Understanding AI-Driven Innovation in Enterprise Software
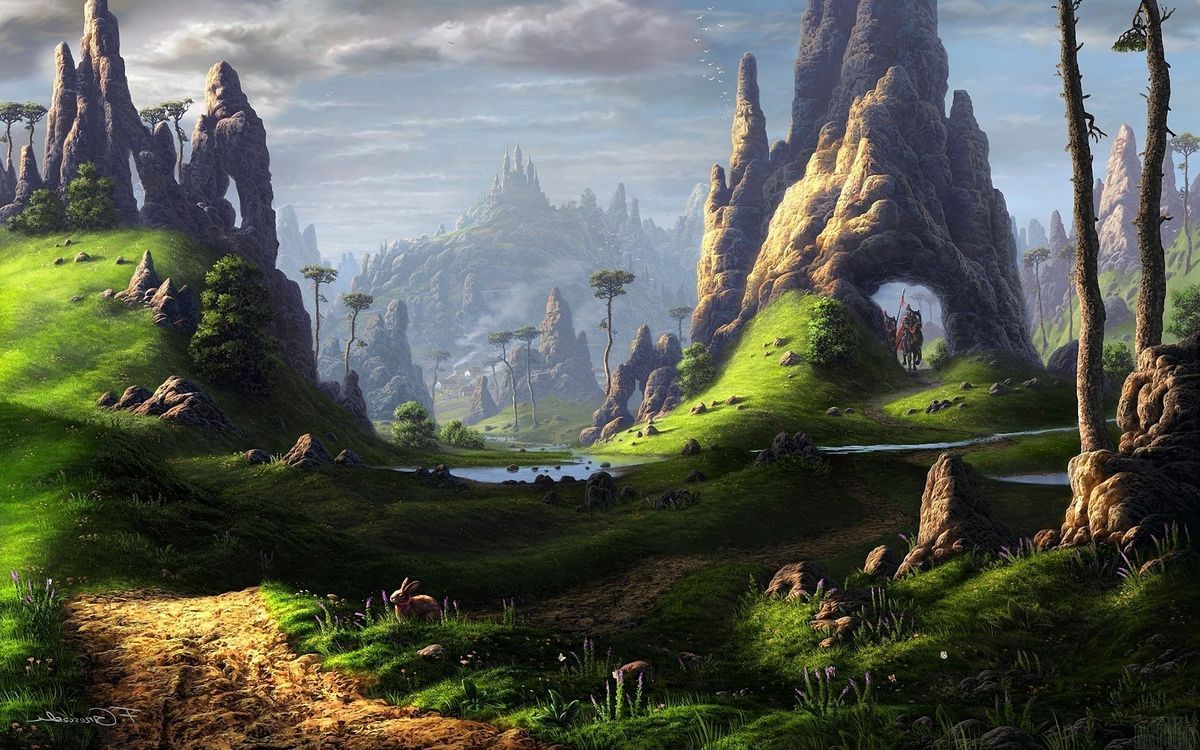
The Role of AI in Enhancing Innovation Processes
Artificial Intelligence (AI) is revolutionizing the corporate innovation process, bringing about a transformative shift in how companies conceptualize, develop, and deploy new products and services. By leveraging AI-driven insights, companies can reduce the time and resources spent on research and development, while increasing the likelihood of success in the market.
AI-powered innovation in software delivery and business processes revolutionizes efficiency, productivity, and competitiveness. Strategic alignment, technical readiness, and agile frameworks are key for success. For companies seeking to enhance their innovation processes with AI, understanding the available tools and technologies is crucial.
AI systems can identify emerging trends, enabling managers to make data-driven decisions and stay ahead of the curve. This proactive approach to innovation is essential for maintaining competitiveness in today's fast-paced business environment.
In the dynamic field of corporate innovation, AI-driven tools are revolutionizing how companies manage their innovation projects, providing deep insights and automating tasks that were once labor-intensive.
AI-Driven Tools for Streamlining Innovation Management
In the realm of enterprise innovation, AI-driven tools are becoming indispensable for their ability to enhance efficiency and provide actionable insights. These tools leverage machine learning and data analytics to automate and refine the innovation management process, offering a significant competitive advantage.
- Data Analysis: AI simplifies complex data analysis, enabling quicker decision-making.
- Idea Generation: Suggests innovative ideas by analyzing existing data trends.
- Process Automation: Streamlines routine tasks, freeing up time for strategic thinking.
By integrating AI into innovation management, companies can digitize their processes up to 10 times faster and at a fraction of the cost.
For organizations like those using Vizologi, an AI-powered innovation management software, the transition to AI-enhanced processes is not just about efficiency; it's about reimagining the potential of their innovation strategies. As AI continues to evolve, it will undoubtedly shape the future of how enterprises manage and execute their innovative ideas.
Predictive Analytics: Forecasting Trends and Outcomes
Predictive analytics, powered by AI, is revolutionizing the way enterprises forecast market trends and customer behaviors. By sifting through historical data, AI algorithms are able to project future market movements with remarkable precision. This foresight is not just about staying ahead; it's about being proactive in a market that's constantly evolving.
Predictive analytics is particularly effective in marketing, where understanding and anticipating customer behavior is paramount. Tailoring marketing strategies to align with predicted trends ensures that efforts are both relevant and timely. Here's how AI-driven predictive analytics can enhance various business aspects:
- Customer Interactions: Through sentiment analysis, businesses can gauge customer emotions and responses.
- Product Performance: Predictive maintenance can forecast potential issues before they arise, ensuring product reliability.
- Market Dynamics: Trend forecasting helps in anticipating shifts in the market, allowing companies to adjust strategies accordingly.
The predictive power of AI not only positions companies to meet market demands preemptively but also aids in risk assessment, steering innovation clear of potential pitfalls.
Machine learning's role in optimizing the innovation process is undeniable. It not only predicts outcomes but also suggests actionable improvements. These data-driven insights are invaluable for making informed decisions throughout the innovation lifecycle.
Navigating the Challenges of AI Implementation
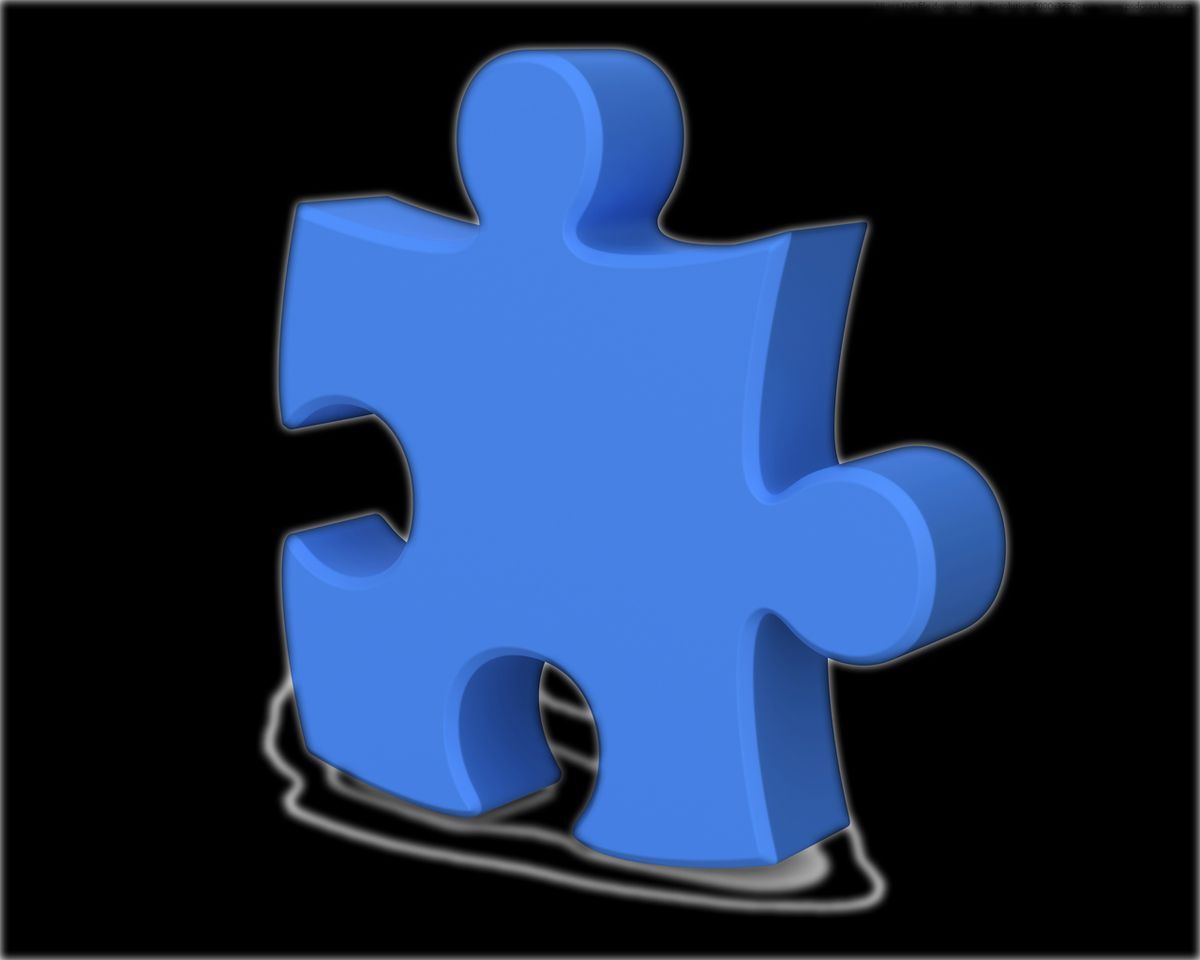
Identifying Common Pitfalls and How to Avoid Them
When integrating AI into enterprise systems, it's crucial to be aware of the potential pitfalls that can arise. Budgetary constraints often pose a significant challenge, especially for smaller organizations, as the cost of AI tools can vary widely. Moreover, the technical complexity and the steep learning curve of new technologies can intimidate non-technical staff.
Integrating human oversight and judgment is essential in AI decision-making processes to avoid serious flaws. This human-AI collaboration ensures that the system's outputs are aligned with organizational values and objectives. It's not just about avoiding errors; it's about harnessing AI to complement and enhance human capabilities.
Each of these challenges requires careful attention and a proactive approach. By addressing these considerations, organizations can enhance the effectiveness and acceptance of AI in their corporate innovation processes, leading to more successful outcomes and sustained growth.
Learning to communicate effectively with AI systems, through prompt engineering, is another critical skill. Asking the right questions is paramount because the quality of AI's output is directly related to the input it receives. This skill becomes particularly important when trying to extract the most value from AI investments.
Ensuring Ethical and Strategic AI Deployment
In the journey to integrate AI into enterprise software, ensuring ethical and strategic deployment is paramount. Addressing resistance to change, financial barriers, fostering AI literacy, and ensuring data security and compliance are key in successful AI deployment. Ethical considerations like data privacy and algorithmic bias must also be prioritized.
Continuous monitoring and auditing are critical to upholding these ethical standards. Regular audits by trained experts are necessary to assess AI performance and identify potential issues like biases that may emerge over time.
To operationalize these principles, organizations should focus on:
- Transparency in AI decision-making processes
- Bias Mitigation through continual testing and updates
- Stakeholder Engagement in ethical AI discussions
Defining an organizational structure for AI governance is essential, including roles, responsibilities, and an independent body to oversee AI compliance. This structure ensures responsible and ethical usage of AI, aligning with the company's values and societal norms.
Data Automation: Achieving Flexibility and Scale
In the realm of enterprise AI, data automation stands as a cornerstone for achieving both flexibility and scale. By standardizing integration with the best AI models and adding new data feeds through configuration-based development, businesses can adapt swiftly to changing needs. Automation enables out-of-the-box elasticity, CI/CD automation, and observability, ensuring that systems can scale without proportional increases in complexity or maintenance burden.
- Data automation provides the agility for AI-driven operations, allowing teams to concentrate on strategic, value-adding tasks.
- It simplifies the process of integrating new AI models and managing existing data, reducing the maintenance overhead.
- The focus shifts from manual, labor-intensive processes to strategic tasks that leverage AI for innovation and efficiency.
Data automation is your defense against building an ever-more-complex environment that is hard to maintain. It is not just about doing more with less; it's about enabling your enterprise to move faster, with greater precision and foresight.
Choosing between building your own AI data platform or opting for a ready-made solution is a strategic decision. While building in-house may offer ultimate flexibility, it can lead to increased complexity over time. On the other hand, ready-made solutions can provide powerful data transformation capabilities and integrated machine learning and AI tools, which are essential for processing and preparing data for analysis.
AI in Action: Case Studies in Shipping and Logistics
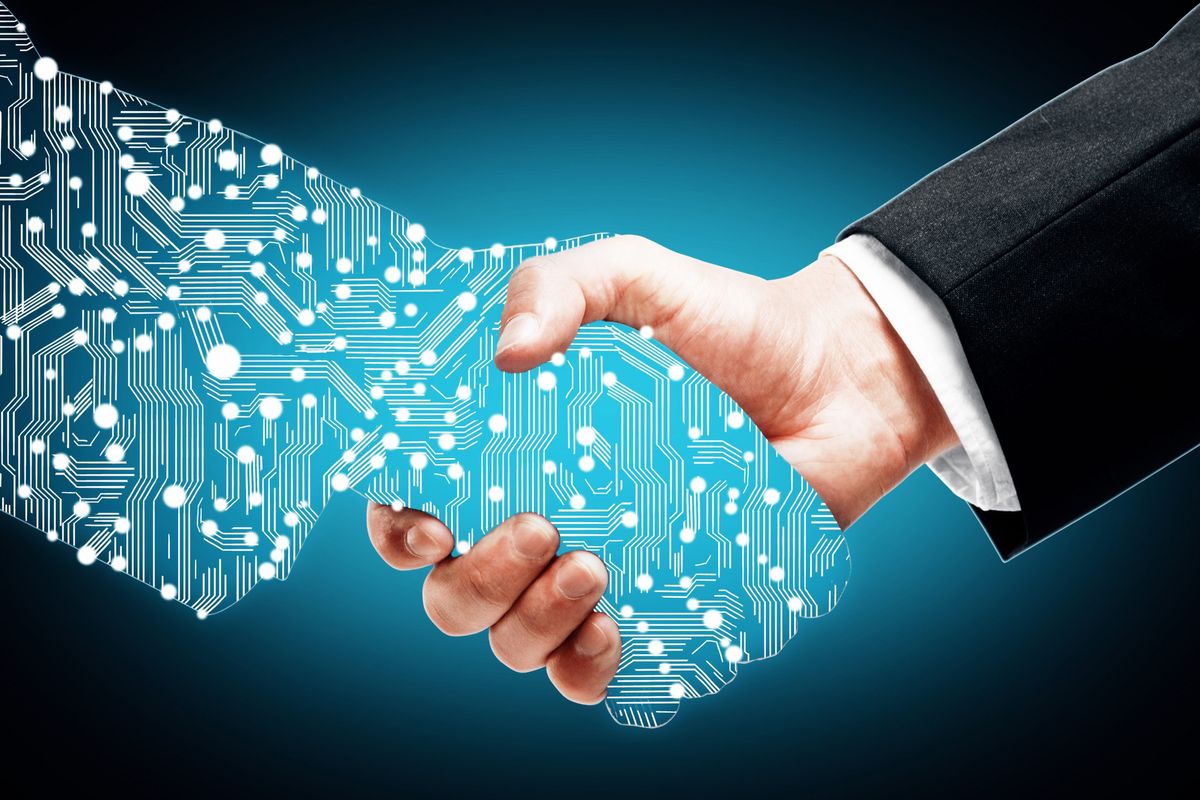
Improving Efficiency with AI-Enabled Technologies
The advent of artificial intelligence (AI) is transforming the landscape of enterprise software delivery. AI is revolutionizing enterprise software delivery by automating tasks, analyzing data, and enhancing decision-making processes. This shift is not just about technology; it's about redefining the way businesses operate to achieve unprecedented levels of efficiency.
Predictive supply chain management and AI-driven customer interactions are reshaping workflows for increased efficiency and productivity.
By integrating AI into their systems, companies are able to automate repetitive tasks, freeing up human resources for more strategic initiatives. The benefits of this automation are twofold: it reduces the potential for human error and accelerates the pace of work. Furthermore, AI's capability to analyze large datasets can uncover insights that drive smarter business decisions.
The table below illustrates the impact of AI on key operational metrics:
Metric | Before AI | After AI |
---|---|---|
Task Completion Time | 8 hours | 2 hours |
Error Rate | 5% | 0.5% |
Customer Response Time | 24 hours | 1 hour |
As AI systems continuously learn and improve, the innovation process becomes more efficient over time. However, it's crucial to ensure that AI implementation is fundamentally connected to business outcomes, avoiding the pitfall of treating AI as a mere technological distraction.
The Future of Machine Learning in Warehouse Management
The integration of machine learning (ML) technology in warehouse management is a transformative force, poised to redefine operational efficiency. AI-driven inventory management is a prime example of this shift, with companies like Heineken already leveraging ML algorithms to forecast demand and maintain optimal stock levels. This not only reduces storage costs but also enhances customer satisfaction by ensuring product availability.
As ML technology continues to evolve, its ability to optimize algorithms over time promises even greater advancements. The use of delivery drones and picking machines, powered by AI, exemplifies the ongoing innovation in the field. These tools are not just a fleeting trend but a glimpse into a future where AI and ML are integral to warehouse operations.
The future of AI in data warehousing is not just about the technology itself, but how it seamlessly integrates into existing processes to create a more agile and responsive environment.
The table below outlines key trends and predictions for AI in warehouse management:
Trend | Prediction |
---|---|
Autonomous Vehicles | Increased safety and efficiency through perpetual ML improvements |
AI-Optimized Inventory | Lower storage costs and higher profitability |
Advanced Picking Technologies | Enhanced productivity with AI-enabled devices |
Assessing Long-Term Benefits of AI Investments
When evaluating the long-term benefits of AI investments, it's essential to consider how AI acts as an exoskeleton to business processes, enhancing a company's existing strengths. Investors should focus on strategic AI partners who understand the nuances of control, ownership, and accountability, ensuring that AI drives sustainable value rather than short-term allure.
Continuous improvement is key in AI integration. Organizations must regularly review and refine their AI strategies to align with innovation goals. This iterative process involves educating teams, measuring AI's impact, and adapting to new insights and market conditions.
The true measure of AI's long-term value lies in its ability to automate repetitive tasks, leading to increased efficiency and strategic freedom for employees. This not only reduces costs but also improves the overall innovation capacity of the enterprise.
Here are some steps to maximize the benefits of AI in innovation:
- Regularly assess AI's impact on efficiency and decision-making.
- Educate teams on leveraging AI-driven tools for financial analysis and forecasting.
- Ensure AI integration is a continuous journey, not a one-time initiative.
Maximizing AI's Impact on Innovation
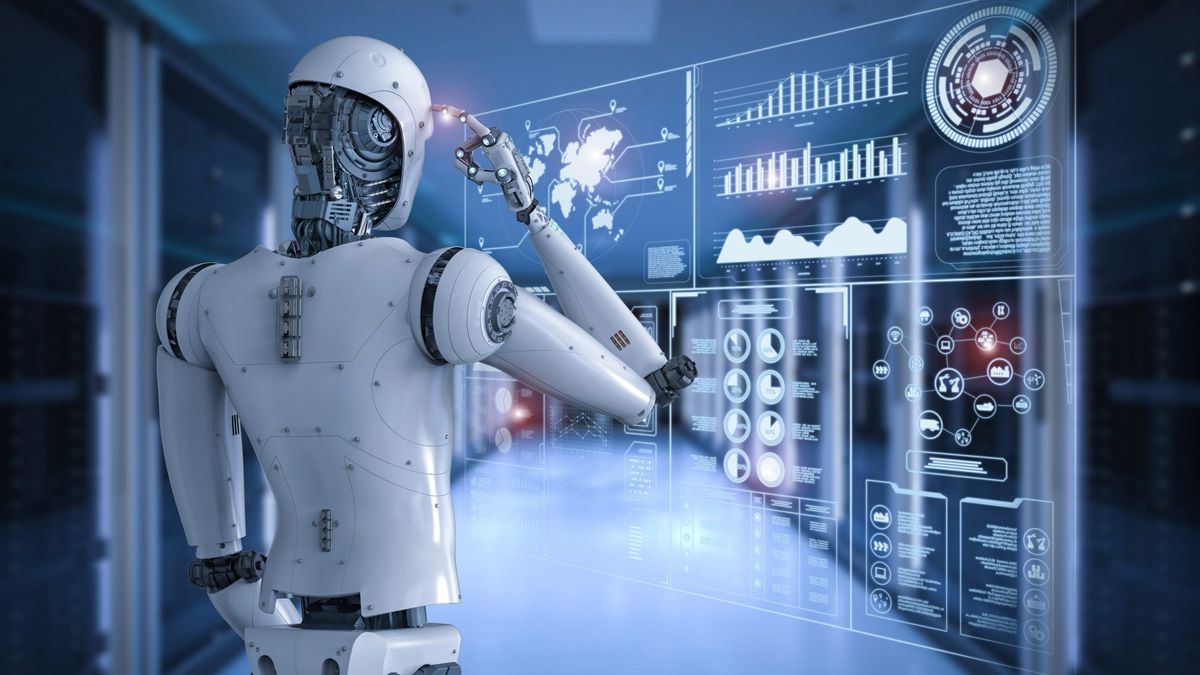
Measuring the Success of AI Integration
To validate the investment in AI, companies must establish metrics that can quantifiably measure its impact on the innovation process. Key performance indicators (KPIs) might include the efficiency of innovation projects, decision-making quality, and success rates. These metrics provide tangible evidence of AI's value, allowing for data-driven decisions about future investments in AI technologies.
Iterative refinement of AI models and algorithms is crucial, based on real-world outcomes and data. Organizations should regularly review their AI strategies and tools to ensure they remain aligned with innovation goals. This includes soliciting feedback from team members on the effectiveness of AI tools and staying informed about new AI developments.
AI integration should not be a one-time initiative but a continuous journey of improvement. Embracing a culture of data-driven innovation fosters a proactive approach to refining AI strategies, leading to more successful outcomes and sustained growth.
Continuous Refinement of AI Strategies for Innovation
The journey of integrating AI into enterprise innovation is ongoing and demands continuous refinement. To stay ahead, organizations must embrace a cycle of evaluation and enhancement, ensuring that AI strategies evolve with the changing landscape of technology and business needs.
Iterative refinement is not just about technology upgrades; it involves reassessing goals, measuring outcomes, and nurturing an innovative culture. Here's a simple framework to guide this process:
- Assess: Regularly evaluate AI performance and strategic alignment
- Measure: Quantify the impact of AI on innovation outcomes
- Learn: Gather insights to inform future AI initiatives
- Adapt: Make necessary adjustments to strategies and tools
By embedding these steps into the innovation workflow, companies can create a resilient framework that adapts to new challenges and opportunities.
Maximizing AI's potential in innovation is a structured journey. It requires a commitment to not just deploy AI tools, but to also invest in the human elements of creativity and strategic thinking. This balanced approach ensures that AI becomes a powerful ally in the quest for groundbreaking innovations.
Educating Teams for Effective AI Utilization
For AI to be successfully integrated into the innovation process, it is essential that teams are well-versed in the capabilities and operation of AI tools. Training should encompass not only the technical aspects but also the strategic application of AI in various innovation phases. This involves:
- Developing comprehensive training programs tailored to the specific AI tools adopted by the organization.
- Encouraging collaborative learning environments where team members can share insights and experiences.
- Providing continuous learning opportunities to keep pace with evolving AI technologies and methodologies.
Harnessing AI for enterprise software delivery requires a concerted effort to educate teams on both the technical and strategic aspects of AI. By focusing on areas that are monotonous, time-intensive, or error-prone, organizations can prioritize AI interventions that tackle these issues head-on, enhancing efficiency and productivity.
To fully realize the benefits of AI, organizations must focus on educating their teams, measuring AI's impact, and continuously refining AI strategies.
It is also vital to:
- Solicit feedback from team members on the effectiveness of AI tools.
- Stay informed about new AI developments and assess their potential application within the company.
- Iteratively refine AI models and algorithms based on real-world outcomes and data.
Empowering Teams with AI Tools
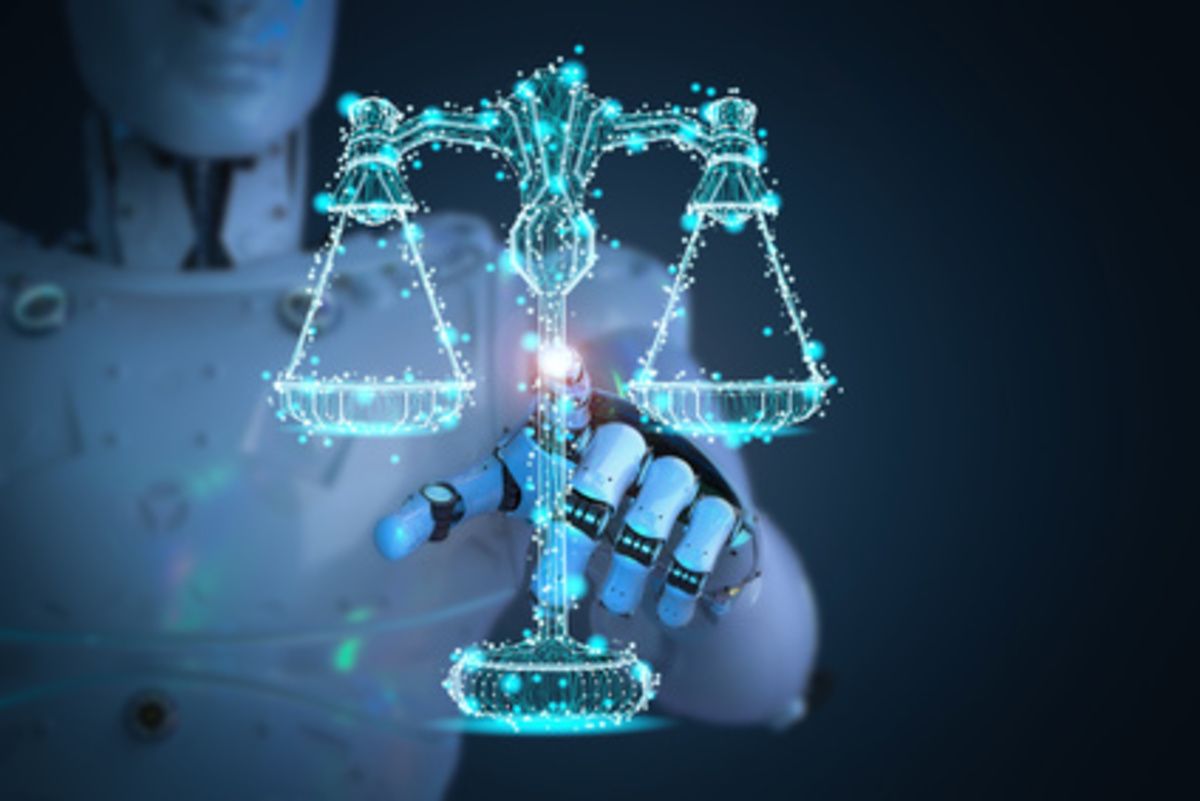
Training for Strategic Use of AI in Business Operations
For businesses to fully harness the power of AI, it is imperative to invest in employee training. This not only involves understanding the technicalities of AI but also ensuring its safe and ethical use. Training programs should aim to explore strategies for safe AI employee training, integrating AI responsibly, ensuring data security, and fostering an environment of continuous learning.
By educating teams on AI ethics and applications, companies empower their workforce to leverage AI tools for enhanced efficiency and innovation while being cognizant of its potential and limitations.
The core functionalities of AI, such as machine learning, natural language processing, and deep learning, are transforming business operations. It is crucial for teams to understand these technologies to optimize decision-making and drive innovation. Here's a list of key areas where AI training can make a significant impact:
- Understanding and applying machine learning algorithms
- Leveraging natural language processing for customer interactions
- Utilizing deep learning to automate complex tasks
- Ensuring data security and privacy in AI applications
Starting with small, targeted initiatives in areas that deliver significant impacts, such as monotonous or error-prone tasks, can pave the way for successful AI integration.
Automating Data Pipelines to Enhance Team Productivity
In the realm of enterprise software delivery, automating data pipelines is a critical step towards enhancing team productivity. By streamlining the integration of AI models and adding new data feeds through configuration-based development, teams can focus on high-value strategic tasks.
Automation in data pipelines not only saves time but also ensures that projects remain on schedule and resources are allocated effectively.
The benefits of data pipeline automation include:
- Standardization of AI model integration
- Configuration-based development for easy addition of new data sources
- Scalability achieved through CI/CD automation and observability
With the right tools and processes in place, the innovation cycle can become increasingly efficient as AI systems learn and improve over time.
The Role of the Chief AI Officer in Enterprise Strategy
The Chief AI Officer (CAIO) plays a pivotal role in steering an organization's AI journey. Strategic AI deployment requires enterprise standards and flexibility for innovation. This executive is responsible for establishing a cohesive AI vision that aligns with the company's overall strategy, while also fostering an environment conducive to innovation.
To achieve this, the CAIO must navigate the complexities of integrating AI into various business processes, ensuring that the technology is leveraged ethically and effectively. Stakeholder engagement and shared ownership are crucial for effective AI integration, ensuring alignment with business objectives and continuous improvement. The CAIO's responsibilities extend beyond mere implementation; they include the cultivation of a data-driven culture and the development of AI governance frameworks to guide responsible usage.
The CAIO's leadership is instrumental in transforming AI from a novel technology into a core component of business strategy, driving growth and maintaining competitive advantage.
A successful AI strategy hinges on the CAIO's ability to collaborate across departments, breaking down silos and fostering cross-functional teams. This ensures that AI initiatives are not only technically feasible but also resonate with the company's mission and customer needs.
Choosing the Right Data Platform for AI
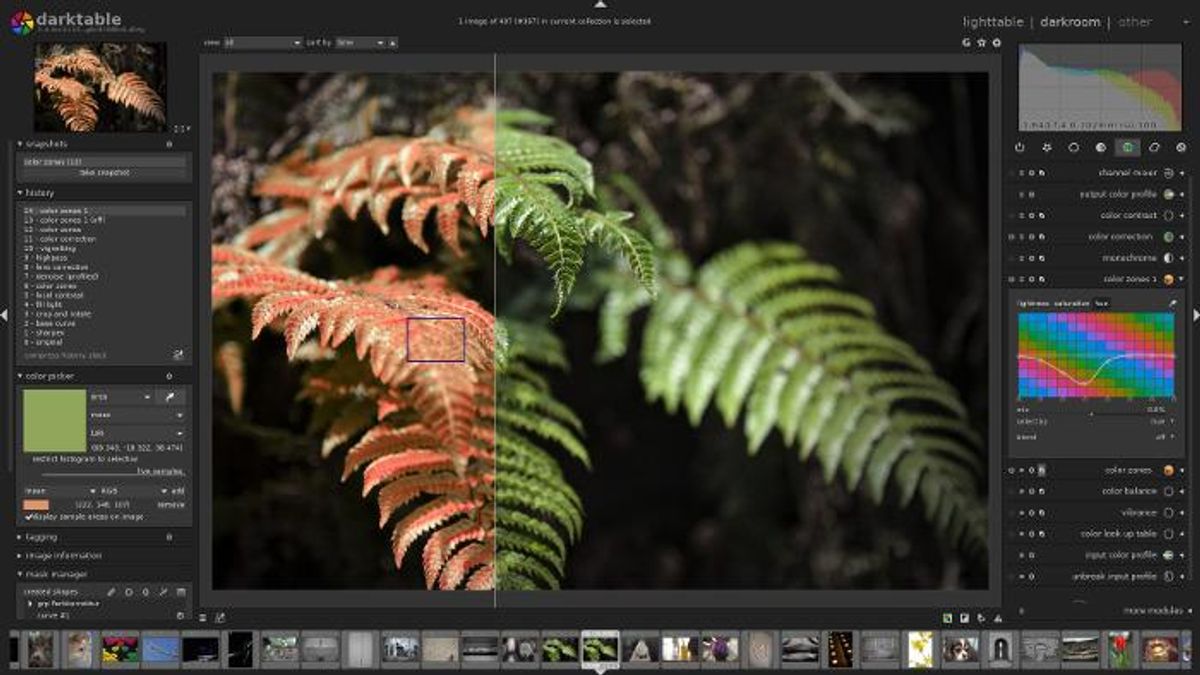
Build Your Own vs. Ready-Made Solutions
When it comes to powering AI projects with the right data platform, enterprises face a critical decision: should they invest in building a custom solution or opt for a ready-made platform? Building your own platform offers unparalleled customization, but it's essential to consider the long-term implications such as maintenance, scalability, and the ability to keep your team's focus on core business objectives.
On the other hand, ready-made solutions can provide a quicker start and often come with a suite of features that have been tested and proven in the market. However, they may lack the flexibility to meet specific business needs or integrate seamlessly with existing systems. Here's a quick comparison to help guide the decision:
- Custom Build: Tailored to specific needs, ultimate control, potential for competitive advantage.
- Ready-Made: Faster deployment, lower initial cost, community support and updates.
The choice between building or buying should align with your company's long-term strategy and capacity for managing AI infrastructure. It's not just about the technology; it's about how it fits into the broader picture of your enterprise's goals and capabilities.
The decision also hinges on the ability to navigate the complexities of AI integration. To make an informed choice, it's crucial to identify potential issues early, understand your data landscape, set clear performance metrics, employ skilled data scientists, ensure robust security measures, and align the solution with your overarching business goals.
Integrated Machine Learning and AI Tools
To harness the full potential of AI in enterprise software, it's crucial to have a data platform that integrates machine learning and AI tools. This allows for a more fluid development process, from building and training models to deploying them into production. Seamless integration is key to accelerating the AI development lifecycle, fostering rapid iteration, and propelling innovation forward.
Machine learning algorithms are the workhorses behind AI tools, analyzing data to identify patterns and make predictions. When integrated within your data platform, these algorithms empower businesses with actionable insights, often revealing opportunities that might otherwise go unnoticed. For example, analyzing customer feedback through machine learning can uncover unmet needs or emerging trends, providing a competitive edge.
Ensuring that your data platform and AI tools work in harmony is not just a technical requirement; it's a strategic imperative that can significantly impact your enterprise's capacity for innovation.
The table below outlines the benefits of integrating machine learning and AI tools into your data platform:
Benefit | Description |
---|---|
Efficiency | Streamlines the AI development process |
Insight | Provides deep insights from data analysis |
Innovation | Enables faster iteration and innovation |
By equipping teams with the right training and understanding of these tools, enterprises can leverage AI to not just enhance but revolutionize their innovation management.
Optimizing Compute Resources for AI Workloads
Acquiring and managing the compute resources required for AI workloads is a critical step in ensuring the efficiency and effectiveness of AI systems. Resource optimization is key to managing computing resources efficiently and avoiding bottlenecks that can impede AI processes.
- Cloud Storage
- Elastic Compute
- Database Compute
- Infrastructure Automation
These components are essential for handling the processing power required for training complex models. Seamless integration with machine learning and AI tools within your data platform can streamline the AI development lifecycle, enabling faster iteration and innovation.
By starting with small, targeted AI initiatives in areas that deliver significant impacts, organizations can gradually build up their AI capabilities while managing compute resources effectively.
It's also important to evaluate if your organization's data environment is equipped to handle the demands of AI. This includes assessing cloud infrastructure requirements and ensuring that your team has the necessary technical knowledge to confidently navigate AI implementation.
AI-Enhanced Customer Engagement Strategies
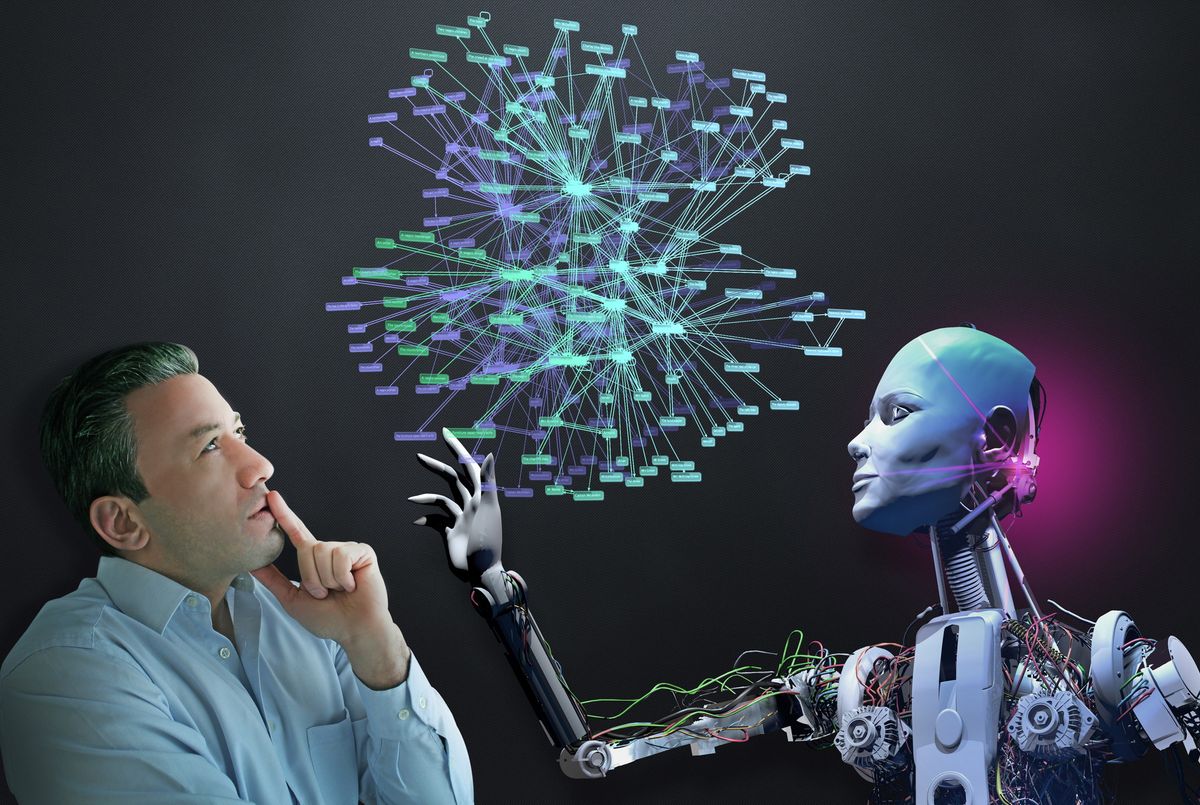
Anticipating Customer Needs with AI
In the realm of customer engagement, AI stands as a transformative force, enabling businesses to anticipate customer needs with unprecedented precision. By harnessing the power of predictive analytics, companies can now forecast customer behaviors and preferences, tailoring their services to meet expectations before they are explicitly expressed.
AI's predictive capabilities extend beyond mere conjecture, transforming vast amounts of data into actionable insights that drive proactive customer support.
For instance, consider the application of AI in predicting maintenance needs. By analyzing historical data and identifying patterns, AI facilitates the creation of maintenance schedules that preemptively address potential issues, thereby enhancing customer satisfaction through reliability and attentiveness.
The integration of AI into customer support systems allows for a more personalized approach. AI algorithms can sift through customer interaction history, enabling support teams to offer recommendations and support experiences that are uniquely tailored to each individual. This level of personalization was exemplified by KFC China's use of facial recognition technology to predict orders, showcasing the potential of AI to revolutionize customer service.
Ultimately, the strategic deployment of AI in anticipating customer needs not only elevates the customer experience but also fosters loyalty and trust, positioning businesses at the forefront of customer-centric innovation.
Crafting Personalized Content at Scale
In the realm of customer engagement, AI-driven personalization stands out as a transformative force. By leveraging user data, AI tools can tailor content to individual preferences and behaviors, much like how Netflix offers personalized recommendations to its viewers. This not only enhances the user experience but also boosts engagement significantly.
The advent of AI in content generation is reshaping how marketers approach their campaigns. Tools like Sprout's Message Ideas by AI Assist exemplify this shift, providing content suggestions that resonate with a brand's voice and audience. Such capabilities allow for the creation of personalized content at scale, without compromising on the strategic elements of marketing.
The potential of AI extends to operational efficiency, enabling automated timing of social media posts and streamlined email marketing campaigns. This operational leverage is crucial for maintaining a consistent and personalized dialogue with consumers.
Marketers are now equipped with AI tools that predict and deliver tailored interactions with remarkable precision. For instance, loyalty programs can benefit immensely from AI, as it enhances key touchpoints for a brand's most valuable customers, from loyalty benefits to differentiated service experiences.
Developing Data-Driven Customer-Centric Approaches
In the realm of customer engagement, developing data-driven customer-centric approaches is paramount. By leveraging AI, enterprises can gain a deeper understanding of customer behaviors and preferences, leading to more personalized and effective strategies. For instance, AI-powered competitive analysis tools can instantly provide insights that inform strategic decisions, ensuring that customer needs are at the forefront of business planning.
AI-Powered Competitive Analysis
- Instant insights for strategic decision-making
- Enhanced understanding of customer needs
- Personalized engagement strategies
By focusing on business outcomes and prioritizing applications that impact the bottom line, organizations can ensure that their AI investments are not just innovative, but also economically beneficial.
Loyalty programs, often underutilized, present a significant opportunity for AI to make a difference. Natural language processing can transform call center interactions into actionable data, improving customer experiences and loyalty program effectiveness. It's essential to adopt a cross-functional approach to fully harness the potential of AI in enhancing loyalty programs and driving business growth.
The Future Landscape of AI in Business
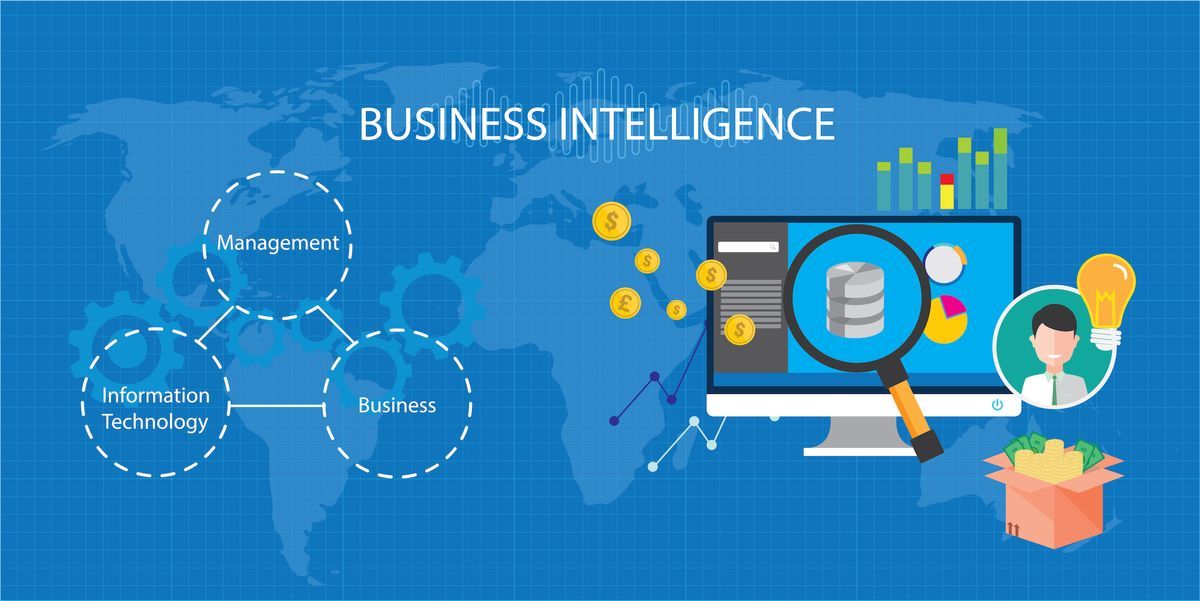
Shaping the Future with Strategic AI Implementation
The integration of artificial intelligence (AI) into business operations is not just about adopting new technologies; it's about strategically enhancing the capabilities of an organization. Businesses that align AI with their core objectives are setting the stage for a transformative future.
By focusing on strategic AI implementation, companies can ensure that their investments are not only technologically sound but also aligned with their long-term vision and ethical standards.
Here's how AI is contributing to different phases of innovation:
Innovation Phase | AI Contribution |
---|---|
Ideation | AI-driven trend analysis and idea generation |
Development | Rapid prototyping and testing with AI simulations |
Commercialization | Market analysis and targeting through AI algorithms |
Post-Launch | AI-enabled performance tracking and feedback analysis |
To fully harness the potential of AI, it is essential to educate and empower teams. This involves not only providing the necessary tools and resources but also fostering an environment that encourages experimentation and continuous learning. As AI continues to evolve, so too must the strategies and approaches of the businesses that utilize it.
Convergence of Technology and Human Ingenuity
The convergence of technology and human ingenuity is not just a futuristic concept; it is a tangible reality that is reshaping the landscape of enterprise software delivery. The future of software engineering is exciting, promising a new era of development where AI-driven tools and human creativity work in tandem. This partnership is crucial for fostering an environment where human intuition and AI's data-driven insights complement each other, leading to innovative solutions.
By integrating AI into the innovation process, companies can digitize their innovation process faster and at a fraction of the cost. However, it is vital to remember that AI is not a silver bullet. It is a powerful tool that, when used thoughtfully, can catalyze significant advancements. As we step into the future, businesses that smartly leverage technology will not only gain a competitive edge but also unlock their full potential.
Balancing human creativity with AI is essential. The integration should be viewed as a partnership, with each bringing unique strengths to the table.
Driving Growth and Customer Satisfaction with AI
The integration of AI into business strategies is not just about adopting new technologies; it's about enhancing the customer experience in profound ways. By analyzing customer data and behavior, AI enables companies to anticipate needs and tailor their offerings accordingly, ensuring that each interaction is as relevant and engaging as possible.
By embracing AI, businesses are not only streamlining operations but also setting the stage for sustained growth and deeper customer relationships.
AI-driven tools, such as those offered by Sprout Social, allow for the crafting of personalized content at scale, which resonates with customers and fosters loyalty. The ability to segment behaviors and target specific customer groups with precision is at the heart of this AI revolution, driving smarter decisions and more personalized strategies.
Here are some strategies to consider for improving personalization and efficiency in loyalty programs:
- Utilize AI for social listening to understand customer sentiment.
- Automate ticketing processes to enhance customer support.
- Leverage trend analysis for deeper insights into customer preferences.
- Craft messages that resonate by analyzing customer interactions.
The future of customer engagement is being shaped by AI's ability to provide a seamless and customized experience, which is crucial for driving growth and ensuring customer satisfaction.
As we stand on the brink of a technological revolution that will fundamentally alter the way we live, work, and relate to one another, the role of AI in business is becoming increasingly significant. In this transformative era, the need for optimized data solutions is paramount. At OptimizDBA, we offer unparalleled database optimization consulting to ensure your business stays ahead of the curve. Don't let your data management lag behind; visit our website to learn how we can help you experience transaction speeds that are not just faster, but potentially 100 times faster. Take the first step towards a smarter business future—connect with us today!
Conclusion
In conclusion, the transformative potential of AI in enterprise software delivery is undeniable. As we have explored, AI-driven tools and technologies offer remarkable opportunities for companies to enhance efficiency, innovation, and decision-making. From automating mundane tasks to providing deep analytical insights and optimizing compute resources, AI is reshaping the landscape of enterprise software delivery. However, it is crucial for businesses to approach AI implementation strategically, with a focus on training, ethical considerations, and continuous refinement of AI strategies. By doing so, enterprises can not only avoid potential pitfalls but also maximize the benefits of AI, ensuring they remain competitive in a rapidly evolving digital world. As we step into the future, embracing AI is not just an option but a necessity for businesses aiming to thrive and excel in their respective industries.
Frequently Asked Questions
What are the benefits of using AI in enterprise software delivery?
AI-powered software delivery solutions enhance innovation processes, provide deep insights, automate repetitive tasks, and offer predictive analytics for better decision-making, ultimately maintaining a competitive edge.
What challenges might companies face when implementing AI tools?
Companies may encounter issues such as data privacy concerns, integration complexities, a lack of expertise, and ethical considerations. Avoiding these pitfalls requires careful planning, strategic deployment, and continuous education.
How can data automation benefit my business?
Data automation standardizes AI model integration, adds new data feeds with ease, and achieves scalability through configuration-based development, CI/CD automation, and improved observability.
In what ways is AI transforming innovation management?
AI-driven tools for innovation management automate labor-intensive tasks, provide actionable insights, and streamline the innovation process, leading to higher efficiency and success rates in innovation projects.
How is AI used in the shipping and logistics industries?
AI enhances efficiency and productivity in shipping and logistics through technologies such as delivery drones, picking machines, and machine learning algorithms that optimize operations and continue to improve over time.
Why is it important for businesses to leverage AI strategically?
Strategic AI utilization catalyzes development and achievements, allowing businesses to stay competitive and reach new heights in the market by leveraging technology as a pivotal strategy.
What role does training play in maximizing the benefits of AI in innovation?
Training is crucial for teams to effectively leverage AI tools, enabling them to focus on strategic tasks and add value, while AI handles manual, time-consuming processes.
Should a business build its own AI data platform or use a ready-made solution?
The choice between building an AI data platform or using a ready-made solution depends on the company's specific needs, resources, and strategic goals. Both options have their own set of advantages and considerations.