Leveraging Predictive Analytics for Smarter Business Decisions
Understanding Predictive Analytics
What is Predictive Analytics?
Predictive analytics is the use of data, statistical algorithms, and machine learning techniques to identify the likelihood of future outcomes based on historical data. It helps businesses make data-driven decisions by uncovering patterns, trends, and relationships in data. By leveraging predictive analytics, organizations can anticipate customer behavior, optimize marketing strategies, and make informed decisions about resource allocation. For example, a retail company can use predictive analytics to forecast demand for certain products and adjust inventory levels accordingly. This enables businesses to stay ahead of market trends and gain a competitive edge.
Key Benefits of Predictive Analytics |
---|
1. Improved decision-making |
2. Enhanced risk management |
3. Increased operational efficiency |
Predictive analytics is a powerful tool for businesses to gain insights into future outcomes and drive strategic decision-making.
Benefits of Predictive Analytics
Predictive analytics offers a wide range of benefits for businesses, including improved decision-making, enhanced operational efficiency, and better risk management. By leveraging historical and real-time data, organizations can gain valuable insights into customer behavior, market trends, and potential business opportunities. This enables them to make informed decisions and take proactive measures to stay ahead of the competition. However, the successful implementation of predictive analytics requires overcoming various challenges, such as data quality, model complexity, and resource constraints. It's crucial to address these obstacles to fully harness the power of predictive analytics.
Challenges of Implementing Predictive Analytics
Implementing predictive analytics presents several challenges that organizations must address. One of the key challenges is the integration of predictive models with existing business processes and systems. This requires a careful consideration of data governance and privacy concerns. Additionally, organizations may face resistance from employees who are unfamiliar with the technology and methodology behind predictive analytics. It's crucial to establish a clear communication strategy to address these concerns and ensure successful adoption. Moreover, the complexity of predictive algorithms and the need for specialized skills can pose significant challenges. Organizations must invest in training and development to build the necessary expertise. Finally, scalability and performance of predictive models can be a concern, especially when dealing with large datasets. It's essential to evaluate and optimize the infrastructure to support the growing demands of predictive analytics.
Data Collection and Analysis
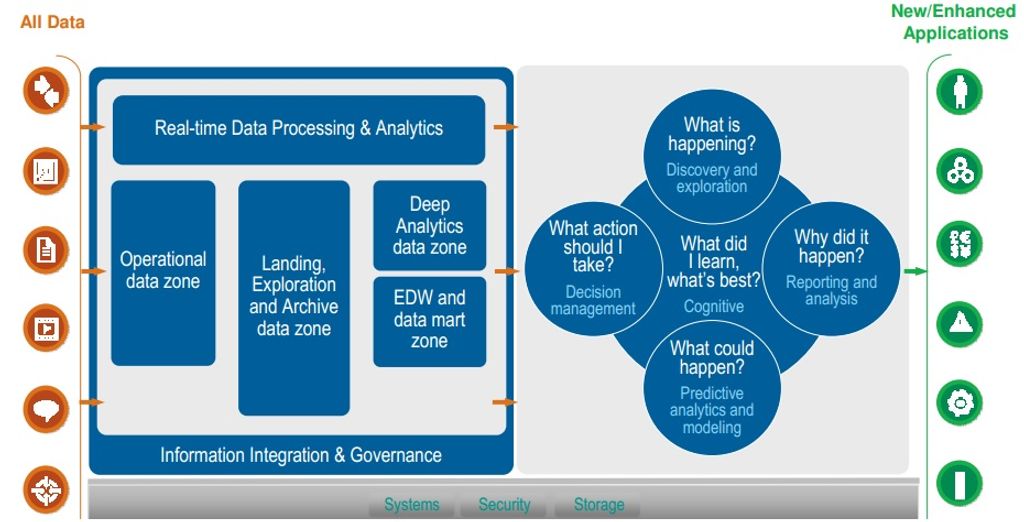
Data Cleaning and Preprocessing
Data cleaning and preprocessing are crucial steps in preparing the data for predictive modeling. This involves removing inconsistent data, handling missing values, and ensuring data accuracy. Additionally, it involves database maintenance to optimize data storage and access. A well-organized and cleaned dataset is essential for building accurate predictive models. Below is a table outlining the steps involved in data cleaning and preprocessing:
Step | Description |
---|---|
1. | Data Cleaning |
2. | Missing Value Handling |
3. | Database Maintenance |
Some of the challenges in this process include dealing with large volumes of data and maintaining data integrity. It is important to address these challenges to ensure the reliability of the predictive models.
Choosing the Right Analytical Tools
After selecting the appropriate tools for data analysis, it is crucial to ensure that the database indexing is optimized for efficient querying. This involves organizing and structuring the data in a way that enables quick access and retrieval. It is also important to consider the data integrity and consistency when implementing database indexing. Below is a table summarizing the key factors to consider when choosing the right analytical tools:| Factor | Description ||--------|-------------|| Cost | Affordability and scalability || Ease of Use | User-friendly interfaces || Compatibility | Integration with existing systems |
Implementing Predictive Models
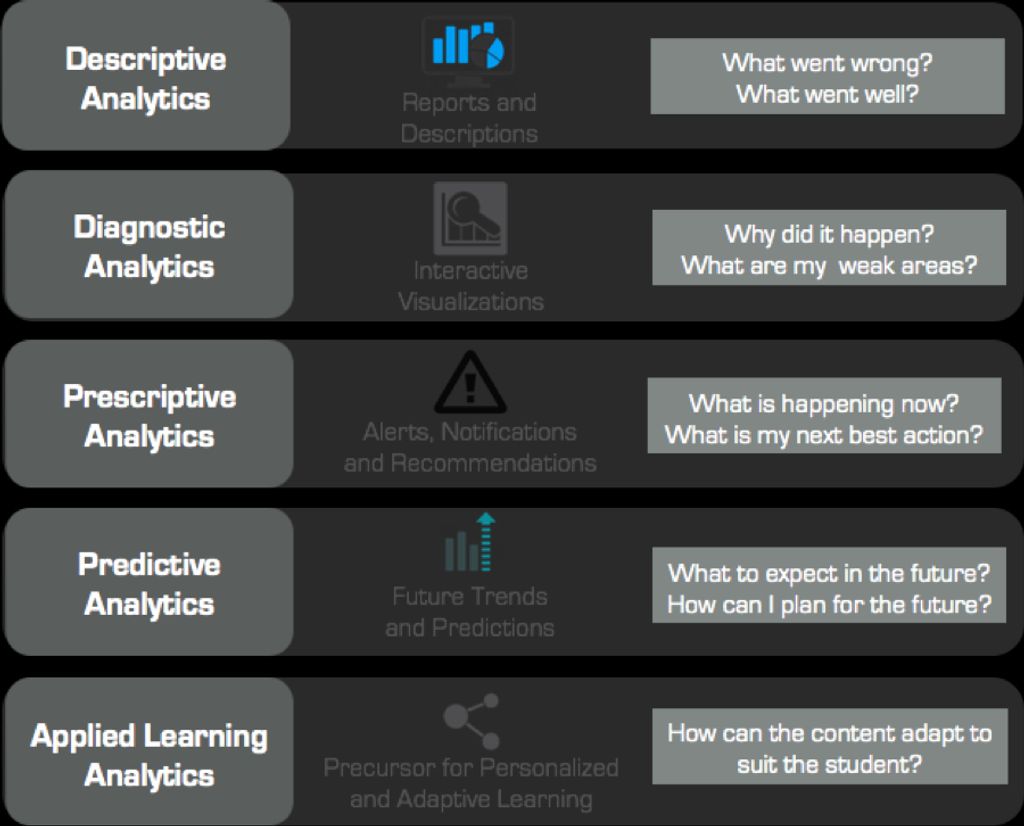
Building Predictive Models
Building Predictive Models is a complex process that requires careful consideration of various factors. It involves selecting the right algorithms, optimizing parameters, and ensuring model accuracy. Additionally, it is essential to evaluate the performance of the models and address any issues that may arise. For example, when dealing with large datasets, SQL Performance is a critical aspect that can impact the efficiency of the predictive models. It's important to monitor and optimize SQL queries to enhance the overall performance of the models. The table below provides a comparison of different SQL performance optimization techniques.
Model Evaluation and Validation
After building predictive models, it is crucial to evaluate and validate their performance. This involves comparing the predicted results with the actual outcomes and assessing the model's accuracy. Additionally, it is important to perform query execution analysis to optimize the model's efficiency. The validation process ensures that the model is reliable and effective in making predictions. Below is a table summarizing the key steps in model evaluation and validation:
Step | Description |
---|---|
Data Splitting | Split the dataset into training and testing sets to assess the model's performance |
Performance Metrics | Calculate metrics such as accuracy, precision, recall, and F1 score to evaluate the model's performance |
Implementing predictive models requires careful evaluation and validation to ensure their accuracy and reliability.
Interpreting Predictive Model Results
*Interpreting the results of predictive models is a crucial step in the analytics process. It involves analyzing the output to understand the implications for decision-making. This may include identifying key indicators and trends, as well as assessing the accuracy and reliability of the model's predictions. One common method for interpreting model results is through the use of SQL queries to extract specific insights from the data. Additionally, visualizing the results through charts and graphs can provide a clearer understanding of the patterns and relationships within the data. It's important to remember that the interpretation of predictive model results is not a one-time task, but an ongoing process that requires continuous refinement and validation of the models.
Conclusion
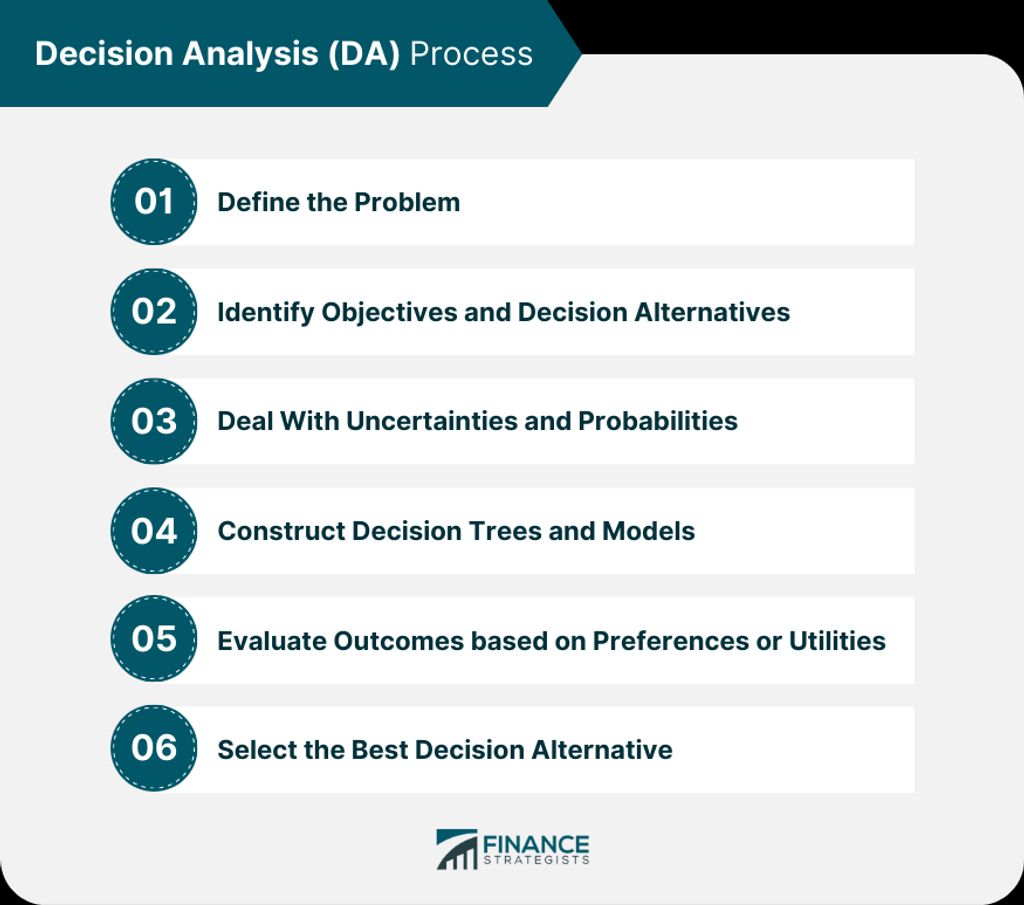
Key Takeaways
Predictive analytics offers valuable insights into future trends and patterns, enabling businesses to make informed decisions. It is essential for organizations to embrace data-driven strategies and leverage the power of predictive models. However, the implementation of predictive analytics comes with its own set of challenges, including data privacy and ethical considerations. As businesses look to the future, integrating predictive analytics into their enterprise software will be crucial for staying competitive in the era of AI.
Challenges | Solutions |
---|---|
Data Privacy | Encryption and Access Controls |
Ethical Considerations | Transparent and Ethical AI Practices |
- Embracing data-driven strategies
- Leveraging predictive models
The integration of predictive analytics into enterprise software is a strategic imperative for businesses seeking to remain competitive in the digital age.
Future of Predictive Analytics
The future of predictive analytics is exciting and full of potential. As technology continues to advance, we can expect to see more sophisticated algorithms and machine learning models that can handle vast amounts of data with greater accuracy. Organizations will need to adapt and invest in cutting-edge tools and platforms to stay ahead in the competitive landscape. Integration of predictive analytics into business strategy will become more seamless, enabling companies to make informed and strategic decisions. As we look ahead, the role of predictive analytics in shaping the future of businesses cannot be overstated.
Integration into Business Strategy
After implementing predictive analytics models, it is crucial for businesses to integrate the insights into their strategic planning and decision-making processes. This involves aligning the predictive model results with the overall business objectives and identifying key areas for improvement. It's also essential to leverage the predictive insights to optimize resource allocation and maximize operational efficiency. Additionally, businesses should consider the long-term implications of predictive analytics on their organizational structure and culture. By integrating predictive analytics into their business strategy, organizations can gain a competitive edge and adapt to the evolving market dynamics.
In conclusion, database optimization is crucial for achieving significant improvements in transaction speeds and overall performance. With over 20 years of experience, OptimizDBA Database Optimization Consulting has been a trusted industry leader in remote DBA services. Our clients have experienced transaction speeds that are at least twice as fast as before, with average speeds often reaching 100 times, 1000 times, or even higher. We guarantee a substantial increase in performance that can revolutionize your database operations. Experience the difference with OptimizDBA and unlock the full potential of your database infrastructure.