How to Use Predictive Analytics to Drive Business Growth
Overview
What is predictive analytics?
Predictive analytics is a branch of advanced analytics that uses historical data, statistical algorithms, and machine learning techniques to predict future outcomes. It involves analyzing large datasets to identify patterns, trends, and relationships that can be used to make data-driven predictions. By leveraging predictive analytics, businesses can gain valuable insights into customer behavior, market trends, and database performance. These insights can then be used to make informed decisions, optimize business processes, and drive business growth.
Benefits of using predictive analytics
Predictive analytics offers numerous benefits for businesses. By analyzing historical data and using statistical algorithms, businesses can gain valuable insights into future trends and patterns. This allows them to make informed decisions and take proactive measures to drive business growth. Some of the key benefits of using predictive analytics include:
- Improved forecasting accuracy
- Enhanced customer segmentation
- Optimized marketing campaigns
- Reduced risk and fraud
In addition, predictive analytics can also help businesses stay ahead of the competition by identifying emerging trends and opportunities. By leveraging predictive insights, businesses can gain a competitive edge and stay relevant in today's dynamic market. With the constant advancements in technology and the increasing availability of data, predictive analytics is becoming an essential tool for businesses to stay ahead and drive growth.
Key components of predictive analytics
Predictive analytics involves several key components that are essential for its successful implementation. These components include data collection and preparation, model development, and application and business impact. Each component plays a crucial role in harnessing the power of predictive analytics to drive business growth. Data collection and preparation involves identifying relevant data sources, cleaning and organizing the data, and performing feature engineering to extract meaningful insights. Model development focuses on choosing the right predictive model, training and testing the model, and evaluating its performance. Finally, application and business impact involve implementing predictive analytics in business processes, leveraging predictive insights for decision-making, and measuring the impact of predictive analytics on business growth. By maintaining a strong focus on these key components, businesses can unlock the full potential of predictive analytics and achieve significant growth.
Data Collection and Preparation
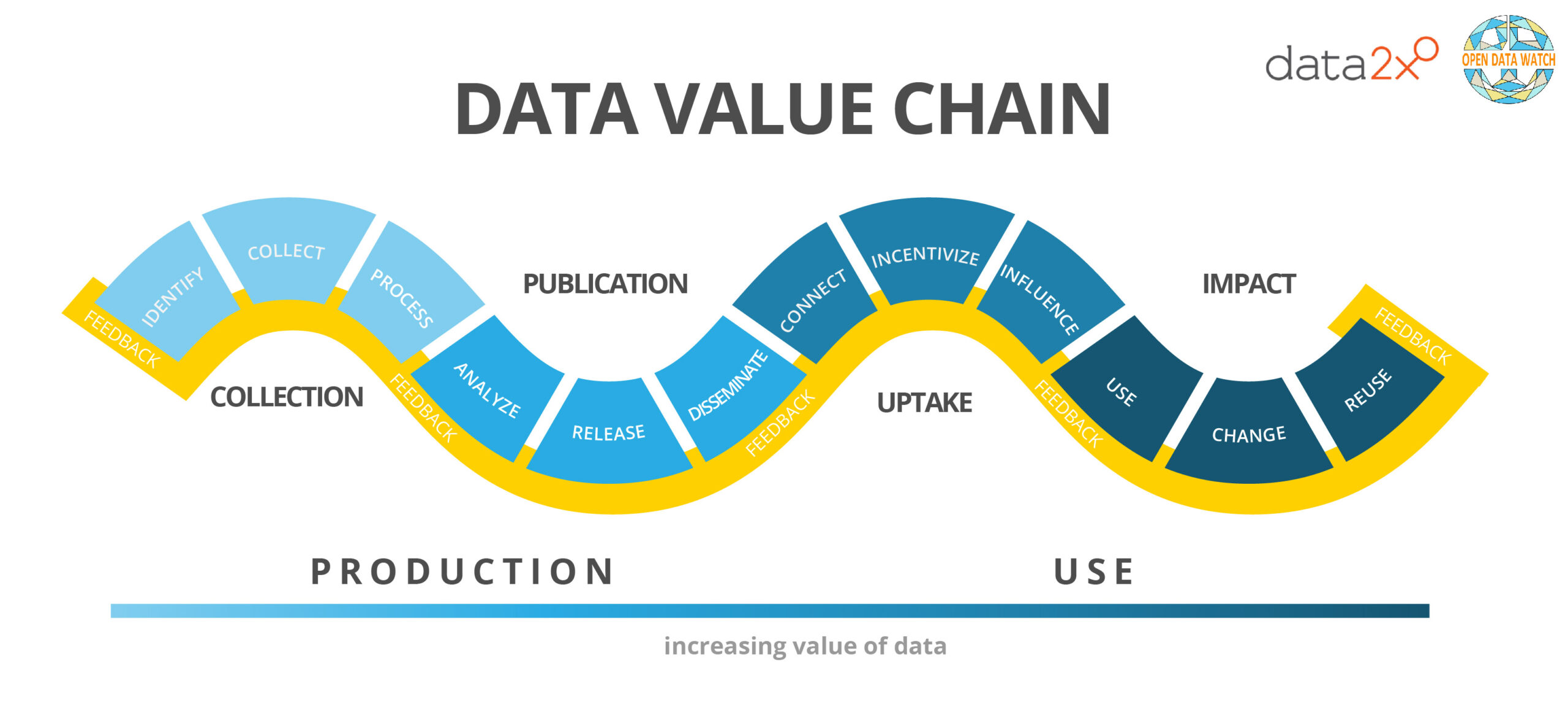
Identifying relevant data sources
Once you have a clear understanding of what predictive analytics is and the benefits it can bring to your business, the next step is to identify the relevant data sources that will be used to build your predictive models. The success of your predictive analytics initiatives heavily relies on the quality and relevance of the data you collect. It is important to involve your Data Officer or data management team in this process to ensure that you have access to the right data. Start by identifying internal data sources such as customer databases, transaction records, and operational data. Additionally, consider external data sources such as social media data, market research reports, and industry benchmarks. By leveraging a diverse range of data sources, you can gain comprehensive insights and make more accurate predictions.
Cleaning and organizing data
Once the relevant data sources have been identified, the next step in the predictive analytics process is cleaning and organizing data. This involves removing any duplicate or irrelevant data, as well as handling missing values. Additionally, the data needs to be organized in a format that is suitable for analysis. Feature engineering techniques may also be applied to transform the data and create new variables that can improve the predictive power of the model. By ensuring that the data is clean and well-organized, businesses can unlock the data potential and extract valuable insights that can drive business growth.
Feature engineering
Feature engineering is a crucial step in the predictive analytics process. It involves transforming raw data into meaningful features that can be used by the predictive model. This process requires domain knowledge and creativity to identify and create new features that capture the relevant information for the prediction task. Feature engineering techniques include scaling, encoding, imputation, and dimensionality reduction. By engineering the right features, businesses can improve the performance of their predictive models and make more accurate predictions.
Model Development
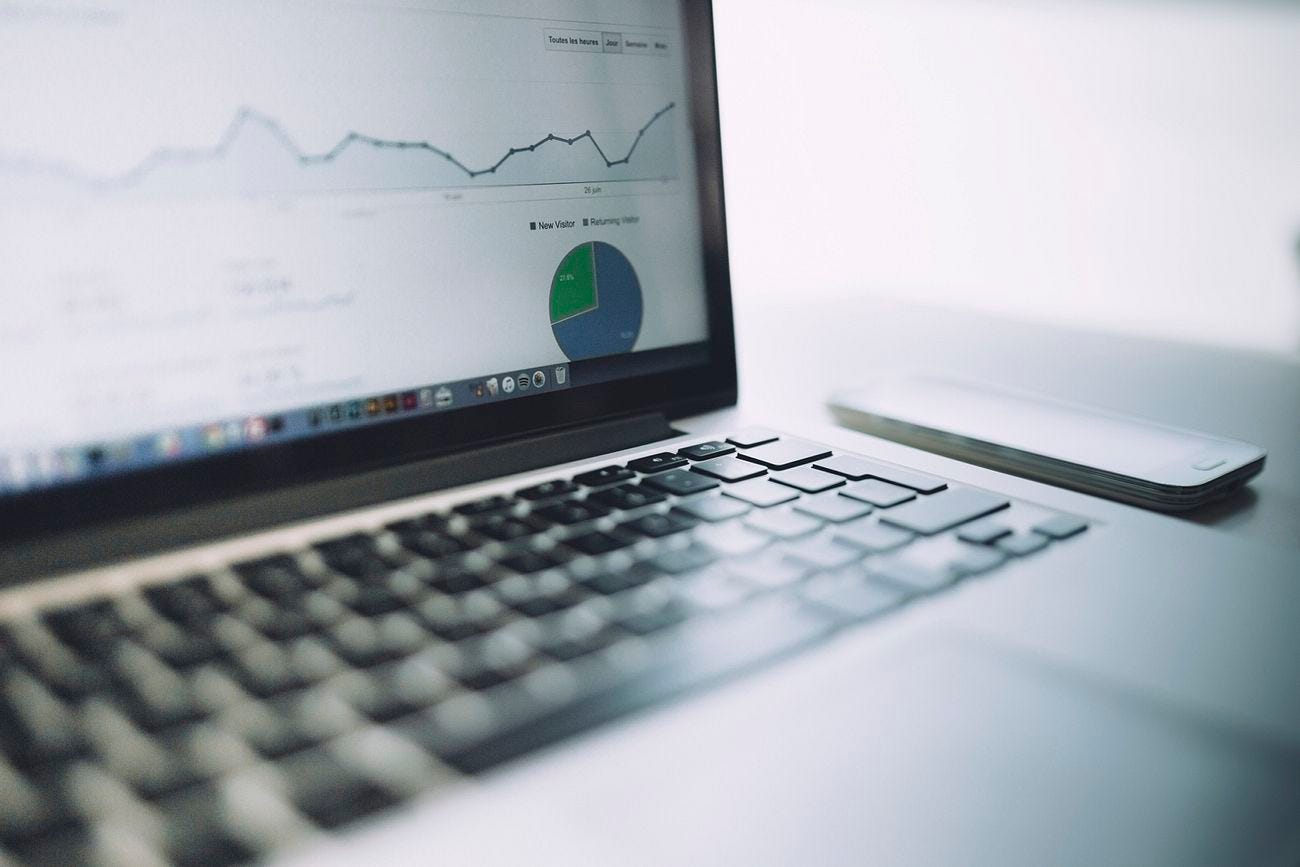
Choosing the right predictive model
Once the relevant data has been collected and prepared, the next step is choosing the right predictive model. This is a crucial decision as the model will determine the accuracy and effectiveness of the predictions. There are various types of predictive models available, such as linear regression, decision trees, and neural networks. The choice of model depends on the nature of the problem and the data available. It is important to consider factors like model complexity, interpretability, and computational requirements. Additionally, database optimization plays a significant role in model selection, as it ensures efficient data retrieval and processing. Evaluating different models through testing and validation is essential to identify the most suitable one for the specific business problem.
Training and testing the model
Once the predictive model has been chosen, the next step is to train and test it. Training involves feeding the model with labeled data and allowing it to learn the patterns and relationships within the data. This process helps the model to make accurate predictions when presented with new, unseen data. Testing, on the other hand, involves evaluating the performance of the trained model using a separate set of data. This allows us to assess how well the model generalizes to new data and identify any potential issues or areas for improvement. During the training and testing phase, it is important to ensure that the data used is clean and reliable. SQL can be used to query and manipulate the data, ensuring its quality and integrity.
Evaluating model performance
Evaluating the performance of predictive models is essential to ensure their accuracy and effectiveness. Machine learning algorithms are commonly used to train and test these models, allowing businesses to make data-driven decisions. Various evaluation metrics, such as accuracy, precision, recall, and F1 score, can be used to assess the model's performance. Additionally, techniques like cross-validation and confusion matrices can provide deeper insights into the model's strengths and weaknesses. By thoroughly evaluating the model's performance, businesses can gain confidence in its predictions and make informed decisions to drive business growth.
Application and Business Impact
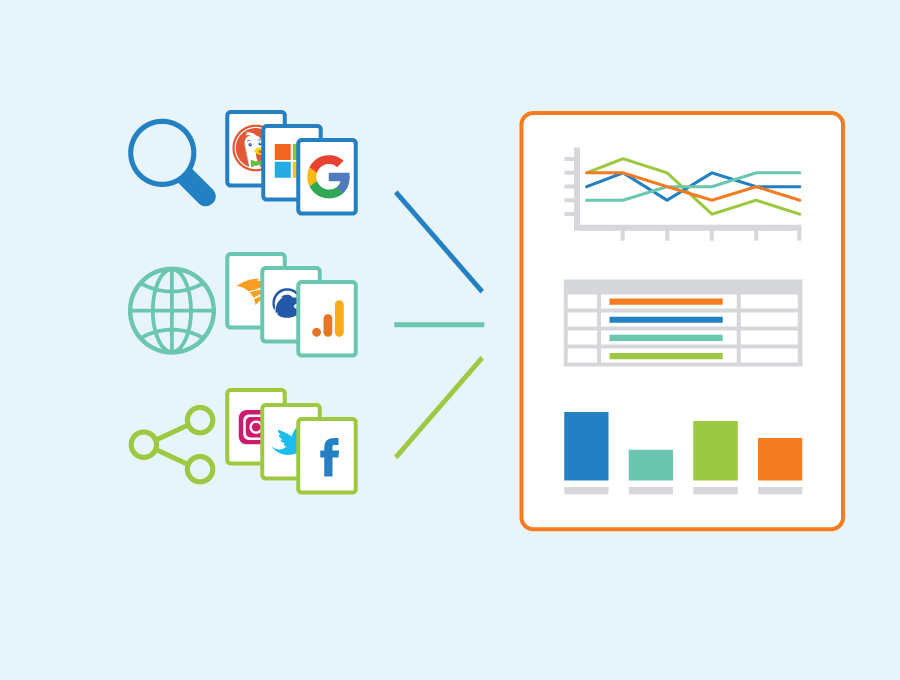
Implementing predictive analytics in business processes
Once the predictive analytics model has been developed and tested, it can be implemented in various business processes to drive business growth. One key aspect of implementing predictive analytics is ensuring that the necessary infrastructure is in place. This includes having a robust data storage and processing system, such as SQL Server, to handle the large volumes of data required for predictive analytics. SQL Server provides powerful capabilities for data management and analysis, allowing businesses to efficiently store, retrieve, and analyze data for predictive modeling. By leveraging the capabilities of SQL Server, businesses can effectively integrate predictive analytics into their existing workflows and decision-making processes.
Leveraging predictive insights for decision-making
Predictive analytics is a powerful tool that can provide valuable insights to inform decision-making processes. By analyzing historical data and identifying patterns, businesses can gain a deeper understanding of their customers' preferences and behaviors. This understanding can then be used to make more informed decisions about product development, marketing strategies, and customer engagement. Additionally, predictive analytics can help businesses identify potential risks and opportunities, allowing them to proactively address issues and capitalize on trends. By leveraging predictive insights, businesses can make data-driven decisions that drive growth and success.
Measuring the impact of predictive analytics on business growth
Measuring the impact of predictive analytics on business growth is crucial for businesses to understand the effectiveness of their analytics initiatives. One way to measure this impact is through database management. By effectively managing and analyzing large datasets, businesses can gain valuable insights that can drive decision-making and improve overall business performance. Additionally, measuring the impact of predictive analytics on business growth can also involve tracking key performance indicators (KPIs) such as revenue growth, customer acquisition, and customer retention rates. By monitoring these metrics, businesses can assess the success of their predictive analytics efforts and make data-driven decisions to further optimize their strategies.
Conclusion

Summary of the benefits of using predictive analytics
Predictive analytics offers several benefits for businesses looking to drive growth. By leveraging historical data and advanced algorithms, businesses can gain valuable insights and make informed decisions. Focused on Short-term Wins, predictive analytics helps businesses identify immediate opportunities and optimize their operations. Additionally, predictive analytics enables businesses to anticipate customer behavior, improve marketing strategies, and enhance overall customer experience. By harnessing the power of predictive analytics, businesses can stay ahead of the competition and achieve sustainable growth.
Future trends in predictive analytics
As predictive analytics continues to evolve, there are several emerging trends that are shaping the future of this field. One of these trends is database tuning, which involves optimizing the performance of databases to improve the speed and efficiency of data retrieval and analysis. By fine-tuning the database infrastructure, businesses can ensure that predictive analytics models have access to high-quality data in a timely manner. Another trend is the increased use of machine learning algorithms in predictive analytics. These algorithms can automatically learn from data and make predictions without being explicitly programmed, allowing businesses to uncover valuable insights and patterns in their data. Additionally, there is a growing focus on real-time predictive analytics, where models are trained and updated continuously to provide up-to-date predictions. This enables businesses to make informed decisions in real-time and respond quickly to changing market conditions. Overall, the future of predictive analytics looks promising, with advancements in database tuning, machine learning algorithms, and real-time analytics driving business growth and innovation.
Call to action for businesses to adopt predictive analytics
To fully capitalize on the potential of predictive analytics, businesses need to embrace its power and incorporate it into their operations. Database Optimization is one of the key areas where predictive analytics can make a significant impact. By leveraging predictive models, businesses can identify and eliminate bottlenecks in their data storage and retrieval processes, resulting in improved efficiency and cost savings. With optimized databases, businesses can access the right information at the right time, enabling faster and more accurate decision-making. By adopting predictive analytics, businesses can gain a competitive edge and drive sustainable growth.
In conclusion, OptimizDBA Database Optimization Consulting is the trusted industry leader in remote DBA services since 2001. With over 500 clients, we guarantee a significant increase in performance, experiencing transaction speeds that are at least twice as fast as before. Our average speeds are often 100 times, 1000 times, or even higher! If you're looking to optimize your database and improve its performance, look no further than OptimizDBA. Visit our website to learn more about our services and how we can help you achieve faster transaction speeds.