Understanding the Basics of Data Analytics
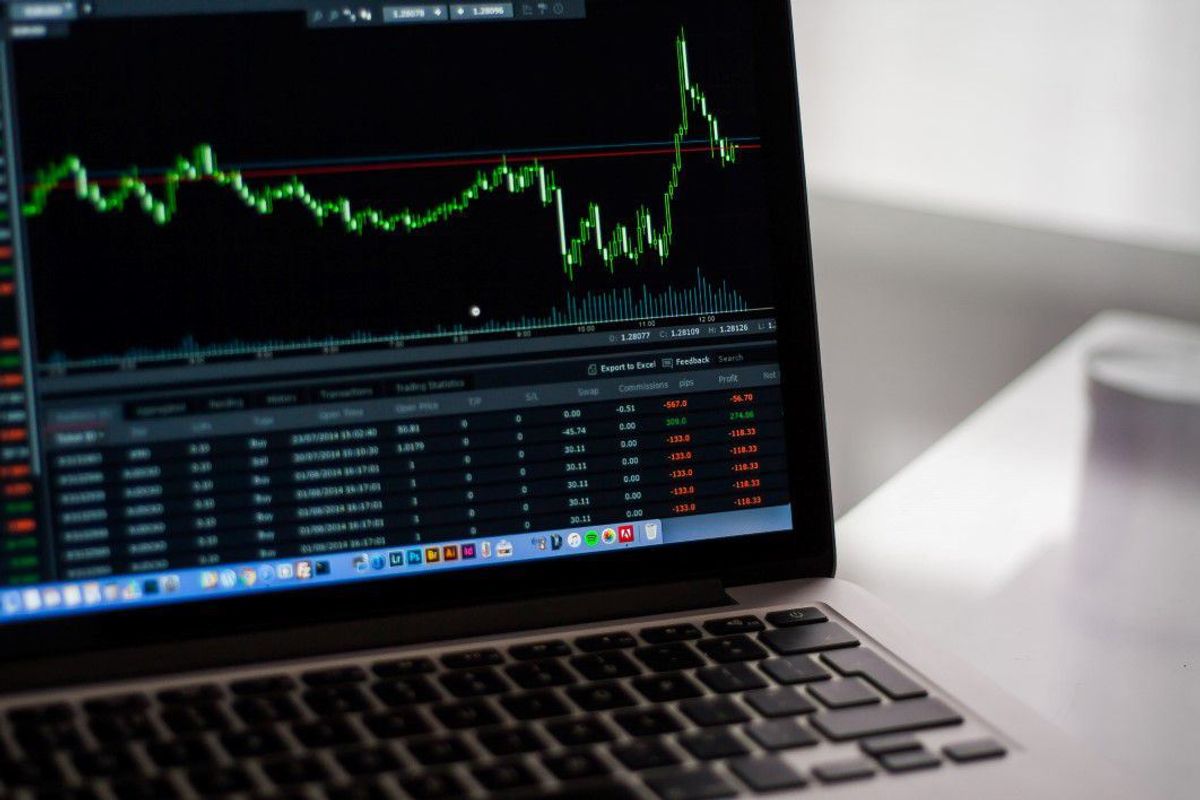
Defining Data Analytics and Its Importance
Data analytics is not a novel concept, but its growing prominence has cast it into the limelight. At its core, data analytics is the systematic approach to collecting, interpreting, transforming, and analyzing data with the goal of extracting valuable insights for informed decision-making. It encompasses a variety of tools and techniques that cater to different data forms, ranging from structured and semi-structured to unstructured data.
Data analytics serves as the cornerstone for businesses to decipher market trends and customer preferences, as well as to analyze complex data sets. It is the key to unlocking the potential hidden within the vast amounts of data that organizations collect.
The importance of data analytics can be likened to the role of oil in the 21st century, with analytics acting as the combustion engine that powers decision-making processes. It is essential to recognize that mere awareness of data analytics is insufficient; its strategic implementation is what drives business success. To further understand the breadth and depth of data analytics, let's delve into its various categories and the latest trends shaping its evolution.
The Five Types of Data Analytics Explained
In the realm of data analytics, understanding the distinct types is fundamental to leveraging data effectively. Descriptive analytics is the most basic form, providing a historical overview of what has happened in your business. It's akin to looking in the rearview mirror; you see the path you've traveled, but not where you're going.
Diagnostic analytics takes a step further by not only showing what happened but also why it happened. It involves drilling down into data to uncover patterns and relationships. This type of analytics is like a detective work, piecing together clues to form a coherent narrative of past events.
Predictive analytics, on the other hand, uses historical data to forecast future events. It employs statistical modeling and machine learning to identify the likelihood of future outcomes, making it an invaluable tool for planning and strategy. Prescriptive analytics goes even further by suggesting actions to benefit from predictions and mitigate risks.
Lastly, real-time analytics provides instant insights by analyzing data as it's being generated. This immediacy can be crucial for time-sensitive decisions, such as in financial trading or emergency response scenarios.
Understanding the types of analytics - descriptive, diagnostic, predictive, and prescriptive - is crucial for data-driven decision-making. Data analytics transforms raw data into actionable insights for informed decisions.
Each type of analytics plays a unique role in the data-driven decision-making process, and businesses must choose the right combination to meet their specific needs.
Transforming Raw Data into Actionable Insights
In the vast sea of data that modern businesses navigate daily, the ability to transform raw data into actionable insights is akin to finding a compass that guides decision-making. Descriptive analytics serves as the starting point, translating numbers and trends into a narrative that explains what has happened. This foundational step is crucial for businesses to understand their past performance and customer behaviors.
The journey from data to insight is not just about having the right tools; it's about asking the right questions and interpreting the answers effectively.
Diagnostic analytics takes the role of a detective, delving deeper to uncover the why behind events. By examining the causes of past outcomes, businesses can refine their strategies and enhance customer experiences. Here's a simple framework to consider when transforming data into insights:
- Collect and organize data from various sources.
- Apply descriptive analytics to summarize past events.
- Use diagnostic analytics to explore causes and correlations.
- Translate findings into actionable strategies for improvement.
Embracing these steps enables organizations to not only react to historical data but also to anticipate future trends, thereby gaining a competitive edge and boosting overall efficiency.
Embracing the Top Data Analytics Trends
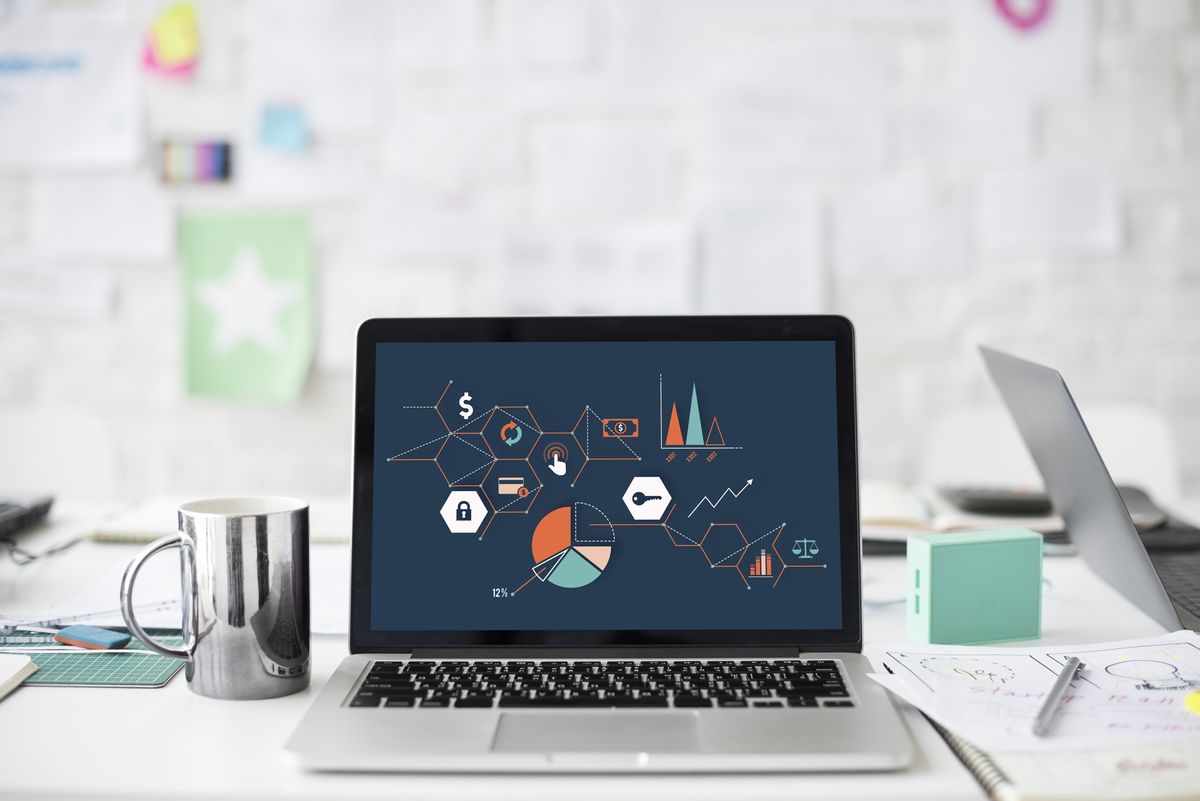
Staying Ahead with Emerging Trends
In the ever-evolving landscape of data analytics, staying ahead means being proactive about embracing new trends. Data democratization is one such trend that is reshaping how businesses approach analytics. By making data more accessible, organizations empower their non-technical users to engage with analytics tools, fostering a culture of informed decision-making across all levels.
Data quality and governance are also at the forefront, ensuring that the insights derived are reliable and actionable. Innovative technologies are not just a buzzword but a strategic necessity, enabling businesses to sift through the data deluge and pinpoint what truly matters for growth and competitiveness.
The rapid evolution of technology has put the gamut of data analytics under massive shifts, demanding businesses to be observant and adaptable.
To effectively navigate these trends, consider the following points:
- Monitor the dynamic nature of data analytics and be prepared to pivot strategies.
- Evaluate and integrate emerging technologies that align with your business goals.
- Prioritize data governance to maintain the integrity and security of your analytics.
- Encourage a culture of continuous learning to keep pace with technological advancements.
Top Trends to Watch in Data Analytics
As we navigate the data landscape in 2024, it's crucial to stay informed about the trends that are shaping the future of data analytics. Generative AI is one such trend that's gaining momentum, offering the ability to create new, synthetic data sets that can enhance predictive modeling and decision-making. Another trend to watch is the emergence of data contracts, which are agreements that stipulate how data is to be used, ensuring compliance and ethical handling of sensitive information.
- Generative AI: Revolutionizing predictive analytics and content creation.
- Data Contracts: Establishing clear guidelines for data usage and privacy.
Embracing these trends not only keeps your business at the forefront of innovation but also ensures that you are prepared for the dynamic shifts in the data analytics domain. As the volume of data continues to grow, these trends will become increasingly important in shaping business strategies and maintaining a competitive edge.
How Trends Influence Business Strategies
The dynamic landscape of data analytics trends plays a pivotal role in shaping business strategies. Businesses must adapt to these trends to stay competitive and make informed decisions. For instance, embracing predictive analytics can forecast customer behavior and market trends, allowing companies to proactively adjust their strategies.
- Predictive Analytics: Anticipate future trends and customer behavior
- Prescriptive Analytics: Recommend actions based on data
- Descriptive Analytics: Understand past performance
- Diagnostic Analytics: Investigate reasons behind past outcomes
- Cognitive Analytics: Enhance decision-making with AI
By integrating these analytics trends into business operations, companies can leverage data to drive growth, innovation, and efficiency.
It is essential to not only keep pace with these trends but also to ensure that the chosen analytics solutions align with the company's long-term goals. Leveraging data analytics for competitive advantage involves addressing challenges, upgrading technology, enhancing security, and investing in skilled professionals. Data accuracy and integrity are crucial for informed decisions.
Building a Scalable Analytics Framework
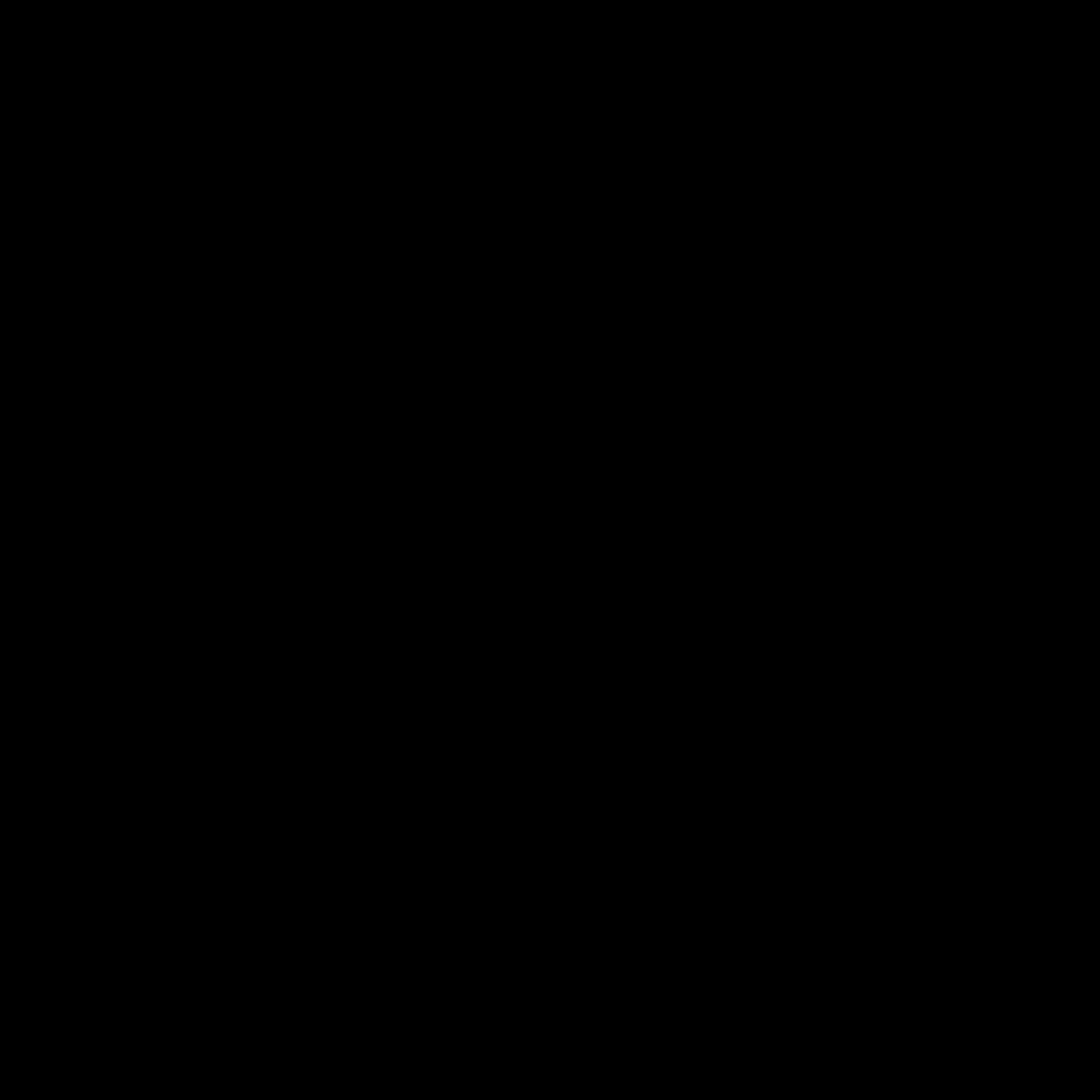
Planning for Business Growth and Data Expansion
As your business scales, the volume and complexity of data you handle will inevitably increase. Choosing the right Customer Data Platform (CDP) is crucial to manage this growth effectively. A CDP that scales with your business needs can integrate increasing volumes of data and support more sophisticated analytics over time.
When planning for data expansion, consider these key factors: Scalability to handle growing data demands An intuitive, user-friendly interface for all skill levels Robust support and training for seamless onboarding
Additionally, it's important to identify quick wins and set high-level milestones as part of your data strategy roadmap. This approach ensures that you're not only prepared for current data challenges but also equipped for future growth.
Remember, the value of a CDP extends beyond its initial cost. Evaluate the long-term benefits and ROI it will bring to your organization, ensuring that your investment will support your business objectives and drive data-driven decision making.
Choosing the Right Customer Data Platform
Selecting the appropriate Customer Data Platform (CDP) is crucial for harnessing the full potential of your business's data. Understand your data needs first to ensure the platform aligns with your business objectives. A CDP should create a unified customer profile by aggregating data from multiple sources, providing real-time, actionable insights.
Consider the following points when choosing a CDP:
- Scalability to grow with your business needs
- A user-friendly interface for ease of use
- Adequate support and training for your team
Remember, the right CDP is one that not only meets your current needs but also has the capacity to evolve as your business and data strategies develop.
Ultimately, the choice of a CDP should be guided by your specific business goals. Whether it's gaining a holistic view of customer behavior, fostering deeper customer relationships, or targeting broad-scale advertising, the platform you choose must be capable of supporting these objectives effectively.
Ensuring Flexibility in Your Analytics Solutions
In the rapidly evolving landscape of data analytics, flexibility is key to staying competitive. As businesses grow, their data needs become more complex, necessitating analytics solutions that can adapt and scale accordingly. A scalable analytics framework is not just a nice-to-have; it's a critical component of a future-proof business strategy.
- Consider scalability: Ensure your analytics solution can grow with your business, handling increased data integration and complexity.
- Prioritize usability: Choose a platform with an intuitive user interface, catering to users of all technical levels.
- Support and training: Look for solutions that offer robust support and training options to empower your team.
Agility in your analytics solutions allows for quick adaptation to new trends and technologies, ensuring that your business remains at the forefront of innovation. Companies must foster a data-driven culture, address hurdles like data quality, and align analytics with business goals. DataOps and agility are crucial for leveraging analytics effectively.
Remember, the trends in data analytics are dynamic, and what works today may not suffice tomorrow. By ensuring your analytics solutions are flexible, you can navigate these changes and maintain a competitive edge.
Optimizing User Experience in Analytics Platforms
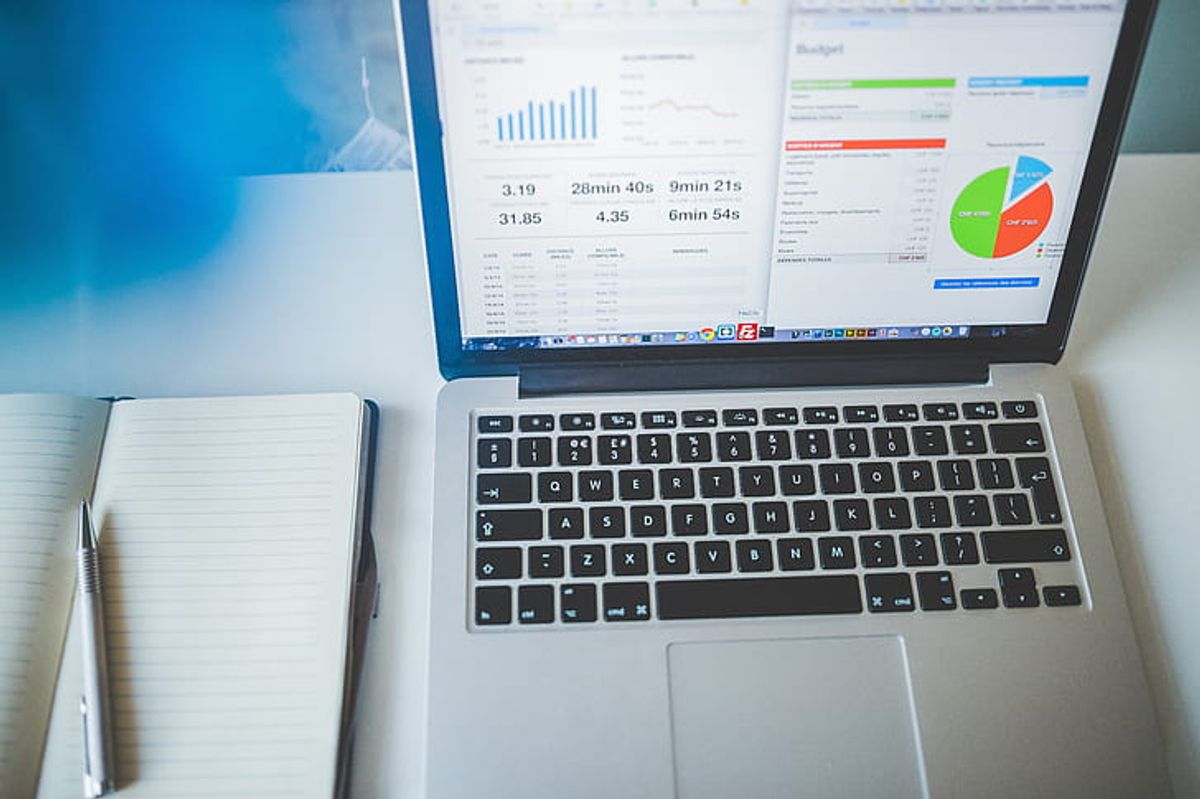
The Importance of a User-Friendly Interface
In the realm of data analytics, the power of a platform is often overshadowed by its complexity. A user-friendly interface is crucial; it ensures that all users, regardless of their technical expertise, can effectively interact with the analytics tools. This accessibility is not just about comfort—it's about efficiency and the ability to leverage data insights across the organization.
Usability is a key factor when selecting an analytics platform. A system that is intuitive and easy to navigate minimizes the need for extensive training and reduces the resources dedicated to troubleshooting and support. Here are some considerations for a user-friendly analytics interface:
- Clear and logical layout of features
- Simple and consistent navigation menus
- Visual cues for common tasks
- Responsive design for various devices
By prioritizing user experience (UX), businesses can facilitate quicker adoption and more meaningful engagement with their analytics systems.
Remember, the goal is to empower users to make data-driven decisions without being hindered by the tool they are using. A platform that balances functionality with simplicity can become a catalyst for a data-informed culture within your organization.
Training and Support for Non-Tech-Savvy Users
In the realm of data analytics, the provision of comprehensive training and support is crucial for users who may not have a technical background. It's essential to prioritize analytics platforms that offer robust training programs and ongoing support to ensure a smooth transition and effective use of the platform.
- Onboarding: Initial training sessions to familiarize users with the platform.
- Documentation: Accessible guides and manuals for self-help.
- Support: Dedicated helpdesk for troubleshooting and assistance.
- Continuous Learning: Regular updates and workshops to keep users adept with new features.
Ensuring that all users are comfortable and capable with the analytics tools not only empowers them to make data-driven decisions but also fosters a culture of data literacy within the organization.
By implementing data cleaning, visualization tools, and advanced analytics techniques, organizations can gather reliable insights and make informed decisions based on accurate data. This approach is particularly beneficial for non-tech-savvy users, as it simplifies the complexity of data analytics, allowing them to focus on deriving actionable insights.
Balancing Power and Simplicity in Analytics Tools
In the realm of business analytics, the quest for powerful capabilities must be harmoniously balanced with the need for simplicity. Business analytics involves advanced techniques like regression analysis, machine learning, and AI. However, the true art lies in crafting tools that are both potent and accessible to users of varying expertise levels.
The goal is to empower users to harness the full potential of analytics without feeling overwhelmed by complexity.
To achieve this balance, consider the following points:
- Prioritize a user-friendly interface that simplifies interaction with complex data sets.
- Ensure that support and training are readily available to facilitate user adoption and proficiency.
- Opt for solutions that offer scalability, allowing for growth and increased data integration as business needs evolve.
Remember, a tool that is too complex may deter users, while one that is too simple may not provide the depth of insight required for informed decision-making. Data governance, security, visualization, and decision guidance are crucial for deriving meaningful insights and guiding strategic actions.
Leveraging Real-Time, Data-Driven AI Analytics
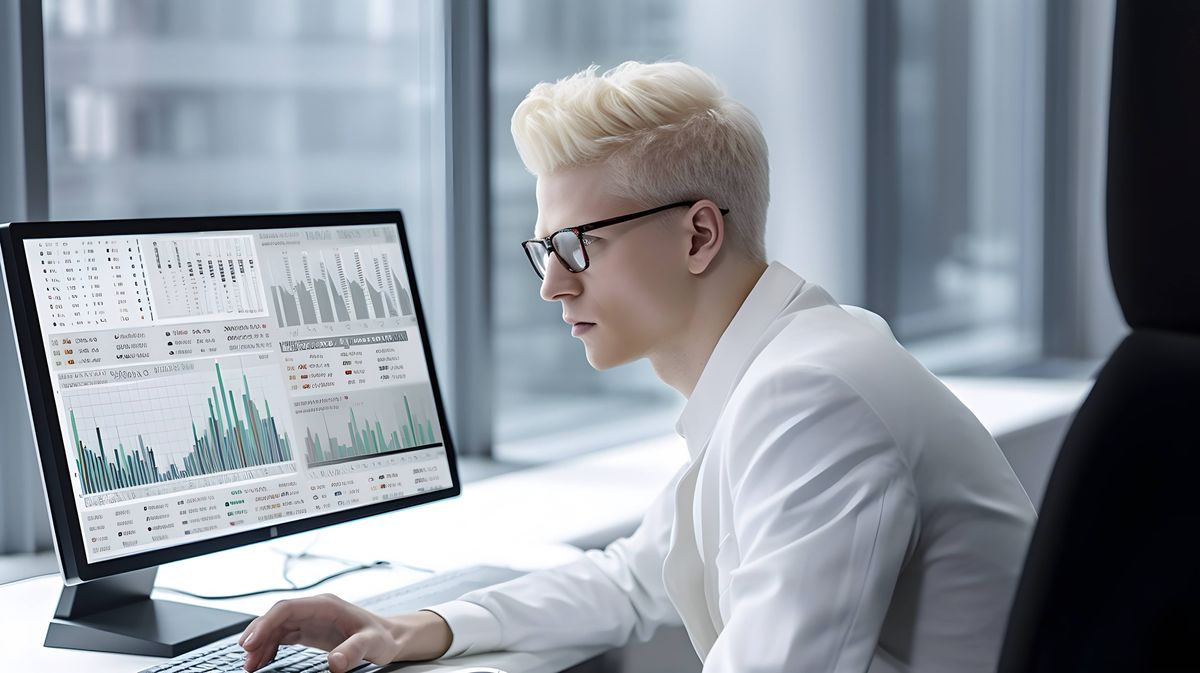
The Role of AI in Modern Data Analytics
The integration of Artificial Intelligence (AI) into data analytics has been a game-changer, enabling businesses to process and analyze vast amounts of data with unprecedented speed and accuracy. AI-powered analytics are essential for business agility and success in a data-driven landscape. Real-time data analytics, integration of AI tools, and data democratization are key for competitive advantage and future-proofing businesses.
AI is not just about automating tasks; it's about augmenting human capabilities and providing deeper insights. Machine learning models, a subset of AI, can autonomously monitor data, identify patterns, and predict outcomes without constant human oversight. This shift towards AI-driven analytics means that businesses can be more proactive and less reactive in their decision-making processes.
The potential of AI in data analytics is vast, with applications ranging from predictive maintenance to customer behavior analysis. By leveraging AI, companies can unlock new opportunities and drive innovation.
Here are some of the key AI trends in data analytics:
- AI-powered data analytics
- Data-centric AI
- Metadata-driven data fabric
- Augmented analytics
- Advanced natural language processing (NLP)
- Evolution of predictive analytics
- Integration of extended reality (XR) in analytics
- Continuous emphasis on data ethics and privacy
- Data democratization
Implementing Real-Time Analytics for Instant Insights
In the fast-paced business environment, real-time analytics is a game-changer. It enables organizations to process and analyze data as it's being generated, providing instant insights that can lead to more informed decision-making and a significant competitive advantage.
The integration of real-time analytics into business intelligence (BI) systems allows companies to react swiftly to changing market conditions and customer behaviors. This agility is crucial for optimizing processes and staying ahead of the curve.
- Identify critical data sources
- Establish data processing pipelines
- Implement analytics algorithms
- Visualize insights for immediate action
By seamlessly incorporating real-time analytics, businesses can pivot quickly, ensuring that strategies and operations are always aligned with the latest data.
Employing real-time analytics is not just about speed; it's about the capacity to harness the power of analytics in BI, transforming raw data into actionable strategies that drive growth.
Integrating AI to Enhance Business Intelligence
The integration of artificial intelligence (AI) into business intelligence (BI) systems is transforming the landscape of data analytics. AI-powered analytics are enabling businesses to automate complex tasks, uncover subtle patterns, and generate deeper insights. This evolution is particularly evident in the rise of machine learning (ML) models that can independently monitor data streams, identify anomalies, and predict future trends without the constant oversight of IT analysts.
- AI-powered data analytics
- Data-centric AI
- Metadata-driven data fabric
- Edge computing
- Augmented analytics
- Advanced natural language processing (NLP)
- Evolution of predictive analytics
- Integration of extended reality (XR) in analytics
- Continuous emphasis on data ethics and privacy
- Data democratization
By strategically implementing AI, businesses can optimize operations and unlock new avenues of success. The key is to navigate the integration process with a comprehensive understanding of both the potential and the challenges involved.
Cognitive analytics, which leverages AI and ML, moves beyond rigid spreadsheets and numerical grids. It delves into the unstructured world of text, images, and other complex data forms, automating data processing and generating in-depth insights. As businesses embrace these advanced capabilities, they can decode market trends, understand customer preferences, and analyze large, mixed data clusters more effectively.
Navigating the Challenges of Data Analytics Implementation
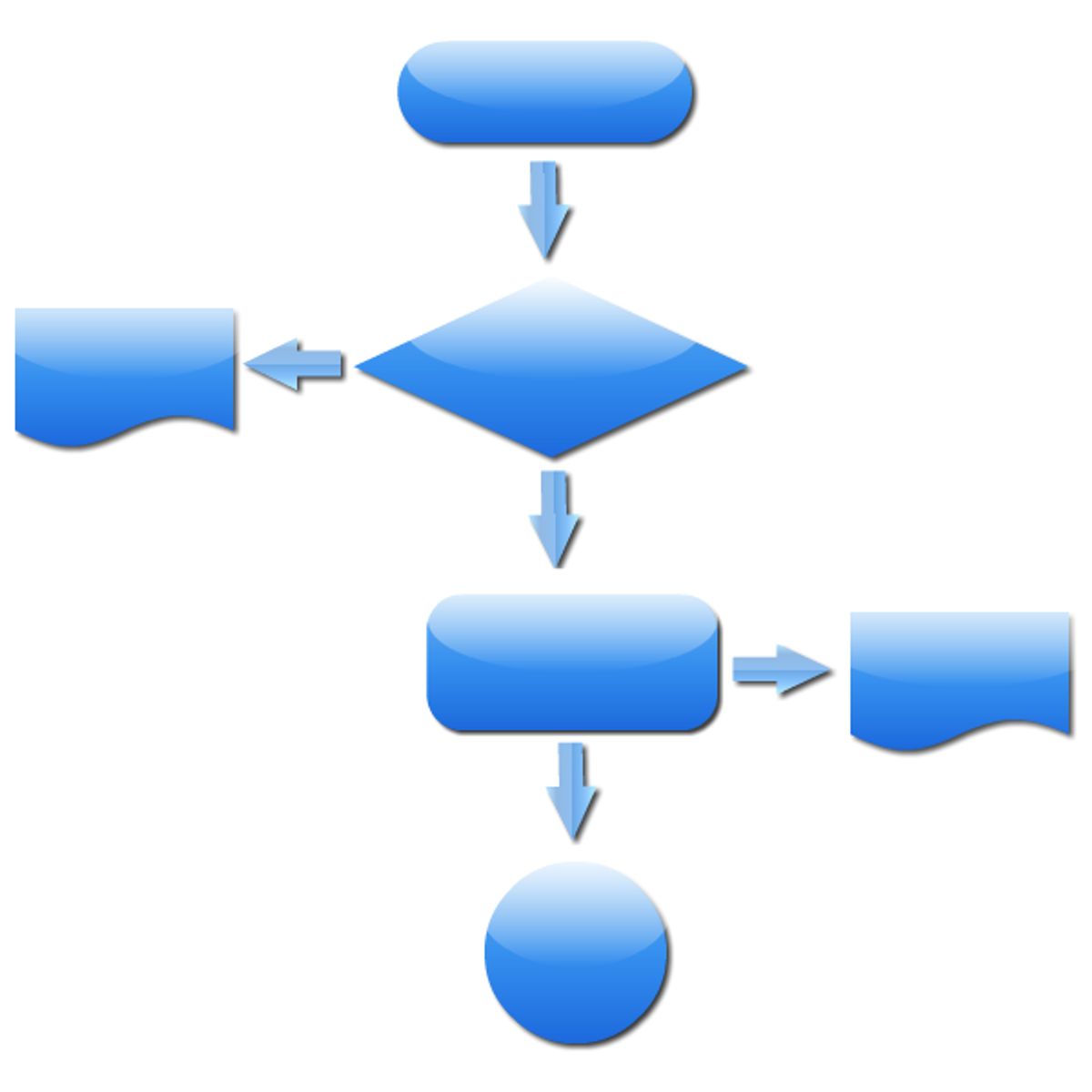
Overcoming Common Data Analytics Obstacles
In the journey of implementing data analytics, businesses often encounter a range of obstacles that can hinder progress. Identifying and addressing these challenges early on is crucial for a successful analytics strategy. One common hurdle is ensuring data quality, which is foundational for reliable insights. Without high-quality data, analytics efforts can lead to misguided decisions and wasted resources.
Another significant challenge is fostering data literacy within the organization. Employees at all levels need to understand and engage with data analytics to fully leverage its benefits. This requires not only the right tools but also ongoing education and support.
To effectively overcome these obstacles, it's essential to integrate analytics into regular business processes and decision-making. This integration ensures that insights are not just a one-time effort but a continuous driver of improvement and innovation.
Lastly, businesses must balance the insights gained from data analytics with intuitive decision-making. While data can provide a wealth of information, it's important to remember that it's one piece of the puzzle. Decision-makers should use data as a tool to inform, not dictate, their choices.
Here are the key steps for data-driven decision-making:
- Establish clear objectives
- Secure leadership buy-in
- Select the right technology
- Implement robust data management practices
- Balance insights with intuition
The benefits of such an approach include increased agility, accuracy, and efficiency in business operations. However, challenges such as data quality, literacy, and the integration of decisions into regular analysis must be navigated carefully.
Ensuring Data Quality and Accuracy
In the realm of business analytics, ensuring data quality and accuracy is paramount. Without it, even the most sophisticated analytics tools are rendered ineffective. Addressing data quality and integrity challenges requires a multifaceted approach:
- Utilizing data cleansing tools to scrub and correct datasets.
- Establishing a common data model to maintain consistency.
- Achieving real-time visibility into data streams.
- Implementing governance rules to enforce data standards.
Ensuring the integrity of your data is not just about maintaining its quality; it's about laying the foundation for advanced analytics that can provide a competitive advantage.
Adherence to data privacy rules and the implementation of robust data governance frameworks are critical. These practices not only protect your business from regulatory repercussions but also build trust with your customers. As your business grows, consider scalability and the ability to integrate more complex analytics to meet evolving requirements.
Addressing Privacy and Security Concerns
In the realm of data analytics, privacy and security are paramount. As businesses collect and analyze increasing volumes of data, they must navigate a complex landscape of regulations and ethical considerations. Ensuring compliance with laws such as GDPR and CCPA is not just about avoiding legal repercussions; it's about building trust with customers by protecting their sensitive information.
- Compare data privacy & compliance features when selecting tools and platforms.
- Implement robust data governance frameworks to manage risks.
- Prioritize responsible data practices and adhere to privacy rules.
Businesses must continuously adapt to the evolving data protection landscape to maintain customer trust and avoid policy violations.
Addressing common challenges in big data analytics privacy requires a proactive approach. Insights on policy violations, breaches, compliance, and effective solutions are essential for enterprises to navigate the data deluge responsibly.
Harnessing the Power of Data Analytics for Competitive Advantage
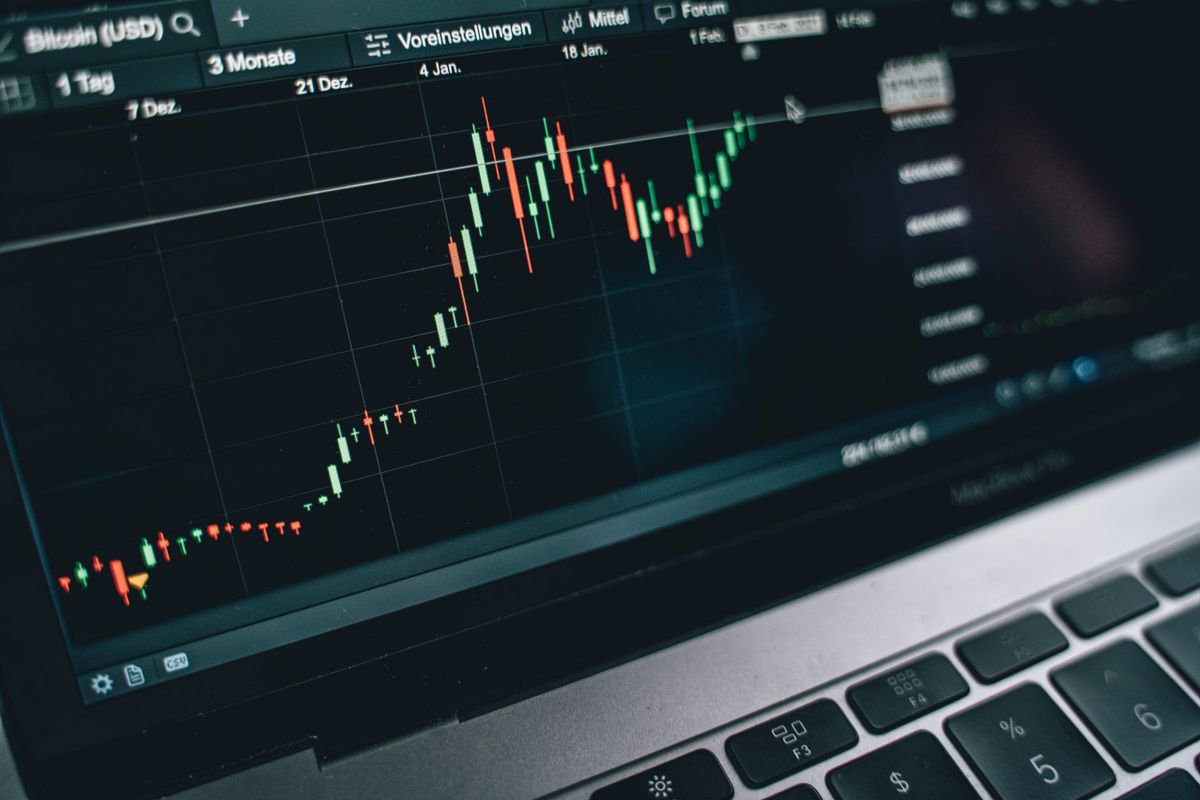
Gaining Insights for Strategic Decision Making
Data-driven decision making is essential for modern businesses. By leveraging business intelligence tools, predictive analytics, and data storytelling, organizations can make informed decisions that drive strategic outcomes.
Business intelligence tools, in particular, play a pivotal role in transforming data into insights. They allow for the analysis of large volumes of data to identify trends, patterns, and opportunities. Predictive analytics goes a step further by not just interpreting data, but also by forecasting future events and behaviors, enabling businesses to anticipate market changes and customer needs.
Data storytelling is a powerful technique that translates complex data findings into compelling narratives, making the insights accessible and actionable for all stakeholders.
To effectively gain insights for strategic decision making, consider the following steps:
- Collect relevant data from various sources.
- Use analytics tools to process and analyze the data.
- Interpret the results to understand the implications for your business.
- Communicate the findings clearly through data storytelling.
- Implement the insights into your business strategy for optimal results.
Benchmarking Against Competitors Using Data
In the competitive landscape of business, benchmarking against competitors using data is a critical strategy for staying ahead. By analyzing competitors' performance metrics, companies can identify areas for improvement and set realistic goals. A structured approach to benchmarking involves several key steps:
- Identifying key performance indicators (KPIs) relevant to your industry
- Collecting data on competitors' performance in these areas
- Comparing your performance against this data
- Setting actionable targets based on insights gained
It's not just about collecting data, but about transforming that data into a strategic asset that informs decision-making and drives growth.
While the process can be complex, the value of data-driven benchmarking is clear. It allows businesses to focus on competitive advantages and allocate resources more effectively. Remember, the goal is not to copy competitors but to outperform them by understanding the market dynamics and customer preferences better.
Improving Customer Experience with Analytics
In today's competitive landscape, leveraging analytics to enhance customer experience (CX) is not just an option, but a necessity. By harnessing the power of customer data platforms (CDPs) and AI, businesses can deliver personalized experiences that resonate with individual preferences and behaviors.
Analytics play a crucial role in understanding customer journeys and identifying key touchpoints for improvement. For instance, analyzing chatbot metrics and customer feedback can reveal insights into user satisfaction and areas where the digital experience may be lacking.
- Understand customer needs through data analysis
- Personalize interactions based on customer data
- Optimize customer journeys for better engagement
By focusing on data-driven strategies, companies can ensure that every customer interaction is informed and intentional, leading to increased satisfaction and loyalty.
To truly improve CX, it's essential to leverage the right data and consider multiple perspectives to drive business strategy. Data analytics not only optimizes operations but also personalizes customer experiences, fostering brand loyalty and growth.
Future-Proofing Your Business with Data Analytics
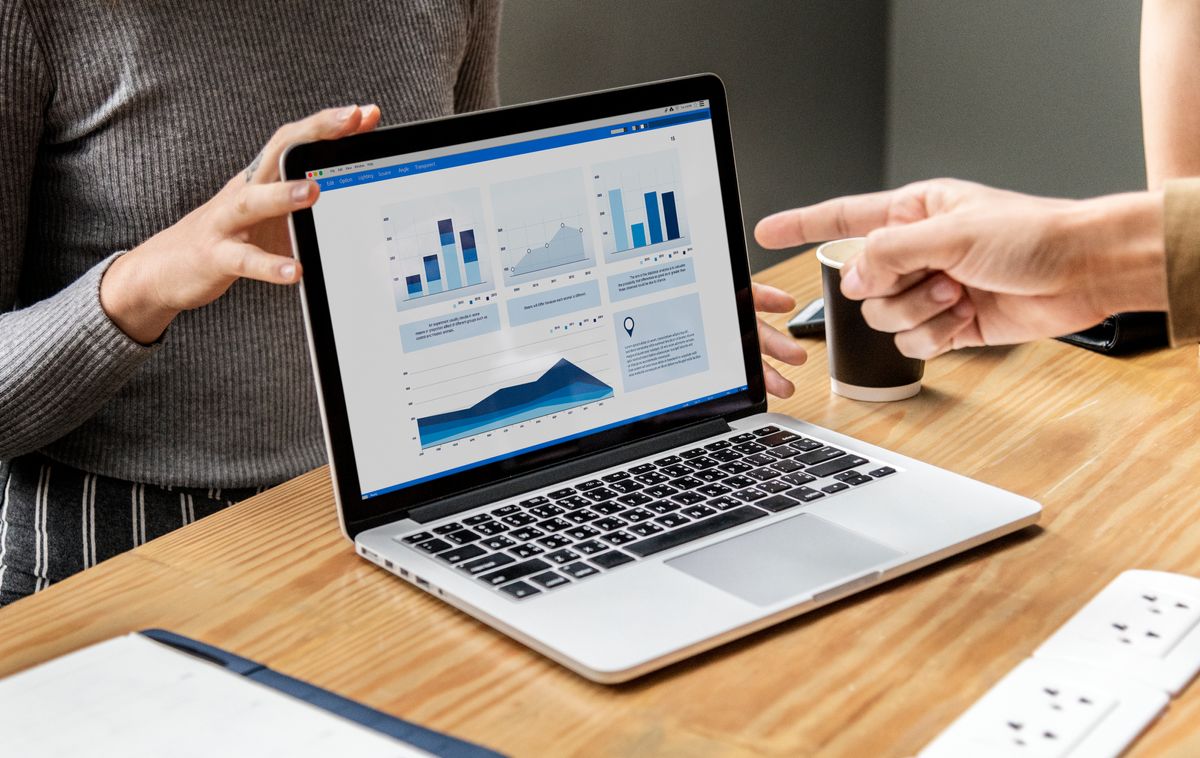
Adapting to the Dynamic Nature of Data Trends
In the ever-changing landscape of data analytics, businesses must adapt to the digital age by embracing new trends that emerge. The dynamics of data trends require a proactive approach to stay ahead. For instance, consider the following key areas where adaptation is crucial:
- Understanding and leveraging the latest data analytics technologies
- Integrating advanced analytics into business processes
- Fostering a culture that values data integration and continuous learning
To remain competitive, it is essential to not only adopt these trends but also to continuously evaluate and refine them as the industry evolves.
However, you must be aware that, like most technological fads, the trends in data analytics don't stay static. This domain is dynamic in nature, and it demands one to be observant. What are the most important concepts and solutions in the field of data and analytics in the upcoming year? Which innovative methods and technologies are businesses adopting, and why? These questions are pivotal for growth and efficiency.
Preparing for Technological Shifts in Analytics
The landscape of data analytics is perpetually evolving, with new technologies and methodologies emerging at a rapid pace. To stay competitive, businesses must not only keep abreast of these changes but also be prepared to integrate them into their existing systems. Adaptability is key to ensuring that your analytics infrastructure can accommodate future technological shifts without major disruptions.
Integration of data analytics should be strategic, focusing on identifying key areas where new technologies can have the most impact. Utilizing visualization tools and prioritizing data relevance will enhance the understanding and utility of analytics. Collaboration and communication within the team are crucial for fostering innovation and achieving efficiency in business analytics.
By proactively planning for technological shifts, businesses can ensure that their analytics capabilities remain robust and relevant, providing them with the insights needed to make informed decisions in a dynamic market.
Staying Informed on the Evolving Data Landscape
In the swiftly changing realm of data analytics, staying informed is not just beneficial; it's a necessity for survival. The data deluge shows no signs of slowing, and those who keep pace with the evolving landscape will find themselves at the forefront of innovation and success. The trends in data analytics are dynamic, constantly being reshaped by new technologies and methodologies.
To remain competitive and agile, businesses must monitor and adapt to these shifts. Here's a snapshot of the top trends that are currently shaping the future of data analytics:
- AI-powered data analytics
- Data-centric AI
- Metadata-driven data fabric
- Edge computing
- Augmented analytics
- Advanced natural language processing (NLP)
- Evolution of predictive analytics
- Integration of extended reality (XR) in analytics
- Continuous emphasis on data ethics and privacy
- Data democratization
Embracing these trends is crucial for integrating analytics into your business strategy effectively. Upskilling your team and adhering to ethical practices will not only give you a competitive advantage but also ensure that your business thrives in 2024 and beyond.
Cultivating a Data-Driven Culture in Your Organization
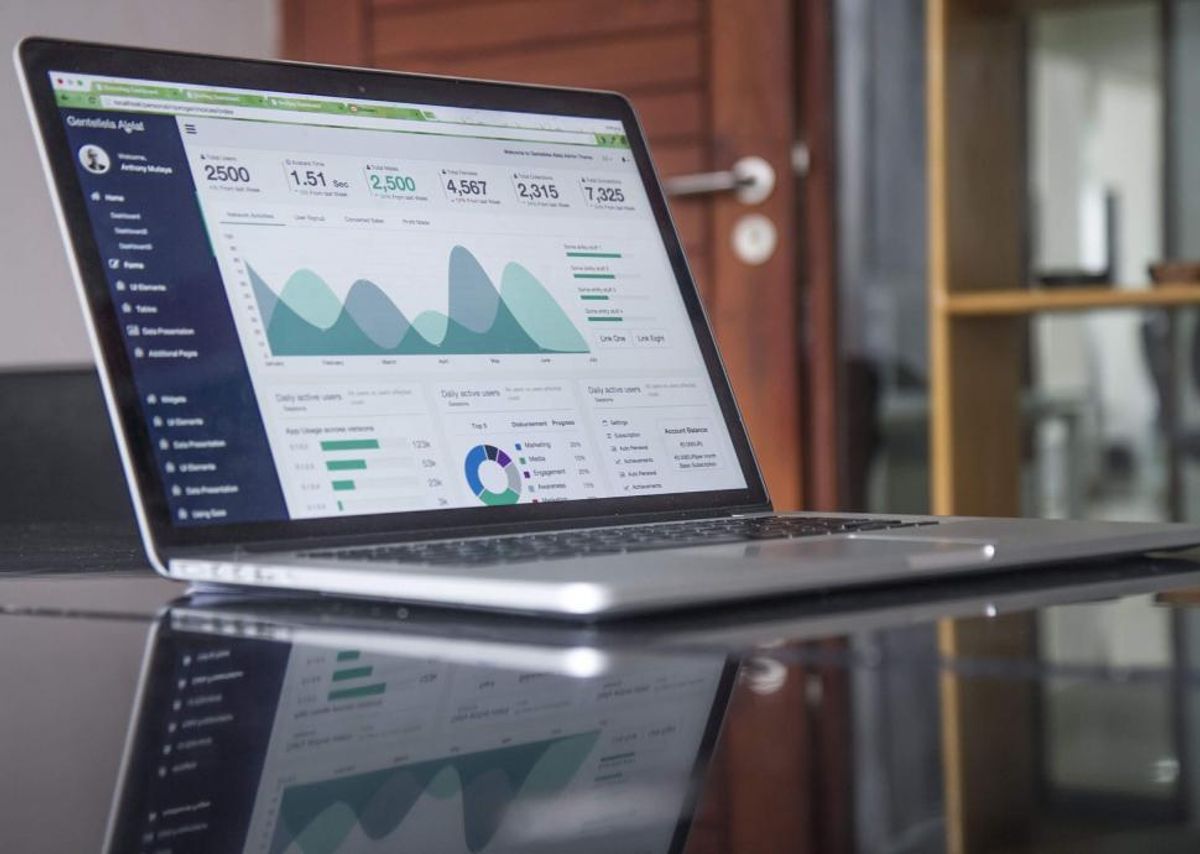
Fostering an Environment for Data Literacy
In the age of data democratization, it's essential for businesses to cultivate an environment where data literacy is a foundational skill for all employees. Data literacy encompasses the ability to access, comprehend, use, evaluate, and extract insights from data, regardless of one's role in the organization. To achieve this, a conscious strategy and unwavering commitment from leadership are imperative.
Data democratization aims to make the benefits of data analytics accessible to all users, irrespective of their technical expertise. This is facilitated by the advent of self-service analytics tools that empower employees to make data-driven decisions. Here are some steps to foster data literacy:
- Establish a baseline of data knowledge for all employees.
- Provide ongoing training and resources.
- Encourage a culture of data-driven inquiry and experimentation.
- Recognize and reward data-driven achievements.
By embedding data literacy into the company's culture, businesses ensure that every team member, from senior executives to front-line employees, can effectively participate in the organization's data-driven journey.
Encouraging Collaboration Through Data Insights
In the pursuit of a collaborative data-driven culture, it's essential to ensure that all members of an organization, from senior executives to front-line employees, are empowered to access and interpret data. Breaking down data silos is a critical step in this process, as it allows individuals to confidently find and utilize the information they need.
By adopting advanced tools for data visualization and analytics, employees are not only able to comprehend complex data sets but also to contribute valuable insights that drive collective decision-making.
Here are some key strategies to encourage collaboration through data insights:
- Promote data literacy across all levels of the organization.
- Implement self-service analytics tools to empower users with varying skill levels.
- Foster an environment where data ethics and privacy are paramount.
- Encourage open dialogue and sharing of insights among teams.
These strategies are not just one-time initiatives but require ongoing commitment and leadership support to cultivate a truly data-savvy and inclusive company.
Building a Team Equipped for Data Challenges
In the era of data-driven decision-making, assembling a team capable of navigating the complex data landscape is crucial. Diverse expertise is the cornerstone of such a team, combining skills from data science, business analysis, and information technology. A well-rounded team can interpret data, draw insights, and translate them into actionable business strategies.
Key roles in a data-equipped team might include:
- Data Scientists: For sophisticated model building and predictive analytics.
- Data Analysts: To interpret and visualize data for business insights.
- Business Analysts: Bridging the gap between data insights and business needs.
- IT Specialists: Ensuring the infrastructure supports data initiatives.
Building a team for data challenges is not just about hiring the right people; it's about creating a culture that values data literacy and continuous learning.
Remember, the goal is not only to address current data challenges but also to anticipate future demands. This foresight involves ongoing training and development, ensuring the team remains agile and informed about emerging trends like AI-powered analytics and data democratization. By fostering a collaborative environment, your team can leverage collective knowledge to overcome obstacles and drive innovation.
Measuring the Impact of Data Analytics on Business Outcomes
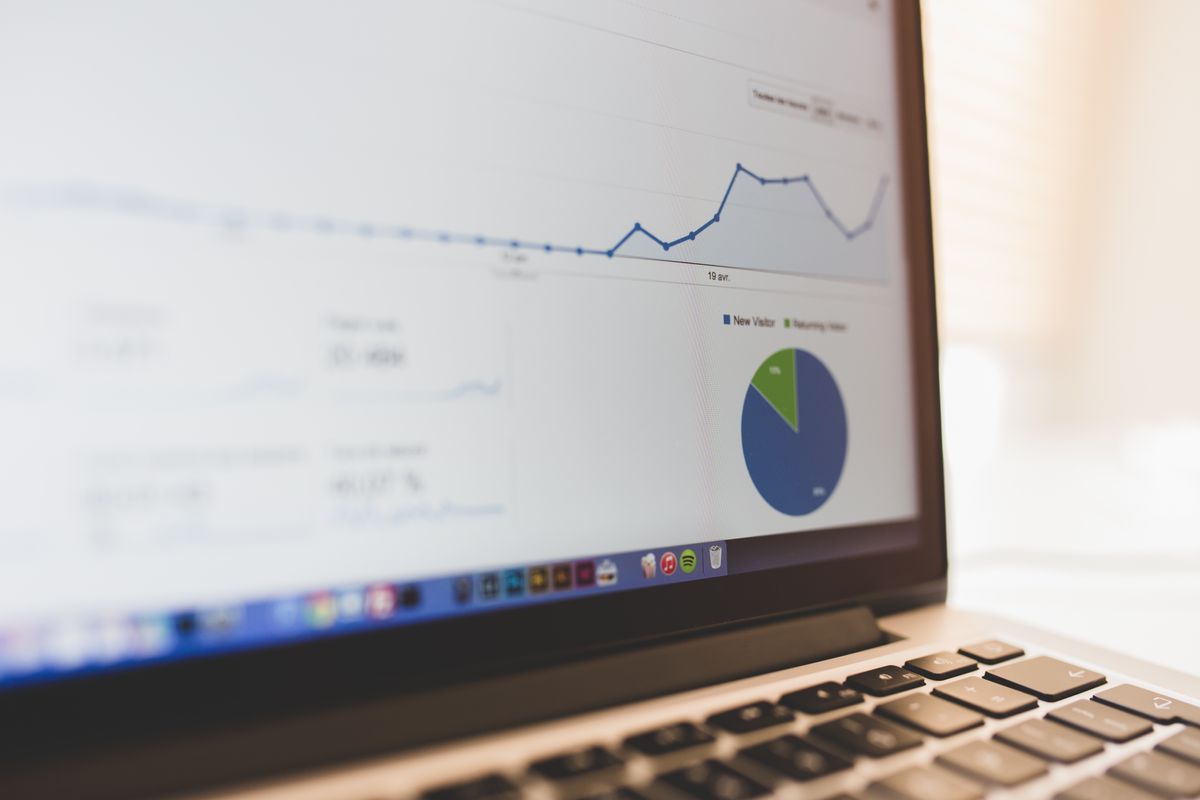
Setting Metrics for Analytics Success
To truly gauge the effectiveness of your analytics initiatives, it's essential to establish clear and measurable metrics. Setting the right metrics is the cornerstone of understanding the impact of your data analytics on business outcomes. These metrics should align with your business goals and provide a quantifiable way to track progress.
One fundamental metric is the return on investment (ROI) for your analytics projects. This involves calculating the benefits gained versus the costs incurred. For marketing strategies, a key metric could be the profitability of campaigns, which helps you determine the success of your marketing efforts by comparing the money spent on marketing to the revenue generated.
Here's an example of how to structure these metrics:
Metric | Description | Target | Actual |
---|---|---|---|
ROI | Return on investment | >100% | TBD |
Profitability | Campaign profitability | Increase | TBD |
Ensuring that these metrics are regularly reviewed and updated is vital for maintaining the relevance and accuracy of your analytics strategy.
By focusing on these metrics, businesses can make informed decisions that drive growth and improve customer experiences. Remember, the goal is not just to collect data, but to transform it into actionable insights that propel your business forward.
Analyzing the ROI of Data Initiatives
Understanding the return on investment (ROI) for data initiatives is crucial for businesses to justify the allocation of resources towards analytics. The key to maximizing ROI lies in the strategic application of insights derived from data analytics. By focusing on areas with the highest potential for impact, companies can optimize their investments and drive meaningful business outcomes.
Italics are often used to highlight the importance of data quality, as it directly influences the accuracy of insights and the subsequent decisions made. A structured approach to measuring ROI involves assessing various metrics that reflect the efficiency and effectiveness of data initiatives:
Metric | Description |
---|---|
Cost Savings | Reduction in expenses due to optimized processes |
Revenue Growth | Increase in sales attributed to data-driven strategies |
Customer Satisfaction | Improvement in customer experience metrics |
Operational Efficiency | Streamlining of workflows and reduction in time-to-market |
By systematically evaluating these metrics, businesses can gain a clear understanding of how data analytics contributes to their bottom line.
However, it's not just about the numbers. Qualitative feedback from stakeholders and customers also provides invaluable insights into the performance of data initiatives. This feedback can help refine strategies and ensure that the analytics solutions in place are truly aligned with business goals.
Continuous Improvement Through Data Feedback Loops
The journey of data analytics is cyclical, not linear. Continuous improvement is achieved by establishing feedback loops that inform and refine each stage of the analytics process. By leveraging these loops, businesses can adapt their strategies in real-time, ensuring that their analytics efforts are always aligned with their evolving goals.
Democratization of data is crucial for this process. It allows individuals at all levels of the organization to engage with data, fostering a culture of informed decision-making. Here are some steps to implement effective data feedback loops:
- Collect data from various sources and touchpoints.
- Analyze the data to extract meaningful insights.
- Share insights across the organization to inform decisions.
- Act on the insights to drive improvements.
- Measure the impact of changes and feed the results back into the cycle.
By nurturing a feedback-rich environment, organizations can maximize the potential of their data, automate processes, and adopt advanced tools for better outcomes.
Remember, the goal is not just to collect and analyze data but to use it as a springboard for innovation and competitive advantage. The data-driven culture that emerges from this approach is a testament to the power of analytics in driving business success.
In today's data-driven landscape, the role of data analytics in shaping business outcomes cannot be overstated. By leveraging cutting-edge data solutions, companies can gain a competitive edge through enhanced decision-making and operational efficiency. If you're looking to transform your business with unparalleled database optimization and experience transaction speeds that defy expectations, visit OptimizDBA for a consultation. Our proprietary methodologies and proven track record since 2001 ensure that your data works for you, not against you. Don't just take our word for it; discover how our expertise has propelled over 500 clients to new heights. Take the first step towards data excellence and contact us today!
Conclusion
As we've navigated the vast seas of data analytics together, it's clear that the ability to effectively manage and analyze data is no longer a luxury but a necessity for businesses aiming to thrive in a competitive landscape. The insights gleaned from this article should serve as a beacon, guiding you through the data deluge towards informed decision-making and strategic growth. Remember, the journey doesn't end here; it's an ongoing process of adaptation and learning. Keep exploring, stay ahead of the trends, and let data analytics be the engine that powers your business forward into a prosperous future.
Frequently Asked Questions
What is data analytics?
Data analytics involves examining raw data to draw conclusions and identify patterns. It is used by businesses to make informed decisions, understand market trends, and gain insights into customer behavior.
Why is data analytics important for my business?
Data analytics is crucial for your business as it helps decode market trends, customer preferences, and manage large data sets, leading to better strategic decisions and a competitive edge.
What are the five types of data analytics?
The five types of data analytics are descriptive, diagnostic, predictive, prescriptive, and cognitive analytics, each offering different insights ranging from what happened to how we can make it happen.
How can I ensure my data analytics is scalable?
To ensure scalability, choose a customer data platform that can grow with your business, allowing for increased data integration and more complex analytics as your needs evolve.
What should I look for in a user-friendly analytics platform?
A user-friendly analytics platform should have an intuitive interface that is accessible to non-tech-savvy users, providing ease of use and effective data analysis without extensive training.
How can real-time AI analytics benefit my business?
Real-time AI analytics provides instant insights into business operations, allowing you to make swift, data-driven decisions that can improve efficiency and customer experiences.
What are the top trends in data analytics for 2024?
The top trends include the use of AI and machine learning, real-time analytics, data privacy and security advancements, and the integration of analytics into business strategies for growth.
How do I measure the impact of data analytics on my business?
Measure the impact by setting specific metrics for success, analyzing the return on investment of data initiatives, and employing continuous improvement through data feedback loops.