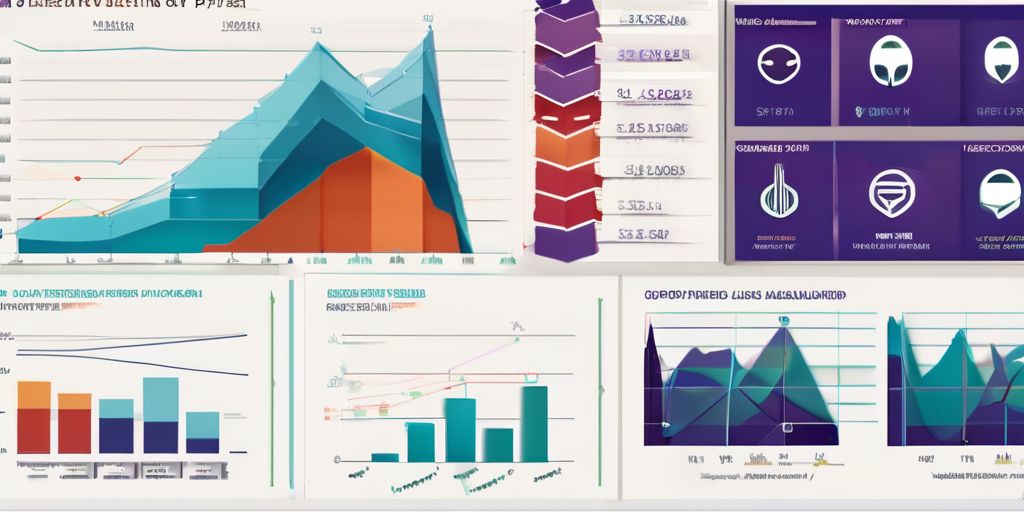
Identifying Growth Opportunities through Data Analysis
Data-driven business development isn't just a fancy phrase--it's the compass that guides companies through the murky waters of the market. It’s the “why” to every strategic decision, from targeting new customers to finessing product offerings. Picture a business world where every move is backed by solid facts and figures--not just gut feelings or guesswork. That's the power of data—it illuminates the path to progress with the bright light of knowledge. Using data effectively means understanding not just where your business stands today, but where it has the potential to go tomorrow. The real magic happens in the analysis—sifting through the numbers to spot patterns, opportunities, and even warning signs. This is where tools and technologies, like analytics software and customer relationship management systems, come into play. They're the sieves and magnifying glasses that help businesses dig into the details and make sense of the stats. But it's not just about having the right tools; it’s also about asking the right questions. What does this data point mean for our customer retention? How can we tweak our marketing strategy to tap into emerging trends? What does this
Optimizing Marketing and Sales Strategies
The real magic happens in the analysis—sifting through the numbers to spot patterns, opportunities, and even warning signs. This is where tools and technologies, like analytics software and customer relationship management systems, come into play. They're the sieves and magnifying glasses that help businesses dig into the details and make sense of the stats. But it's not just about having the right tools; it’s also about asking the right questions. What does this data point mean for our customer retention? How can we tweak our marketing strategy to tap into emerging trends? What does this bright light of knowledge. Using data effectively means understanding not just where your business stands today, but where it has the potential to go tomorrow. The How-To of Harnessing Data: Strategies for Insight So, how do businesses grab hold of this data and wield it to their advantage? It starts with collecting the right information—customer behaviours, market trends, sales figures, online interactions, and more. But collection is just step one. The real magic happens in the analysis—sifting through the numbers to spot patterns, opportunities, and even warning signs. This is where tools
Improving Operational Efficiency with Data Analytics
Improving operational efficiency is a key goal for businesses looking to streamline their processes and maximize productivity. By leveraging data analytics, companies can gain valuable insights into their operations and identify areas for improvement. Analyzing data from various sources allows businesses to identify bottlenecks and inefficiencies in their workflows. With this information, organizations can make data-driven decisions to optimize their processes and reduce costs. Additionally, data analytics can help businesses forecast demand more accurately, allowing for better resource allocation and inventory management. By using data analytics to improve operational efficiency, companies can gain a competitive edge in today's fast-paced business landscape.
Leveraging Customer Insights for Personalization
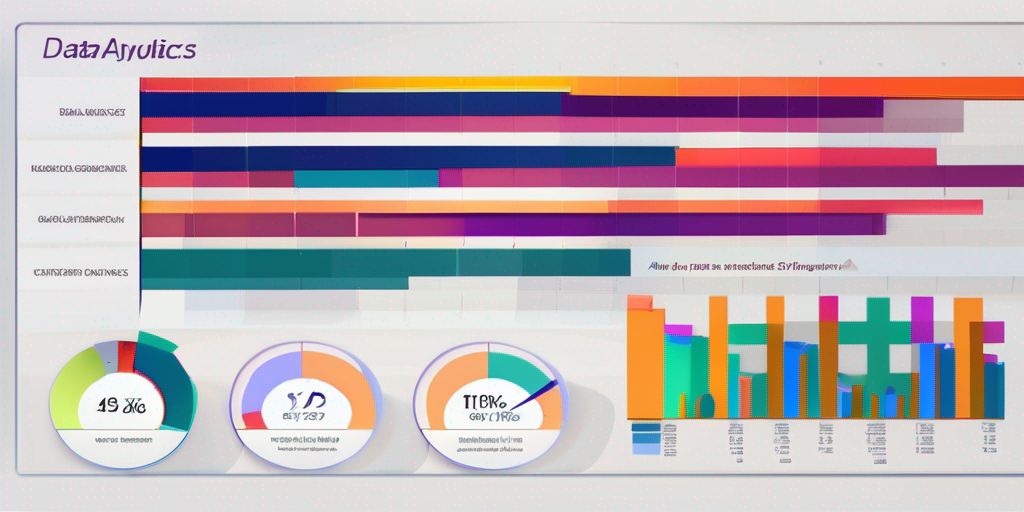
Segmenting Customers for Targeted Marketing
Segmenting customers is a crucial step in developing targeted marketing strategies. By dividing the customer base into distinct segments based on their characteristics, behaviors, or preferences, businesses can tailor their marketing efforts to each segment's specific needs. This allows for more personalized and relevant communication, which can lead to higher engagement and conversion rates.
There are various methods and criteria that can be used for customer segmentation. Some common approaches include demographic segmentation, psychographic segmentation, and behavioral segmentation. Each method provides unique insights into customer preferences and can help businesses identify the most valuable segments to target.
Implementing customer segmentation requires the collection and analysis of relevant data. This data can include customer demographics, purchase history, online behavior, and more. By leveraging data analytics tools and techniques, businesses can gain a deeper understanding of their customers and create more effective marketing strategies.
Segmenting customers for targeted marketing is an ongoing process. As customer preferences and behaviors evolve, businesses need to regularly review and update their segmentation strategies to ensure they remain relevant and effective.
Understanding Customer Behavior through Data
Understanding customer behavior through data is crucial for businesses looking to personalize their marketing strategies and create tailored customer experiences. By analyzing customer interactions, purchase histories, and engagement with entrepreneurial content, businesses can target specific customer segments with relevant offers and promotions. This level of personalization not only adds value to the customer journey but also fosters loyalty and satisfaction. Additionally, data analysis allows businesses to stay agile and adapt to changing market trends and consumer preferences. Real-time data analysis acts as an early warning system, providing businesses with the information they need to pivot, adapt, and stay ahead of the competition.
Creating Personalized Customer Experiences
Crunching numbers also leads to something a little warmer and more personal—tailoring experiences that resonate with customers. In a world where folks can feel like just another entry in a spreadsheet, businesses that use data to personalize services or products truly stand out from the crowd. It’s noticing that a customer always buys eco-friendly products and sends them a discount for the new green range, or seeing that someone checks out the same guitar daily and reaches out with advice or a special offer. And as we explore the realm of creating tailored customer experiences using data, it’s crucial to recognize the value of offering personalized incentives.
Using Data Analytics to Enhance Product Development
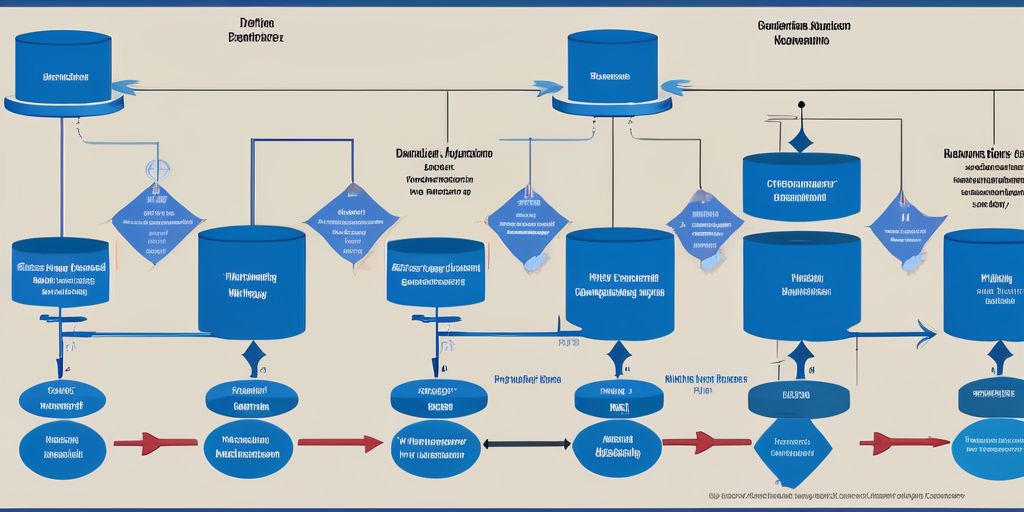
Identifying Customer Needs and Preferences
Understanding customer needs and preferences is crucial for businesses to effectively target their marketing efforts and create personalized customer experiences. By analyzing customer interactions, purchase histories, and engagement with entrepreneurial content, businesses can identify potential or existing entrepreneurs among their customer base. This allows them to tailor their offers and promotions to this specific segment, demonstrating a keen understanding of their individual needs and aspirations. Implementing data analytics in this process enables businesses to elevate customer experience, fostering loyalty and satisfaction.
Optimizing Product Features and Design
When it comes to optimizing product features and design, data analytics plays a crucial role. By analyzing customer feedback and behavior, businesses can identify areas for improvement and make data-driven decisions. Big data insights can provide valuable information on customer preferences, allowing companies to create products that exceed expectations. Additionally, data analytics can help businesses identify trends and patterns in user behavior, enabling them to optimize product features and design for maximum customer satisfaction.
Testing and Iterating with Data Analytics
Testing and iterating with data analytics is a crucial step in the product development process. It allows businesses to gather insights and make data-driven decisions to improve their products. By analyzing the data collected from user feedback, A/B testing, and other sources, companies can identify areas for improvement and optimize their product features and design. This iterative approach helps businesses create products that better meet customer needs and preferences.
Implementing Data Analytics in Supply Chain Management
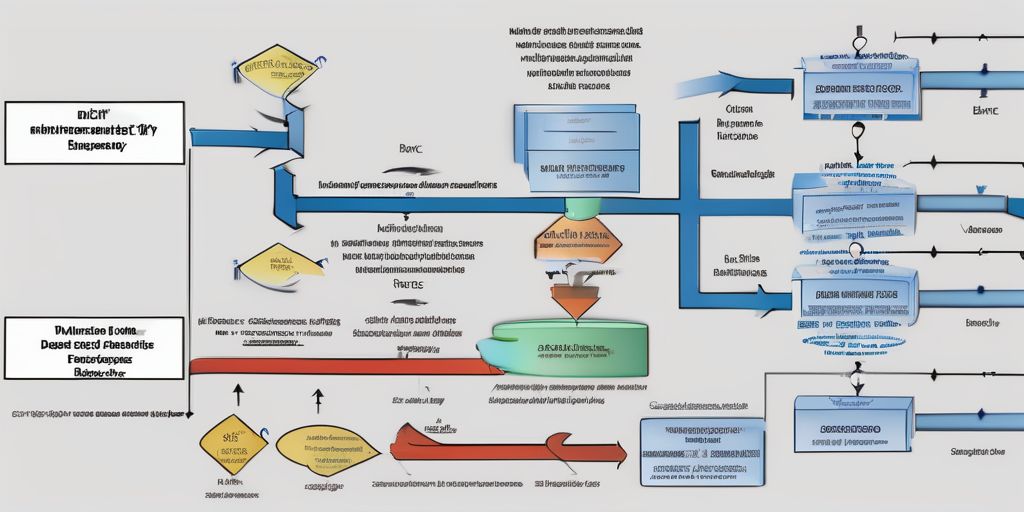
Demand Forecasting and Inventory Optimization
Demand forecasting and inventory optimization are crucial aspects of supply chain management. By accurately predicting customer demand and optimizing inventory levels, businesses can ensure efficient operations and minimize costs. Demand forecasting involves analyzing historical data, market trends, and customer behavior to estimate future demand for products or services. This information helps businesses plan production, procurement, and distribution activities. Inventory optimization focuses on finding the right balance between holding excess inventory and facing stockouts. By using data analytics techniques, businesses can determine the optimal inventory levels to meet customer demand while minimizing carrying costs and obsolescence risks.
Improving Supplier Performance with Data
Improving supplier performance is crucial for optimizing supply chain management and ensuring smooth operations. By leveraging data analytics, businesses can gain valuable insights into supplier performance and identify areas for improvement. Here are some strategies to enhance supplier performance:
- Track Key Performance Indicators (KPIs): Implement a table to monitor and measure supplier performance based on important metrics such as on-time delivery, quality, and cost.
- Identify Bottlenecks and Risks: Analyze data to identify bottlenecks in the supply chain and potential risks that may impact supplier performance. This can help businesses proactively address issues and minimize disruptions.
- Establish Clear Communication Channels: Maintain open and transparent communication with suppliers to ensure mutual understanding of expectations and requirements. This can help build strong relationships and improve performance.
- Collaborate on Continuous Improvement: Work closely with suppliers to identify opportunities for process optimization and continuous improvement. Use data analytics to track the effectiveness of implemented changes and drive performance enhancements.
- Implement Supplier Scorecards: Develop supplier scorecards to evaluate and compare supplier performance over time. This can provide a structured approach for supplier evaluation and enable data-driven decision-making.
Improving supplier performance through data analytics can lead to cost savings, increased efficiency, and better overall supply chain management.
Streamlining Logistics and Distribution
Streamlining logistics and distribution is crucial for optimizing supply chain management. By implementing data analytics, businesses can gain valuable insights into their supply chain operations and identify areas for improvement. One effective way to streamline logistics is by using predictive analytics to forecast demand and optimize inventory levels. This helps businesses avoid stockouts and reduce excess inventory, leading to cost savings and improved customer satisfaction. Additionally, data analytics can be used to improve supplier performance by analyzing historical data and identifying opportunities for collaboration and process optimization.
Implementing Data Analytics in Supply Chain Management is crucial for businesses to gain insights and make informed decisions. At OptimizDBA Database Optimization Consulting, we specialize in improving the performance of your database, resulting in faster transaction speeds. With over 20 years of experience, we have helped over 500 clients achieve significant increases in performance. Whether you need remote DBA services or assistance with database optimization, we are here to help. Contact us today to experience transaction speeds that are at least twice as fast as before. Don't miss out on the opportunity to optimize your database and improve your business efficiency!
Conclusion
In conclusion, data analytics is the driving force behind business growth in the 21st century. By harnessing the power of data, businesses can make informed decisions, identify opportunities, and stay ahead of the competition. Data-driven development allows companies to understand their customers better, tailor experiences, and improve customer satisfaction. It provides the foundation for agile and responsive business strategies, enabling businesses to adapt to changing market trends and consumer preferences. In a world where information is the new oil, data analytics is the combustion engine that propels businesses towards success.
Frequently Asked Questions
Why is data analytics important for business growth?
Data analytics provides valuable insights into customer behaviors, market trends, and sales figures, which can help businesses make informed decisions and identify growth opportunities.
How does data analytics contribute to decision making?
Data analytics helps businesses make data-driven decisions by analyzing and interpreting large volumes of data to uncover patterns, trends, and correlations.
What are the benefits of implementing data analytics in business?
Implementing data analytics in business can lead to improved operational efficiency, optimized marketing and sales strategies, and better customer experiences through personalized targeting.
How can businesses choose the right data sources?
Businesses should choose data sources that are relevant to their industry and objectives, ensuring the data collected is accurate, reliable, and up-to-date.
What are some common data collection methods and tools?
Common data collection methods include surveys, interviews, observations, and web scraping. Tools such as data management systems and analytics software can also facilitate data collection.
How can businesses ensure data quality and cleanliness?
To ensure data quality and cleanliness, businesses can establish data governance policies, implement data validation processes, and regularly clean and update their datasets.
What is exploratory data analysis?
Exploratory data analysis is a technique used to analyze and summarize data sets to uncover patterns, relationships, and anomalies, which can guide further analysis and decision making.
How can data visualization enhance data analysis?
Data visualization techniques, such as charts, graphs, and dashboards, can help businesses visually represent complex data sets, making it easier to identify trends, patterns, and insights.