Understanding the Role of Data Analytics in Strategic Decision-Making
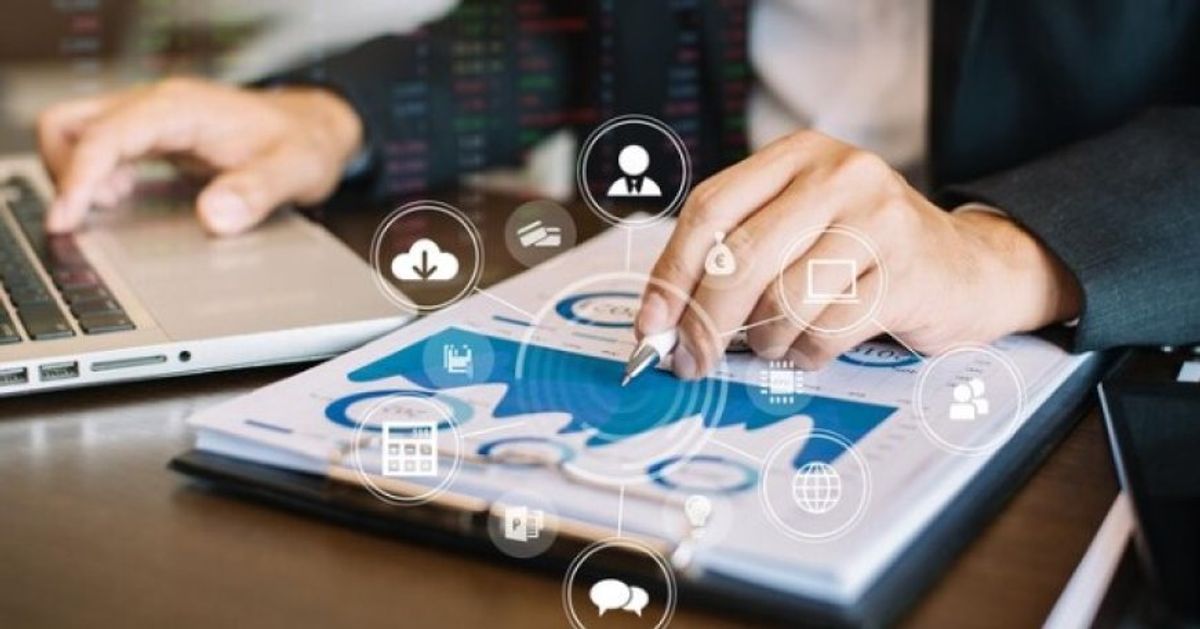
Defining Data Analytics in the Business Context
In the realm of business, data analytics is the cornerstone of informed decision-making. It is the practice of scrutinizing large volumes of data to extract patterns, correlations, and insights that are crucial for strategic planning. By leveraging analytics, companies can transition from a hunch-based approach to one that is rooted in empirical evidence.
Data analytics serves multiple purposes in a business environment:
- Understanding customer behavior: By analyzing customer data, businesses can tailor their products and services to better meet consumer needs.
- Optimizing operational efficiency: Through data analysis, companies can identify areas where they can improve processes and reduce costs.
- Predicting market trends: Data analytics enables businesses to anticipate changes in the market and adjust their strategies accordingly.
- Strategic decision-making: Armed with insights from data, businesses can make informed choices that drive growth and innovation.
Data analytics transforms raw data into strategic insights, providing a clear path from chaos to clarity in decision-making.
As the digital landscape evolves, the role of data analytics in business becomes increasingly significant. It is not just about having access to data but about harnessing the power of that data to forge a competitive edge and achieve long-term success.
The Shift from Intuition to Data-Driven Strategies
The transition from relying on intuition to embracing data-driven strategies marks a pivotal change in the business landscape. Businesses are now prioritizing data analytics to inform their strategic decisions, moving away from the uncertainty of gut feelings to the clarity of empirical evidence. This shift is not just about having access to data but about effectively interpreting and leveraging it to drive growth and success.
Data analytics plays a crucial role in providing up-to-date financial information, identifying patterns, and enabling proactive measures to mitigate risks and capitalize on opportunities. By adopting a data-centric approach, organizations can minimize risks and maximize potential for expansion.
- Strategic decision-making backed by data insights
- Enhanced business efficiency through outsourcing analytics
- Cultivating a data-driven culture for informed decision-making
Leveraging data analytics for startups is crucial for understanding customer preferences, making informed decisions, and cultivating a data-driven culture to drive growth and success.
Outsourcing Analytics for Enhanced Expertise
Outsourcing analytics is a strategic move that allows businesses to leverage external expertise for deeper insights and more informed decision-making. By partnering with analytics service providers, companies can focus on their core competencies while benefiting from the specialized skills of data scientists and analysts.
Outsourcing analytics empowers businesses to optimize performance and make strategic decisions. It provides access to a global talent pool, offering a diversity of thought that can lead to innovative solutions and a competitive edge. Moreover, it offers scalability and flexibility, allowing businesses to adjust their analytics capabilities as needed without the overhead of maintaining a large in-house team.
Prescriptive analytics and AI-powered analytics are particularly transformative when outsourced, as they require a level of expertise that may not be available internally. These advanced analytics techniques empower businesses to forecast trends and make informed decisions for strategic planning and competitive edge.
Outsourcing analytics not only enhances efficiency but also contributes to cost savings and resource optimization, making it a valuable strategy for businesses looking to stay ahead in a data-driven market.
The Analytical Approach to Decision-Making
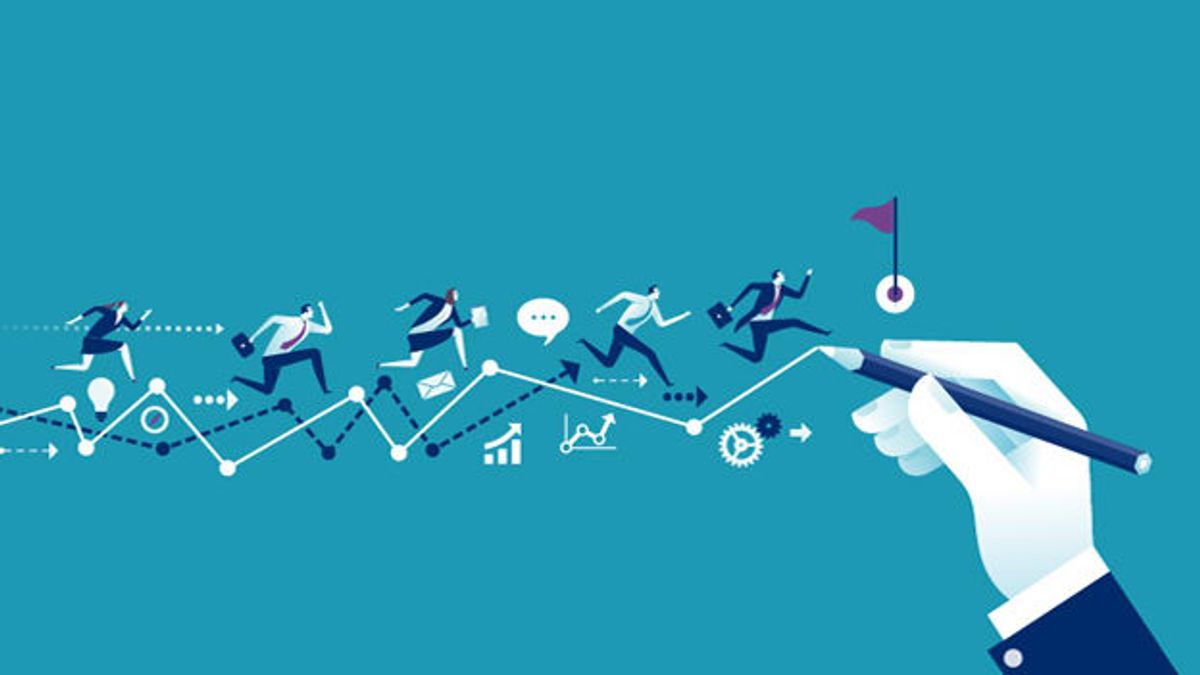
Data-Driven Insights Versus Gut Feelings
In the realm of strategic decision-making, the contrast between data-driven insights and gut feelings is stark. Data-driven insights provide a clear path through the complex maze of business challenges, much like a map in a labyrinth. Gut feelings, while valuable, can often lead to aimless wandering in the absence of data.
Analytics process transforms raw data into strategic decisions, offering a structured approach to understanding customer behavior and market trends. This methodical process is supported by tools like data visualization and AI, ensuring decisions are not just educated guesses but are aligned with organizational goals. The real-world impact of such an approach is evident in various sectors, including healthcare, where data-driven strategies have revolutionized patient care and operational efficiency.
Data allows us to dive into the heart of what drives customers, unveiling hidden motivations and identifying key moments that create lasting impressions. With this knowledge, strategies can be crafted to genuinely connect with audiences and deliver exceptional experiences.
The significance of making decisions based on data cannot be overstated. It entails utilizing both quantitative and qualitative information to guide strategic decisions, promoting corporate expansion and minimizing risks. By leveraging data, businesses can spot patterns, anticipate market movements, and take proactive measures to stay ahead of the curve.
Statistical Models and Expert Analysis in Business
In the landscape of strategic decision-making, statistical models and expert analysis play pivotal roles. Businesses use statistical modeling, regression, and machine learning for forecasting. Data quality is crucial for accurate insights and informed decisions, driving business growth.
Expertise in data analytics is not just about having the right tools; it's about interpreting the data to make strategic decisions.
The analytical approach to decision-making emphasizes the use of data-driven insights and empirical evidence. By leveraging advanced analytics, organizations can predict customer behavior, optimize supply chains, and even forecast market trends. This knowledge empowers decision-makers to steer their organizations towards better outcomes, staying competitive in the market.
Here's how businesses typically apply statistical models and expert analysis:
- Data Mining: Unearthing valuable insights from large datasets.
- Predictive Modeling: Anticipating future trends and customer behavior.
- Optimization: Enhancing operational efficiencies and supply chain management.
- Fraud Detection: Identifying and mitigating potential risks.
Objective Decision-Making with Empirical Evidence
In the realm of strategic decision-making, the transition to an analytical approach is marked by a steadfast reliance on empirical evidence. Businesses are increasingly recognizing the value of data analytics in guiding their strategic choices. Empirical research, defined as studies whose conclusions are drawn from concrete, verifiable evidence, plays a pivotal role in this process.
The analytical approach to decision-making ensures that decisions are not just educated guesses but are informed by robust data and statistical models. This method minimizes risks and paves the way for calculated, strategic growth. By leveraging empirical evidence, organizations can make objective decisions that are both defensible and forward-looking.
The shift from intuition to evidence-based decision-making is a transformative step towards a more strategic and successful business operation.
Here is a simple illustration of how empirical evidence supports decision-making:
- Identification: Recognize the decision to be made.
- Data Collection: Gather relevant data and empirical evidence.
- Analysis: Use statistical models to interpret the data.
- Decision: Make the choice based on the analysis.
- Evaluation: Assess the outcome to inform future decisions.
Types of Data Analytics and Their Impact on Business Decisions
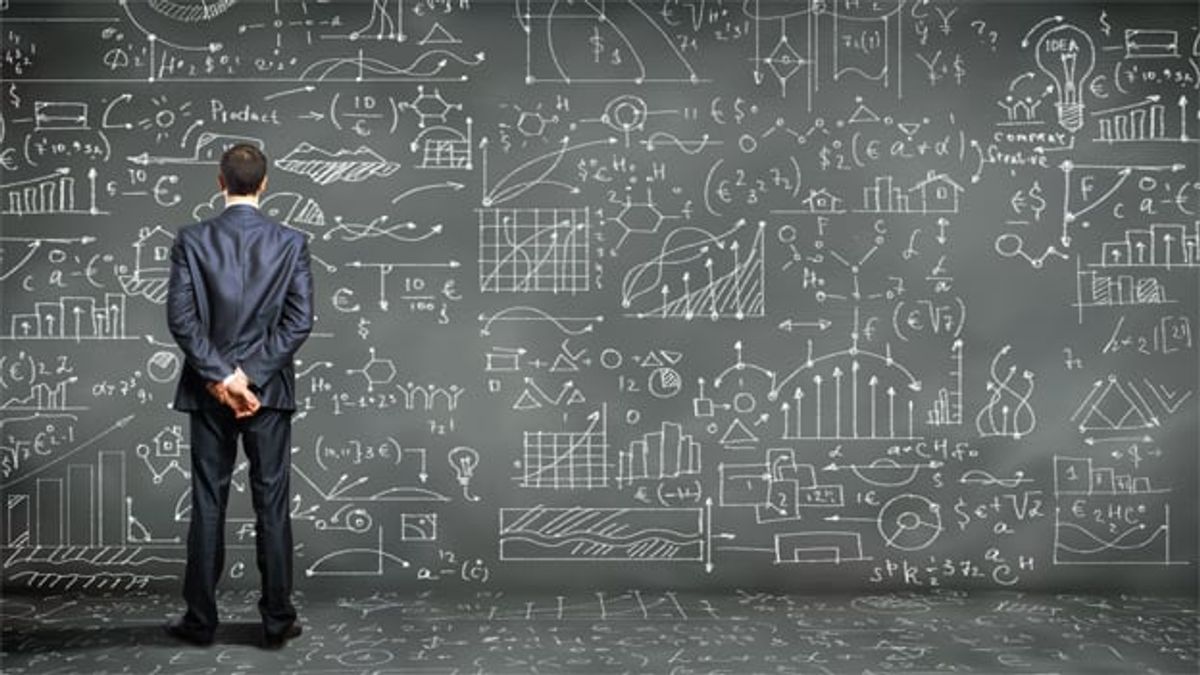
Descriptive Analytics: Understanding the Past
Descriptive analytics serves as the foundational layer of business analytics, providing a retrospective view of an organization's operations and performance. By analyzing historical data, companies can identify trends, measure performance against goals, and gain a comprehensive understanding of where they have been. This form of analytics is crucial for contextualizing current events and laying the groundwork for more advanced analytical techniques.
Descriptive analytics is the first step in the data analytics journey, offering a snapshot of the past that informs future strategies. It typically involves the use of dashboards and reports that summarize data into actionable insights. For instance, a sales dashboard might include the following key metrics:
Metric | Q1 2020 | Q2 2020 | Q3 2020 | Q4 2020 |
---|---|---|---|---|
Total Sales Revenue | $1.2M | $1.5M | $1.3M | $1.7M |
Number of Units Sold | 10,000 | 12,500 | 11,000 | 14,000 |
Average Price per Unit | $120 | $120 | $118 | $121 |
The evolution from descriptive to prescriptive analytics empowers businesses to not only understand their history but also to shape their future. Predictive analytics forecasts future trends, enhancing decision-making, while real-time data analysis transforms the business analytics landscape.
By establishing a solid foundation with descriptive analytics, organizations can confidently navigate through the subsequent stages of the analytics process, ultimately leading to more informed and strategic decisions.
Diagnostic Analytics: Explaining the Why
Diagnostic analytics takes the insights gained from descriptive analytics and delves deeper to answer the question of why certain events occurred. This form of analytics is crucial for organizations that want to understand the underlying factors and causes behind observed trends and anomalies. By identifying the root causes of issues, companies can develop targeted strategies to address them effectively.
- Identification of trends and anomalies
- Root-cause analysis
- Development of targeted strategies
Diagnostic analytics empowers businesses to move beyond simple observations to gain a more comprehensive understanding of their data.
The process often involves techniques such as drill-down, data discovery, data mining, and correlations. Businesses can leverage diagnostic analytics to perform a thorough analysis, which is essential for informed decision-making and strategic planning.
Predictive Analytics: Anticipating the Future
Predictive analytics serves as a crystal ball for businesses, offering a glimpse into the future actions of customers. By sifting through historical data and discerning patterns, these advanced algorithms empower companies to foresee and influence customer behavior. Armed with predictive insights, businesses can transition from a reactive to a proactive stance, ensuring they are always a step ahead in delivering personalized experiences.
Purchase intent is a prime example of predictive analytics at work. Consider the following table illustrating the impact of predictive analytics on marketing strategies:
Customer Segment | Predicted Purchase Intent | Marketing Action |
---|---|---|
Segment A | High | Personalized Offer |
Segment B | Medium | Targeted Email Campaign |
Segment C | Low | General Newsletter |
By anticipating when customers are most likely to make a purchase, businesses can craft targeted offers and recommendations that resonate at the perfect moment, enhancing conversion rates and fostering revenue growth.
Predictive analytics not only anticipates individual customer actions but also identifies broader market trends. This allows businesses to strategize proactively, seizing opportunities and staying competitive in a rapidly evolving marketplace.
Prescriptive Analytics: Recommending Actions
Prescriptive analytics represents the pinnacle of data-driven decision-making, providing not just insights but actionable recommendations. By leveraging advanced algorithms and machine learning, businesses can receive specific guidance on the best course of action to achieve their goals. Prescriptive analytics and AI integration in business analytics offer actionable insights, optimize decision-making, and shape the future of data-driven strategies with advanced tools and techniques.
Prescriptive analytics goes beyond predicting what might happen to suggesting a range of prescribed actions and the potential outcomes of each. It's a proactive tool that empowers decision-makers to not only anticipate the future but to shape it.
For instance, in marketing, prescriptive analytics can analyze customer data to recommend personalized promotions that are most likely to result in a conversion. In supply chain management, it can suggest the most efficient distribution routes or inventory levels. The applications are vast and varied, but the goal is the same: to provide optimal solutions that drive business success.
Here are some key benefits of prescriptive analytics:
- Tailored strategies for customer engagement
- Improved operational efficiency
- Enhanced risk management
- Increased profitability through optimized decision-making
Optimizing Operations with Data Analytics
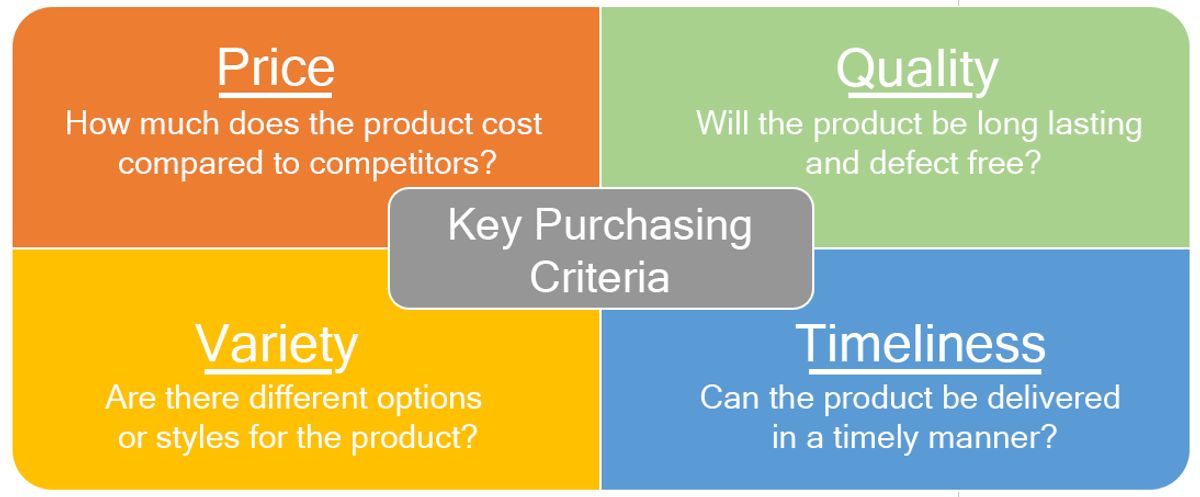
Improving Efficiency and Reducing Costs
In the realm of strategic decision-making, data analytics serves as a catalyst for operational efficiency and cost reduction. By dissecting complex data sets, businesses can pinpoint inefficiencies and streamline their processes, leading to significant savings and heightened productivity.
- Data-driven insights allow for the optimization of resources, from manpower to material costs, ensuring that every aspect of the business is running at peak efficiency. For instance, logistics companies have transformed their operations by utilizing analytics to refine delivery routes, thereby minimizing fuel expenses and improving delivery times.
By embracing analytics, organizations can not only enhance their operational workflows but also gain a competitive edge by reducing overheads and boosting their bottom line.
Here's how analytics can impact various business areas:
- Human Resources: Identifying patterns in employee performance and turnover to improve hiring and retention strategies.
- Supply Chain Management: Streamlining inventory levels to reduce waste and avoid stockouts.
- Customer Service: Analyzing customer feedback to improve service quality and response times.
The integration of analytics into business operations is not just about cutting costs—it's about smartly reallocating resources to where they can generate the most value.
Enhancing Customer Experiences through Insights
Unlocking the power of data and analytics provides an unparalleled insight into customer behavior, preferences, and needs. By identifying key touchpoints and pain points through data analysis, businesses can proactively streamline processes and elevate the overall customer journey. This approach not only enhances customer satisfaction but also fosters loyalty and retention.
Data-driven personalization is akin to a salesperson greeting you by name, aware of your preferences, and ready to recommend products you'll love. It's the magic of leveraging customer data to create experiences that make customers feel truly valued and understood.
By harnessing the abundance of data at our disposal, we can anticipate desires, tailor messaging, and curate experiences that resonate with our customers at every stage of their journey.
Businesses must address data challenges, upgrade technology, enhance data privacy, and invest in skilled professionals to leverage insights. Understanding the analytics process and extracting value from data are crucial for informed decision-making.
Anticipating Market Trends to Stay Competitive
In the dynamic arena of business, anticipating market trends is not just an advantage; it's a necessity for survival. Data analytics serves as the compass that guides companies through the murky waters of market fluctuations. By harnessing the power of historical data and external factors, such as economic indicators and consumer behavior, businesses can forecast with greater accuracy and develop proactive strategies.
Analytics is pivotal in strategic planning, enabling organizations to adapt swiftly to market trends, competitor activities, and consumer sentiment. This agility minimizes risks and carves out opportunities for innovation and growth. For example, an e-commerce company might use data analytics to pinpoint emerging trends and craft marketing campaigns tailored to specific customer segments.
The essence of staying competitive lies in transforming data into actionable insights. It's about making informed decisions that not only respond to current market dynamics but also shape future outcomes.
Social media monitoring is another tool that sharpens a company's competitive edge. It allows for the detection of new trends and preferences, informing marketing strategies and ensuring relevance in a fast-paced digital world.
Data Analytics as a Competitive Advantage
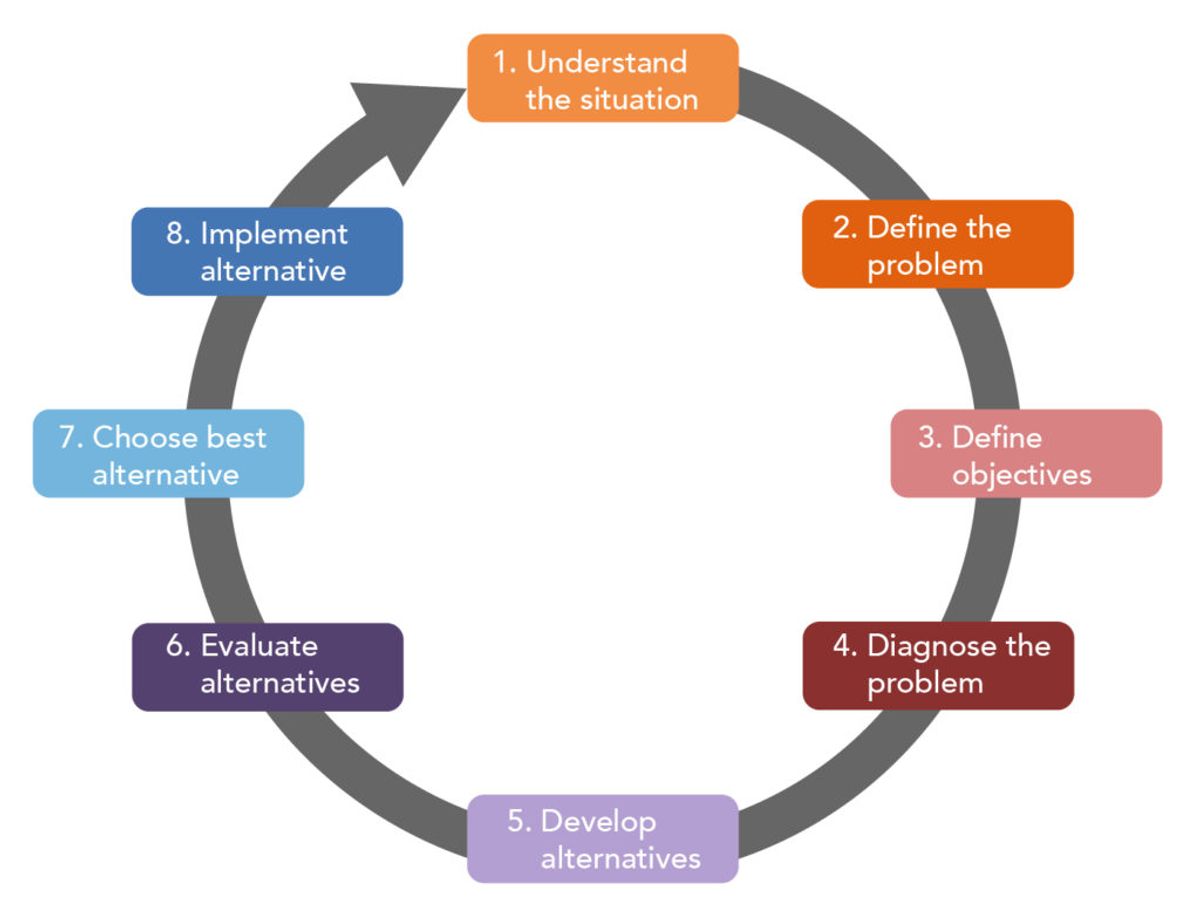
Gaining an Edge in a Data-Driven Market
In the relentless pursuit of market leadership, data analytics serves as a critical tool for businesses to gain a competitive edge. By harnessing the power of data, companies can not only react to market changes but also proactively shape their strategies to stay ahead of the curve.
- Enhanced Decision-Making: Real-time data access enables swift, informed decision-making.
- Personalized Customer Experiences: Deep insights into customer preferences lead to tailored experiences.
- Operational Efficiency: Predictive analytics and automation streamline operations and reduce costs.
Data analytics is not just a pathway to success; it's a strategic imperative in a data-driven market. It empowers businesses to make informed decisions, optimize operations, and anticipate market trends.
Embracing data analytics allows organizations to not only interpret complex market dynamics but also to innovate and adapt with agility. The companies that excel in decoding data's hidden insights are often the ones rewriting the rules of competition and achieving sustainable growth.
Strategizing with Data: Innovating for Success
In the realm of strategic business decision-making, data analytics is the cornerstone of innovation. It empowers organizations to not only react to current trends but also to shape future market movements. By harnessing the power of big data, companies can craft innovative business models that disrupt traditional markets and create new value propositions.
- Innovative Business Models: Analyze diverse data types to identify unmet needs.
- Enhanced Analytics Capabilities: Leverage advanced tools for deeper insights.
- Robust Data Strategies: Align analytics with overarching business goals.
Data analytics drives competitiveness, enhances decision-making, and fosters long-term success by leveraging insights, strategic investments, and a data-driven culture for sustainable growth and innovation.
The strategic use of data analytics goes beyond mere number-crunching; it involves a comprehensive understanding of market dynamics, consumer behavior, and technological advancements. Businesses that excel in decoding the hidden insights within data can adapt their strategies to minimize risks and seize new opportunities for growth.
Benchmarking and Performance Tracking
In the realm of strategic decision-making, benchmarking and performance tracking are indispensable tools for organizations aiming to secure a competitive advantage. By systematically comparing business processes and performance metrics against industry best practices, companies can identify areas of improvement and optimize their strategies to achieve even better results.
Key Performance Indicators (KPIs) serve as the compass that guides businesses towards their goals, providing a clear view of progress and the efficacy of their strategies. For instance, using platforms like Xero Analytics, businesses can track sales growth, inventory turnover, and customer acquisition costs in real-time. This enables them to set benchmarks, monitor advancements, and adjust their data-driven strategies accordingly.
Data-driven leadership not only enhances strategic success but also plays a crucial role in boosting employee engagement, retention, and recruitment.
Remember, every experiment is an opportunity to learn and iterate, fostering a culture of continuous improvement and growth. Embracing A/B testing and experimentation allows for informed decisions that optimize customer experiences and keep businesses ahead in a dynamic market.
Implementing a Data Analytics Framework
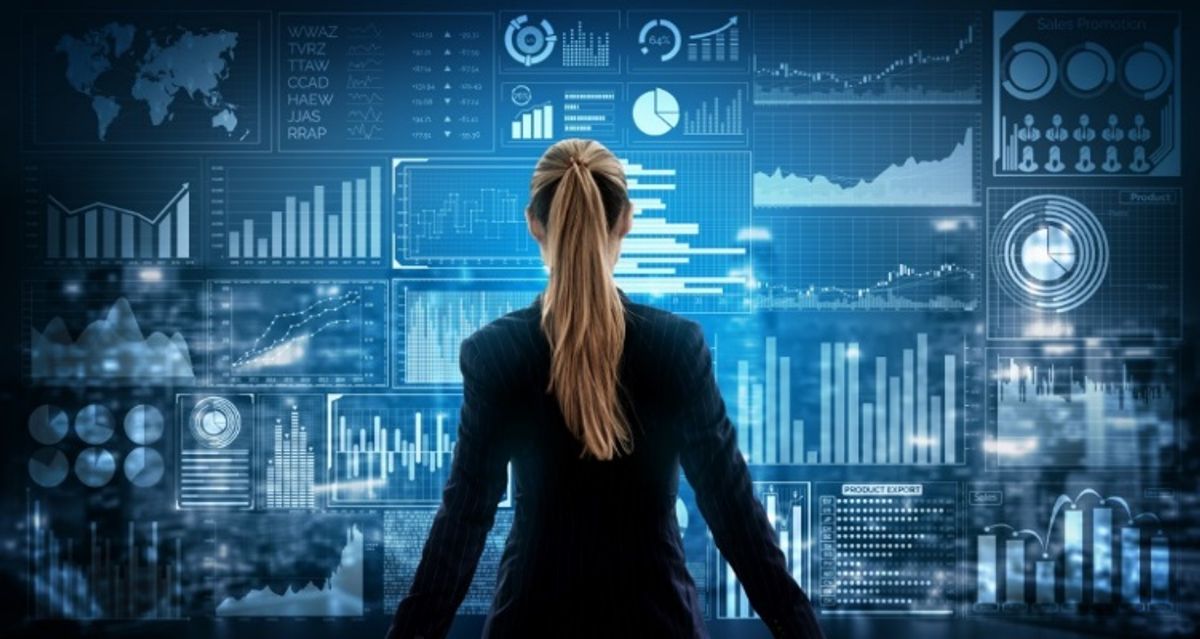
Building the Analytics Team: In-house Versus Outsourcing
When it comes to building an analytics team, organizations face a strategic decision: develop in-house expertise or outsource to specialized service providers. In-house development allows for greater control over the analytics process and a deeper integration with the company's culture and goals. However, it requires significant investment in recruitment, training, and technology.
On the other hand, outsourcing offers access to a broader range of expertise and can be more cost-effective. It enables businesses to leverage the knowledge of seasoned professionals who are adept at navigating complex data landscapes. This approach can drive business efficiency, as external teams are often more agile and can scale quickly to meet changing demands.
The choice between in-house and outsourcing should align with the company's long-term strategic objectives and operational capabilities.
Here are some considerations to weigh when deciding between in-house and outsourcing analytics:
- Expertise: In-house teams may require time to develop, whereas outsourcing provides immediate access to expert knowledge.
- Cost: Initial costs for setting up an in-house team can be high, but outsourcing may lead to ongoing expenses.
- Control: Maintaining in-house analytics ensures direct oversight, while outsourcing may reduce hands-on management.
- Scalability: Outsourcing allows for flexible scaling of resources, a crucial factor during periods of rapid growth or change.
Developing a Data-Driven Culture in the Organization
Fostering a data-driven culture is pivotal for organizations aiming to thrive in the modern business landscape. It requires a strategic alignment with business objectives and a commitment to building data literacy across all levels of the organization. Overcoming cultural barriers and ensuring data quality are essential steps in this transformative journey.
- Align organizational goals with data initiatives
- Educate employees on the importance and use of data
- Promote transparency and open access to data
- Encourage experimentation and learning from data
By embedding data into the organizational DNA, companies can make more informed decisions, innovate faster, and maintain a competitive edge.
The path to a data-driven culture is not without its challenges, but the rewards are substantial. It leads to a more agile and responsive organization, capable of adapting to market changes and customer needs with unprecedented speed and accuracy.
Choosing the Right Tools and Technologies
Selecting the appropriate tools and technologies is crucial for implementing a successful data analytics framework. The right set of tools can empower your team to efficiently analyze data and extract actionable insights. For instance, front-end technologies like Angular, ReactJS, and Vue.js enable the creation of dynamic user interfaces for data visualization, while back-end technologies such as Ruby on Rails, Node.js, and Python provide the robustness needed for data processing.
Front-End Technologies
- Angular: Level up your development with Angular expertise
- ReactJS: Transform your web development with ReactJS experts
- Vue.js: Delivering agile and cutting-edge solutions
Back-End Technologies
- Ruby on Rails: Build robust web applications
- Node.js: Optimize your backend with Node.js specialists
- Python: Drive innovation and automation
It's essential to choose technologies that not only align with your business needs but also integrate seamlessly with your existing systems. The goal is to create a harmonious ecosystem that facilitates data-driven decision-making and supports your strategic objectives.
Moreover, the landscape of data analytics tools is constantly evolving. Partnering with experts who have a deep understanding of data mining, predictive modeling, and the latest advancements ensures that your organization stays ahead of the curve. This expertise is a key factor in overcoming challenges and setting measurable goals for future planning.
Challenges and Solutions in Data Analytics
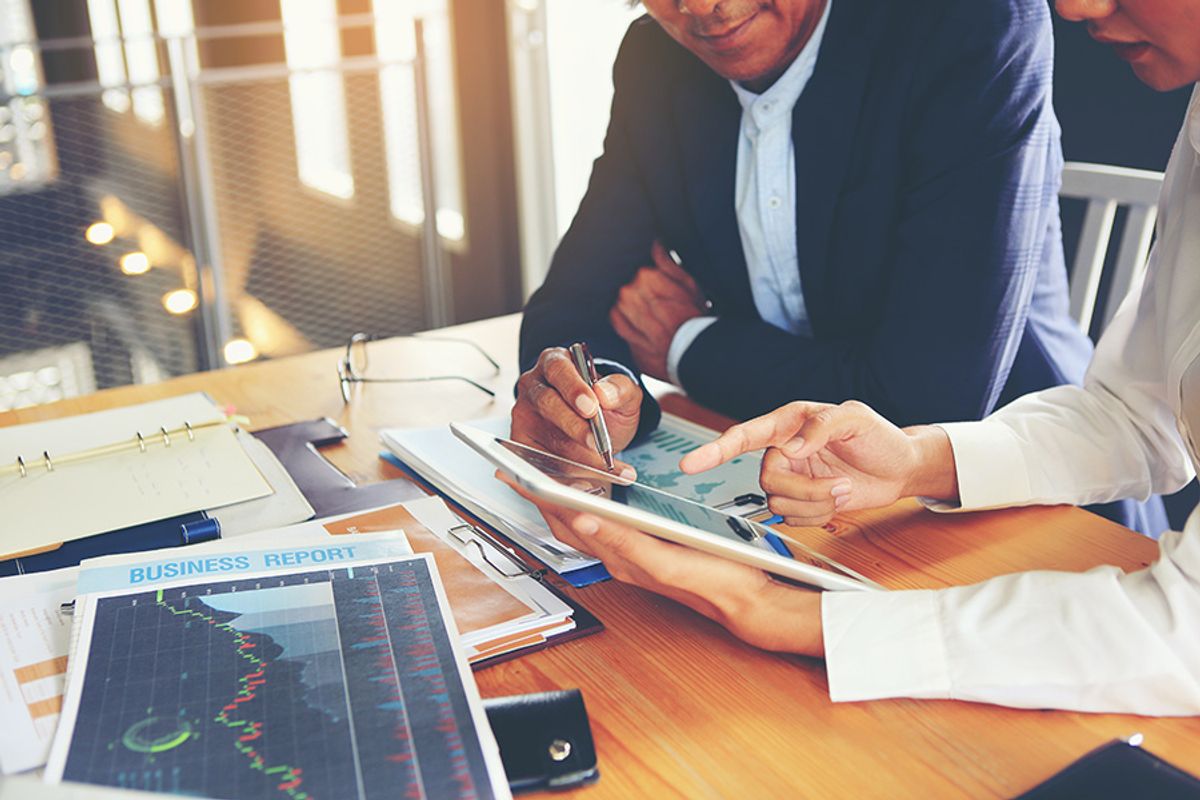
Data Quality and Integrity Issues
In the realm of strategic decision-making, data quality is paramount. Data quality is a measure of a data set's condition based on factors such as accuracy, completeness, consistency, reliability, and validity. Without high-quality data, businesses risk making decisions based on flawed insights.
Clean and reliable data forms the basis for sound decision-making, as erroneous or incomplete information can lead to misguided judgments.
Big data management plays a crucial role in enhancing data quality and integrity. It involves processes such as data cleansing, standardization, and validation, which are essential to minimize errors and inconsistencies. Here's how businesses can address data quality issues:
- Implementing sophisticated algorithms for data cleansing
- Conducting regular data quality assurance checks
- Utilizing data validation techniques to ensure accuracy
- Addressing the challenges of parsing semi-structured data for valuable insights
By prioritizing data integrity, organizations can avoid the pitfalls of unreliable data and establish a strong foundation for analytics-driven strategies.
Overcoming Analysis Paralysis
Analysis paralysis occurs when the abundance of data and choices leads to a state of overthinking, making it difficult to make timely decisions. To overcome this, organizations must prioritize actionable insights over exhaustive analysis.
Prioritization is key in avoiding analysis paralysis. By focusing on the most impactful data and making incremental decisions, businesses can maintain momentum. Here's a simple approach:
- Identify critical data points that influence key outcomes.
- Set thresholds for decision-making to avoid excessive deliberation.
- Encourage a culture of 'good enough' to foster faster decision cycles.
Emphasizing speed and agility in decision-making processes can help organizations navigate through the noise of data and focus on what truly matters.
Remember, the goal is not to eliminate all uncertainty but to make well-informed decisions that propel the business forward. By establishing clear criteria and empowering teams to act, companies can sidestep the trap of analysis paralysis and maintain a competitive edge.
Ensuring Privacy and Compliance in Data Usage
In the era of big data, ensuring privacy and compliance in data usage is not just a legal obligation but a cornerstone of consumer trust. As organizations navigate through the complexities of data management, they must address privacy problems and adhere to stringent regulations to avoid policy violations and breaches.
- Conduct regular audits to identify and address privacy issues.
- Implement robust data management systems for secure data storage and processing.
- Employ sophisticated algorithms and data validation techniques to maintain data integrity.
- Stay updated with the latest compliance requirements and adjust policies accordingly.
By proactively managing privacy and compliance, businesses can mitigate risks and maintain the integrity of their decision-making processes.
The journey to compliance is ongoing, with new challenges such as the handling of dark data—information passively collected and often overlooked. Illuminating and harnessing this data responsibly can unlock new opportunities for growth and innovation, provided it is done within the bounds of privacy laws and ethical standards.
Case Studies: Success Stories of Analytics-Driven Decision-Making
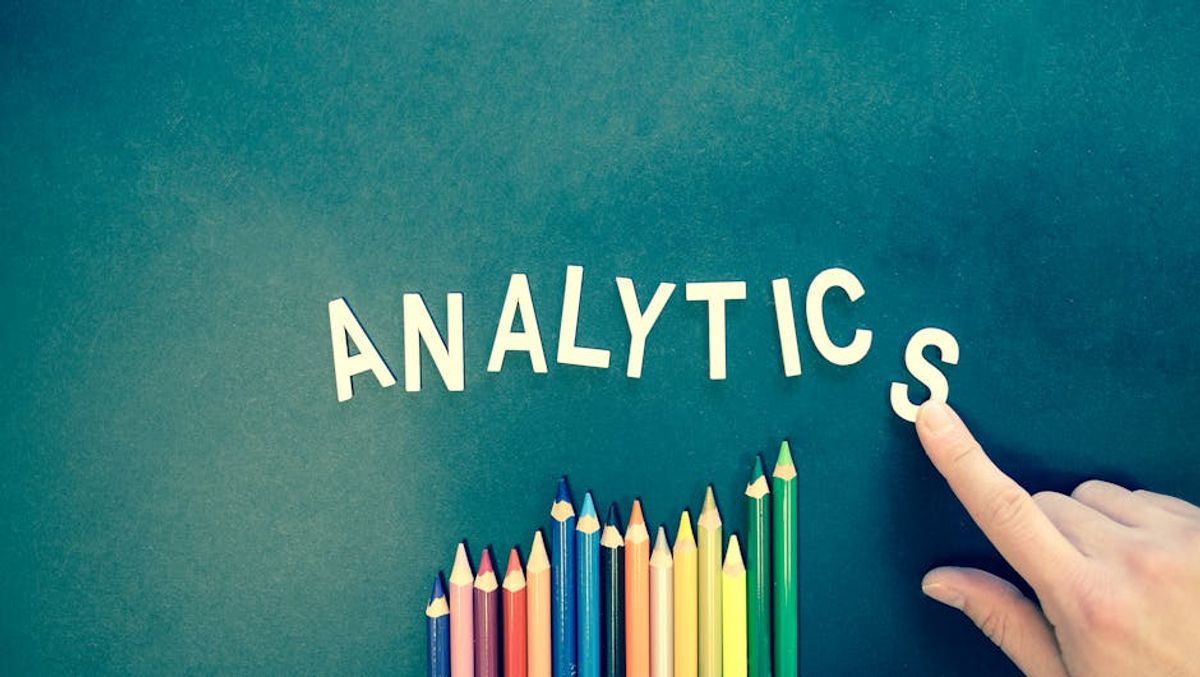
Transforming Industries with Strategic Insights
The integration of analytics into strategic planning is not just a trend; it's a proven method for enhancing business performance. Integrating analytics into strategic planning boosts revenue, with key steps including the establishment of unified data platforms, the adoption of real-time processing, and the promotion of shared insights across departments. However, businesses often encounter challenges such as data silos, integration issues, and talent shortages.
Analytics has become the cornerstone of industry transformation, enabling companies to decipher trends, sentiments, and patterns that are invaluable for strategic decision-making. The deployment of the right technologies is crucial to mine this vast, untapped resource efficiently.
The success stories of analytics-driven decision-making are numerous, yet they also highlight the challenges faced in the journey towards data-driven decisions. Each case study provides a blueprint for overcoming obstacles and leveraging data for strategic advantage.
In conclusion, the power of data analytics lies in its ability to turn information into actionable insights, driving efficiency and strategic foresight. As we continue to witness the reshaping of business strategies through data, it becomes clear that the path to informed decision-making is paved with data.
Small Businesses Leveraging Big Data
The notion that big data is solely the domain of large corporations is a myth. Small businesses are increasingly harnessing big data to carve out a competitive edge in their respective markets. With the advent of affordable analytics tools and technologies, these nimble entities can now tap into the same rich vein of data insights that power the giants of industry.
Innovative business models are emerging as small businesses analyze diverse data types to uncover unmet needs and create disruptive solutions. This strategic use of data is not just about managing numbers; it's about driving growth and innovation.
- Real-time data integration
- Seamless analysis
- Swift decision-making
By embracing big data, small businesses are not only competing more effectively but are also setting new standards in responsiveness and customer engagement. The Decision Hub model, as leveraged by Walmart, exemplifies the transformative potential of data-driven strategies.
The vast quantities of data generated daily represent a goldmine for those willing to invest in big data capabilities. It's a game-changer that levels the playing field, allowing smaller players to make informed decisions that were once the privilege of their larger counterparts.
Innovative Approaches to Complex Problem-Solving
In the realm of complex problem-solving, innovation is key. Companies are increasingly turning to semi-structured data, which serves as a bridge between the highly organized world of structured data and the more elusive, unstructured data. The ability to harness this type of data can lead to groundbreaking insights and solutions.
Embracing a global perspective in data analysis is crucial. By outsourcing analytics, businesses gain access to a diverse pool of talent, which can offer unique perspectives and enhance the richness of the analysis. This global collaboration can be a catalyst for innovative problem-solving strategies.
The analytical approach to decision-making is at the heart of these innovative strategies. It relies on data-driven insights and empirical evidence, ensuring decisions are not just made, but crafted with precision and foresight. As we look to the future, the integration of big data into business strategies will become increasingly important, with organizations that leverage this asset well positioned to lead in the digital revolution.
The challenge lies in accurately parsing and interpreting semi-structured data to extract valuable insights. This is where the true power of analytics is realized, transforming raw data into strategic knowledge.
The Future of Decision-Making with Evolving Data Analytics
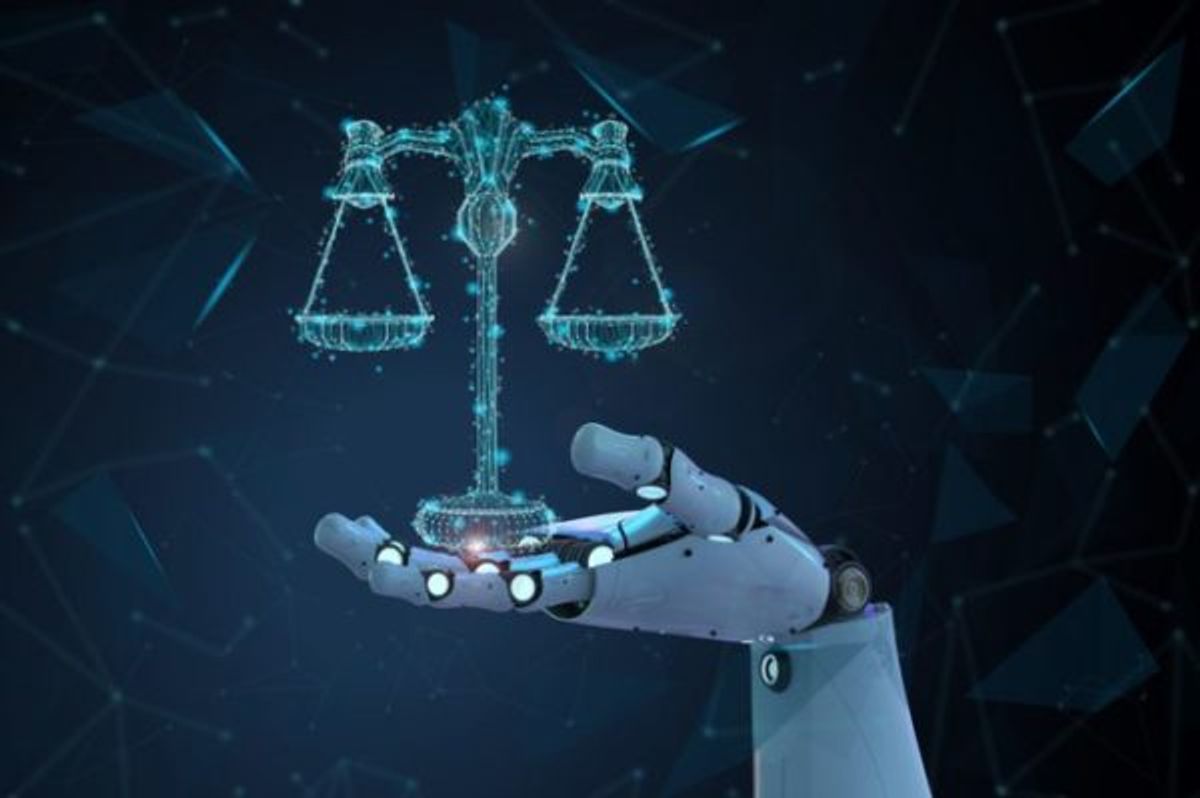
The Rise of Artificial Intelligence and Machine Learning
The integration of Artificial Intelligence (AI) and Machine Learning (ML) into data analytics is revolutionizing how businesses approach decision-making. AI not only automates and predicts but also significantly enhances the decision-making process. By providing deeper insights and enabling the processing of vast datasets, particularly unstructured and semi-structured data, AI is transforming the landscape of strategic business decisions.
Machine Learning, a subset of AI, is particularly adept at identifying patterns and making predictions based on historical data. This capability allows businesses to anticipate market trends, optimize operations, and personalize customer experiences. The following list outlines the key applications of AI and ML in data analytics:
- Predictive analytics for customer behavior
- Supply chain optimization
- Fraud detection
- Market trend forecasting
The role of AI in handling big data is pivotal, enhancing the accuracy of insights and automating complex analytical processes. It is not just about the volume of data but the ability to turn it into actionable business insights.
As the technology continues to evolve, the potential for AI and ML to drive strategic decision-making grows. Companies that leverage these technologies can gain a significant competitive advantage, staying ahead of market dynamics and innovating for success.
Predictive Analytics and Real-Time Decision-Making
The advent of predictive analytics has revolutionized the way businesses approach decision-making. By harnessing the power of historical data, companies can now anticipate future events with remarkable accuracy. Real-time data analysis further enhances this capability, allowing for swift adjustments to strategies and operations.
- Enhanced Decision-Making: Access to real-time data empowers businesses to make informed decisions, reducing risks and capitalizing on opportunities swiftly.
- Personalized Customer Experiences: Big data enables businesses to understand customer preferences deeply, delivering tailored experiences that foster loyalty and engagement.
- Operational Efficiency: Through predictive analytics and automation, organizations can streamline operations, cut costs, and improve overall efficiency.
Predictive analytics acts as a crystal ball, offering insights into customer behavior and future market trends. This foresight is invaluable for businesses aiming to stay competitive and responsive to the ever-changing market dynamics.
One of the most significant applications of predictive analytics is in anticipating purchase intent. By identifying patterns in historical data, businesses can predict when customers are most likely to make a purchase, allowing for targeted marketing efforts that increase conversion rates and drive revenue growth.
Ethical Considerations in Automated Decision-Making
As businesses increasingly integrate AI and data analytics into their decision-making processes, ethical considerations become paramount. Prioritizing transparency, fairness, and accountability is essential to foster trust among stakeholders and ensure adherence to regulatory standards. Ethical frameworks guide the deployment of automated systems, ensuring they operate within the bounds of societal norms and values.
The implementation of ethical practices in analytics is not just a legal imperative but a strategic one as well. It strengthens the bond between businesses and their customers, and it is a critical component of sustainable business models.
While the benefits of automated decision-making are vast, they come with a responsibility to address potential biases and privacy concerns. A structured approach to ethics in analytics includes:
- Establishing clear guidelines for data usage
- Ensuring the explainability of algorithmic decisions
- Regularly auditing systems for discriminatory patterns
- Engaging with diverse stakeholders to assess the impact of decisions
As the landscape of data analytics continues to evolve, the ability to make informed decisions swiftly is more crucial than ever. At OptimizDBA, we pride ourselves on delivering faster data solutions that revolutionize decision-making processes. Our proprietary methodology has been trusted by over 500 clients since 2001, ensuring performance increases that are not just twice, but often 100 to 1000 times faster. Don't let outdated analytics slow you down. Visit our website to learn how we can optimize your database performance and empower your decision-making with cutting-edge analytics.
Conclusion
In the journey through the transformative landscape of data analytics, we have explored its pivotal role in reshaping business strategies and decision-making processes. From the initial understanding of leveraging data analytics to the intricate details of the four types of analytics, this comprehensive guide has aimed to equip readers with the knowledge to harness the power of data for strategic advantage. As we conclude, it is clear that the ability to interpret and act upon data-driven insights is not just a competitive edge but a necessity in the digital era. Businesses that effectively outsource and integrate analytics into their operations can anticipate trends, optimize performance, and make informed decisions that drive success. Embrace the analytical approach to decision-making, and let the clarity derived from data analytics illuminate the path to your business's sustainable growth and prosperity.
Frequently Asked Questions
What is leveraging data analytics in business?
Leveraging data analytics in business refers to the strategic use of data to gain insights that inform decision-making processes. It often involves outsourcing to specialists who can maximize the potential of data for strategic planning and operational efficiency.
How does the analytical approach to decision-making differ from intuition-based strategies?
The analytical approach to decision-making relies on data-driven insights, statistical models, and empirical evidence, rather than on intuition or gut feelings. It ensures a more objective and strategic process, leading to informed and effective business decisions.
What are the benefits of outsourcing analytics?
Outsourcing analytics offers businesses access to specialized expertise and advanced analytical tools, which can enhance the quality of insights derived from data. This can lead to better strategic decisions and improved operational efficiency.
What are the four main types of data analytics?
The four main types of data analytics are descriptive analytics (understanding the past), diagnostic analytics (explaining the why), predictive analytics (anticipating the future), and prescriptive analytics (recommending actions).
How can data analytics optimize business operations?
Data analytics can optimize business operations by uncovering inefficiencies, enhancing customer experiences, and anticipating market trends. This allows businesses to strategize and innovate effectively, staying competitive in a dynamic market.
What does building a data-driven culture in an organization entail?
Building a data-driven culture involves fostering an organizational mindset that values data as a critical asset for decision-making. It includes training employees to use data analytics tools, encouraging data-informed strategies, and promoting a culture of continuous learning and improvement.
What are some common challenges in data analytics and how can they be addressed?
Common challenges in data analytics include ensuring data quality and integrity, avoiding analysis paralysis, and maintaining privacy and compliance. Solutions involve implementing robust data governance practices, focusing on actionable insights, and adhering to relevant regulations.
How is the future of decision-making evolving with advancements in data analytics?
The future of decision-making is evolving towards more real-time, predictive, and automated processes, thanks to advancements in artificial intelligence and machine learning. Ethical considerations will also play a significant role in guiding the use of automated decision-making systems.