The Intersection of Data Science and Business Analytics
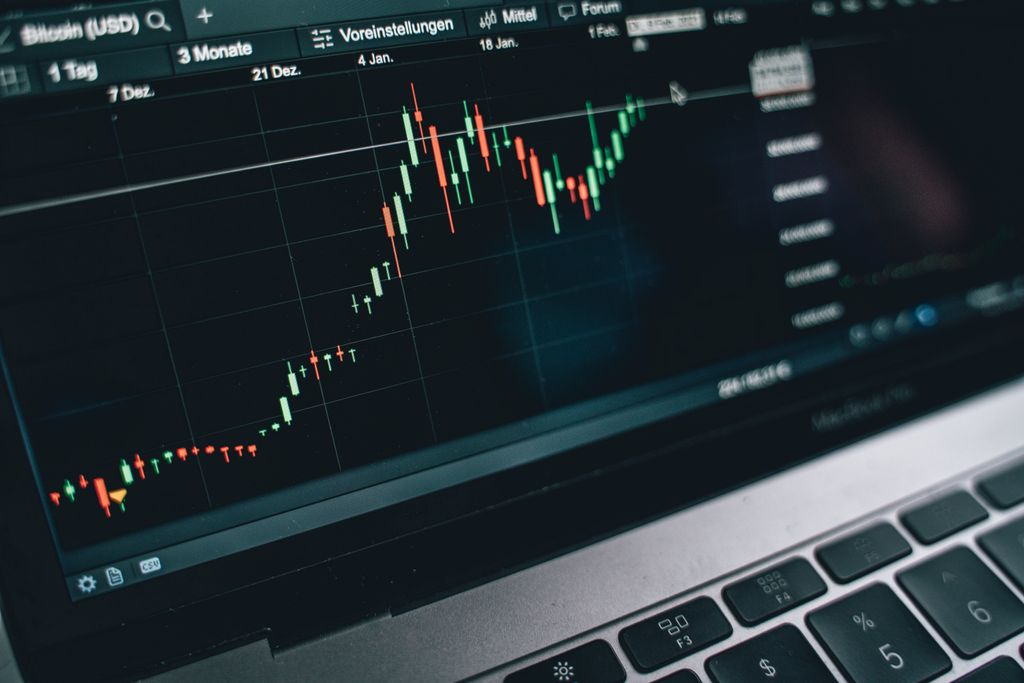
Amplifying Business Analytics with Data Science
The synergy between data science and business analytics is transforming the landscape of strategic decision-making. Data science serves as a catalyst, enhancing the capabilities of business analytics by introducing advanced algorithms and machine learning techniques. This fusion not only accelerates the extraction of insights from raw data but also empowers organizations to predict trends and optimize operations.
Data science is not without its challenges, yet its integration into business analytics is a game-changer. The table below highlights the key areas where data science amplifies business analytics:
Area of Impact | Description |
---|---|
Predictive Analytics | Utilizing historical data to forecast future outcomes. |
Customer Insights | Gaining a deeper understanding of customer behavior and preferences. |
Operational Efficiency | Streamlining processes to enhance productivity and reduce costs. |
Risk Management | Identifying and mitigating potential risks before they materialize. |
By embedding data science into business analytics, companies can unlock a new realm of possibilities, driving innovation and maintaining a competitive edge in their respective markets.
Challenges in Implementing Data Science
The journey to integrate data science into business analytics is fraught with obstacles. The scarcity of skilled data scientists stands as a formidable barrier, with businesses grappling to hire experts capable of deciphering complex datasets. A potential remedy lies in the realm of AI services, which promise to automate aspects of data analysis and lessen the reliance on human expertise.
Another hurdle is the seamless integration of data science within established business workflows. A strategic, phased approach can ease this transition, allowing for the gradual infusion of data science methodologies across various business sectors. AI tools also play a pivotal role in facilitating this process, making the integration more efficient.
Data privacy remains a pressing concern, necessitating strict adherence to data protection laws. Employing robust security protocols and leveraging AI for secure cloud services are essential steps in safeguarding sensitive information.
Looking ahead, the promise of data science in business analytics shines bright. As we advance, the synergy between data science and business intelligence tools will undoubtedly propel organizations towards a data-driven future.
Benefits of Data Science Integration
Integrating data science into business analytics reaps numerous benefits, including better decision-making and enhanced performance measurement. By translating complex data into actionable insights, businesses can operate more efficiently and stay ahead of the competition.
One of the key advantages is the ability to identify and prevent risks, which is crucial for maintaining a robust business strategy. Data scientists play a vital role in this process, employing a diverse skill set from statistical analysis to machine learning.
The integration of data science techniques into business operations paves the way for innovative solutions and strategies that drive business growth.
Despite the challenges, the future of data science in business analytics looks promising, with AI and machine learning paving the way for more sophisticated analysis and improved business performance.
Business Intelligence Dashboard
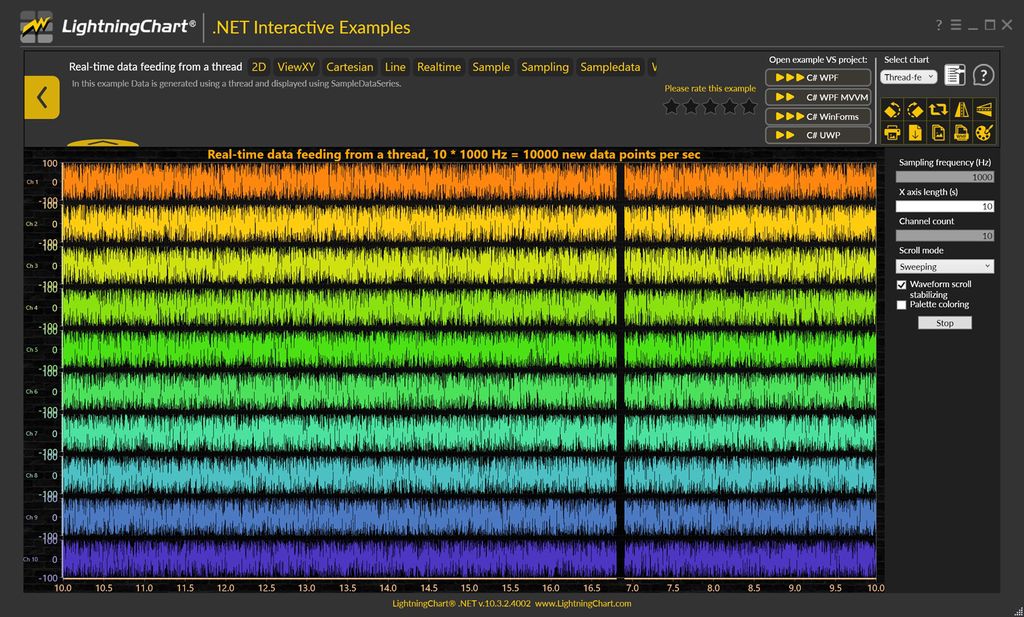
Key Features of BI Dashboards
Business Intelligence (BI) dashboards are pivotal in synthesizing complex data into actionable insights. Quick insights and real-time performance monitoring are at the heart of their functionality, enabling strategic decision-making and fostering cost-saving measures. A well-designed BI dashboard is characterized by its data visualization capabilities, offering charts and graphs that contextualize data across various dimensions.
- Interactive charts allow users to delve deeper into the data by hovering, clicking, and expanding visual elements.
- Widgets serve as pre-made functions that streamline the creation of each dashboard module.
With a customizable dashboard, decision-makers gain an instant insight-generation tool, simplifying complex data and focusing on relevant metrics.
Implementing an effective BI dashboard requires careful consideration of these features to ensure that it aligns with the strategic goals of the organization. The integration of interactive elements and widgets not only saves time but also enhances the user experience, making data analysis more intuitive and efficient.
Implementing BI Dashboards Effectively
To harness the full potential of Business Intelligence (BI) dashboards, effective implementation is crucial. Data integration and preparation are foundational steps, ensuring that the data feeding into your dashboards is accurate, consistent, and clean. This process mitigates the risk of corrupted data leading to misleading analyses.
Data-driven decision making is at the heart of BI dashboard utility. By providing real-time or near-time insights through well-designed visualizations, stakeholders can make informed decisions swiftly. However, it's important to avoid overly complex or busy designs that can obfuscate key information.
Effective BI dashboard implementation requires a balance between comprehensive data representation and clear, actionable visualizations.
Here are some considerations for implementing BI dashboards effectively:
- Ensure data quality through rigorous cleaning and validation processes.
- Design with the end-user in mind, focusing on usability and relevance.
- Prioritize key performance indicators (KPIs) to provide context and focus.
- Regularly review and update the dashboard to reflect changing business needs.
Remember, the goal is to create a tool that enhances strategic decision-making and performance monitoring without becoming a source of confusion.
Data Science in Business Analytics
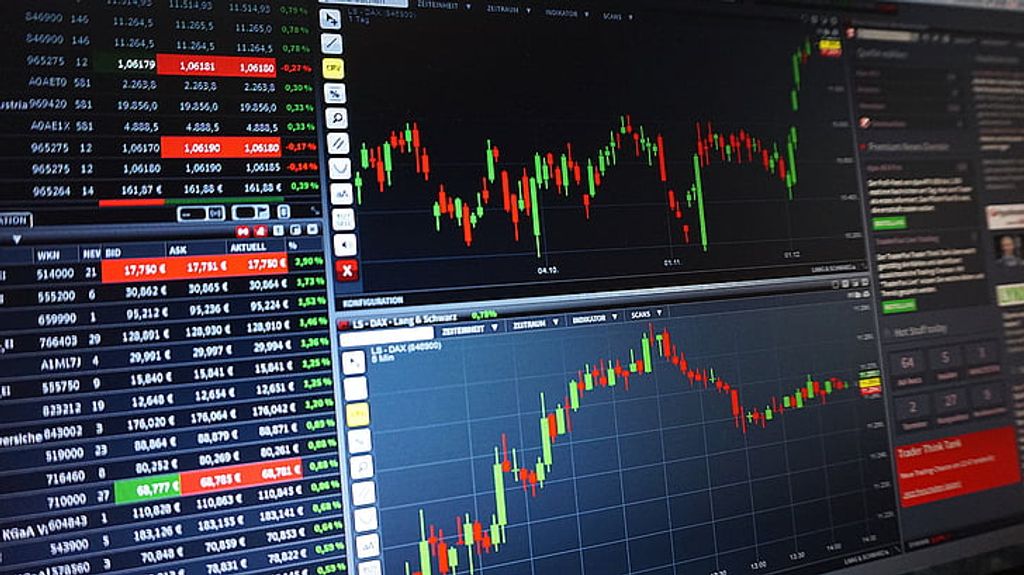
Role of Data Science in Analytics
Data science serves as the backbone of business analytics, providing essential tools and methodologies to glean valuable insights from raw data. This multidisciplinary field, which harnesses scientific methods, algorithms, and systems, is indispensable for businesses aiming to make data-driven decisions.
Data scientists and data analysts are often thought to be interchangeable, yet their roles are distinct, with data scientists delving deeper into predictive models and complex analysis. For instance, data scientists apply advanced AI tools and strategies to unlock the full potential of data, a capability that is increasingly crucial in the competitive business landscape.
The integration of data science into business analytics enables companies to not only understand past performance but also to forecast future trends and optimize operations.
Understanding the role of data science in analytics involves recognizing its contribution to real-time data analysis and the necessity for robust data management strategies. As big data continues to expand, the importance of data governance becomes more pronounced, ensuring that businesses can effectively leverage the insights provided by data science.
FAQs about Data Science in Analytics
In the realm of business analytics, data science plays a pivotal role, serving as the backbone that provides the necessary tools and methodologies to extract valuable insights from raw data. This integration enables businesses to make informed decisions based on quantifiable evidence, transcending beyond mere statistics to include machine learning, data mining, and predictive analytics.
Misconceptions about data science in business analytics are not uncommon. One prevalent myth is that data science is reserved for large corporations, but in reality, businesses of all sizes can harness its power. Addressing the challenges such as the scarcity of skilled data scientists and the integration of data science into existing business processes is essential for successful implementation.
Predictive analytics offers benefits for businesses, but challenges like data quality and ethics must be addressed. Prioritize ethical considerations for fair outcomes.
Strategies for Data Leaders
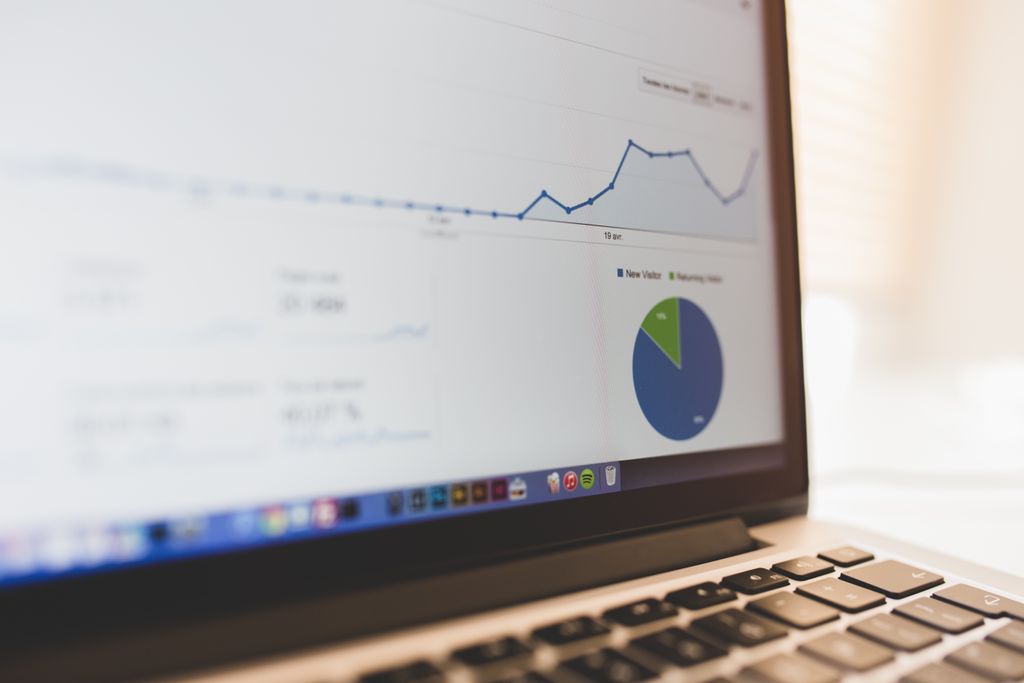
Driving Business Value with Data
In the realm of data leadership, the primary goal is to extract tangible outcomes from the vast sea of information. Data strategies that fuel real business value are not just about collecting and analyzing data; they are about using insights or technologies that drive impactful business outcomes.
The key to driving business value lies in the ability to measure and communicate the effectiveness of data initiatives.
For instance, aligning data quality metrics to your business process ensures that teams understand the 'why' and 'how' of data management. This approach fosters a culture of proactive data correction and continuous improvement.
Here are some tools employed by successful data leaders:
- Definition and management of data products
- Implementation of observability capabilities
- Documentation of data evolution through systems and processes
Each of these tools contributes to the overall visibility and quality of data, which in turn, supports better decision-making and alignment across organizations.
Governance and Compliance in Data Leadership
In the realm of data leadership, governance and compliance are pivotal for maintaining the integrity and security of data. Data governance is essential for ensuring data quality, security, and compliance, involving a wide range of roles within an organization. Regulatory compliance is a critical aspect that cannot be overlooked.
To effectively implement governance, organizations must prioritize data assets and focus leadership efforts where they are most needed. A holistic approach to data governance examines all data sets for reporting and innovation projects, ensuring that data security and privacy laws, such as GDPR, are met.
The integration of governance at the production and consumption levels, rather than functioning independently, fosters a culture of data accountability within product teams.
Best practices in data governance require the attention of senior management and a cultural shift towards a master data-based organization. A feedback mechanism is also crucial for continuous improvement. Below are six essential best practices:
- Secure senior management buy-in.
- Link governance to transformation themes.
- Prioritize essential data assets.
- Ensure data security and compliance with laws.
- Integrate governance with product teams.
- Establish a feedback mechanism for governance processes.
The Future of Business Intelligence
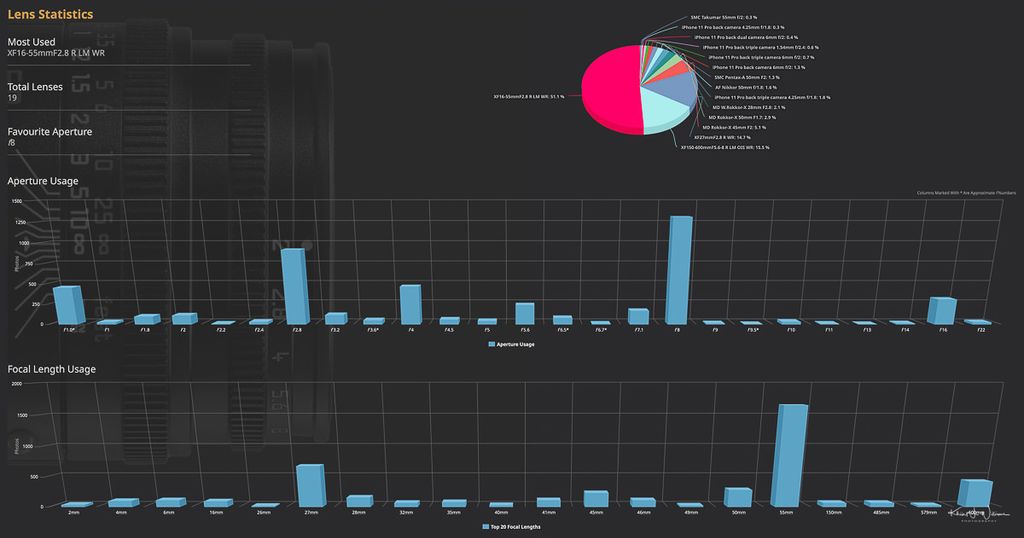
AI and Cloud Computing in BI
The integration of Artificial Intelligence (AI) and cloud computing is revolutionizing the landscape of Business Intelligence (BI). Cloud-based BI tools are becoming increasingly sophisticated, harnessing the power of AI to provide deeper insights and predictive analytics. This synergy allows for scalable solutions that can adapt to the growing data needs of businesses.
- AI enhances data processing and analysis capabilities.
- Cloud computing offers flexible and scalable infrastructure.
- Combined, they enable real-time data insights and decision-making.
The convergence of AI and cloud computing in BI is not just a trend; it's a strategic shift that empowers businesses to operate more intelligently and efficiently.
As companies continue to prioritize analytics, the challenges of Big Data and the need for rapid deployment from cloud to edge are addressed by AI systems. The future of BI is one where strategic planning and execution activities are increasingly automated, leading to a significant transformation in how businesses leverage data for competitive advantage.
Automation's Role in Business Analytics
The advent of automation in business analytics has marked a significant shift in how companies approach data-driven decision-making. Automation streamlines operations, reducing the need for manual intervention and allowing for more efficient data processing. This efficiency is not just about speed; it's about the capacity to handle complex tasks with precision.
One of the key benefits of automation is its ability to perform repetitive tasks without fatigue, ensuring consistent quality of analysis. For instance, automated systems can continuously monitor data streams for anomalies, a task that would be both tedious and error-prone if done by humans. Moreover, automation can be tailored to specific business needs, offering a level of customization that is difficult to achieve manually.
The integration of automation with business analytics tools has opened up new possibilities for predictive analytics and decision support. By leveraging machine learning algorithms, businesses can anticipate market trends and customer behavior, leading to more strategic decision-making.
While the potential of automation is vast, it is important to recognize the challenges it presents. The transition to automated systems requires careful planning and a clear understanding of the desired outcomes. Here are some key considerations:
- Ensuring data quality and integrity
- Selecting the right tools and platforms
- Training staff to work alongside automated systems
- Maintaining transparency and governance over automated processes
Embracing automation in business analytics is not just about adopting new technology; it's about fostering a culture that values data-driven insights and continuous improvement.
Conclusion
In conclusion, data science is undeniably a vital component of modern business analytics. Its ability to extract meaningful insights from vast amounts of data is invaluable in today’s data-driven world. As such, businesses must stay abreast of the latest trends and adapt accordingly to remain competitive. The future of business analytics lies in the effective application of data science, and the businesses that recognize this will be the ones to thrive.
FAQs about Data Science in Business Analytics
What is the role of data science in business analytics?
Data science is the backbone of business analytics, providing the tools and methodologies to extract valuable insights from raw data.
What are the challenges in implementing data science in business analytics?
Implementing data science in business analytics presents challenges such as scarcity of skilled data scientists and integration into existing processes.
How can businesses overcome the scarcity of skilled data scientists?
Businesses can invest in AI services that automate data analysis, reducing the need for human intervention.
What are the benefits of integrating data science into business analytics?
Integrating data science enhances decision-making, optimizes operations, and enables data-driven strategies.
What are key features of business intelligence dashboards?
BI dashboards offer quick insights, real-time monitoring, strategic decision-making, and cost-saving efficiency improvements.
How can BI dashboards be implemented effectively?
BI dashboards should be prepared with high-quality, consistent data to ensure accurate insights for effective decision-making.
What is the future of business intelligence with AI and cloud computing?
The future of BI involves leveraging AI and cloud computing to enhance analytics capabilities and drive innovation.
What role does automation play in business analytics?
Automation plays a critical role in streamlining processes, improving efficiency, and enabling faster data analysis in business analytics.