Understanding Predictive Analytics
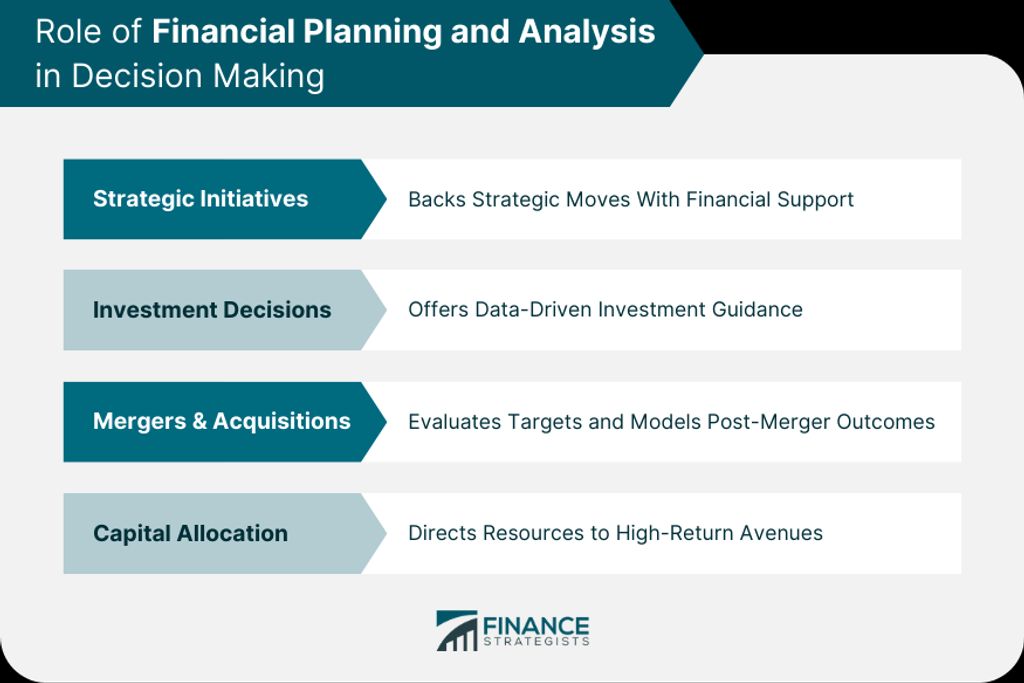
Core Concepts of Predictive Analytics
At the heart of predictive analytics is the ability to forecast future events, trends, and behaviors using historical and current data. This field combines statistical techniques, such as predictive modeling, machine learning, and data mining, to analyze vast amounts of data and uncover patterns that can inform business decisions.
Key elements of predictive analytics include:
- Data mining to discover patterns
- Predictive modeling to forecast outcomes
- Machine learning to improve predictions over time
Remember, the accuracy of predictive analytics heavily relies on the quality of the data used. Incomplete or poor-quality data can lead to unreliable forecasts.
Businesses must also consider the tools and expertise required to effectively implement predictive analytics. It's not just about having the right software but also ensuring that your team has the necessary skills to use these tools and interpret the results. Continuous training and development are crucial for maintaining the relevance and accuracy of predictive models.
Benefits of Predictive Analytics in Business
Predictive analytics offers the foresight needed to navigate the business landscape more effectively. It helps forecast sales, market trends, and customer behavior, enabling businesses to plan strategies more effectively. Businesses can optimize inventory levels by predicting future demand and avoiding overstocking or stockouts. Analyzing past sales data and market trends can help set the correct prices to maximize profits and market. Predictive analytics revolutionizes industries by providing the best assessment of what will happen in the future. Benefits include increased accuracy in forecasts, improved resource allocation, enhanced risk management, and the ability to create more effective, targeted strategies that can give businesses a competitive edge. Predictive analytics can streamline operations, optimize supply chains, and ensure that capital is not tied up in surplus inventory, thereby safeguarding the financial health and stability of the business.
Implementing Predictive Analytics in Business
Predictive analytics uses data, statistical algorithms, AI, and machine learning techniques to identify the likelihood of future outcomes based on historical data. It's a bit like a weather forecast for your business, helping you predict sunny days and stormy periods. Predictive analytics helps businesses anticipate customer needs, manage risks, and tailor marketing campaigns so they hit the mark every time. But here's the catch: it's not foolproof. Like any forecast, it's based on probabilities. There's always a margin of error, but it's still much better.
Integration of Predictive Analytics
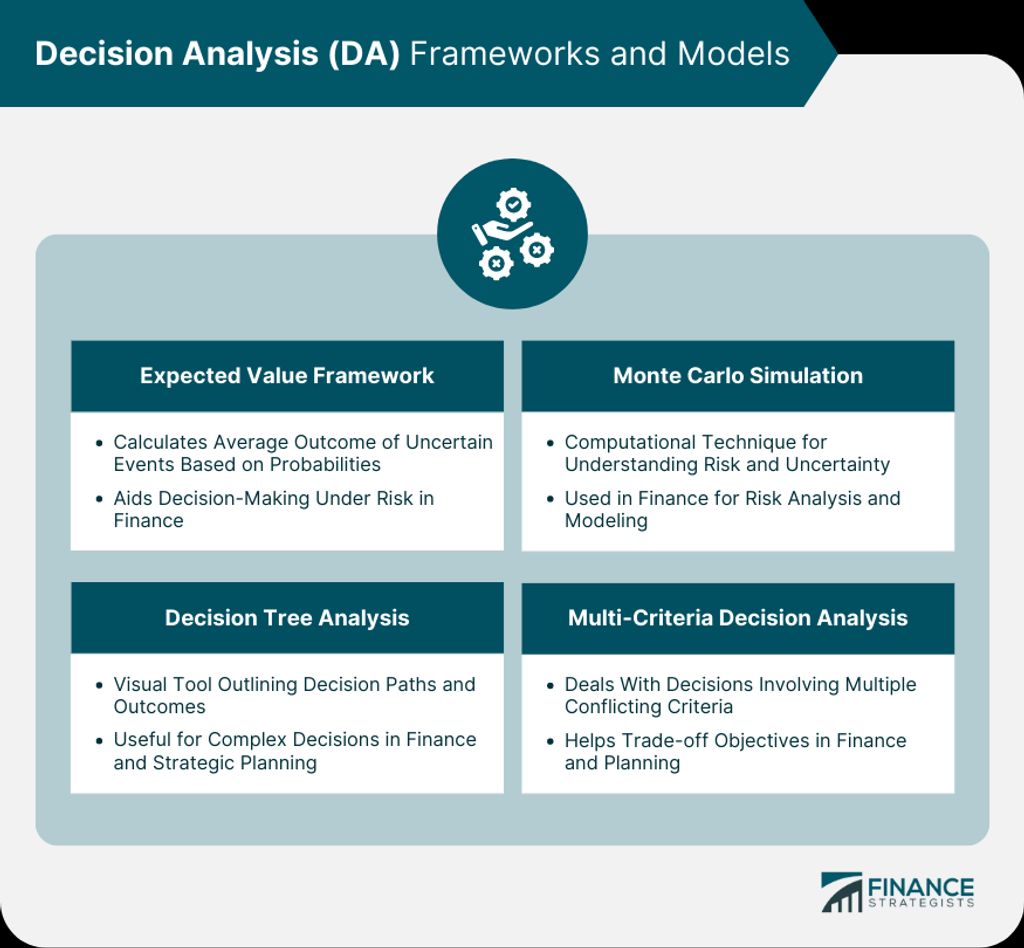
Integrating Predictive Analytics into Business Operations
The integration of predictive analytics into business operations is a transformative process that enables companies to harness the power of predictive analysis. By leveraging historical data, statistical algorithms, and machine learning techniques, businesses can anticipate future trends, understand customer behaviors, and make data-driven decisions that propel growth and success.
To effectively integrate predictive analytics, organizations must align their operations with predictive models that are tailored to their unique industry and market dynamics. This alignment involves a series of steps:
- Identifying key areas of operation that will benefit from predictive insights.
- Collecting and preparing high-quality data that feeds into the predictive models.
- Ensuring that the predictive models are finely tuned to the specific parameters of the business.
- Integrating these models seamlessly with existing business systems.
Tip: Start small by applying predictive analytics to a single business function or process. This allows for manageable testing and refinement of predictive models before scaling up to more complex operations.
The ultimate goal is to create a symbiotic relationship between sophisticated analytical tools and in-depth business knowledge. This synergy allows organizations to not only forecast with greater accuracy but also to optimize efficiency, personalize customer experiences, and mitigate risks, thereby driving informed strategic planning and operational excellence.
Challenges of Integrating Predictive Analytics
Integrating predictive analytics into a business's existing systems can be a complex task, often fraught with challenges that can impede its successful adoption. One of the primary hurdles is ensuring the quality of data; inaccurate or poor-quality data will inevitably lead to skewed results and unreliable forecasts that could misguide rather than inform strategic decisions.
Another significant challenge is the seamless integration of predictive models into the existing workflow. The process must be intuitive; if it introduces complexity or confusion, it can reduce user adoption and trust in the system. This limitation can severely impact the effectiveness of predictive insights in driving business operations.
Moreover, businesses must possess the right mix of skills — from data scientists and analysts to IT professionals — to interpret data, manage technology, and apply insights effectively. Here are some steps to consider for overcoming these challenges:
- Start with small, manageable projects to ensure gradual improvements.
- Ensure ongoing training and development for your team.
- Align predictive insights with your strategic goals for maximum impact.
Tip: Don't expect to revolutionize your business overnight with predictive analytics. It's a gradual process that requires patience and persistent refinement.
Best Practices for Seamless Integration
Ensuring the integration of predictive models into existing workflow process should be seamless and intuitive. Complexity or confusion in the integration process will reduce user adoption and trust in the system, limiting the effectiveness of predictive insights in driving business operations. Additionally, businesses must possess high-quality data to avoid skewed results and unreliable forecasts. Poor-quality data can misguide rather than inform strategic decisions. Implementing a structured, quantitative data table can help present data succinctly and effectively, while a bulleted list can be used for less structured content such as steps or qualitative points.
Data Management for Predictive Analytics
Quality Management of Data for Accurate Predictions
The foundation of effective predictive analytics lies in the data quality. Ensuring that data is accurate, relevant, and free from errors is paramount for the reliability of predictions. It's not just about the volume of data; the quality of each data point is crucial.
A robust data quality management system involves several key steps:
- Data Cleansing: Removing inaccuracies and inconsistencies from data.
- Data Transformation: Converting data into a format suitable for analysis.
- Data Encoding: Preparing data for the predictive model by encoding categorical variables.
Tip: Always verify the relevance of your data to your business goals to avoid the pitfall of using incomplete or poorly chosen data sets.
The integration of predictive analytics tools should complement these data management practices. Select tools that align with your business needs and ensure your team has the right mix of skills to manage and interpret the data effectively. Remember, the success of predictive analytics is not instantaneous; it requires a gradual approach, starting with small projects and scaling over time.
Data Collection and Integration
Quality is paramount in prescriptive analytics, so organizations should ensure that they have access to accurate, relevant, and comprehensive data. Integration of data from various sources may be necessary, requiring robust data preprocessing to create a unified dataset suitable for analysis. Collaboration between data scientists, domain experts, and decision-makers is essential throughout the process. Domain experts provide valuable insights into the contextual nuances of decision-making, while data scientists leverage their analytical expertise to build and refine prescriptive. Implement a table for presenting structured, quantitative data. Ensure it's succinct and formatted correctly in Markdown. Use a bulleted or numbered list for less structured content, like steps, qualitative points, or a series of related items. Optionally, include a Markdown blockquote for important tips or advice, avoiding citing sources. Do not include images. Content should be split into multiple short paragraphs.
Data Privacy and Security Considerations
Managing Ethical and Privacy Concerns Challenge: The use of AI in decision-making raises concerns about privacy, data security, and ethical use of information. Consideration: Adhere to data protection laws and ethical guidelines. Be transparent about AI use and data collection methods with stakeholders. Overcoming Implementation Complexities Challenge: Integrating AI into existing systems and workflows can be technically complex and resource-intensive. Consideration: Start with small-scale implementations. Consider partnering with AI experts or vendors for a smoother integration.
Use the right tools: Choose tools that fit your business needs and can handle your specific data types and volumes.
Act on the results: Integrate the understanding you gain from predictive analytics into your business strategies to make better-informed decisions.
Be mindful of ethical implications: Consider the ethical implications of using customer data and your predictions.
Communicate results with relevant stakeholders: Communicate the findings from your predictive analytics to stakeholders to ensure alignment and transparency.
Privacy compliance: The collection of certain types of customer data for predictive analytics may be limited or prohibited by privacy laws in some countries. For instance, the General Data Protection Regulation (GDPR) in the European Union imposes strict rules on how personal data is collected and used. It also requires explicit consent for data usage. AI-driven platforms like Pecan can help overcome these challenges by helping marketers combine attribution and marketing mix modeling (MMM) techniques. This combination of analytics techniques addresses privacy compliance and ensures ethical use of customer data.
Predictive Analytics Tools and Technologies
Choosing the Right Predictive Analytics Tools
Businesses need to align their forecasting strategies with predictive models that are finely tuned to their specific industry parameters and market dynamics, creating a seamless marriage of data-driven insights and actionable business intelligence. Starting with a robust infrastructure is paramount when implementing predictive analytics in business forecasting, as it demands a stable and scalable platform that can handle vast amounts of data and complex computational processes; businesses should invest in advanced data management tools and analytic systems that can aggregate. Before anything else, know what you want to achieve. Are you looking to improve customer retention, increase sales, optimize operations, or something else? The accuracy of your predictions hinges on the quality management of your data. Ensure you collect comprehensive, relevant, and high-quality data from various sources. There's a plethora of predictive analytics tools out there. Choose one that aligns with your business needs and integrates well with your existing systems. You need a mix of skills — data scientists, analysts, IT professionals — to interpret data, manage technology, and apply insights effectively. Don’t expect to revolutionize your business overnight. Start with small, manageable projects. Gradual improvements lead to significant gains over time. Ensure your team understands how to use predictive analytics tools and interpret the results. Ongoing training and development are essential. Predictive insights should be a core part of your strategic implementation. Implement a table for presenting structured, quantitative data. Ensure it's succinct and formatted correctly in Markdown. Use a bulleted or numbered list for less structured content, like steps, qualitative points, or a series of related items. For more information about this, check out Pecan’s guide to choosing the right strategy and platform. Which companies use predictive analytics? Many large-scale businesses and multinational corporations such as Amazon,
Integration with Existing Systems
Integrating predictive analytics tools with existing systems is a critical step in leveraging their full potential. It's essential to ensure that the new tools can communicate effectively with the current infrastructure, which often involves data compatibility and system interoperability. This process can be complex, depending on the heterogeneity of the existing systems and the architecture of the analytics solutions.
To facilitate a smoother integration, businesses should consider the following points:
- Assess the current IT landscape and identify potential integration points.
- Establish clear data governance policies to maintain data integrity.
- Ensure that the analytics tools are compatible with existing data formats and databases.
- Plan for scalability to accommodate future growth and data volume increases.
Tip: Start with a pilot project to test the integration process and address any issues before scaling up.
Once integrated, predictive analytics can transform raw data into actionable insights, driving smarter business decisions. However, it's important to maintain a continuous improvement approach, regularly updating and refining the analytics tools to keep pace with evolving business needs and technology advancements.
Skills and Expertise Required
Choose one that aligns with your business needs and integrates well with your existing systems. You need a mix of skills — data scientists, analysts, IT professionals — to interpret data, manage technology, and apply insights effectively. Don’t expect to revolutionize your business overnight. Start with small, manageable projects. Gradual improvements lead to significant gains over time. Ensure your team understands how to use predictive analytics tools and interpret the results. Ongoing training and development are essential. Predictive insights should be a core part of your strategic business processes based on AI insights. This cultural shift can be instrumental in smoothly integrating AI into decision-making processes. As AI becomes more integral to business operations, having a team skilled in AI is imperative. Consider investing in training programs to build AI expertise within your organization. This might include workshops, courses, or partnering with academic institutions or AI training providers. With the power of AI comes responsibility. Ensure that your AI initiatives adhere to ethical guidelines and regulatory requirements. This includes being transparent.
Strategic Implementation of Predictive Insights
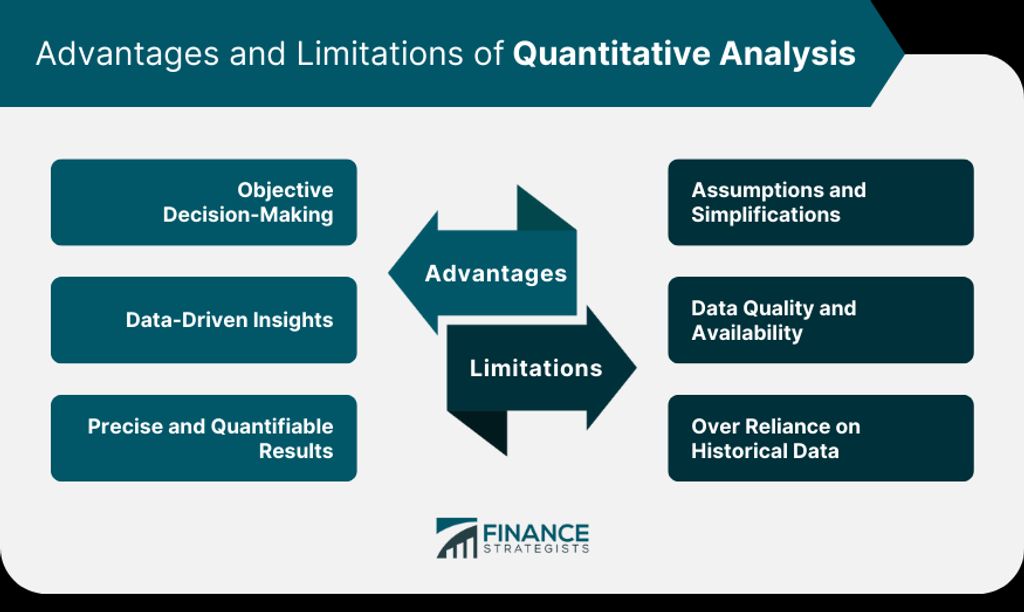
Aligning Predictive Insights with Business Goals
To harness the full potential of predictive analytics, businesses must ensure that their predictive insights are in harmony with their overarching business goals. This alignment is crucial for translating data-driven predictions into actionable strategies that drive growth and competitive advantage.
- Identify business objectives and goals.
- Assess the current data landscape to understand capabilities and limitations.
- Identify gaps and pain points that could be addressed with predictive insights.
- Develop a data strategy that incorporates predictive analytics.
- Implement predictive insights in a way that supports strategic business objectives.
Tip: Start with small, manageable projects and scale up. Gradual improvements can lead to significant gains over time. Ensure your team is well-versed in using predictive tools and interpreting results through ongoing training and development.
Predictive models are not static; they require regular review and adjustments to remain relevant. Encourage a culture that values data-driven insights and integrates them into strategic decision-making. Always be mindful of privacy and ethical considerations when leveraging predictive analytics.
Continuous Training and Development
Continuous training and development are crucial for ensuring that the workforce is equipped with the necessary skills to leverage predictive insights effectively. This involves ongoing training programs that cover the latest tools, techniques, and methodologies in predictive analytics. Additionally, fostering a culture of continuous learning and development within the organization can lead to greater adoption and application of predictive insights across various business functions. It's essential to measure the impact of training initiatives and adjust them as needed to ensure maximum effectiveness.
Review and Adjustment of Predictive Models
The dynamic nature of business and market conditions necessitates the regular review and adjustment of predictive models to maintain their relevance and accuracy. As new data becomes available, it's crucial to integrate it into existing models to reflect the latest trends and insights. This ongoing process ensures that the predictive analytics continue to provide actionable results that are both timely and reliable.
Regular updates and monitoring are not just about maintaining accuracy; they also allow for the agility required in fast-paced environments. For example, a model predicting customer churn needs to deliver rapid predictions to be effective, even if that means accepting a slight compromise on precision for the benefit of speed.
Always be mindful of privacy laws and ethical considerations when using customer data. Transparent and responsible data practices build trust and protect your business.
It's important to keep track of the impact predictive analytics has on your business. This not only justifies the investment but also informs future enhancements. Below is a simplified checklist for reviewing predictive models:
- Ensure models are aligned with current business goals
- Update models with fresh data regularly
- Balance accuracy with the need for quick insights
- Monitor the business impact of predictive analytics
- Stay compliant with data privacy and ethical standards
Business Transformation with Predictive Analytics
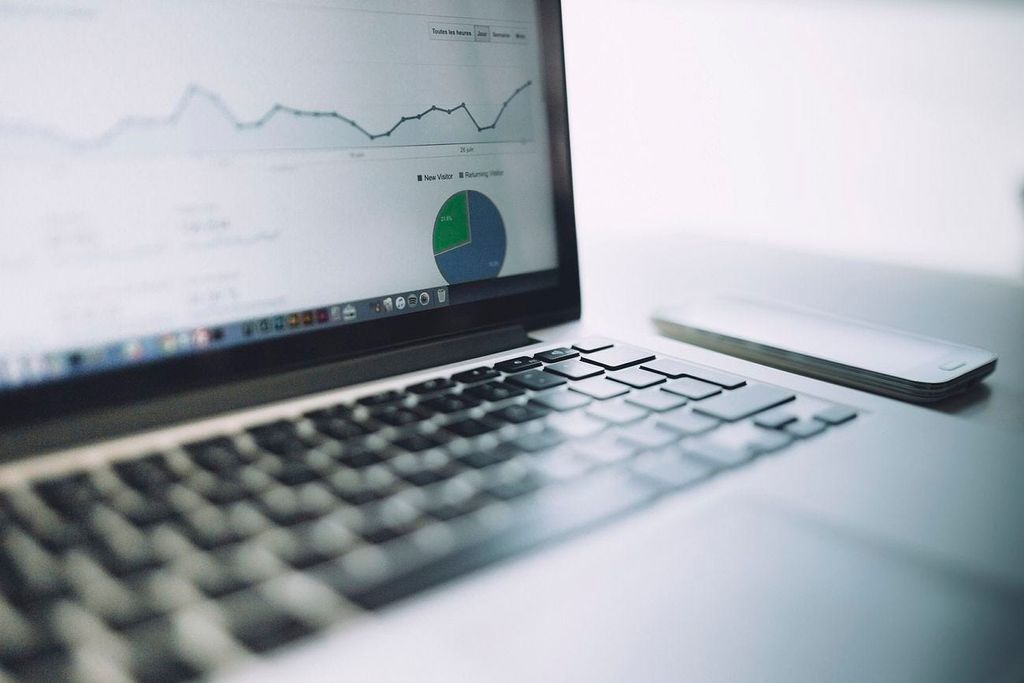
Proactive Decision-Making with Predictive Insights
Predictive analytics is an evolutionary step in how companies plan for the future, with the primary benefit being the ability to anticipate market trends and customer needs with a higher degree of accuracy; by utilizing advanced algorithms and machine learning techniques, predictive analytics has the power to parse through vast quantities of data, identify patterns that would be inscrutable to human analysts, and in a manner that is both efficient and data-driven, predict likely future outcomes, which can be a deciding factor in gaining a competitive edge. Further to this, predictive analytics can be a boon for businesses when it comes to resource allocation; by forecasting demands and potential market shifts with enhanced precision, companies can streamline their operations, optimize supply chains, and ensure that capital is not tied up in surplus inventory—an approach that can significantly reduce operational costs and mitigate the risks associated with overproduction or understocking, thereby safeguarding the financial health and stability of the business overnight. Start with small, manageable projects. Gradual improvements lead to significant gains over time. Ensure your team understands how to use predictive analytics tools and interpret the results. Ongoing training and development are essential. Predictive insights should be a core part of your strategic decisions. Encourage a culture where data-driven insights are valued and acted upon. Predictive models aren't set-and-forget. Regularly review and adjust your models to stay relevant and accurate as your business and the environment evolve. Always be mindful of privacy.
Optimizing Operations and Customer Experiences
In the realm of predictive analytics, the optimization of operations and customer experiences stands as a pivotal goal. By leveraging data-driven insights, businesses can streamline operations, reduce costs, and deliver products and services that not only meet but exceed customer expectations. This proactive approach to business management can lead to significant improvements in efficiency and customer satisfaction.
Predictive analytics plays a crucial role in tailoring customer experiences. It enables companies to anticipate needs and preferences, thus fostering a more personalized interaction. For instance, in the retail industry, predictive models can forecast trends, manage inventory levels, and suggest the right products to the right customers at the right time.
Tip: Always align predictive analytics initiatives with specific business objectives to ensure that the insights generated lead to actionable and impactful outcomes.
Here's a look at how predictive analytics can transform various business aspects:
- Forecasting Demand: Predicting future sales to manage inventory more effectively.
- Personalization: Customizing marketing and sales efforts to individual customer profiles.
- Operational Efficiency: Identifying bottlenecks and improving process flows.
- Customer Retention: Anticipating and addressing factors that lead to customer churn.
By integrating predictive analytics into the core of business operations, organizations can not only react to market changes but also proactively shape their future, ensuring a competitive edge in today's fast-paced business environment.
Risk Management and Forecasting
Predictive analytics plays a pivotal role in risk management by enabling businesses to forecast and mitigate potential risks before they materialize. Financial institutions leverage these insights to evaluate the creditworthiness of borrowers, thus reducing the likelihood of defaults. Similarly, by detecting patterns indicative of fraudulent activities, companies can proactively address such threats.
In the realm of supply chain management, predictive analytics aids in optimizing inventory levels, balancing the fine line between overproduction and understocking. This not only protects the financial well-being of a company but also ensures customer satisfaction by preventing stockouts.
Tip: Always consider the dynamic nature of risk factors and continuously update predictive models to reflect current trends and data.
For customer relationship management, predictive analytics is a game-changer. It allows businesses to analyze historical purchasing data and customer behavior, leading to personalized experiences and marketing strategies that resonate with consumer expectations. The result is a stronger customer base, characterized by heightened satisfaction and loyalty.
Predictive Analytics for Sales and Marketing
Forecasting Sales and Market Trends
Predictive analytics plays a pivotal role in forecasting sales and market trends, enabling businesses to anticipate future demands and adjust their strategies accordingly. By analyzing historical sales data, seasonal patterns, and market dynamics, companies can make informed decisions to optimize inventory, set competitive prices, and tailor marketing efforts.
- Historical Sales Data: Understanding past performance to predict future sales.
- Market Dynamics: Analyzing external factors that influence demand.
- Seasonal Patterns: Adjusting for predictable fluctuations throughout the year.
Tip: Always consider the broader economic context when forecasting, as it can significantly impact consumer behavior and market trends.
The ability to forecast effectively can lead to numerous benefits, such as improved inventory management, avoiding overstocking or stockouts, and setting prices that maximize profits and market share. Small businesses, in particular, can leverage predictive analytics to compete more effectively by focusing on the most promising products and customer segments.
Personalization of Marketing Efforts
In the realm of marketing, predictive analytics has become a cornerstone for crafting personalized customer experiences. By analyzing historical data and browsing behavior, businesses can predict which products or services a customer is likely to be interested in. This enables the delivery of personalized recommendations, a strategy that has proven its worth; a significant percentage of consumers have expressed a preference for retailers who offer personalized experiences, with many becoming repeat buyers.
The implementation of personalized marketing efforts can be broken down into several key areas:
- Optimizing the marketing mix: Determining the most effective combination of marketing channels to enhance ROI.
- Lead scoring: Prioritizing prospects based on their predicted likelihood to convert.
- Enhanced customer experience: Tailoring interactions to individual preferences to boost satisfaction and reduce churn.
Tip: Always ensure that personalization efforts are subtle and respect customer privacy to maintain trust and compliance with data protection regulations.
The impact of personalization is not just felt in customer engagement and loyalty; it also translates into tangible business results. For instance, a health and wellness app focusing on personalized diet plans saw a substantial increase in premium subscribers and average revenue per user, simply by leveraging predictive analytics to fine-tune their marketing approach.
Customer Behavior Analysis
Predictive analytics is a powerful tool to increase customer engagement, loyalty, and bottom line. It's selling savvy. Every customer leaves a digital footprint through their interactions with your business. Predictive analytics decode these digital footprints, using advanced AI algorithms and machine learning to identify patterns and predict future behavior. Customer behavior prediction means getting a sneak peek into future customer actions. For instance, by analyzing past purchase history and browsing habits, predictive analytics models predict buying behaviors or identify which customers might be at risk of churning. This allows you to tailor your marketing efforts or customer service strategies to keep your customers engaged and loyal.
Predictive customer analytics FAQ
- How can predictive analytics deepen customer relationships?
Predictive analytics help you better understand your customers’ behaviors and needs, now and in the future. With this understanding, you can:- Provide personalized marketing
- Anticipate the needs of your customers
- Improve your customers’ experience
- Provide better customer support
All these factors contribute to a deeper relationship with your customers.
Predictive Analytics for Customer Insights and Sales Strategies
Predictive customer analytics can be used as part of your business strategy.
- Look-alike modeling: Look-alike marketing targets potential new customers who resemble existing customers. Predictive analytics evaluates the characteristics and behaviors of current customers to accurately identify these “look-alike” customers.
- Customer segmentation: Predictive analytics examines data to identify distinct customer groups with shared characteristics such as demographics, behavior, and preferences. It then forecasts the specific needs of each segment, enabling businesses to provide more personalized experiences.
Predictive Analytics Business Case Studies
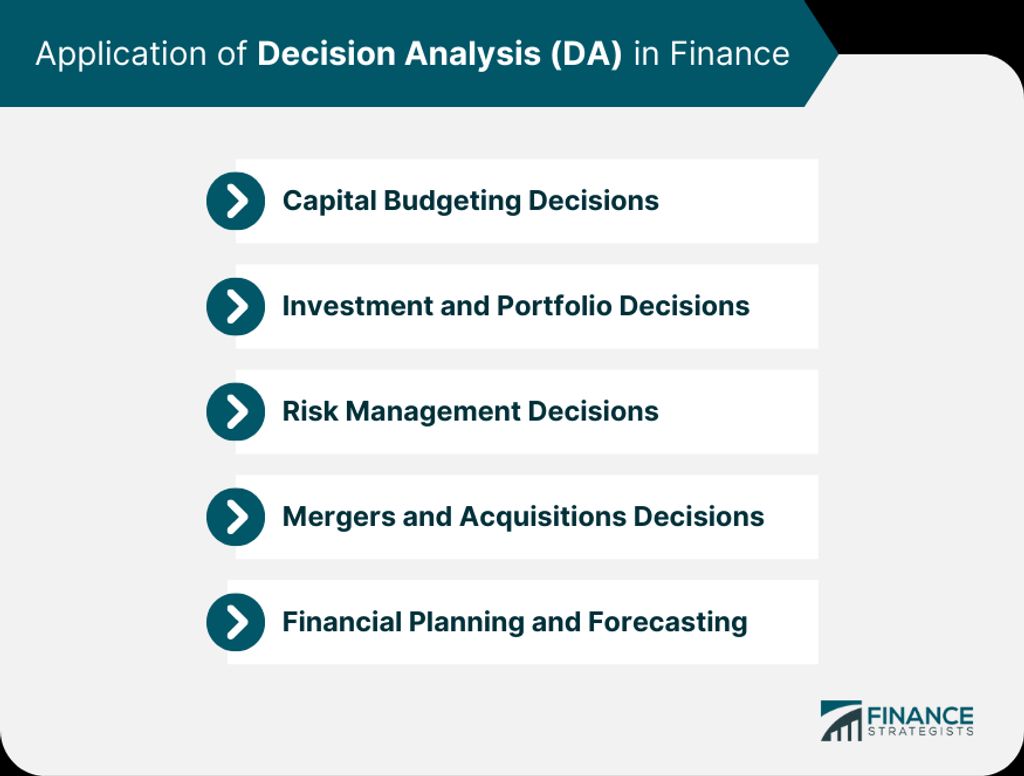
Health and Wellness App Case Study
In the competitive health and wellness industry, leveraging predictive analytics can be a game-changer. A case study of a wellness app illustrates this point effectively. Initially, the app struggled with user retention and monetization. By employing predictive analytics, the team could identify key features that drove user engagement and subscription upgrades.
For instance, a deep dive into user behavior data revealed that personalized diet plans were instrumental in converting free users to premium subscribers. The app's focus on enhancing this feature and tailoring marketing efforts led to a significant uptick in user retention and revenue. Here's a snapshot of the impact:
- User retention rates doubled in the first two weeks
- A 50% increase in new sign-ups over the next quarter
- Premium subscribers grew from 5% to 12% of the user base
- Monthly revenue doubled to $40,000 in six months
Tip: Always look for patterns in user engagement and monetization data. These insights can guide feature development and marketing strategies for better ROI.
This case study underscores the importance of data-driven decision-making. By focusing on the analytics, the app not only improved its financial performance but also provided more value to its users, fostering a stronger community around the product.
Success Factors in Predictive Analytics
Success factors may include having a clear strategy for how to use analytics, the quality and relevance of the data collected, choosing the appropriate algorithms and models, and having the right mix of skills and technology to interpret and act on the predictions generated. To truly maximize the potential of predictive analytics, organizations must emphasize the accuracy and quality of the data they collect; it is the bedrock upon which all predictive models are built. Models based on incomplete or poor-quality data will inevitably lead to skewed results and unreliable forecasts that could misguide rather than inform strategic decisions. Organizations should focus on collecting relevant data, building predictive models using statistical and machine learning methods, and integrating these models into their existing decision-making processes for enhanced forecasting.
Hypothetical Business Case Scenario
In this hypothetical business case scenario, we explore the transformative power of predictive analytics in a mid-sized e-commerce company. The company, facing stiff competition and a plateau in growth, decides to leverage predictive analytics to gain a competitive edge.
The first step involves a thorough analysis of historical sales data, customer behavior, and market trends. By applying advanced analytics, the company identifies key areas for improvement and potential market opportunities. The insights gained enable the company to optimize its inventory, personalize marketing campaigns, and improve customer retention strategies.
To illustrate the impact of these changes, consider the following outcomes within the first year of implementation:
- Increased customer lifetime value by 20%
- Reduction in overstock inventory by 30%
- Boost in conversion rates by 15%
Tip: Always align predictive analytics initiatives with specific business objectives to ensure measurable outcomes and a clear path to ROI.
The case study underscores the importance of not only collecting and analyzing data but also acting on the insights to drive tangible business results. It serves as a compelling example of how predictive analytics can be a game-changer for businesses willing to embrace data-driven decision-making.
Conclusion
In conclusion, predictive analytics is a powerful tool for businesses to forecast future trends, customer behaviors, and market dynamics. By leveraging data, statistical algorithms, and machine learning, businesses can make smarter decisions and optimize operations. While predictive models are not foolproof, they offer valuable insights that can transform business forecasting and strategic planning. It is essential for businesses to embrace a culture of data-driven insights and continuous improvement to stay ahead in today's competitive landscape.
Frequently Asked Questions
How can predictive analytics be used in business?
Predictive analytics can be used in business to forecast future trends, customer behaviors, and market dynamics, enabling proactive decision-making and strategic planning. It also helps optimize operations, personalize customer experiences, enhance marketing efforts, and improve predictive analytics for risk management by providing data-driven insights and predictions.
What are the benefits of predictive analytics in business?
The benefits of predictive analytics in business include better decision-making, efficient use of resources, improved inventory management, creating better products, managing risks, gaining more customers, and tailoring products or services based on customer preferences.
How does predictive analytics transform business forecasting?
Predictive analytics transforms business forecasting by providing deeper insights into future market conditions and customer behavior, enabling businesses to be proactive and strategic rather than reactive. It helps businesses anticipate customer needs, manage risks, and tailor marketing campaigns for better outcomes.
What are the success factors for implementing predictive analytics in business?
Success factors for implementing predictive analytics in business include having a clear strategy for using analytics, collecting high-quality and relevant data, choosing appropriate algorithms and models, and having the right mix of skills and technology to interpret and act on the predictions generated.
What are the core concepts of predictive analytics?
Predictive analytics uses data, statistical algorithms, AI, and machine learning techniques to identify the likelihood of future outcomes based on historical data. It helps businesses anticipate customer needs, manage risks, and tailor marketing campaigns for better outcomes.
What are the challenges of integrating predictive analytics into business operations?
Challenges of integrating predictive analytics into business operations include ensuring the accuracy and quality of data, choosing the right predictive analytics tools, and integrating these models into the business's existing systems to ensure seamless operation.
What are the best practices for seamless integration of predictive analytics?
Best practices for seamless integration of predictive analytics include having a clear strategy for how to use analytics, collecting high-quality and relevant data, choosing appropriate algorithms and models, and having the right mix of skills and technology to interpret and act on the predictions generated.
What are the data privacy and security considerations for predictive analytics?
Data privacy and security considerations for predictive analytics involve ensuring the privacy and security of collected data, complying with data protection regulations, and implementing secure data management and storage practices to protect sensitive information.