Key Trends and Technologies in Analytics for Businesses
Emerging Trends in Analytics
Artificial Intelligence and Machine Learning
Artificial Intelligence (AI) and Machine Learning (ML) are revolutionizing the field of analytics. AI refers to the simulation of human intelligence in machines that are programmed to think and learn like humans. ML, on the other hand, is a subset of AI that focuses on the development of algorithms and models that allow machines to learn from and make predictions or decisions based on data. These technologies are enabling businesses to analyze large amounts of data and uncover valuable insights. They are also improving the accuracy and efficiency of tasks such as data processing, pattern recognition, and decision-making. With AI and ML, businesses can automate processes, personalize customer experiences, and optimize operations. However, implementing AI and ML requires database management and data integration to ensure the availability and quality of data for analysis.
Big Data and Data Visualization
Big data refers to the massive amount of structured and unstructured data that is generated by businesses. It includes data from various sources such as social media, sensors, and online transactions. Data visualization is the process of presenting this data in a visual format, such as charts, graphs, and maps, to help businesses gain insights and make informed decisions. Big data and data visualization are essential components of modern analytics, enabling businesses to identify patterns, trends, and correlations in large datasets. By visualizing data, businesses can easily understand complex information and communicate it effectively to stakeholders. Data visualization tools, such as Tableau and Power BI, provide interactive and intuitive ways to explore and analyze data. However, the challenge lies in managing and analyzing the vast amount of data to extract meaningful insights. Organizations need to invest in advanced analytics techniques, such as machine learning and predictive analytics, to unlock the full potential of big data and data visualization.
Predictive Analytics
Predictive analytics is a powerful tool that enables businesses to make data-driven decisions and anticipate future outcomes. By analyzing historical data and applying statistical models, organizations can identify patterns and trends to forecast future events. This helps in optimizing business operations, reducing risks, and improving overall efficiency. However, the successful implementation of predictive analytics requires a well-structured data organization and advanced analytics capabilities. It is crucial for businesses to have a clear understanding of their data sources, data quality, and data governance to ensure accurate predictions.
Technologies in Analytics
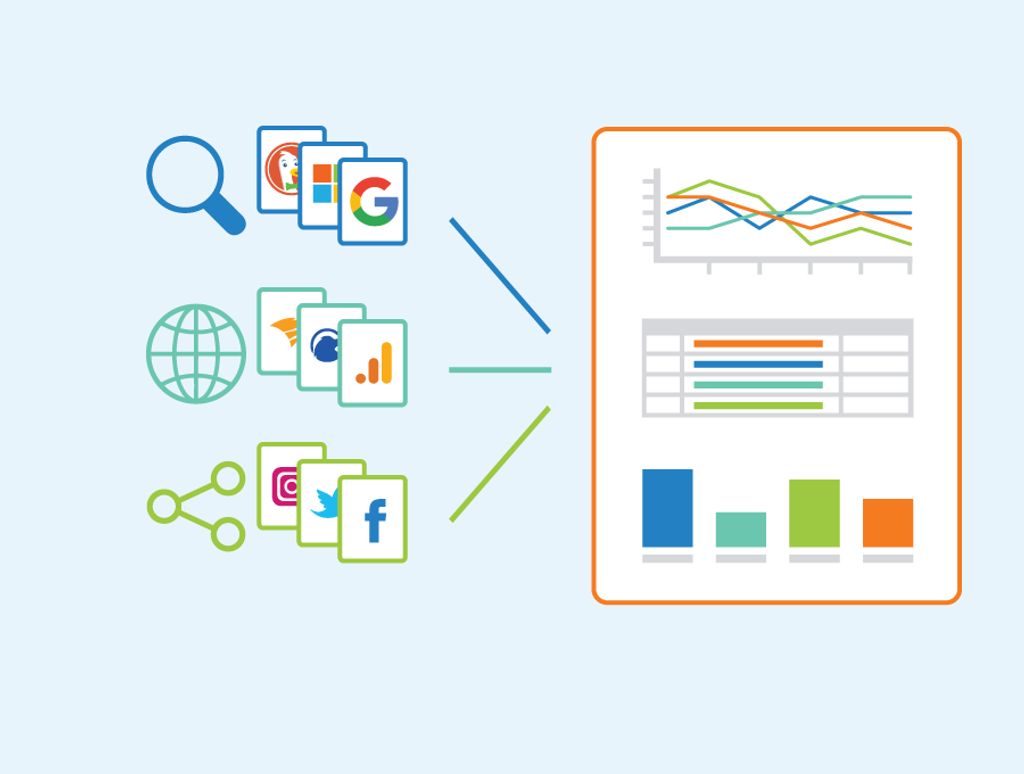
Cloud Computing
Cloud computing is a key technology in analytics that enables businesses to store and access large amounts of data efficiently and cost-effectively. With cloud computing, businesses can leverage the power of remote servers to perform complex database analysis and generate valuable insights. Additionally, cloud computing provides scalability and flexibility, allowing businesses to easily scale their analytics infrastructure as their needs grow. However, it is important for businesses to address potential data privacy and security concerns when adopting cloud computing solutions.
Internet of Things (IoT)
The Internet of Things (IoT) is a rapidly growing technology that connects various devices and objects to the internet, enabling them to collect and exchange data. This connectivity allows businesses to gather valuable insights and make data-driven decisions. IoT has the potential to revolutionize the way businesses operate by providing real-time data and improving operational efficiency. However, with the increasing number of connected devices, data security becomes a major concern. It is essential for businesses to ensure the protection of sensitive data and implement robust security measures. Additionally, the analytics impact of IoT is significant, as it enables organizations to analyze large volumes of data generated by connected devices and extract valuable insights. By leveraging IoT analytics, businesses can enhance their decision-making processes and gain a competitive edge.
Natural Language Processing (NLP)
Natural Language Processing (NLP) is a branch of artificial intelligence that focuses on the interaction between computers and humans through natural language. It enables computers to understand, interpret, and generate human language, allowing for more seamless communication and interaction. NLP has become increasingly important in the field of analytics as it allows businesses to extract valuable insights from unstructured data such as customer reviews, social media posts, and emails. With the help of NLP, businesses can analyze large volumes of text data to identify patterns, sentiments, and trends. This technology plays a crucial role in data-driven transformations, empowering businesses to make informed decisions and gain a competitive edge in the market.
Here is a table highlighting the key benefits of NLP:
Benefits of NLP |
---|
Improved customer service through chatbots |
Enhanced sentiment analysis for customer feedback |
Efficient text summarization for large documents |
In addition, NLP can be used to create personalized recommendations for customers based on their preferences and past interactions. By leveraging NLP technology, businesses can automate various tasks, streamline processes, and improve overall efficiency. As the amount of text data continues to grow exponentially, the demand for NLP in analytics is expected to increase.
Challenges in Analytics Implementation
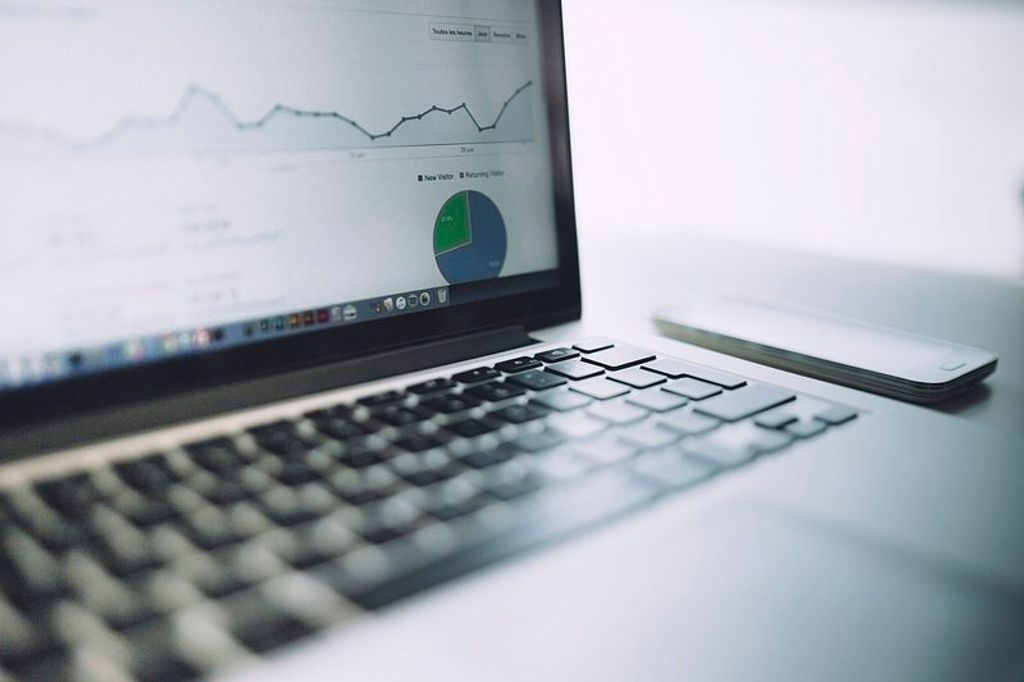
Data Privacy and Security
Data privacy and security are major concerns in the field of analytics. With the increasing amount of data being collected and analyzed, businesses need to ensure that they have robust security measures in place to protect sensitive information. This includes implementing encryption techniques, access controls, and data anonymization. Additionally, organizations must comply with data protection regulations to avoid legal and reputational risks. It is crucial for businesses to prioritize data privacy and security in order to build trust with their customers and stakeholders.
Data Quality and Integration
Data quality and integration are crucial aspects in analytics implementation. Ensuring accurate and reliable data is essential for generating meaningful insights and making informed business decisions. Organizations need to establish robust data governance processes and implement effective data cleansing techniques to address data quality issues. Additionally, integrating data from various sources and systems requires seamless data integration solutions to ensure data consistency and completeness. Data quality and integration play a vital role in enabling data-driven innovation and driving business success.
Skills and Talent Gap
The skills and talent gap is one of the major challenges in implementing analytics in businesses. With the rapid advancement of technology and the increasing demand for data-driven insights, there is a shortage of professionals with the necessary skills and expertise in analytics. Organizations are struggling to find individuals who possess the technical knowledge and analytical skills required to effectively analyze and interpret data. This gap in talent hinders the successful adoption and implementation of analytics solutions. To bridge this gap, companies are investing in training programs and initiatives to develop the analytics capabilities of their workforce. However, the shortage of skilled professionals remains a significant obstacle in harnessing the full potential of analytics in business decision-making.
Challenges in Analytics Implementation