Understanding Data Analytics in Business
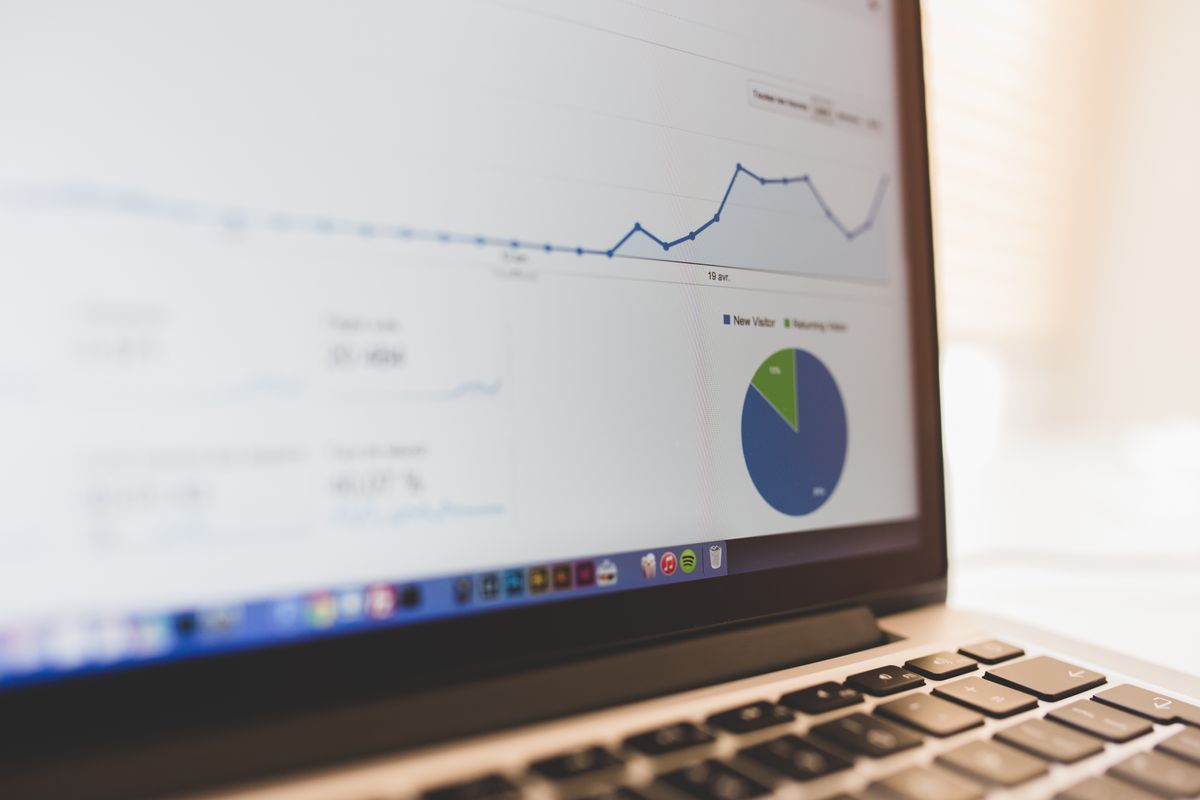
Data Visualization Techniques
In the realm of business analytics, data visualization is a powerful tool that transforms complex data sets into intuitive graphical representations, enabling stakeholders to grasp difficult concepts and identify new patterns easily. Effective visualizations can lead to quicker decision-making and better communication among team members.
- Identify the key metrics that align with business objectives
- Choose the right type of chart or graph for the data
- Use color and design elements to enhance understanding
- Ensure the visualization is accessible and interpretable by the intended audience
By focusing on clarity and simplicity, data visualizations can reveal insights that might otherwise remain hidden in spreadsheets or reports.
It's important to remember that prescriptive analytics, which suggest actions to take, require quality data integration. Collaboration between experts is key to overcoming challenges such as data privacy and security concerns. Companies should address these concerns with transparency and consider starting small with AI implementations to build trust and verify results.
Predictive Analytics Applications
The realm of predictive analytics is transforming how businesses anticipate future trends and customer behaviors. By leveraging historical data and advanced algorithms, companies can forecast outcomes with a remarkable degree of accuracy. Predictive models are now integral to strategic planning, enabling organizations to allocate resources more effectively and gain a competitive edge.
Predictive analytics applications span various business functions, from risk assessment to optimizing marketing campaigns. They empower decision-makers to act proactively rather than reactively.
To illustrate the breadth of predictive analytics tools available, consider the following list extracted from a recent TechTarget article:
- Altair AI Studio
- H2O Driverless AI
- IBM Watson Studio
- Microsoft Azure Machine Learning
- SAP ...
Each tool offers unique features that cater to different business needs, such as real-time data processing or automated model building. Selecting the right tool is crucial for harnessing the full potential of predictive analytics.
Data-driven Decision Making
In the realm of modern business, data-driven decision making stands as a pivotal practice that can significantly enhance operational efficiency and strategic planning. By systematically analyzing data, companies can uncover valuable insights that inform critical business decisions. This approach contrasts sharply with reliance on intuition or experience alone.
Data-driven decision making involves several key steps to ensure its effectiveness:
- Identifying relevant data sources
- Collecting high-quality, accurate data
- Processing and cleaning the data
- Analyzing the data to uncover trends and patterns
- Interpreting the results to make informed decisions
- Implementing the decisions and monitoring outcomes
Embracing a data-driven culture within an organization empowers leaders to make more objective, informed, and potentially profitable business choices.
The benefits of this methodology are manifold, including improved customer satisfaction, optimized operational processes, and a stronger competitive edge. By leveraging data to its fullest potential, businesses can not only react to market changes but also anticipate them, staying one step ahead in the ever-evolving commercial landscape.
Implementing Machine Learning in Business Operations
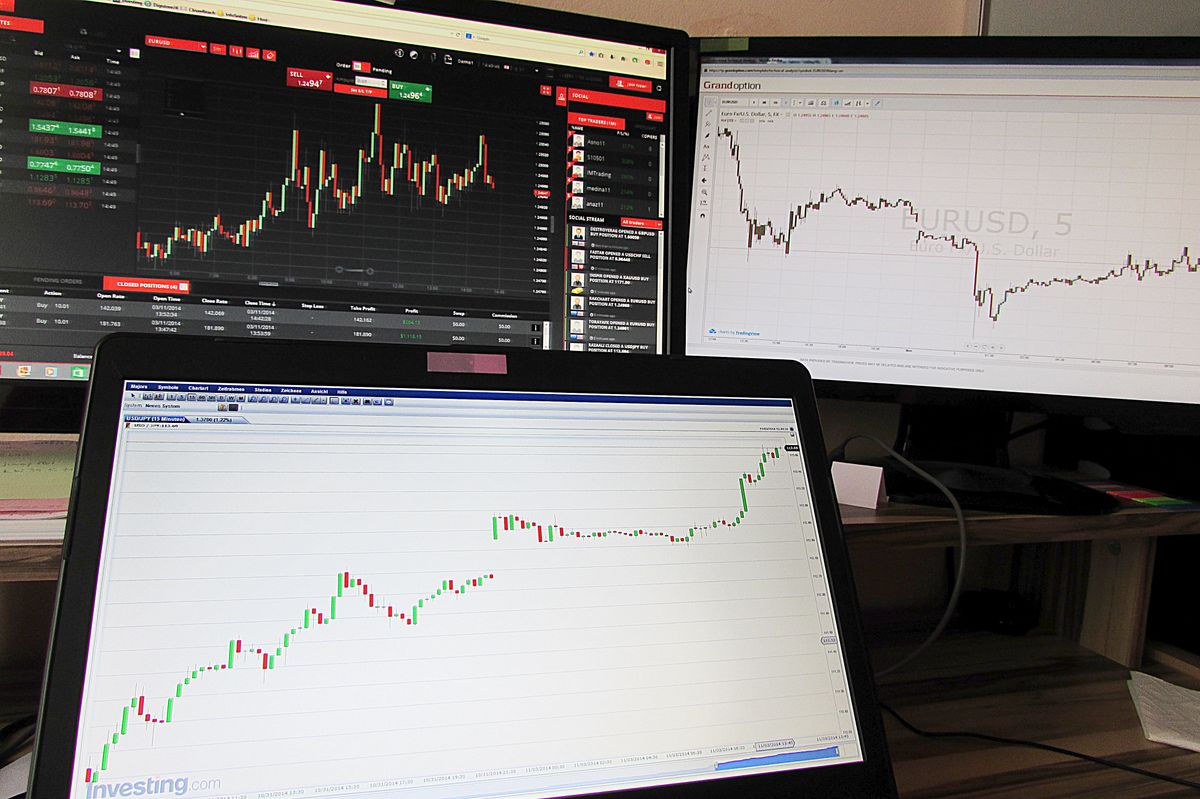
Machine Learning Models for Customer Segmentation
Customer segmentation is a pivotal strategy for businesses aiming to tailor their marketing efforts and product offerings to different customer groups. Machine learning models elevate this strategy by uncovering patterns and segments that may not be apparent through traditional analysis. One of the most powerful techniques is cluster analysis, which groups customers based on similarities in their behavior or demographics.
By leveraging machine learning for customer segmentation, companies can achieve a more nuanced understanding of their customer base, leading to more effective targeting and personalization.
Another approach is the RFM (Recency, Frequency, Monetary) model, which segments customers based on their transaction history. This model can be enhanced with machine learning to predict future purchase behaviors. Combining various segmentation models allows for a multi-dimensional view of the customer base, which is crucial for developing targeted strategies.
Here is a brief overview of some common machine learning models used in customer segmentation:
- Cluster Analysis Segmentation: Groups customers based on shared characteristics.
- RFM Segmentation: Segments based on purchase history.
- Longevity Segmentation: Focuses on the length of the customer relationship.
- Combining Segmentation Models: Integrates multiple models for a comprehensive view.
The integration of machine learning not only refines existing models but also enables the discovery of new segments, leading to innovative strategies for business growth.
Automating Business Processes with ML
The integration of Machine Learning (ML) into business operations is revolutionizing the way companies approach process automation. By leveraging ML algorithms, businesses can significantly reduce manual effort, minimize errors, and increase efficiency. Automation through ML is not just about replacing repetitive tasks but also about enhancing the capabilities of human workers, allowing them to focus on more strategic activities.
Machine learning can be applied to various business processes, including customer service, HR operations, and financial management. For instance, chatbots powered by ML can handle customer inquiries without human intervention, while ML algorithms can sift through resumes to identify the best candidates for a job.
- Streamlining customer support with intelligent chatbots
- Enhancing HR processes through automated candidate screening
- Optimizing financial operations with predictive budgeting
The strategic implementation of ML in business processes can lead to a significant competitive edge, as it enables organizations to act swiftly and adapt to market changes with unprecedented agility.
Machine Learning in Sales Forecasting
Incorporating machine learning (ML) into sales forecasting represents a transformative leap from traditional analytical methods. ML algorithms can analyze vast datasets, uncovering patterns and trends that may not be immediately apparent to human analysts. This capability allows businesses to anticipate market changes with greater accuracy.
Sales forecasting with ML involves training models on historical data, which then predict future sales based on a variety of factors, including seasonal trends, economic indicators, and consumer behavior. The predictive power of ML models means that businesses can optimize inventory levels, adjust marketing strategies, and allocate resources more efficiently.
By leveraging ML for sales forecasting, companies gain the ability to make proactive, data-driven decisions that can significantly enhance their competitive edge.
Here's an example of how ML can improve forecasting accuracy over time:
Quarter | Traditional Forecasting | ML-based Forecasting | Improvement (%) |
---|---|---|---|
Q1 | $2M | $2.1M | 5% |
Q2 | $2.5M | $2.7M | 8% |
Q3 | $3M | $3.4M | 13% |
Q4 | $4M | $4.5M | 12% |
The table illustrates the potential incremental improvements in forecasting accuracy when ML is applied. As the model is refined and more data is incorporated, the accuracy of sales predictions is expected to increase, leading to better strategic decisions and ultimately, business growth.
Optimizing Marketing Strategies with Data Science
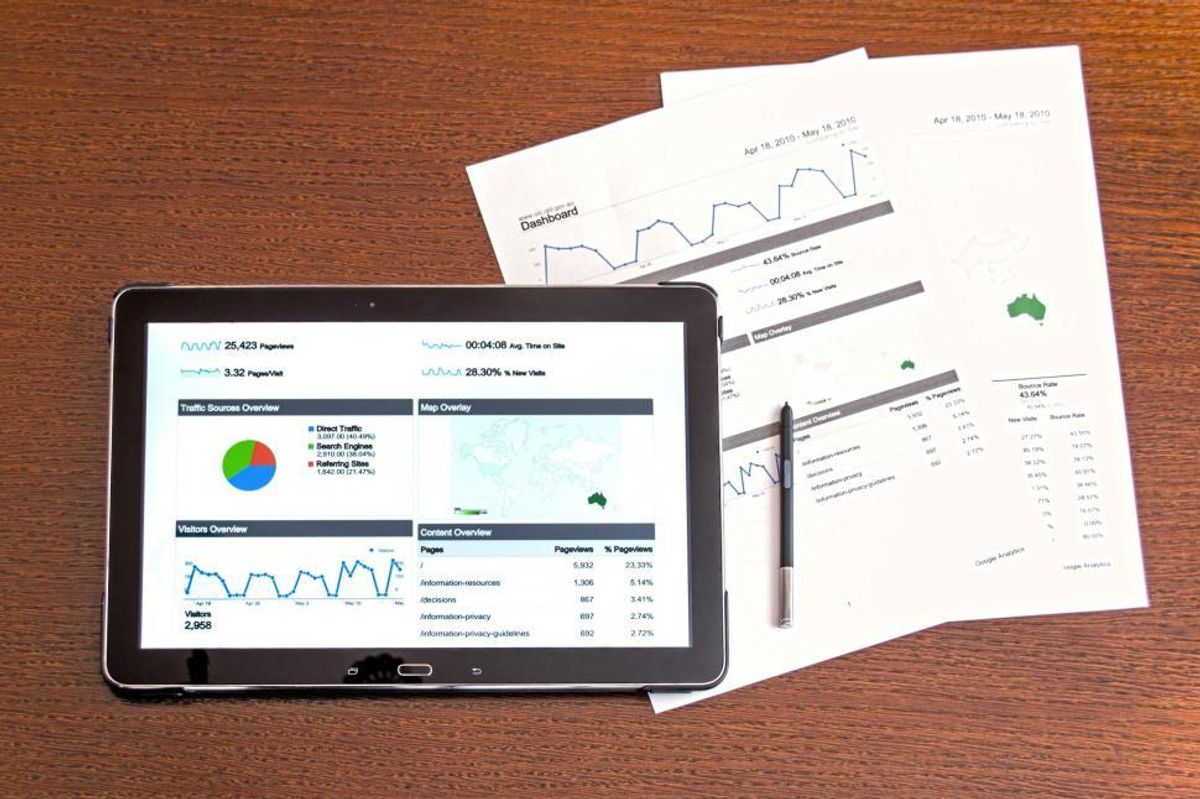
Customer Behavior Analysis
Understanding customer behavior is pivotal for tailoring marketing strategies that resonate with target audiences. By analyzing patterns in purchasing habits, preferences, and feedback, businesses can create more effective and personalized campaigns. The insights gained from customer behavior analysis are instrumental in driving engagement and conversions.
Customer segmentation is a critical aspect of behavior analysis, allowing companies to categorize their customers into distinct groups based on shared characteristics. This segmentation enables more focused and relevant marketing efforts. Here's a simple breakdown of customer segments based on purchasing behavior:
- Loyal Customers: Repeat buyers with high lifetime value
- Bargain Hunters: Price-sensitive customers often responding to discounts
- Occasional Shoppers: Infrequent buyers, usually driven by specific needs
- First-time Buyers: New customers potentially acquired through outreach
By leveraging advanced analytics, businesses can predict future buying trends and tailor their marketing to meet the evolving needs of their customer base. This proactive approach can significantly enhance customer satisfaction and loyalty.
It's essential to continuously refine the analysis techniques to keep pace with the dynamic nature of consumer behavior. The use of sophisticated data analytics tools can uncover hidden patterns that might otherwise go unnoticed, providing a competitive edge in the market.
Personalized Marketing Campaigns
In the realm of marketing, personalization has become a cornerstone for brands aiming to enhance customer engagement and boost conversion rates. Personalized marketing campaigns are tailored to meet the individual preferences and behaviors of customers, often resulting in a more meaningful connection between the consumer and the brand. By leveraging customer data, companies can create targeted messages that resonate on a personal level.
Personalization strategies can vary widely, but they often include the use of customer names, recommendations based on past purchases, and content that aligns with the user's browsing history. To illustrate the effectiveness of personalized marketing, consider the following table showing a simplified comparison of campaign performance metrics:
Metric | Standard Campaign | Personalized Campaign |
---|---|---|
Open Rate | 15% | 25% |
Click-Through Rate | 2% | 5% |
Conversion Rate | 1% | 4% |
Personalized marketing not only increases the likelihood of customer engagement but also significantly improves the chances of converting prospects into loyal customers.
To take personalized marketing to a new level, brands are exploring innovative approaches that strike a balance between being relevant and respecting privacy. The goal is to learn how personalized marketing works and see examples of brands that practice it in a fun, non-intrusive way, ensuring they do not come off as creepy. This approach to marketing is crucial in an era where consumers are increasingly aware of and sensitive to how their data is used.
Social Media Analytics
In the realm of social media, analytics play a pivotal role in understanding and engaging with the audience. Data from social platforms can reveal patterns and trends that are invaluable for shaping marketing strategies. For instance, by analyzing likes, shares, and comments, businesses can gauge the impact of their content and refine their approach.
Engagement rates and follower growth are key metrics that can be tracked over time to assess the effectiveness of social media campaigns. Additionally, the importance of establishing relationships between tables for data integrity and efficient querying is crucial in social media analytics, where primary and foreign keys are key concepts.
By leveraging social media analytics, companies can gain insights into customer preferences and behaviors, enabling them to create more targeted and compelling marketing messages.
Understanding the nuances of different platforms is essential for maximizing the impact of social media efforts. Here's a simple breakdown of key analytics metrics across various platforms:
- Facebook: Engagement, Reach, Page Views
- Twitter: Tweet Impressions, Profile Visits, Mentions
- Instagram: Likes, Comments, Saves
- LinkedIn: Post Interactions, Impressions, Clicks
Each platform offers unique insights, and by combining data from multiple sources, businesses can develop a comprehensive view of their social media presence.
Enhancing Supply Chain Management with Advanced Analytics
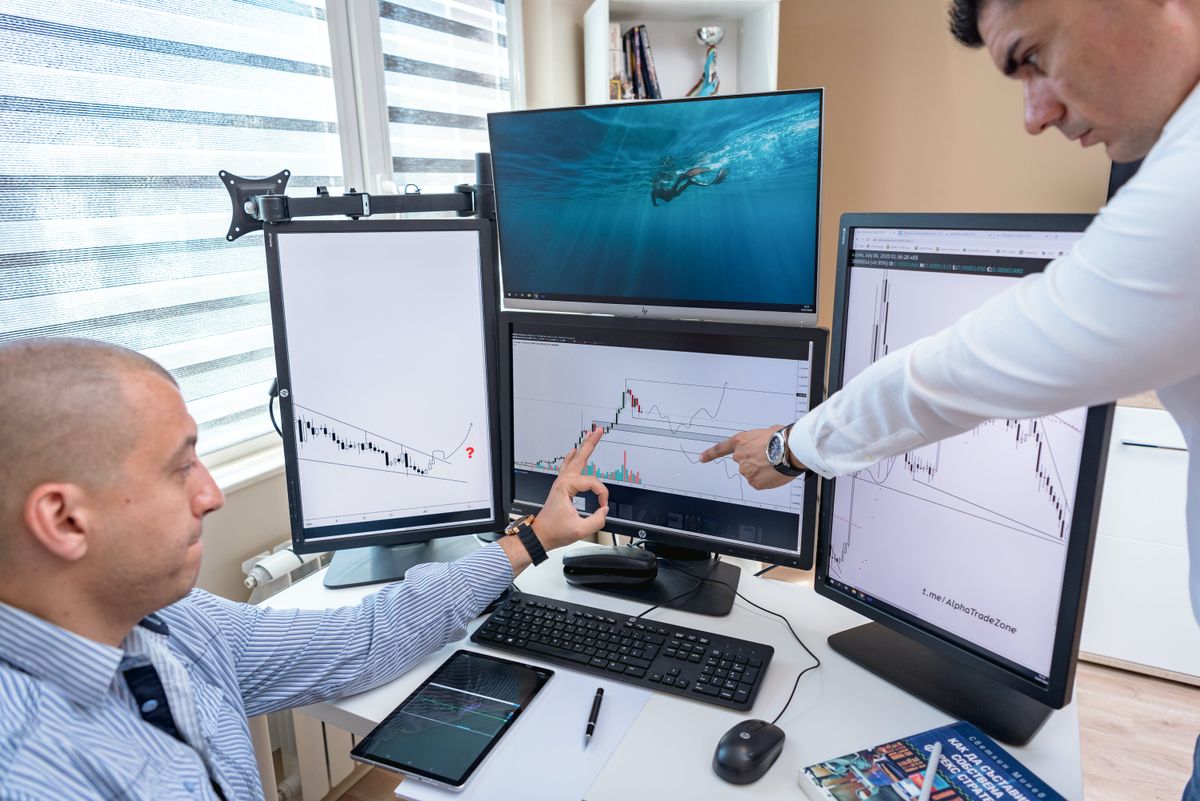
Demand Forecasting Techniques
Effective demand forecasting is pivotal for maintaining optimal inventory levels and ensuring customer satisfaction. By analyzing historical sales data, seasonal trends, and market conditions, businesses can predict future demand with greater accuracy. Accurate demand forecasting enables companies to reduce excess inventory costs and avoid stockouts.
Demand forecasting techniques vary from simple extrapolation of past sales to complex machine learning algorithms that consider a multitude of variables. The choice of technique often depends on the data available and the specific industry context.
- Historical Sales Analysis
- Time Series Analysis
- Causal Models
- Machine Learning Predictions
Embracing advanced analytics in demand forecasting can lead to more informed and strategic business decisions.
Cloud computing plays a crucial role in demand forecasting by providing the necessary computational power and data storage capabilities. It offers cost savings, scalability, and flexibility, which are essential for handling large datasets and running complex algorithms.
Inventory Optimization Strategies
In the realm of supply chain management, inventory optimization is a critical component that can significantly reduce costs and improve customer satisfaction. Effective inventory strategies ensure the right products are available at the right time, avoiding both overstock and stockouts. By leveraging advanced analytics, businesses can predict demand more accurately and optimize stock levels accordingly.
- Identification of slow-moving items that can be reduced
- Use of predictive analytics to adjust inventory levels for seasonal fluctuations
- Real-time tracking of inventory to enable dynamic reordering
Inventory optimization not only streamlines warehouse operations but also contributes to a more agile and responsive supply chain. It is a balance between minimizing the costs of holding inventory and meeting customer demand without delay.
To illustrate the impact of inventory optimization, consider the following table showing a simplified before-and-after scenario:
Metric | Before Optimization | After Optimization |
---|---|---|
Holding Costs | High | Reduced |
Stockouts | Frequent | Fewer |
Excess Inventory | Common | Minimal |
By adopting advanced analytics for inventory management, companies can achieve a leaner, more cost-effective supply chain that is better equipped to respond to market changes.
Supply Chain Risk Management
In the realm of supply chain management, risk management is pivotal to maintaining resilience against disruptions. By leveraging advanced analytics, businesses can anticipate potential risks and devise strategies to mitigate them. The use of predictive models allows for the identification of risk factors before they escalate into costly problems.
- Identification of potential supply chain disruptions
- Analysis of supplier risk profiles
- Continuous monitoring of logistic performance indicators
Advanced analytics empower organizations to create a proactive risk management culture, fostering a responsive and agile supply chain.
Effective risk management also involves the assessment of external factors such as geopolitical events, natural disasters, and market volatility. By integrating these insights into a comprehensive risk management plan, companies can safeguard their operations and ensure continuity even in the face of unforeseen challenges.
Utilizing Big Data for Competitive Advantage
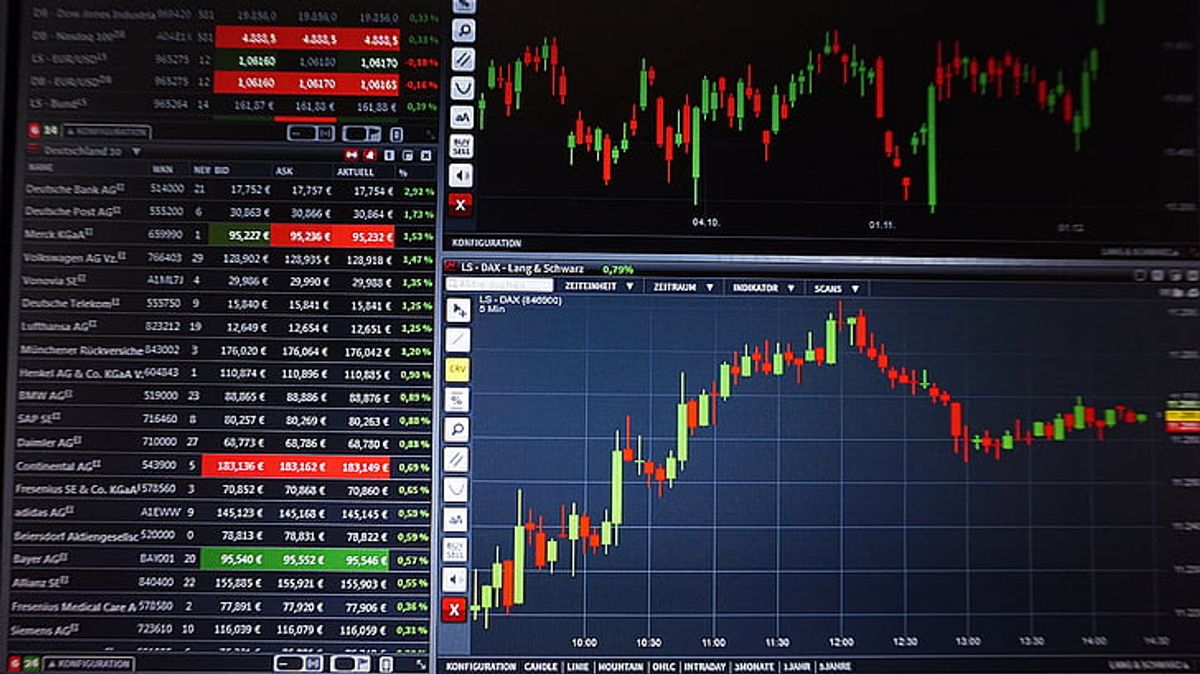
Real-time Data Processing
In the age of instant gratification, real-time data processing is a critical component for businesses looking to stay ahead. By processing data as it's generated, companies can make immediate decisions, gaining a competitive edge in fast-paced markets. This capability is powered by advancements in artificial intelligence (AI), which enable trustworthy data, automation of tasks, and interactive data communication.
Real-time analytics transform raw data into actionable insights, allowing businesses to respond to market changes with unprecedented speed.
The benefits of real-time data processing extend to various aspects of business operations, from customer service enhancements to more efficient supply chain management. Below is a list of key advantages:
- Enhanced operational effectiveness
- Improved cost management
- Instant customer feedback analysis
- Accelerated decision-making processes
Big Data Analytics Tools
In the realm of big data, analytics tools are the linchpin that transform vast amounts of raw data into actionable insights. Business Intelligence (BI) tools are at the forefront of this transformation, offering a suite of capabilities that cater to businesses of all sizes. These tools simplify the complex process of data analysis, enabling organizations to make informed decisions swiftly.
Analytics tools are not just about processing data; they also encompass the sharing and presentation of that information. It's crucial for businesses to effectively communicate their findings, and modern BI tools facilitate this by integrating with various platforms, including social media. This integration allows for immediate feedback and engagement with a wider audience.
The right big data analytics tool can be a game-changer for a business, providing a competitive edge by revealing trends and patterns that might otherwise remain hidden.
Here's a quick look at some of the top BI tools currently transforming the business landscape:
- Tableau: Renowned for its data visualization capabilities.
- Power BI: Microsoft's offering that integrates seamlessly with other MS products.
- Qlik: Known for its associative data modeling.
- SAS: Offers advanced analytics and is widely used in industries like healthcare and finance.
- Splunk: Focuses on analyzing machine-generated data.
- Looker: Now part of Google Cloud, known for its business analytics and user-friendly interface.
- Domo: Connects to multiple data sources and provides real-time dashboards.
- Sisense: Allows for interactive dashboard creation and is great for large datasets.
- Zoho Analytics: A budget-friendly option with a strong set of analytical tools.
Data Security and Privacy
In the era of big data, ensuring the security and privacy of collected information is paramount. Companies must navigate a complex landscape of regulatory requirements and ethical considerations to protect sensitive data. The use of advanced analytics can be a double-edged sword; while it enables deeper insights, it also raises significant privacy concerns.
- Establish clear data governance policies
- Implement robust encryption methods
- Regularly update security protocols
- Conduct frequent privacy impact assessments
By proactively addressing data security and privacy, businesses can build trust with their customers and avoid costly breaches.
It is crucial for organizations to stay ahead of potential threats by continuously evolving their security strategies. This includes investing in the latest technologies and training employees on best practices for data handling.
Driving Innovation through AI and Analytics
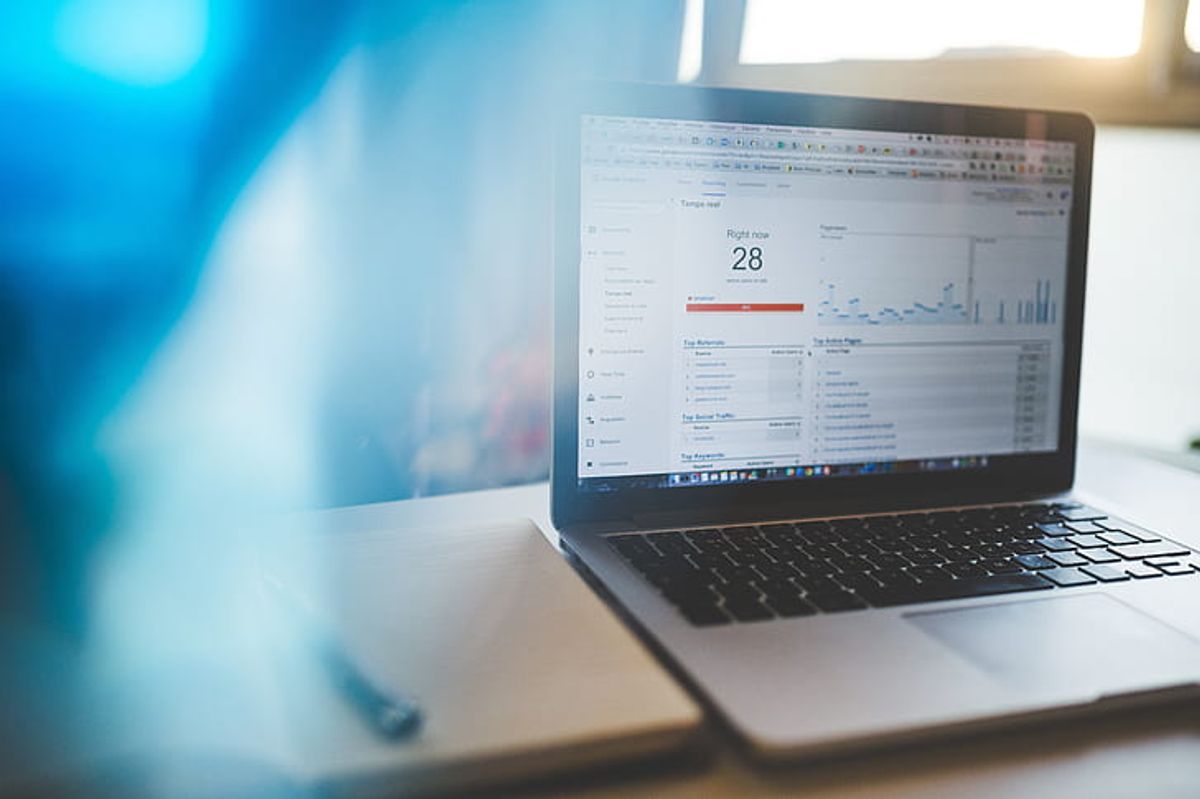
AI-powered Product Development
In the realm of product development, Artificial Intelligence (AI) is revolutionizing the way businesses innovate and bring new products to market. By leveraging AI algorithms, companies can analyze vast amounts of data to identify patterns and insights that would be impossible for humans to discern. This leads to the creation of more targeted and effective products that meet the evolving needs of consumers.
AI-powered product development is not just about automation; it's about augmenting human creativity with machine intelligence. For instance, AI can suggest design modifications, predict potential manufacturing issues, and optimize materials for sustainability and cost.
- Rapid prototyping and testing
- Enhanced customization options
- Predictive maintenance for product longevity
The integration of AI into product development cycles can significantly shorten the time from concept to market, ensuring that businesses stay ahead in competitive industries. By adopting AI, companies can expect to see a marked improvement in innovation efficiency and product success rates.
Enhanced Customer Experience with AI
Artificial Intelligence (AI) is revolutionizing the way businesses interact with their customers. By harnessing the power of AI, companies can provide a more personalized and efficient customer experience. AI-driven analytics can predict customer preferences and behavior, leading to more targeted and effective service offerings.
One of the key benefits of AI is its ability to process vast amounts of customer data in real-time. This enables businesses to respond quickly to customer inquiries and issues, often with automated solutions that can resolve problems without human intervention. For instance, chatbots powered by AI can handle a multitude of customer service tasks, from answering frequently asked questions to processing returns.
Customer loyalty is significantly enhanced when AI is integrated into customer service platforms. Personalized recommendations and proactive customer support can transform a one-time buyer into a repeat customer. The following table illustrates the impact of AI on customer service metrics:
Metric | Without AI | With AI |
---|---|---|
Response Time | >24 hours | <2 hours |
Issue Resolution | 70% | 95% |
Customer Satisfaction | 75% | 90% |
Embracing AI in customer experience initiatives is not just about technology; it's about creating a culture that values customer satisfaction and continuous improvement.
The integration of AI into customer experience also aligns with broader business strategies, such as cloud adoption and migration. These strategies ensure that customer data is handled securely and efficiently, providing a seamless experience that builds trust and loyalty.
Innovative AI Applications
The advent of Artificial Intelligence (AI) has opened up a plethora of innovative applications that are transforming industries. Businesses are now able to solve complex problems and create new opportunities by leveraging AI's advanced capabilities. One such application is the use of AI in enhancing customer interactions through chatbots and virtual assistants, providing a seamless and personalized experience.
Smart automation and AI-driven analytics are enabling companies to optimize operations and gain insights at an unprecedented scale. For instance, AI algorithms can predict maintenance needs in manufacturing, reducing downtime and increasing efficiency. Moreover, AI is instrumental in advancing healthcare through predictive diagnostics and personalized treatment plans.
- AI in autonomous vehicles: Enhancing safety and efficiency
- AI in finance: Fraud detection and algorithmic trading
- AI in agriculture: Crop monitoring and yield prediction
The integration of AI into business ecosystems is not just about technological advancement; it's about redefining the way we approach problem-solving and innovation.
The potential of AI to drive growth and create competitive advantage is immense. As AI technology continues to evolve, it will undoubtedly unveil new applications that will further revolutionize the business landscape.
Conclusion
In conclusion, leveraging advanced analytics is crucial for driving business growth in today's competitive landscape. By implementing data-driven strategies and gaining valuable insights, organizations can make informed decisions, optimize operations, and stay ahead of the curve. As businesses continue to prioritize data-driven decision-making, the role of advanced analytics will only become more significant. Stay tuned for more insightful articles on Business Intelligence and Analytics.
Frequently Asked Questions
What is the importance of data visualization in business analytics?
Data visualization helps businesses to easily interpret complex data and make informed decisions based on visual insights.
How can predictive analytics benefit a business?
Predictive analytics enables businesses to forecast future trends, identify potential risks, and optimize operations for better outcomes.
Why is data-driven decision making crucial for business success?
Data-driven decision making ensures that business decisions are based on factual data and insights, leading to more effective strategies and outcomes.
What are the key applications of machine learning in customer segmentation?
Machine learning can segment customers based on behavior, preferences, and demographics to personalize marketing strategies and improve customer engagement.
How can machine learning automate business processes?
Machine learning algorithms can automate repetitive tasks, streamline workflows, and improve efficiency in various business operations.
In what ways can data science enhance customer behavior analysis in marketing?
Data science techniques can analyze customer interactions, purchase patterns, and preferences to create targeted marketing campaigns and improve customer retention.
What are the benefits of real-time data processing in gaining competitive advantage?
Real-time data processing allows businesses to react quickly to market changes, identify opportunities, and make timely decisions to stay ahead of competitors.
How does AI-powered product development drive innovation in businesses?
AI technologies can analyze market trends, consumer feedback, and product performance data to develop innovative products that meet customer needs and preferences.