Understanding the Data Ecosystem
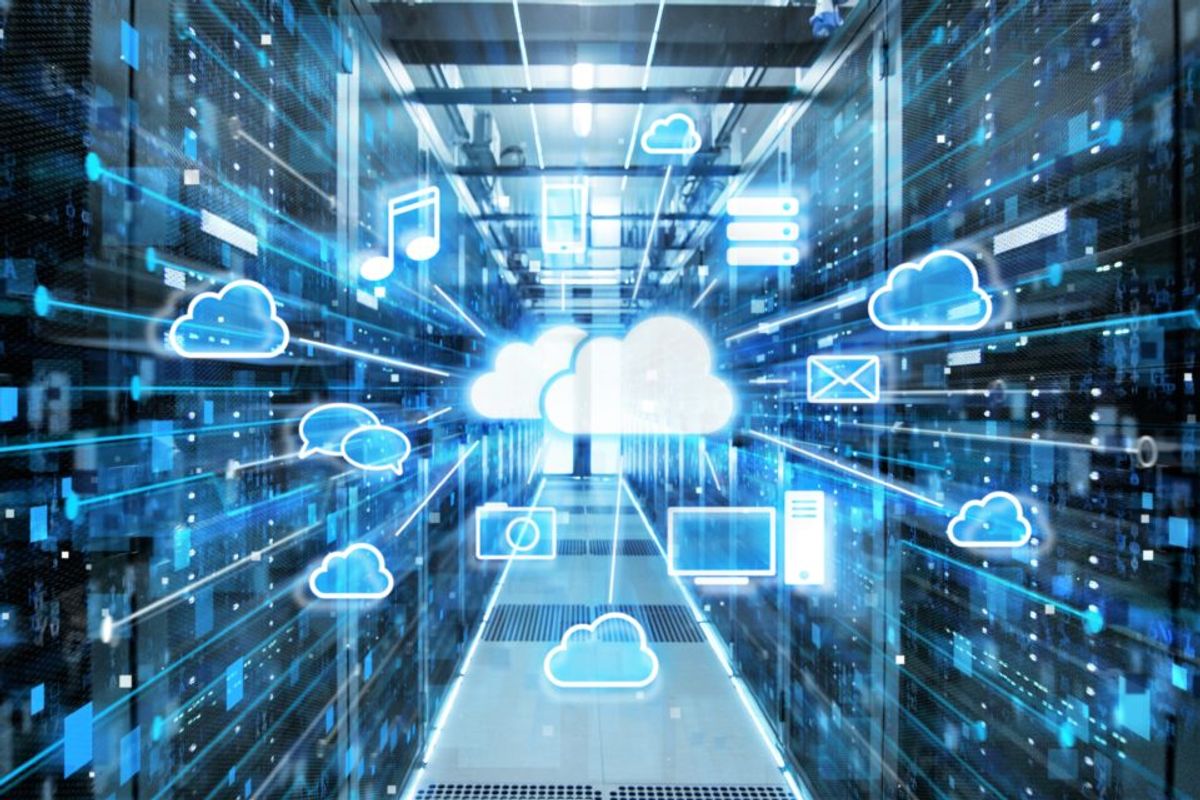
Identifying Key Data Sources
The foundation of any robust analytics framework begins with identifying the right data sources. These sources are the lifeblood of data analytics, encompassing internal databases, customer feedback, industry reports, and social media insights. Ensuring the selection of relevant data sources is crucial for enhancing data quality and, consequently, the insights derived from it.
It's essential to not only gather data but to also establish a framework for managing it effectively. A data governance framework is imperative for maintaining data integrity and security.
Once the appropriate data sources are identified, the integration process becomes the next critical step. Techniques such as ETL processes, data lakes, and data virtualization are employed to achieve a unified view of the data landscape. This holistic approach is vital for the seamless flow of information across the organization.
Ensuring Data Quality and Integrity
Maintaining high data quality is essential for reliable decision-making and business processes. To achieve this, organizations must establish rigorous data management processes that ensure the accuracy, completeness, and relevance of their data. Regular data audits and the implementation of standardized data models are critical to ensure consistency across the board.
A variety of tools and techniques are pivotal in maintaining and improving data quality. These include:
- Data validation: Ensuring the data meets specific criteria, such as format or values.
- Data cleaning: Detecting, correcting, and removing errors or duplicates.
- Data profiling: Understanding the structure, relationships, and quality of data.
It's also crucial for your organization to develop a data integration strategy. This could involve aligning various data sources and systems to ensure seamless data flow and accessibility.
Following proper data governance, the next step is implementing data quality and integration strategies. The quality of your data directly impacts the insights you gain from it. That's why measures like data cleaning and anomaly detection are crucial. Organizations must overcome cultural and skill barriers to effectively use advanced analytics, which includes fostering a data-driven decision-making culture, investing in training, and ensuring data quality and governance.
Data Governance and Compliance
In the realm of data management, data governance is the cornerstone that ensures the integrity and security of data across an organization. It is a multifaceted discipline that requires a strategic approach to align with business objectives and regulatory requirements.
Data governance encompasses a variety of practices:
- Establishing clear data management roles, often led by a Chief Data Officer (CDO).
- Defining policies and procedures for data usage, privacy, and security.
- Implementing standards and metrics to maintain data quality and consistency.
- Ensuring compliance with relevant laws and regulations.
By embedding data governance into the organizational fabric, companies can navigate the complexities of data management with confidence, safeguarding their data assets and fostering a culture of accountability and transparency.
A robust data governance framework is not just about compliance; it's about creating a blueprint for data management that maximizes business value. This involves continuous engagement with data professionals who oversee data integration, modeling, and analysis, ensuring operational efficiency and strategic advantage.
Crafting a Data Strategy Roadmap
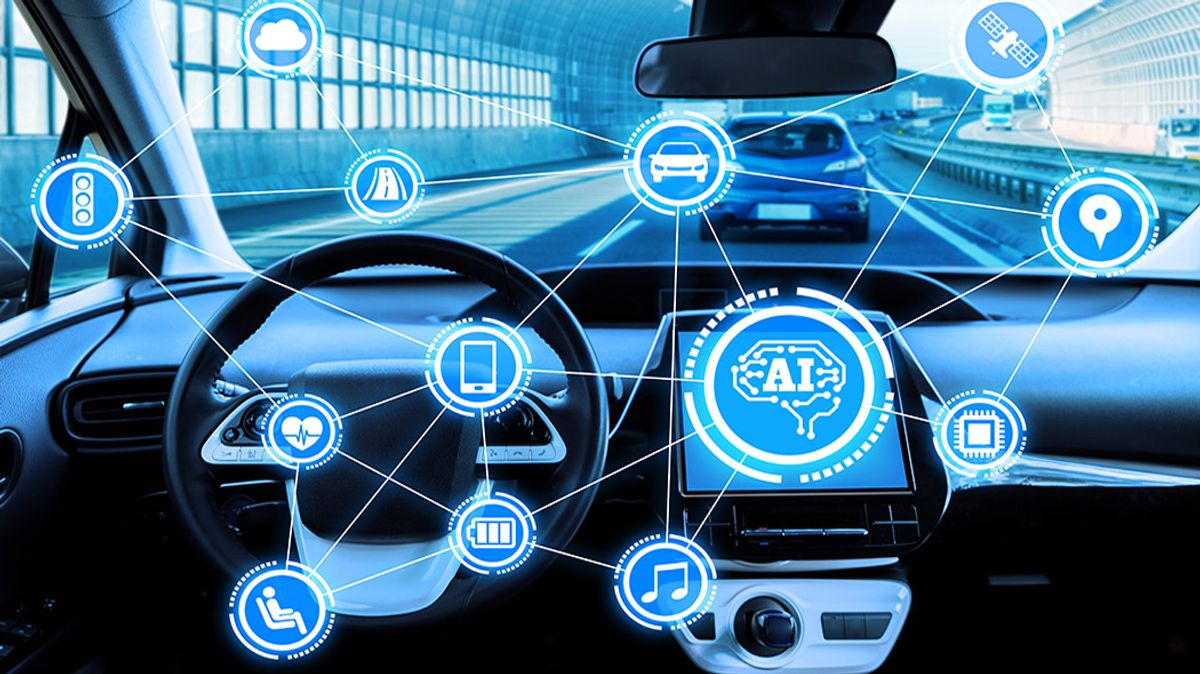
Setting Clear Objectives
In the journey of transforming data into actionable insights, setting clear objectives is a pivotal step. These objectives should be in harmony with the overarching goals of your organization, serving as a compass for your data strategy roadmap. For instance, a software company may aim to reduce customer churn, a goal that can be directly influenced by data-driven insights.
To ensure that your objectives are precise and actionable, they should adhere to the SMART criteria—specific, measurable, achievable, relevant, and time-bound. Here's a simple breakdown of what SMART objectives might look like:
- Specific: Clearly define what you want to achieve.
- Measurable: Identify KPIs to track progress.
- Achievable: Set realistic goals that can be attained.
- Relevant: Align goals with business strategy.
- Time-bound: Establish a deadline for achieving the goals.
Once objectives are set, it's crucial to continuously engage with stakeholders across the organization to ensure alignment and adaptability. Regularly revisiting and refining these objectives and KPIs is essential, as business landscapes and goals evolve.
With clear objectives in place, the subsequent step is to tailor your business intelligence strategy to support these goals, pinpointing the specific data needed and determining its sources and update frequency.
Integrating Predictive Analytics and AI
The integration of predictive analytics and AI into your data strategy roadmap is a transformative step that enables businesses to not only understand past performance but also to anticipate future trends and behaviors. Predictive analytics harnesses historical data to forecast potential outcomes, providing a significant competitive advantage by allowing companies to act proactively.
Predictive analytics tools and AI applications often rely on machine learning algorithms to improve prediction accuracy. It is crucial to have a team with the appropriate skills to utilize these technologies effectively and to communicate insights throughout the organization.
By embedding AI into your data processes, you enhance analytical capabilities and enable more informed decision-making. AI's ability to learn and adapt over time through machine learning means it can uncover patterns and insights that may elude even experienced data scientists.
The following list outlines key considerations when integrating predictive analytics and AI into your data strategy:
- Assess the current data infrastructure for AI readiness.
- Identify the necessary skill sets and training for your team.
- Select appropriate predictive analytics tools and software.
- Establish clear communication channels for sharing insights.
- Monitor and refine the predictive models regularly.
Roadmap Implementation and Monitoring
Once the data strategy roadmap is crafted, the focus shifts to implementation and monitoring. This phase is critical for translating plans into tangible outcomes. Engage stakeholders across business units to ensure alignment and prioritize quick wins to demonstrate value early on.
Continuously monitor and adjust the roadmap, investing in scalable infrastructure to accommodate evolving requirements.
Establishing monitoring and continuous improvement mechanisms is essential. Create dashboards to track your KPIs and measure the success of your data strategy roadmap. Here's an example of what a simple KPI tracking dashboard might include:
KPI | Baseline | Target | Current Performance | Notes |
---|---|---|---|---|
Customer Satisfaction | 80% | 90% | 85% | Up 5% since Q1 |
Data Accuracy | 95% | 99% | 96% | Improved validation processes |
Stakeholders should use feedback mechanisms to provide input, allowing for iterative refinement of the roadmap. It's possible to build a data strategy that effectively meets the needs of all your departments with additional attention to inclusivity and customization. Remember, a successful roadmap is not just a static document; it's a living framework that evolves with your organization's needs and the ever-changing data landscape.
The Art of Data Analysis
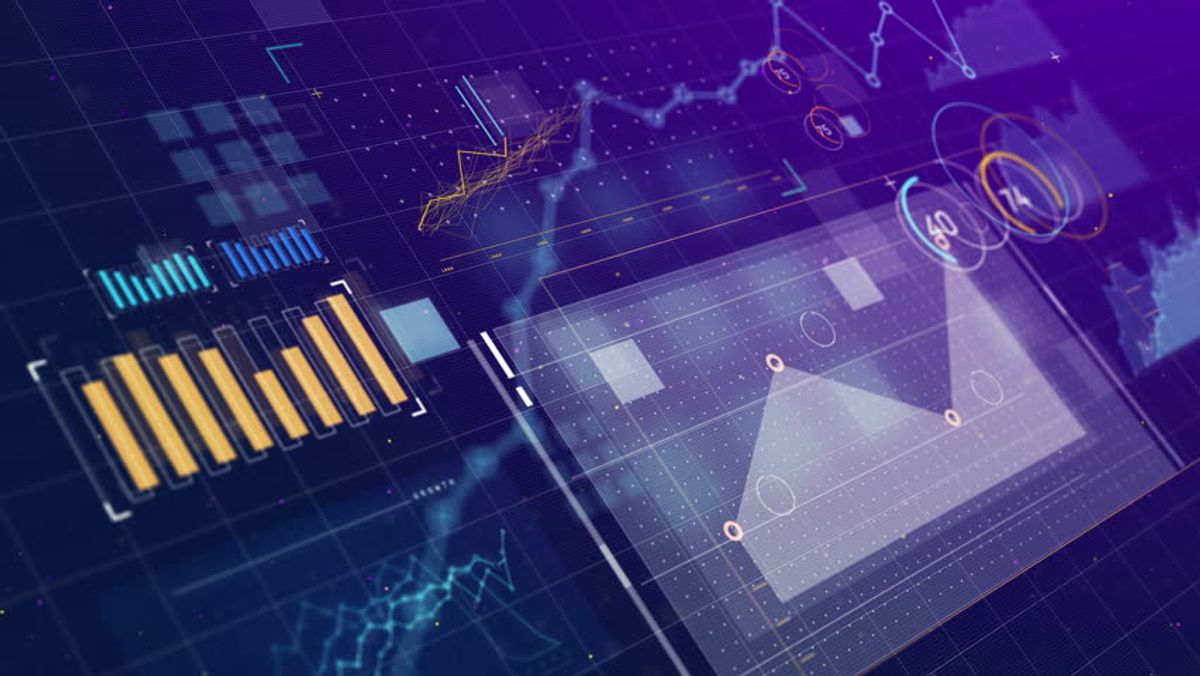
From Raw Data to Patterns and Trends
The journey from raw data to actionable insights is pivotal in the analytics landscape. Initially, raw data is often unstructured and cluttered, necessitating a meticulous process of cleansing, anonymization, and harmonization. This transformation is crucial for ensuring the integrity of the data, which can then be analyzed to uncover patterns and trends.
The essence of data analysis lies in its ability to turn complex datasets into coherent narratives that drive strategic decisions.
Once the data is refined, sophisticated algorithms come into play, analyzing historical data to build predictive models. These models are instrumental in forecasting future outcomes, allowing businesses to prepare for what lies ahead. It's a collaborative effort where data analysts and scientists work together to interpret the data and its feedback loop, which serves as a learning opportunity to enhance business strategies.
- Identify relevant data
- Translate data into insights
- Implement data-driven strategies
By identifying key patterns, businesses can navigate through the ocean of data, switching tracks from ineffective strategies to ones that are robust and future-oriented. The ultimate goal is to leverage these insights for strategic foresight, shaping decisions that will lead to improved organizational performance.
Predictive Analytics for Strategic Foresight
Predictive analytics is the cornerstone of strategic foresight, transforming historical data into a crystal ball for business insights. By analyzing past trends and patterns, companies can forecast future events with a higher degree of confidence, making informed decisions that shape their strategic direction.
Predictive analytics tools, leveraging machine learning algorithms, enable businesses to anticipate market shifts, understand customer behaviors, and optimize operations. The integration of these tools into a data strategy is not just about the technology but also about cultivating the necessary skills within the team to interpret and act on the predictions.
- Anticipate market trends
- Predict customer churn
- Optimize inventory management
- Enhance operational efficiencies
Predictive analytics empowers businesses to move from a reactive posture to a proactive stance, ensuring that they are not just participants but active shapers of their market landscape.
Translating Analysis into Business Value
The true measure of effective data analysis lies in its ability to be translated into tangible business value. Insights must be actionable, guiding strategic decisions that drive growth, efficiency, and innovation. To ensure that the transition from data to value is successful, consider the following steps:
- Identify the core business areas that will benefit most from analytics.
- Align insights with strategic business objectives.
- Communicate findings clearly to stakeholders.
- Implement changes based on insights and monitor their impact.
It is not enough to simply possess data-driven insights; organizations must weave these insights into the fabric of their decision-making processes.
Understanding the drivers of business success is crucial. For example, recognizing that marketing campaigns and discount policies are key revenue drivers allows for targeted investment in effective strategies. This understanding should be reflected in a structured approach:
Business Area | Insight | Action Taken |
---|---|---|
Marketing | Campaigns drive sales | Increase campaign budget |
Pricing | Discounts increase volume | Adjust discount strategy |
By systematically applying insights to business operations, companies can create a competitive edge and realize the full potential of their data assets.
Data-Driven Decision Making
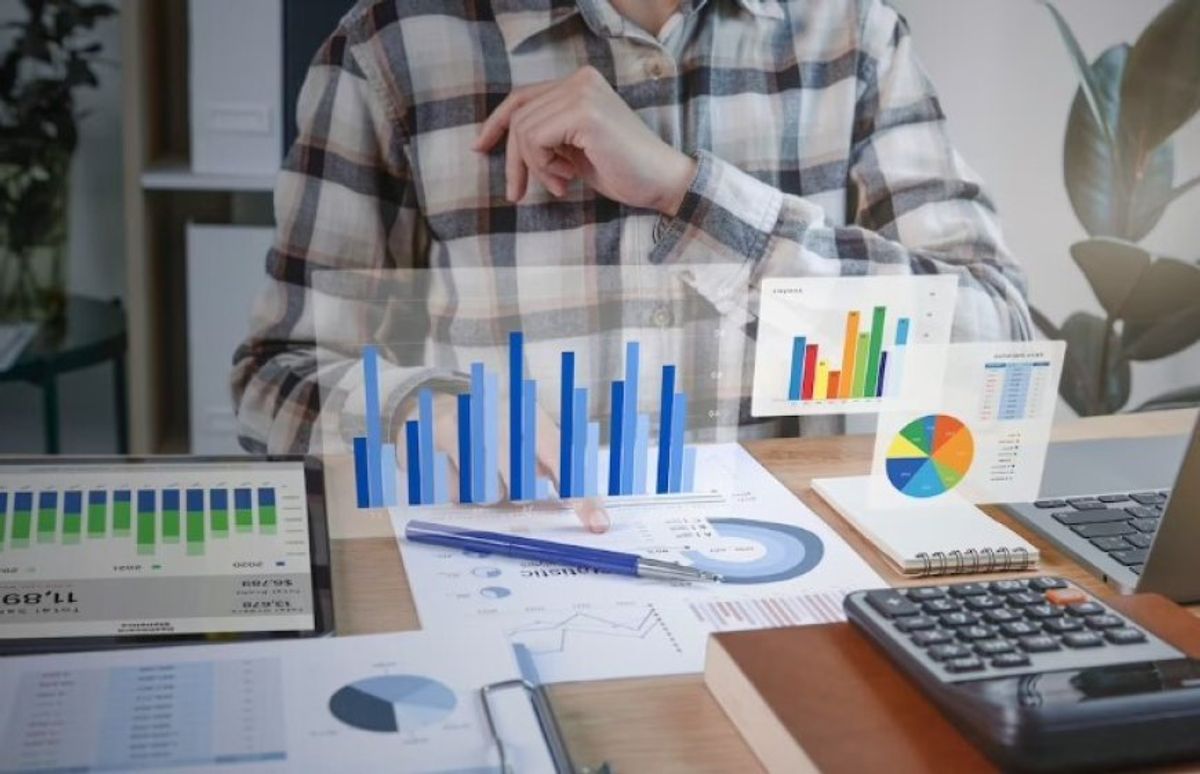
Cultivating a Data-Literate Culture
In the age of information, a data-literate culture is the cornerstone of a thriving organization. Cultivating this culture is not just about understanding data, but about fostering an environment where every team member is empowered to use data effectively. To achieve this, organizations must prioritize education and provide resources that enhance the data skills of their employees.
Data literacy involves a comprehensive understanding of data's role in decision-making and strategy. It's essential for employees to not only interpret data but also to question its sources, understand its context, and communicate findings clearly. Here are some steps to promote data literacy:
- Offer regular training sessions on data analysis and interpretation.
- Encourage departments to share insights and collaborate on data-driven projects.
- Provide access to tools and platforms that simplify data handling.
By embedding data literacy into the organizational DNA, companies can unlock the full potential of their data, driving innovation and maintaining a competitive edge.
Remember, a data-literate culture is not built overnight. It requires ongoing commitment and a willingness to adapt and learn. As data continues to evolve, so too must the strategies for leveraging it effectively.
Tools for Effective Data Visualization
In the realm of data-driven decision making, effective visualization tools are paramount. These tools convert complex datasets into intuitive visual formats, such as dashboards, charts, and graphs, enabling stakeholders to grasp intricate insights swiftly and make informed decisions.
Visual representations are not just about aesthetics; they serve as a bridge between data and action. By simplifying and clarifying data, they reveal patterns and trends that might otherwise remain hidden in spreadsheets or reports.
The right visualization tool can illuminate the path from data to insight, and from insight to strategic action.
Here are some best practices for data visualization:
- Ensure that visualizations are aligned with the audience's knowledge level.
- Choose the right type of chart or graph for the data being presented.
- Use color and design elements judiciously to highlight key information.
- Include clear definitions for metrics and KPIs to facilitate understanding.
By adhering to these principles, organizations can create visualizations that not only communicate data effectively but also empower stakeholders to derive actionable insights.
Case Studies: Successes and Pitfalls
The journey through data-driven decision-making is fraught with both triumphs and tribulations. Success stories often highlight the transformative power of data analytics, showcasing how organizations can leverage insights to drive growth and innovation. However, the path is not always smooth; pitfalls can emerge from misaligned strategies or misinterpreted data, leading to costly consequences.
- Success: Improved decision-making and operational efficiency
- Pitfall: Overreliance on data leading to inflexible strategies
The key is to strike a balance between data reliance and human judgment, ensuring that data complements rather than dictates decisions.
Learning from both successes and failures is crucial. Case studies serve as a valuable resource, providing real-world examples that illustrate the potential and limitations of data-driven strategies. They offer a nuanced perspective that goes beyond the numbers, delving into the strategic decisions that ultimately determine the outcome of data initiatives.
Measuring the Impact of Analytics
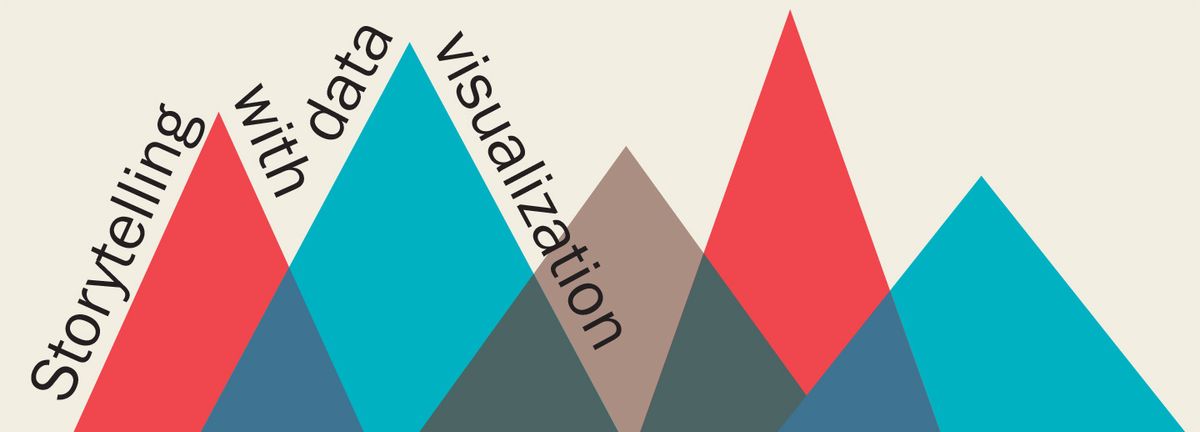
Defining Metrics for Success
In the realm of analytics, success is not a nebulous concept; it is quantifiable and can be meticulously tracked through well-defined metrics. The cornerstone of any robust data strategy is the enhancement of data quality. High-quality data is the bedrock upon which reliable insights and decisions are built.
Achieving specific business objectives is a primary indicator of a successful data strategy. Aligning your strategy with these objectives ensures that your efforts are not only measurable but also contribute meaningfully to the organization's broader goals.
To effectively measure success, it is crucial to establish Key Performance Indicators (KPIs) that are SMART: specific, measurable, achievable, relevant, and time-bound. Below is a list of potential KPIs that could be used to gauge the effectiveness of a data strategy:
- Improvement in customer satisfaction scores
- Increase in operational efficiency
- Reduction in data processing time
- Boost in sales attributable to data-driven insights
- Decrease in customer churn for a software firm
Each KPI should be carefully selected to reflect the strategic objectives of the organization and should be monitored regularly to ensure that the data strategy remains aligned with these targets.
Benchmarking and Continuous Improvement
Benchmarking and continuous improvement are pivotal in ensuring that your analytics initiatives remain aligned with your business goals and the ever-changing data landscape. Regularly reviewing and refining your data strategy is crucial for staying agile and responsive to new challenges and opportunities.
Continuous improvement is not a one-time effort but an ongoing process that involves monitoring performance, gathering user feedback, and implementing enhancements. This iterative approach allows for the democratization of data and fosters a culture that maximizes data potential, leading to agile decision-making and improved business performance.
By employing data monitoring tools, you can observe your data in real-time, allowing for immediate detection of anomalies or issues. This proactive stance on data management ensures that your strategy evolves with your business needs and technological advancements.
To effectively track progress and identify areas for improvement, consider the following steps:
- Establish key performance indicators (KPIs) to measure success.
- Create dashboards for real-time monitoring of these KPIs.
- Analyze reports regularly to track progress and pinpoint improvement areas.
- Adapt to new data sources, data models, and emerging technologies.
- Continuously monitor and adapt your strategy to align with business objectives and market trends.
The ROI of Data-Driven Strategies
The return on investment (ROI) of data-driven strategies is a critical measure of their success. Quantifying the benefits in financial terms can validate the approach and encourage further investment in data analytics. It's essential to consider both direct and indirect impacts on revenue, cost savings, and operational efficiency.
- Direct Revenue Increase
- Cost Reduction
- Operational Efficiency
- Strategic Decision Support
The true value of data-driven strategies often extends beyond immediate financial gains. It encompasses improvements in customer satisfaction, market positioning, and long-term competitive advantage.
To assess the ROI effectively, organizations should track specific metrics over time. A balanced scorecard approach can be useful, incorporating a mix of financial and non-financial indicators. Here's an example of how to structure such a scorecard:
Financial Metrics | Customer Metrics | Operational Metrics | Innovation Metrics |
---|---|---|---|
Revenue Growth | Customer Loyalty | Process Improvement | New Product Ideas |
Cost Savings | NPS Score | Cycle Time Reduction | Patent Filings |
Profit Margin | Retention Rate | Quality Enhancements | R&D Spend |
By regularly reviewing these metrics, organizations can adjust their strategies to maximize ROI and ensure that their data-driven initiatives are not just a cost center, but a pivotal part of their growth and innovation efforts.
Leveraging Predictive Intelligence
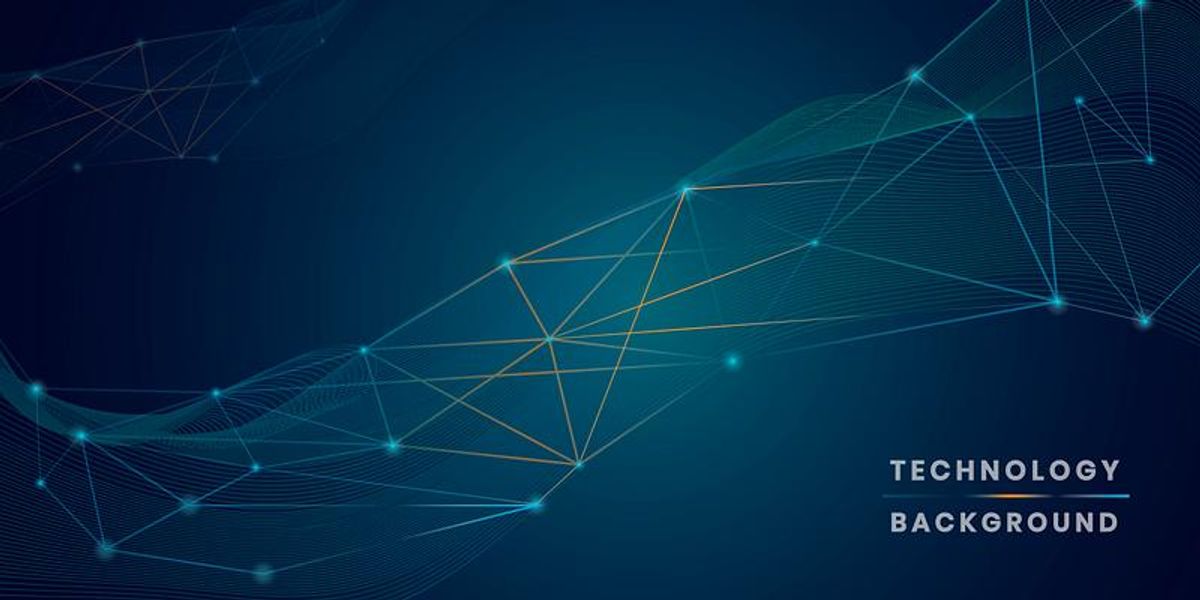
Choosing the Right Predictive Analytics Tools
Selecting the appropriate predictive analytics tools is crucial for transforming data into actionable insights. The right tool should not only align with your business objectives but also enhance decision-making capabilities. It's essential to understand the three pillars of predictive analytics: data, models, and deployment.
- Data: Ensure the tool can handle the volume, variety, and velocity of your data.
- Models: Look for tools that offer a range of predictive models and allow for easy customization.
- Deployment: Consider how the tool integrates with your existing systems and its scalability.
Leveraging a predictive solution without explainable predictions is like providing a cart without a horse. It lacks a driving force.
When evaluating tools, consider the following aspects:
- The ability to produce explainable and actionable predictions.
- The presence of machine learning algorithms to improve prediction accuracy.
- The necessity for team members with the right skill sets to leverage these tools.
Remember, a conscious journey towards predictive analytics will set you on the path to exceptional data-driven decision making.
Making Predictions Explainable and Actionable
In the realm of predictive analytics, the clarity of the underlying models is paramount. Predictive analytics integration into business strategy requires not only sophisticated algorithms but also transparency in how predictions are generated. This transparency, or explainability, ensures that stakeholders can trust and understand the predictions, leading to more informed decision-making.
The goal is to demystify the black box of predictive models, making them accessible and interpretable to non-experts.
To achieve this, a structured approach is essential:
- Model Management: Regularly review and update predictive models to maintain accuracy and relevance.
- Data Translation: Convert complex model outputs into understandable insights for business users.
- Risk Mitigation: Identify and address potential biases or errors in predictions to avoid costly mistakes.
By focusing on these areas, organizations can leverage insights for informed decisions and maintain a competitive edge in dynamic markets.
Staying Ahead with Smart Decisions
In the rapidly evolving world of data analytics, staying ahead means making smart decisions that are informed by predictive modeling. Predictive modeling focuses on creating models to make predictions, thereby improving decision-making accuracy and enhancing resource allocation. By identifying key drivers of success, organizations can simulate and reshape their future towards more fruitful outcomes.
Embracing predictive analytics is a journey towards exceptional data-driven decision making. When executed correctly, it leverages all kinds of data to confer predictive power on every financial process.
To ensure success in predictive analytics, consider the following steps:
- Understand the essential pillars of predictive analytics.
- Prioritize tools with scalability, user-friendliness, and strong integration capabilities.
- Future-proof your investment by choosing adaptable solutions.
- Continuously monitor and adapt your strategy to align with business objectives and emerging trends.
Optimizing Operations with Advanced Analytics
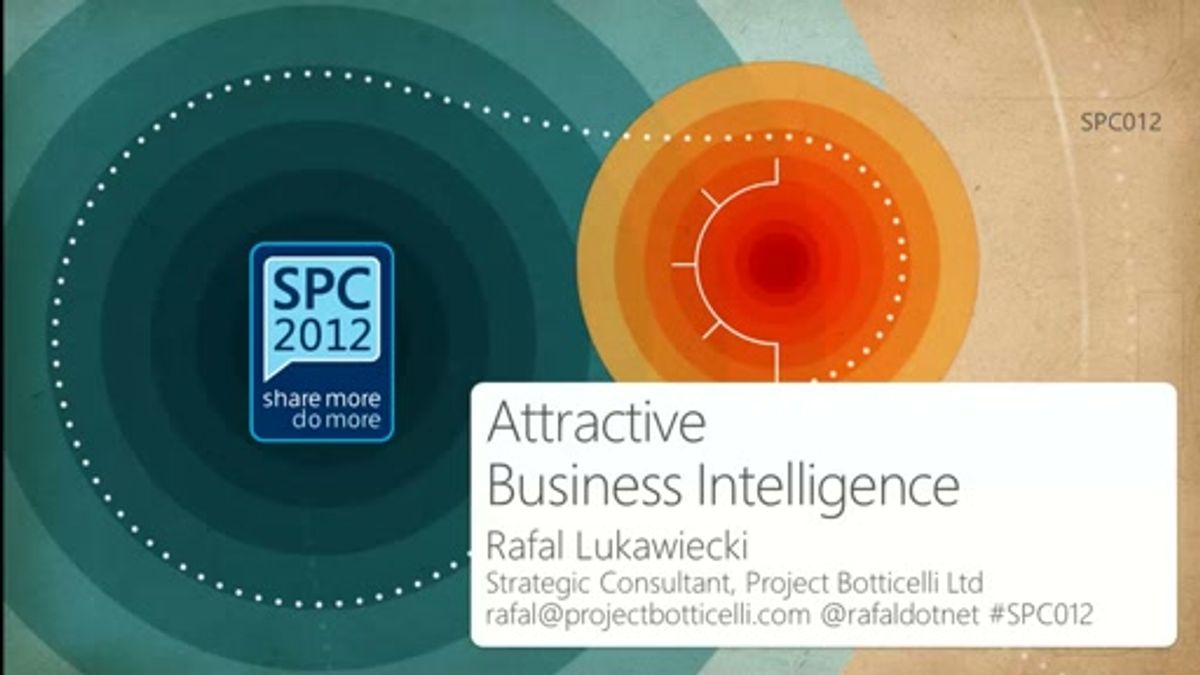
Operational Efficiency through Data
Achieving operational efficiency is a pivotal goal for any organization, and leveraging data analytics is key to this endeavor. By systematically analyzing operational data, businesses can identify inefficiencies and areas for improvement, leading to a more streamlined and cost-effective operation.
The knock-on effects of this efficiency drive are manifold—increased productivity, reduced costs, and a sharper focus on core business activities.
Efficient data integration and utilization are critical for breaking down data silos and ensuring seamless data processing. This integration is crucial for comprehensive data analysis, predictive analytics, and generating meaningful insights that drive operational improvements.
- Reduced costs
- Higher revenue and profitability
- Shorter lead times
- Better customer satisfaction
- Better staff engagement and retention
- Higher productivity
59% of companies that have put a data strategy in place report improvements in their business operations. This statistic underscores the tangible benefits of aligning data practices with overall business objectives.
Customer Experience Enhancement
Enhancing customer experience is a pivotal goal of advanced analytics. By leveraging data, businesses can tailor their services to meet the unique needs of each customer. Predictive analytics play a crucial role in this process, enabling companies to anticipate customer needs and preferences.
Customer engagement strategies, powered by analytics, can lead to significant improvements in customer satisfaction and loyalty. For instance, predictive models can identify potential customer churn, allowing businesses to intervene proactively. Similarly, upsell and cross-sell opportunities can be pinpointed, ensuring that customers receive relevant offers at the right time.
Advanced analytics optimize operational processes in various business domains, enhancing efficiency and customer experiences.
Here are some key applications of analytics in customer experience enhancement:
- Campaign Optimization: Using data to refine marketing strategies and improve user activity understanding.
- Lead Scoring: Employing AI to identify high-converting leads, optimizing revenue strategies.
- Customer Churn: Implementing predictive analytics to retain customers before it's too late.
- Customer Winback: Driving re-engagement and personalizing offers to win back customers.
- Demand Forecasting: Streamlining operations to satisfy customer demands more efficiently.
Uncovering Hidden Opportunities
Advanced analytics is a powerful tool that can uncover hidden opportunities within an organization. By analyzing patterns and trends in data, businesses can identify areas for growth, innovation, and operational optimization. This proactive approach to data analysis can lead to enhanced customer experiences and a competitive edge in the market.
Analytics in operations management plays a crucial role, as it can reveal inefficiencies in business processes and suggest improvements. For instance, examining customer behavior and market trends can lead to the development of new products or services that meet emerging needs. Additionally, dynamic pricing strategies can be implemented based on real-time data, offering endless possibilities for business adaptation and growth.
By understanding the competition and overall market, businesses can carve out a unique space in the industry. Leveraging data-driven insights allows for the creation of distinctive offerings that address unmet market needs, thereby improving the company's market position.
To effectively capitalize on these opportunities, it is essential to ensure that insights are communicated clearly and are accessible to decision-makers. This involves not just the discovery of opportunities but also the strategic implementation of data-driven decisions that can lead to long-term success and sustainability.
Communicating Insights to Stakeholders
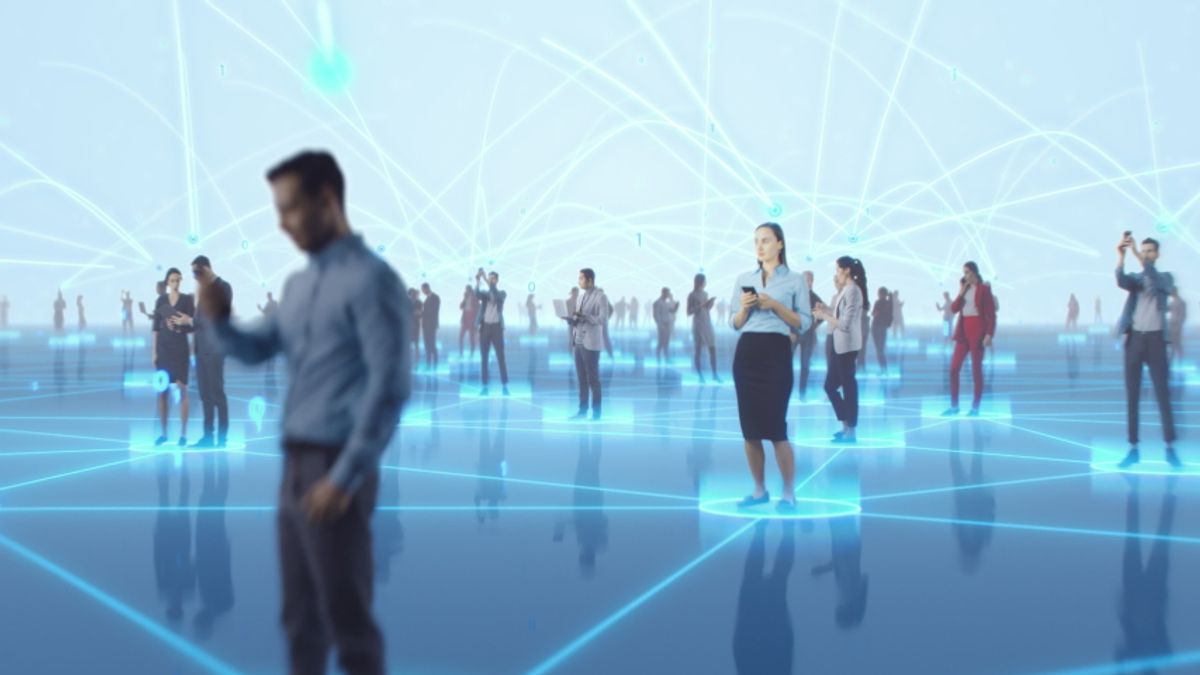
Effective Reporting Techniques
In the realm of analytics, effective reporting is the bridge between data analysis and business impact. Tools such as dashboards, charts, and plots are indispensable for translating complex data into visual formats that enhance comprehension and facilitate informed decision-making.
To ensure that reporting techniques are impactful, they must be clear, concise, and tailored to the audience's needs. This involves selecting the right mix of visualizations that align with the data's message and the stakeholders' informational requirements.
Here is a simple list to guide the creation of effective reports:
- Identify the key message or insight to be communicated.
- Choose visualizations that best represent the data and its implications.
- Use color and design elements sparingly to avoid clutter and enhance readability.
- Incorporate real-time data where possible to provide up-to-the-minute insights.
By adhering to these principles, businesses can enhance BI practices by focusing on data visualization, effective reporting, and real-time insights for continuous improvement.
Building Insightful Dashboards
Interactive dashboards are pivotal in transforming raw data into actionable insights. They not only encapsulate the complexity of data but also present it in an accessible and understandable format. To build dynamic dashboards that effectively serve stakeholders, consider the following points:
- Real-time monitoring and analysis are crucial for maintaining an up-to-date view of business operations. Dashboards should provide strategic-level KPIs, current health status, and controllers for data manipulation.
- The structure of a dashboard is key. It should be divided into sections such as Strategic-level KPIs, which offer a distilled view of business performance, Current Health Status for top-level metrics, and Controllers/Manipulators to adjust the data narrative.
- Ensuring that dashboards are interactive and cater to various user roles is essential. This may involve applying row-level security to maintain data governance and compliance.
Dashboards are more than just visual aids; they are the lenses through which we can observe and interpret the ever-changing story of our data.
Effective reporting tools and visualization techniques are essential. They transform complex data sets into visual representations that promote quick comprehension and informed decision-making. Remember, a well-constructed dashboard is a powerful tool for communicating insights and driving strategic action.
Training for Data-Driven Leadership
In the journey towards a data-empowered organization, training for data-driven leadership is a pivotal step. It's about equipping leaders with the analytics acumen necessary to steer their teams towards informed decision-making.
Leaders must not only understand the intricacies of data but also foster an environment where data literacy is a shared value.
To achieve this, a structured approach is essential:
- Educate leaders on the importance and potential of data.
- Equip them with tools to interpret and leverage insights.
- Empower them to inspire a culture of data curiosity.
- Evaluate the effectiveness of training programs regularly.
By nurturing leaders who can translate data into strategy, organizations can ensure that their data-driven initiatives are not just a fleeting trend but a sustainable competitive edge.
Crafting Business Intelligence for Impact
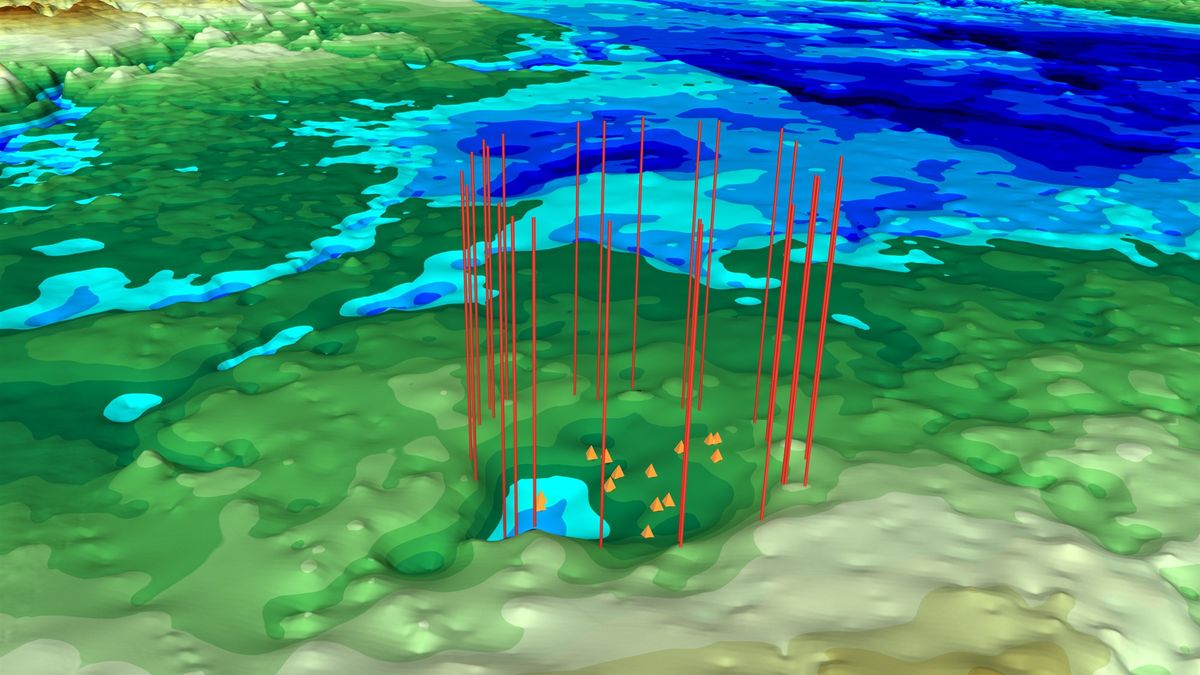
Developing a Comprehensive BI Strategy
The Business Intelligence (BI) journey involves transforming raw data into actionable insights through data collection, analysis, and visualization. Visualizations enhance decision-making and ensure that BI aligns with organizational goals, including forecasting customer demands using predictive analytics.
Crafting a comprehensive BI strategy is not only about the technicalities of data management but also about ensuring that every step taken is in harmony with the organization's overarching objectives.
To embark on this journey, one must consider the following steps:
- Assessing business objectives and aligning them with BI capabilities.
- Designing a robust data infrastructure to support data warehousing and storage.
- Implementing advanced analytics capabilities to uncover deep insights.
- Continually evolving the BI strategy to maintain agility and competitiveness.
Remember, a sophisticated BI strategy is not a one-time effort but a continuous process that demands a mix of technical expertise, strategic thinking, and a deep understanding of the business model and goals.
Key Components of Impactful BI
To craft an impactful BI strategy, it's essential to utilize advanced visual analytics and BI tools that cater to your organization's specific needs. These tools should not only aggregate and analyze data but also be user-friendly to encourage widespread adoption.
Interactive visualizations play a crucial role in enhancing decision-making processes and fostering collaboration within organizations. By presenting data in an easily digestible format, stakeholders can extract actionable insights more efficiently.
- Assess business objectives
- Design data infrastructure
- Implement advanced analytics capabilities
These steps are fundamental in transforming raw data into actionable insights, which is the cornerstone of a sophisticated BI strategy. A well-implemented strategy enables businesses to make decisions based on empirical evidence, leading to smarter business moves and reduced risks.
Businesses that leverage a quality BI strategy can uncover hidden opportunities for growth and innovation. This strategic foresight allows for anticipating market shifts and consumer demands, setting the stage for long-term success.
Steps for Successful BI Strategy Execution
Executing a successful BI strategy is a complex endeavor that requires careful planning and attention to detail. Ensure that each step of the strategy is aligned with the organization's overarching goals to maximize the impact of your BI initiatives. The process involves a series of critical actions:
- Assessment of business objectives and data needs: This foundational step ensures that the BI strategy is tailored to the specific goals and requirements of the organization.
- Design of data infrastructure: A robust and scalable infrastructure is key to supporting the BI strategy and accommodating future growth.
- Implementation of advanced analytics: Leveraging analytics capabilities can provide a competitive edge and enhance decision-making.
It is essential to maintain a cycle of continuous improvement, regularly revisiting and refining the BI strategy to stay agile and competitive in a data-driven world.
Remember, the execution of a BI strategy is not a one-time event but an ongoing process. It requires a commitment to evolution and improvement, ensuring that the strategy remains relevant and effective in the face of technological advancements and changing business landscapes.
Future-Proofing Your Analytics Approach
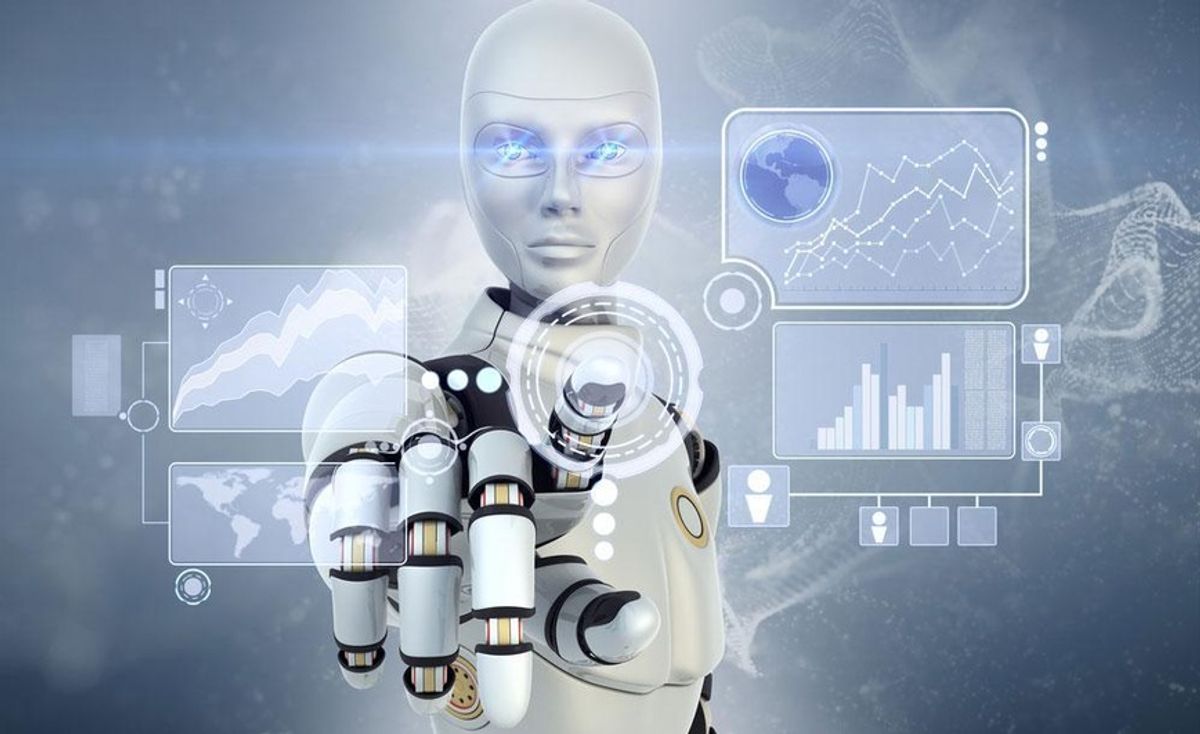
Embracing Change in the Data Landscape
In the ever-evolving world of data, staying ahead requires a proactive approach to change. Organizations must be agile, ready to leverage data analytics for competitive advantage by addressing challenges, upgrading technology, enhancing security, and investing in skilled professionals. Data accuracy is crucial for informed decisions, and as such, regular audits and updates to data governance policies are essential.
By embracing change, companies can ensure their data strategies are not only current but also primed for future advancements.
To maintain relevance in a shifting landscape, consider the following steps:
- Stay vigilant for changes in data regulations and processing technologies.
- Establish a routine for updating policies, processes, and tools.
- Cultivate a data-driven culture to support strategy implementation.
- Prioritize scalability and integration capabilities in your tools.
- Continuously monitor and adapt to align with business objectives and technological advancements.
Innovations in Data Analytics and Management
The landscape of data analytics is continuously evolving, with new technologies enhancing our ability to harness the power of data. Advanced analytics is at the forefront of this transformation, offering unprecedented opportunities for businesses to optimize operations and enhance customer experiences. The integration of emerging technologies can dramatically speed up the analysis process, enabling quick derivation of meaningful insights.
Effective data management is not just about safeguarding data—it’s about unlocking the full potential of your data assets.
Efficient data integration is key, breaking down silos and ensuring seamless processing. Organizations are now leveraging advanced tools to merge diverse data, from relational databases to unstructured data, into a unified system. This is crucial for comprehensive analysis, predictive analytics, and generating actionable insights.
The measure of success in data management strategy is evident in the improvement of decision-making capabilities. With robust data strategies, business users are empowered to make informed, data-driven decisions, reinforcing trust among stakeholders and supporting business growth.
Preparing for the Next Wave of Data Challenges
As we look towards the future, the data landscape continues to evolve at a rapid pace. Organizations must be agile and forward-thinking to adapt to the surprising data trends and challenges that lie ahead. By 2024, data volumes are expected to have grown exponentially, presenting both opportunities and obstacles for businesses seeking to harness the power of big data.
To stay ahead, a robust data management strategy is essential. This strategy should encompass a comprehensive approach to collecting, processing, and leveraging data to enhance quality, bolster security, and transform scattered data into a unified, powerful tool. Here are some key considerations for future-proofing your data capabilities:
- Assess the volume, velocity, and variety of data your organization handles.
- Evaluate the capacity of your current systems to manage these aspects effectively.
- Develop a predictive analytics and AI integration plan to anticipate and act on future trends.
By implementing these strategies, businesses can ensure that their data remains high-quality and useful, accessible, and interpretable by all relevant stakeholders.
Evolving towards a sophisticated business intelligence strategy is not just about managing the present; it's about preparing for the future. Continually advancing your data analytics and intelligence capabilities will help your business remain agile, adaptable, and capable of handling the ever-increasing volumes and complexity of data.
In a rapidly evolving digital landscape, ensuring your analytics systems are robust and adaptable is crucial. At OptimizDBA, we specialize in DATABASE OPTIMIZATION CONSULTING, offering unparalleled data solutions that keep you ahead of the curve. Don't let outdated analytics slow you down. Visit our website to learn how our expertise can accelerate your data processes and future-proof your business. Let's embark on this journey together—your analytics revolution awaits!
Conclusion
Navigating the analytics landscape is a complex but rewarding journey. By understanding which data is relevant and mastering the art of translating it into actionable insights, organizations can uncover hidden opportunities, optimize operations, and enhance customer experiences. The key lies in establishing measurable goals aligned with business strategy, assessing data assets critically, and leveraging advanced analytics to inform strategic decisions. It is essential to ensure that insights are communicated effectively and are accessible to decision-makers, bridging any gaps in data literacy. With the right approach to data analysis, reporting tools, and visualization techniques, businesses can transform raw data into a powerful catalyst for informed decision-making and strategic growth.
Frequently Asked Questions
What are the key data sources for analytics?
Key data sources for analytics include internal databases, customer interactions, social media, sensors/IoT devices, transaction records, and third-party data services. Identifying which data is relevant to your business objectives is crucial for deriving actionable insights.
How can one ensure data quality and integrity?
Ensuring data quality and integrity involves implementing robust data validation processes, regular audits, and cleansing routines. It also requires establishing clear data governance policies to maintain accuracy, consistency, and reliability of data.
What is data governance and why is it important?
Data governance is the framework for managing data availability, usability, integrity, and security in an organization. It's important because it ensures compliance with regulations, protects against data breaches, and maintains trust in data for decision-making.
How do you craft an effective data strategy roadmap?
Crafting an effective data strategy roadmap involves setting clear, measurable objectives aligned with business goals, integrating predictive analytics and AI, and continuously monitoring and adjusting the strategy to ensure it meets the evolving needs of the business.
What is the role of predictive analytics in business strategy?
Predictive analytics plays a key role in business strategy by using historical data to forecast future trends, behaviors, and outcomes. This allows businesses to anticipate market changes, optimize operations, and make informed strategic decisions.
How do you measure the impact of analytics on a business?
The impact of analytics on a business is measured by defining success metrics, benchmarking performance, and assessing the return on investment (ROI). This involves tracking the effectiveness of data-driven decisions in achieving business objectives and improving operations.
What are the best practices for communicating insights to stakeholders?
Best practices for communicating insights to stakeholders include using effective reporting techniques, creating insightful dashboards that highlight key metrics, and training leaders to understand and use data-driven insights in their decision-making processes.
How can an organization future-proof its analytics approach?
An organization can future-proof its analytics approach by embracing change, staying informed about innovations in data analytics and management, and preparing to adapt to the next wave of data challenges. This includes investing in continuous learning and technology upgrades.