The Evolving Role of the Database Administrator
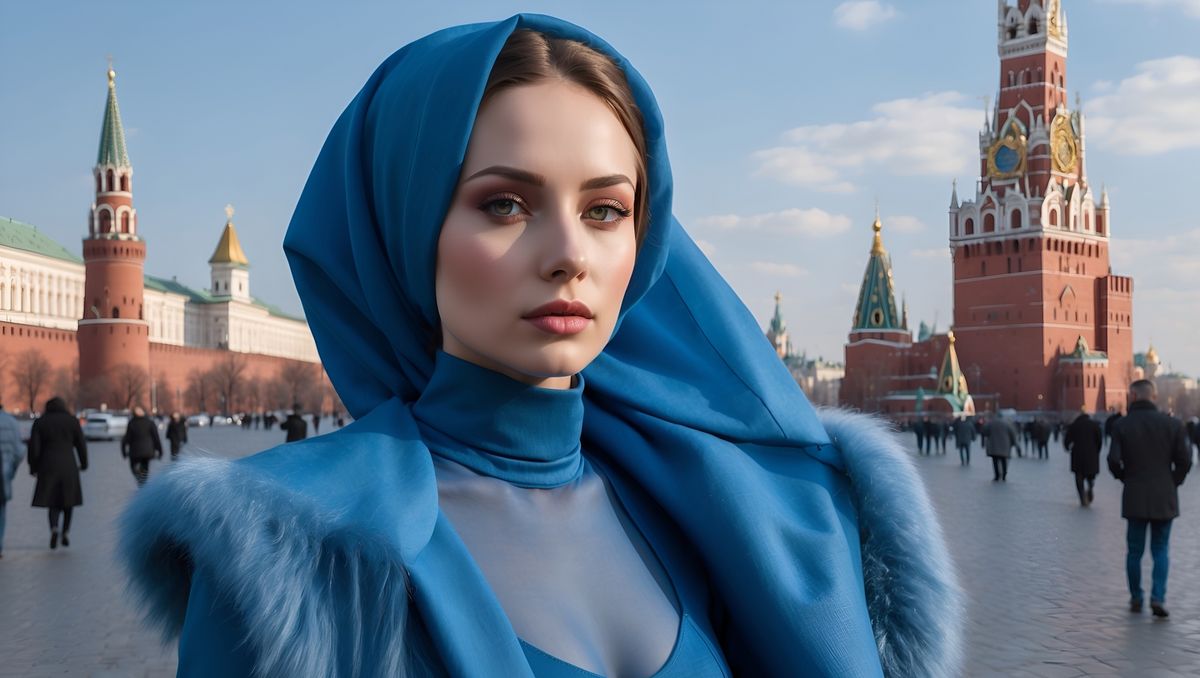
Adapting to Automation and AI
As we step into 2024, a few data management trends are set to reshape the landscape of how organizations handle, process, and protect their data. AI-powered automation is fundamentally transforming the operational landscape of businesses, streamlining tasks and enhancing efficiency across various industries. This revolution is not only about reducing costs but also about creating new opportunities for innovation and strategic advantage.
The integration of AI into database management systems is enabling more sophisticated data analysis and decision-making processes. For database administrators, this means a shift from routine maintenance to strategic roles that focus on leveraging AI for business insights and competitive edge.
- AI Adoption in Banks: Banks are increasingly hiring AI experts and developing proprietary tools to stay ahead.
- Challenges in AI Adoption: Financial services face hurdles such as data privacy and the need for specialized talent.
Embracing AI and automation requires a proactive approach to upskill and adapt to the evolving technological landscape, ensuring that database administrators remain indispensable in the era of intelligent systems.
Navigating Cloud Migration and Security
As organizations continue to embrace the cloud-first imperative, the journey of cloud migration becomes critical. Ensuring data security during this transition is paramount. A recent survey indicates that 62% of enterprises now manage databases in hosted cloud environments, reflecting a significant shift from traditional on-premise solutions.
- Identify sensitive data and apply appropriate protections
- Choose the right cloud service provider with robust security measures
- Implement continuous monitoring and threat detection
- Regularly update and patch systems to mitigate vulnerabilities
The success of cloud migration hinges not only on the strategic movement of data and applications but also on maintaining rigorous security protocols throughout the process.
Exploring SQL 2024 trends reveals a clear trend towards cloud adoption, with a notable increase in cloud budgets for the year. However, the complexity of cloud environments demands a comprehensive approach to security, one that encompasses both preventive and reactive strategies.
Managing Diverse Database Environments
In the face of rapidly expanding and diversifying data landscapes, database administrators (DBAs) are tasked with mastering a variety of database systems. The ability to manage and integrate data across hybrid and multi-cloud environments has become a critical skill. As organizations adopt a mix of on-premise, cloud, and hybrid databases, DBAs must ensure seamless data governance and security.
Agility in data management is key to providing fast and easy access to data, meeting the mandate for availability whenever and wherever it's needed. This requires a deep understanding of different database technologies and the ability to adapt to the unique demands of each environment.
- Establishing robust data governance frameworks
- Ensuring consistent data security protocols
- Integrating disparate data sources effectively
- Monitoring and optimizing database performance
The evolving landscape demands that DBAs not only maintain their traditional skills but also continuously learn and apply new strategies to manage the complexity of modern database ecosystems.
Cloud-First Strategies in Database Management
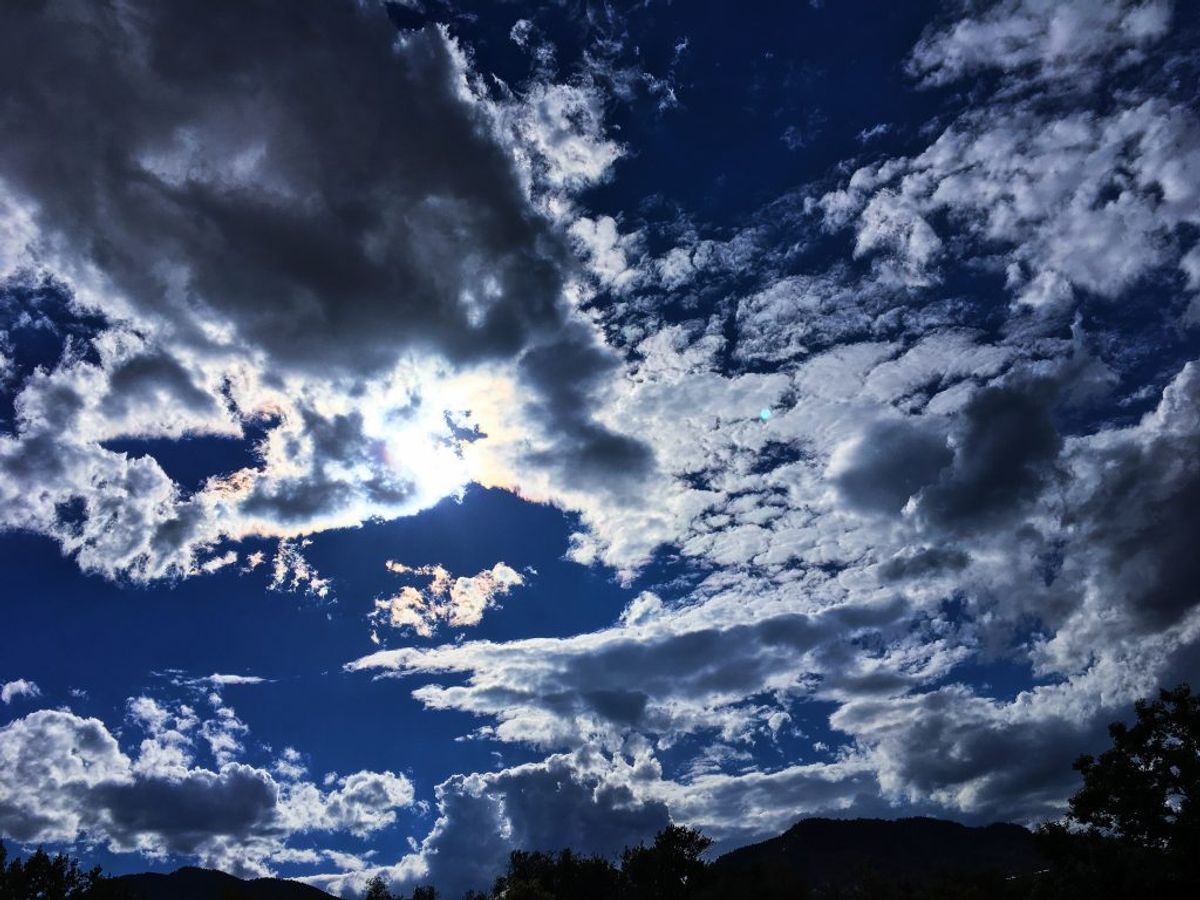
The Shift to Cloud Database Platforms
The landscape of database management is undergoing a significant transformation as businesses increasingly adopt cloud platforms. Cloud adoption accelerates as companies seek to leverage the scalability, flexibility, and cost-efficiency of cloud services. This shift is not just a trend but a strategic move to stay competitive in a data-driven market.
SQL 2024 trends highlight the importance of cloud platforms in enabling ERP transformation and fostering data security innovations. As the cloud becomes the new norm, database administrators must evolve their skills to manage and secure data across diverse environments.
- Cloud-native databases are on the rise, with a majority of enterprises migrating their data to hosted cloud solutions.
- Hybrid and multi-cloud strategies are becoming essential for effective data governance and integration.
- The focus on automation within cloud platforms is intensifying, requiring new strategies for database management.
The move to the cloud is not without its challenges, but the opportunities for growth and enhanced security in the evolving data management landscape are substantial.
Enhancing Data Management with Cloud Services
The cloud computing revolution is reshaping the SQL landscape, offering unprecedented scalability, flexibility, and accessibility. With cloud services, businesses can leverage enhanced data recovery, on-demand resources, and simplified collaboration, fostering the democratization of technology and opening new growth avenues.
Cloud services streamline the management of SQL databases by providing a suite of tools that cater to the diverse needs of modern businesses. This includes automated backups, advanced security protocols, and the ability to quickly scale resources to meet fluctuating demands.
Here are some of the key benefits of using cloud services for data management:
- Enhanced data recovery capabilities to safeguard against data loss
- Access to on-demand resources that can be scaled up or down as needed
- Simplified collaboration across teams and geographies
- Reduced overhead and maintenance costs compared to traditional on-premise solutions
By integrating cloud services into their data management strategies, organizations can not only improve their operational efficiency but also gain a competitive edge in today's fast-paced business environment.
Cost-Benefit Analysis of Cloud vs On-Premise Solutions
When considering the transition to cloud computing, businesses must conduct a thorough cost-benefit analysis to weigh the financial implications. The cloud offers notable scalability, allowing companies to adjust resources based on demand, which can lead to significant cost savings. On the other hand, on-premise solutions provide a sense of control and immediate access to physical hardware, which some organizations may prefer for regulatory or operational reasons.
The decision to migrate to the cloud or maintain on-premise infrastructure is not merely a financial one; it also encompasses strategic considerations such as business agility, innovation potential, and long-term growth.
A comparison of Total Cost of Ownership (TCO) often reveals that cloud solutions can be more cost-effective over time, especially when factoring in the expenses associated with maintaining and upgrading on-premise hardware. However, the initial migration costs and potential disruptions to business processes should not be overlooked.
Here is a simplified breakdown of key factors to consider:
- Initial investment and setup costs
- Ongoing operational expenses
- Scalability and flexibility
- Security and compliance requirements
- Potential for business continuity and disaster recovery
By carefully evaluating these aspects, businesses can identify the most suitable infrastructure for their specific needs, ensuring that they are well-positioned to capitalize on future growth opportunities while maintaining robust security in the cloud.
Real-Time Analytics: Transforming Data into Instant Insights
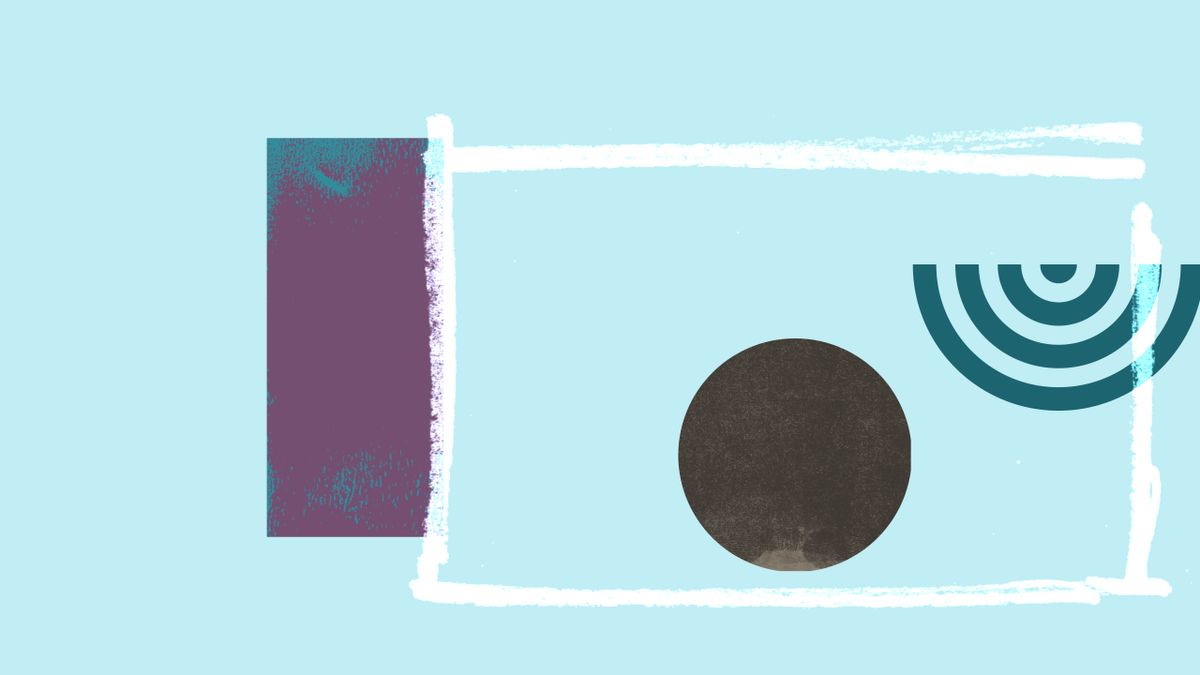
Architectures for Speed and Efficiency
In the pursuit of speed and efficiency, modern data architectures are being designed to handle the complexities of today's data-driven landscape. Microservices architectures, for instance, offer the agility and scalability necessary for high-performance operations. However, they also introduce challenges such as data consistency and monitoring.
Real-time analytics are at the forefront of this architectural evolution, enabling businesses to transform data into instant insights. This shift is not only about technology but also about adopting new strategies that align with the emerging SQL business insights for 2024.
To fully harness the potential of these architectures, a balance between innovative approaches and robust data management practices is crucial.
The table below outlines key components of a next-generation data architecture that supports speed and efficiency:
Component | Description |
---|---|
Cloud Services | Scalable and flexible data storage options |
Data Platforms | Foundations for advanced analytics |
Legacy Infrastructure | Integration with traditional systems |
AI and ML | Enabling predictive and prescriptive analytics |
As we navigate the future, the role of data architecture in business intelligence and decision-making will continue to grow, shaping the way organizations leverage their data for competitive advantage.
The Impact of Real-Time Data on Business Decisions
The advent of real-time analytics has revolutionized the way businesses operate, providing the ability to act swiftly on fresh information. Predictive analytics drive strategic decision-making, with applications ranging from market trend identification to optimizing marketing strategies. The integration of AI in SQL 2024 has been pivotal, enabling advanced data visualization and agile responses to market dynamics.
Real-time data streaming, powered by technologies like Apache Kafka and Apache Flink, is essential for AI-first enterprises. It ensures that data is processed with immediacy, which is crucial for delivering personalized user experiences and maintaining operational efficiency. The strategic value of streaming data is further enhanced when combined with historical context, such as in lakehouse formats, allowing for more comprehensive insights.
The integration of new data governance capabilities within cloud data warehouses and databases simplifies the creation and sharing of reusable data products. This advancement is instrumental in fostering the adoption of real-time data analytics across various business layers.
As businesses navigate the data-driven landscape, the ability to harness real-time data for decision-making is no longer a luxury but a necessity. It is a key factor in maintaining competitiveness and achieving success in today's fast-paced market.
Case Studies: Success Stories of Real-Time Analytics Implementation
The transformative power of real-time analytics is vividly illustrated through a variety of success stories across different industries. Healthcare analytics, for instance, has revolutionized patient care by enabling personalized treatment plans derived from immediate data analysis. In the financial sector, real-time data analysis has sharpened the competitive edge of firms by facilitating swift, data-driven decisions.
Apache Flink and similar technologies have been pivotal in accelerating real-time decision-making, allowing businesses to respond to market changes with unprecedented speed. The strategic importance of SQL skills, as highlighted in white papers and case studies, has been instrumental in driving business growth and fostering innovation within this evolving data ecosystem.
The adoption of real-time analytics has not only improved operational efficiency but also significantly enhanced customer experiences, proving to be a key factor in achieving a variety of business objectives.
To encapsulate the impact of real-time analytics, consider the following table summarizing key outcomes in different sectors:
Sector | Outcome | Improvement |
---|---|---|
Healthcare | Personalized Care | Enhanced Treatment Outcomes |
Finance | Data-Driven Decisions | Competitive Advantage |
As we navigate through 2024, webinars and data leadership roles continue to shed light on the best practices and considerations for enabling real-time data and analytics, ensuring that organizations remain agile and informed in a rapidly changing business landscape.
Data Storage Solutions for the Zettabyte Era
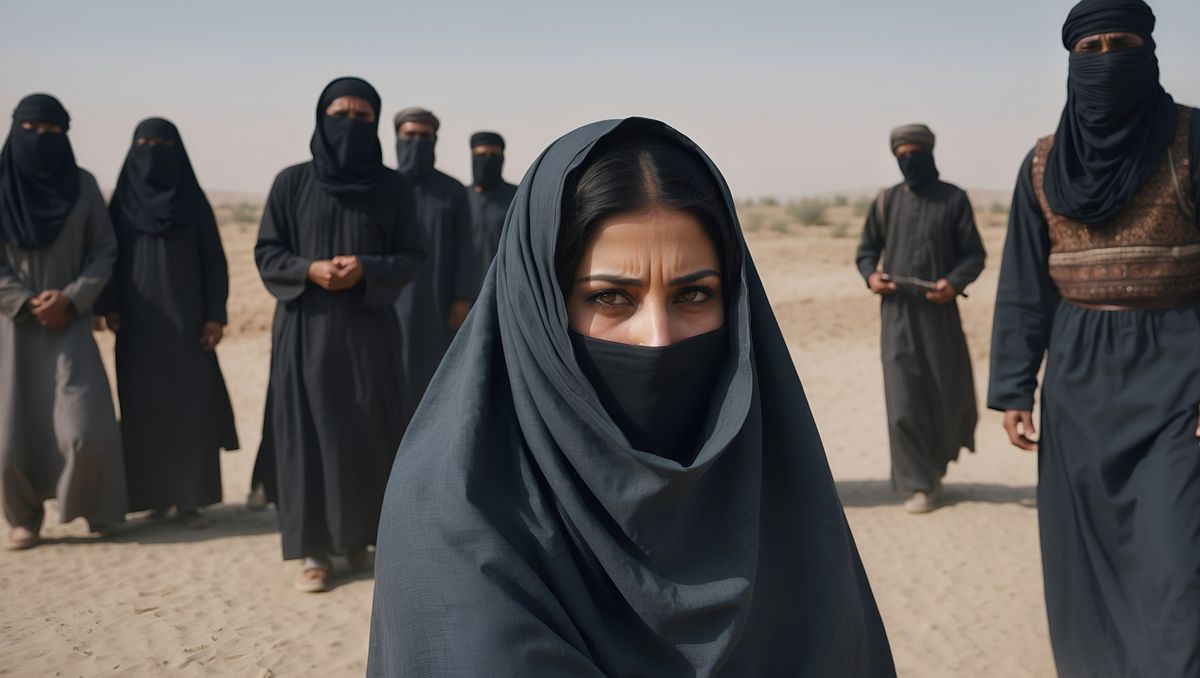
Scaling Infrastructure for Exponential Data Growth
As the digital universe continues to expand, businesses face the daunting task of scaling their data infrastructure to manage the exponential growth of data. The separation of compute and storage has emerged as a significant trend, enabling organizations to independently scale resources, which leads to more efficient utilization and cost savings.
To keep pace with this growth, a foundation for scalability is essential. Data pipeline automation lays the groundwork for scaling data operations, avoiding the resource allocation paradox that can stifle innovation and agility.
The following table outlines key considerations for scaling infrastructure:
Factor | Importance | Action Required |
---|---|---|
Compute-Storage Separation | High | Implement flexible architectures |
Data Pipeline Automation | Critical | Invest in automation tools |
Cost Efficiency | Medium | Optimize resource allocation |
By addressing these factors, businesses can ensure that their data infrastructure is robust enough to handle current needs while being adaptable for future demands.
Innovations in Data Storage Technologies
As we delve into the landscape of data storage in 2024, a pivotal innovation is the separation of compute and storage. This architectural shift is revolutionizing how businesses approach data scalability and cost efficiency. By decoupling these two components, organizations can independently scale their resources, leading to a more agile and cost-effective infrastructure.
- Tiered Storage: Kafka's Tiered Storage is a prime example of this trend, offering significant benefits in handling fluctuating workloads.
- Oracle Database Storage: Strategies for unlocking Oracle database storage excellence focus on overcoming performance bottlenecks.
- Real-Time Analytics: Advanced architectures are essential for supporting the high-speed demands of modern analytics.
The ability to scale storage and compute independently is a game-changer, enabling businesses to adapt swiftly to changing data demands without incurring unnecessary costs.
Exploring SQL trends in 2024 is not just about keeping up with technology; it's about harnessing these innovations to drive business growth strategies. SQL remains a crucial tool for data analysis, cloud technology, and gaining consumer insights, with advanced techniques like CTEs and Window Functions enhancing efficiency.
Balancing Performance with Storage Costs
In the era of exponential data growth, businesses face the challenge of balancing performance with storage costs. The separation of compute and storage has emerged as a significant trend, allowing for independent scaling of resources and more efficient utilization.
- Traditional setups with tightly coupled compute and storage often lead to inefficiencies.
- Tiered storage solutions, like Kafka's Tiered Storage, promise cost savings and flexibility.
- Realizing these benefits at scale remains a key focus for the future.
As workloads fluctuate, the ability to dynamically adjust storage and compute resources becomes crucial for maintaining both performance and cost-effectiveness.
The table below outlines the potential cost savings when adopting a decoupled architecture:
Resource | Traditional Setup | Decoupled Architecture |
---|---|---|
Compute | High fixed cost | Variable based on use |
Storage | High fixed cost | Pay-as-you-go pricing |
Adopting strategies for effective large-scale environment management can unlock superior performance while keeping storage costs in check. As we navigate the complexities of database storage in 2024, it is essential to stay informed about emerging trends and solutions that can help strike the right balance.
Data Management Meets DevOps: A Synergy for Agility
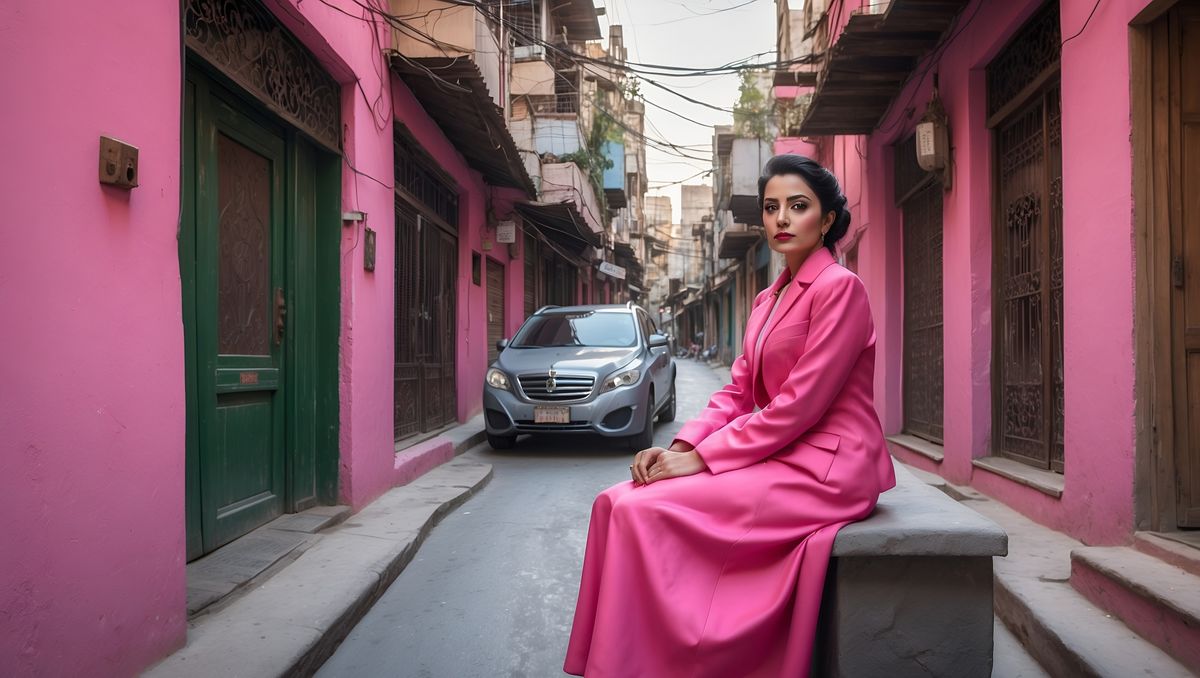
Integrating Data Management into the DevOps Pipeline
The convergence of data management with DevOps practices is a pivotal trend, fostering an environment where agility and speed are paramount. DataOps, a derivative of DevOps, emphasizes the seamless integration of data management into the continuous delivery pipeline, ensuring that data is reliable, accessible, and ready for use in real-time applications.
Automation plays a critical role in this integration, allowing for the rapid provisioning of data environments and the efficient handling of complex data workflows. By incorporating data management tasks into the DevOps cycle, organizations can achieve a more streamlined and collaborative approach to data operations.
The goal is to create a symbiotic relationship where data management enhances DevOps processes, and DevOps methodologies improve data handling.
The benefits of this integration are clear:
- Enhanced collaboration between data professionals and development teams
- Faster time-to-market for data-driven applications
- Improved data quality and consistency
- Greater responsiveness to changing data requirements
As the data landscape continues to evolve, the fusion of DataOps within the DevOps pipeline will be crucial for businesses seeking to capitalize on the value of their data assets.
Achieving Interoperability and Flexibility in Data Operations
In the quest for data unification, organizations are increasingly turning to Data Management Clouds to foster interoperability across diverse data environments. Achieving a state of interoperable core data is not just about technology; it requires a strategic plan and a mindset shift towards more agile data operations.
The integration of new data governance capabilities within cloud data warehouses and databases is a pivotal step in modernizing data infrastructure. It simplifies the creation and sharing of reusable data products, enhancing the adoption of real-time data analytics.
To ensure data is available where and when it's needed, consider the following steps:
- Assess current data management practices and identify areas for improvement.
- Implement open table formats to transcend traditional structures and unify real-time and batch data.
- Leverage streaming data architectures to enable instant access and analysis.
- Adopt modern data integration and governance strategies to overcome data silos.
These measures pave the way for businesses to compete more effectively on intelligence, driving agility and innovation in a data-centric world.
The Role of Automation in Data Management
In the realm of SQL 2024, the integration of automation and AI is not just a trend but a strategic imperative for data-driven businesses. AI-powered automation is fundamentally transforming the operational landscape, enabling organizations to achieve unprecedented levels of efficiency and insight. This shift is particularly evident in the management of data operations, where automation streamlines processes, reduces costs, and enhances productivity.
As businesses leverage AI and automation for insights and efficiency, it's crucial to understand the emerging trends that focus on distributed databases and automation for data operations. The table below outlines the key benefits of automation in data management:
Benefit | Description |
---|---|
Efficiency | Automation accelerates data-related tasks, minimizing manual effort. |
Accuracy | Reduces human error, ensuring data integrity. |
Scalability | Facilitates handling of growing data volumes with ease. |
Cost Savings | Lowers operational expenses by optimizing resource utilization. |
Embracing automation in data management is not just about adopting new technologies; it's about reshaping the culture and workflows to foster a more agile and responsive data environment.
Cybersecurity remains a crucial aspect of this transformation, as the reliance on automated systems necessitates robust security measures to protect sensitive data. The future of data management is one where automation and human expertise converge to create a more resilient and dynamic ecosystem.
Harnessing Knowledge Graphs for Enhanced Data Intelligence
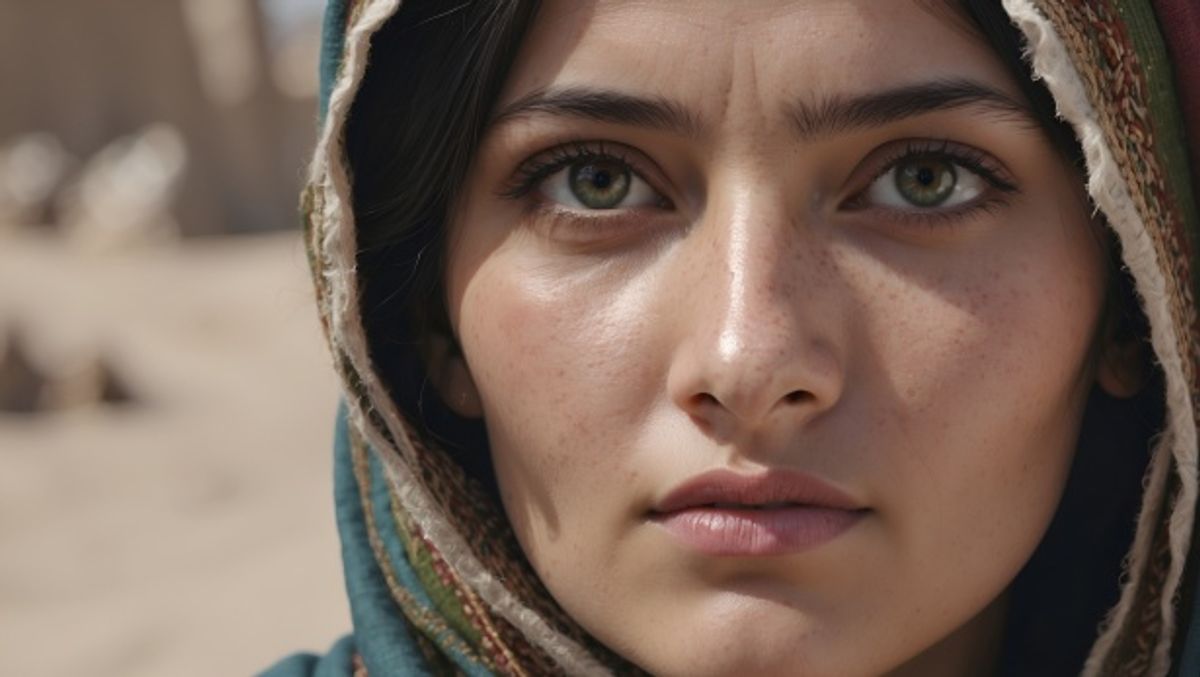
Building Context-Aware Recommendation Systems
In the era of personalized user experiences, context-aware recommendation systems stand at the forefront of innovation. By leveraging knowledge graphs, these systems can understand and predict user preferences with remarkable accuracy. They are particularly transformative in industries where tailored suggestions can significantly enhance customer engagement and satisfaction.
Knowledge graphs enable a nuanced understanding of user behavior, which is critical for delivering personalized content and recommendations.
For instance, in e-commerce, a recommendation system can suggest products based on a user's browsing history, purchase records, and even the time of day. In media streaming services, these systems can curate playlists or movie selections that resonate with an individual's mood or recent interests.
- Key Benefits of Context-Aware Systems:
- Enhanced user engagement
- Increased conversion rates
- Improved customer retention
While the potential is vast, the challenge lies in constructing and maintaining a robust knowledge graph that can scale and evolve with user data. This requires a strategic approach to data collection, analysis, and the integration of real-time data streams.
Solving Complex Data Challenges with Graph Technology
Knowledge graphs have emerged as a powerful tool for tackling the intricate challenges of modern data networks. By representing data in interconnected structures, they enable deeper insights and more nuanced understanding of relationships. Graph analytics plays a pivotal role in revealing patterns within these complex networks, despite facing hurdles such as heterogeneity and noise in real-world data.
Knowledge graphs not only enhance the accuracy of recommendation systems but also address the echo chamber effect, ensuring a diverse range of suggestions.
The implementation of graph technology can be seen across various industries, with notable improvements in areas such as healthcare analytics and finance. Here's a glimpse into how different sectors are leveraging graph technology:
- Healthcare: Enhancing patient treatment outcomes through collaborative data analysis.
- Finance: Advancing data analysis to inform better decision-making.
As organizations continue to navigate the vast seas of data, the adoption of graph technology is proving to be an invaluable asset in solving complex data challenges.
Case Studies: Knowledge Graphs in Action
The transformative power of knowledge graphs is evident across various industries, where they redefine data management and enhance decision-making processes. Healthcare analytics is one such domain where knowledge graphs have made a significant impact. By integrating diverse patient data, these graphs facilitate a new level of personalized treatment, leading to improved outcomes.
In the financial sector, knowledge graphs assist in unraveling complex data patterns, enabling analysts to make more informed decisions. The advent of AI tools like ChatGPT has further expanded the potential of knowledge graphs, allowing for rapid information retrieval and advanced data analysis.
The synergy between knowledge graphs and AI is creating unprecedented opportunities for innovation and efficiency in data management.
Here are some notable use cases where knowledge graphs have demonstrated their value:
- Healthcare Analytics: Enhancing patient care through personalized insights.
- Finance and Data Analysis: Uncovering hidden patterns for strategic financial planning.
- Content Generation: Assisting in the creation of nuanced and contextually relevant content.
- Chatbots and Virtual Assistants: Enabling more intelligent and responsive user interactions.
Modern Data Architectures: Enabling Scalability and Agility
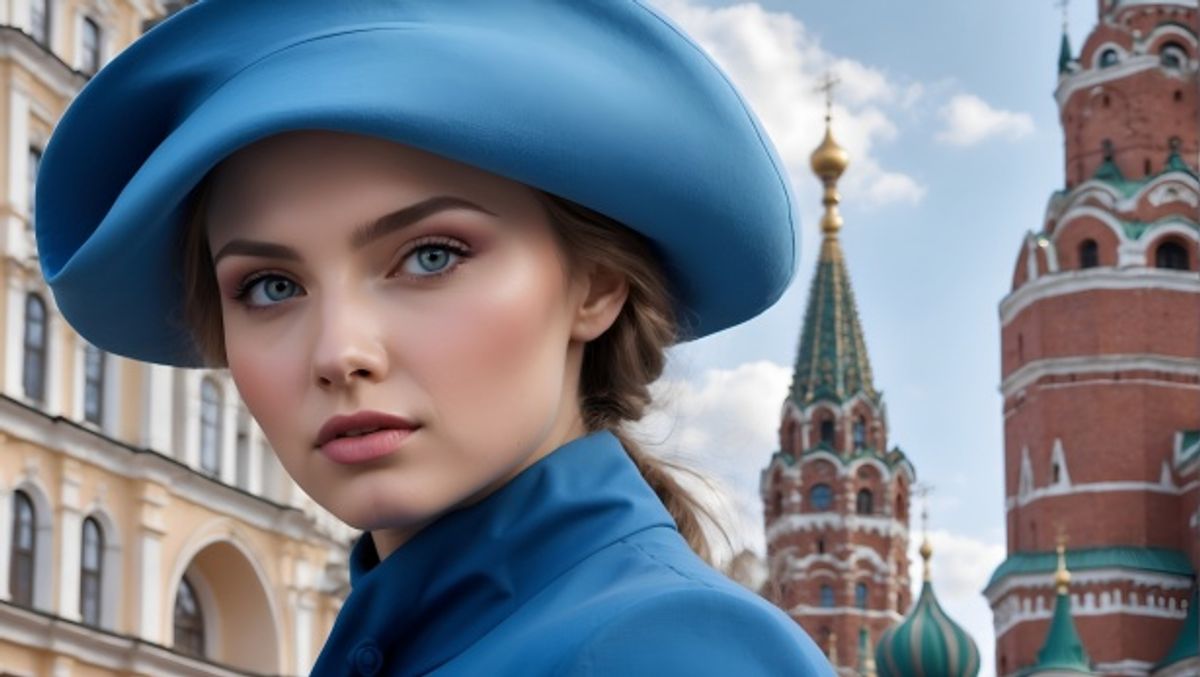
The Rise of Data Lakehouses and Data Fabrics
The advent of data lakehouses and data fabrics marks a significant milestone in the evolution of data management. Transactional data lake architectures are redefining the landscape, merging the best of data lakes and warehouses. By leveraging open table formats and streaming, these architectures facilitate a unified approach to handling real-time and batch data, setting the stage for advanced analytics and AI.
Organizations are increasingly adopting these modern data architectures to meet the demands for scalability and agility. The table below outlines the core differences between traditional and modern data storage solutions:
Feature | Traditional Data Warehouse | Data Lakehouse | Data Fabric |
---|---|---|---|
Data Structure | Structured | Structured & Unstructured | Distributed |
Storage | Centralized | Decentralized | Decentralized |
Scalability | Limited | High | High |
Real-Time Processing | Not Supported | Supported | Supported |
The integration of data lakehouses and fabrics into business systems is not just a procedural update; it's a fundamental shift that empowers organizations to harness the full potential of their data.
As the complexity of data environments grows, the role of SQL developers becomes increasingly critical. They are at the forefront of designing systems that not only accommodate but also capitalize on emerging technologies like cloud services and machine learning, ensuring industry relevance.
Best Practices in Data Architecture Design
In the realm of data architecture, the integration of new technologies is pivotal for fostering growth and innovation. Best practices in data architecture design are essential to harness the full potential of SQL 2024 trends, ensuring that organizations can adapt to the demands of agility and scalability.
Integration with cloud computing, big data, and edge computing is no longer optional but a necessity. By reducing design, deployment, and maintenance time, businesses can focus on strategic initiatives rather than operational challenges.
- Ensure compatibility with existing systems and future technologies
- Prioritize security and compliance from the outset
- Adopt a modular approach to facilitate scalability
- Embrace automation for efficiency and accuracy
By adhering to these best practices, organizations can navigate the future of data with confidence, turning challenges into opportunities for innovation.
The landscape of data architecture is continuously evolving, with a focus on reducing complexity and enhancing performance. As we move forward, the emphasis will be on creating architectures that are not only robust and secure but also flexible enough to accommodate the rapid pace of technological change.
Evaluating the Impact of Data Architecture on AI and ML
The symbiosis between data architecture and artificial intelligence (AI) and machine learning (ML) is more pronounced than ever. Modern data architectures are the bedrock upon which AI and ML models are built, providing the necessary infrastructure for data ingestion, processing, and analysis. As organizations strive to harness the full potential of AI and ML, the design and implementation of these architectures become critical.
- Scalability to handle vast datasets
- Flexibility to incorporate diverse data types
- Speed to enable real-time analytics
- Modularity to support evolving AI and ML use cases
The right data architecture not only streamlines operations but also unlocks new possibilities in AI and ML, fostering innovation and competitive advantage.
Evaluating the impact of data architecture on AI and ML involves assessing how well it supports the entire data lifecycle. From data collection to model deployment, every stage must be optimized for the unique demands of AI and ML workloads. This includes considering ethical principles such as bias, fairness, and transparency, which are becoming increasingly important in the development and deployment of ML models.
Data Engineering: The Unsung Hero of the AI Revolution
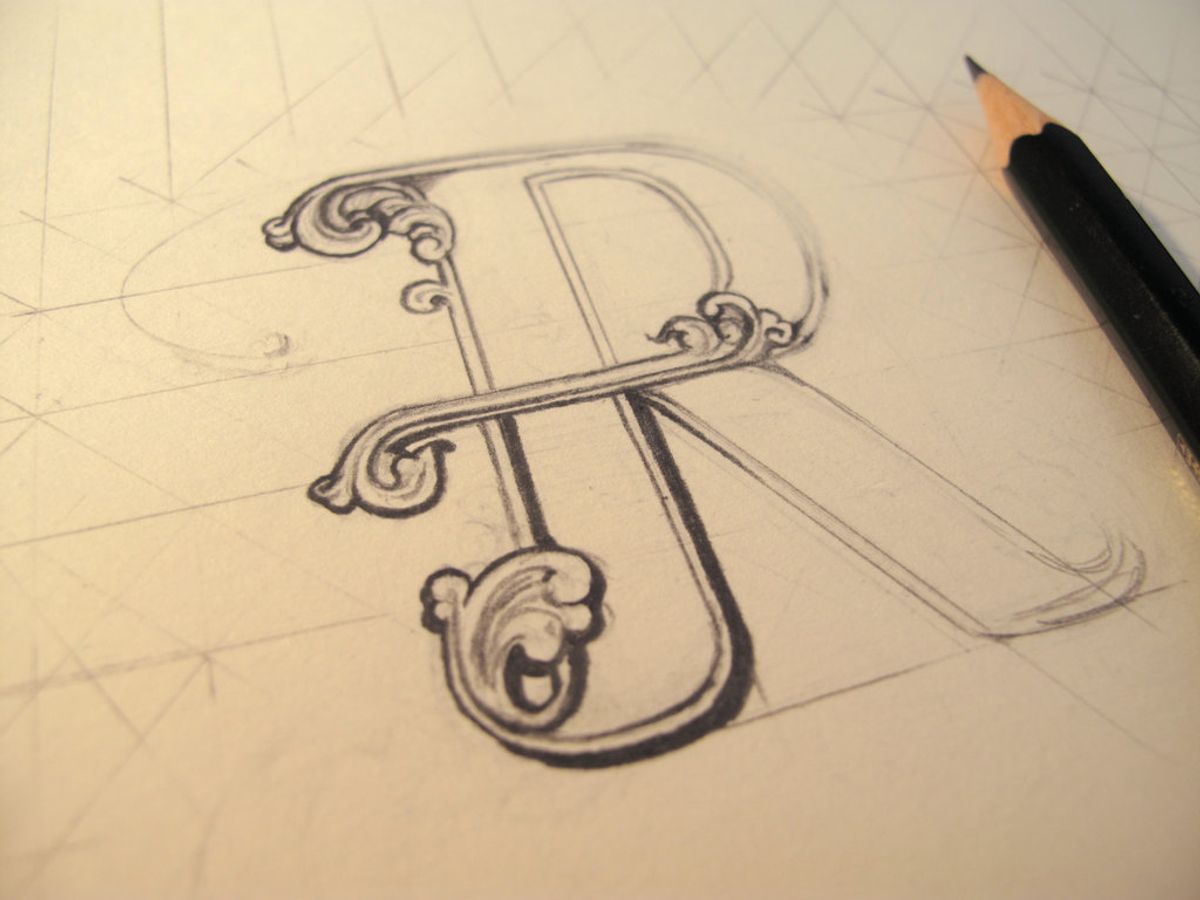
Emerging Trends in Data Engineering
As we approach 2024, the landscape of data engineering is witnessing a significant transformation. SQL remains the backbone of data operations, with its role in decision-making evolving from mere data processing to transforming data into valuable assets. Continuous learning and the integration of emerging technologies are becoming indispensable for SQL professionals.
The skill set for data engineers is expanding to include expertise in AI prompt engineering and advanced data analysis. This reflects the broader trend towards sophisticated data processing and the utilization of generative AI tools. Here are some of the key trends:
- Emphasis on engineering principles in data analysis
- Growing importance of AI and machine learning competencies
- Increasing demand for full-stack data experts
The ongoing data revolution, powered by AI and machine learning, is reshaping our professional landscapes. Staying abreast of these changes is crucial for data engineers aiming to lead in their field.
Best Practices for Data Pipeline Construction
Constructing a data pipeline is a critical task that requires careful planning and execution. Ensuring data quality and consistency is paramount, as it forms the foundation of reliable analytics and decision-making processes. A step-by-step approach, as detailed by Astera Software, can guide engineers through the intricate process of building an effective pipeline.
Automation plays a key role in modern data pipeline construction. By automating repetitive tasks and data validation checks, engineers can focus on more complex aspects of the pipeline, such as integrating new data sources or optimizing performance. Here are some best practices to consider:
- Define clear objectives and requirements for the data pipeline.
- Choose the right tools and technologies that align with your data strategy.
- Implement robust error handling and recovery mechanisms.
- Regularly monitor and maintain the pipeline to ensure its health and efficiency.
Embracing these best practices will not only streamline the development process but also enhance the overall robustness and scalability of the data pipeline.
As the complexity of data environments grows, the skills required to manipulate and prepare large datasets, often in real-time, become increasingly valuable. A solid understanding of the underlying systems and architectures is essential for the optimization of data pipelines.
The Critical Role of Data Engineering in AI Implementation
Data engineering is the unsung hero in the AI revolution, providing the foundation upon which machine learning models are built. Data engineers play a pivotal role in aggregating and organizing data within data warehouses, making it accessible and usable for vector databases and AI tools. This symbiotic relationship between AI and data engineering ensures that the data is not only available but also primed for generating valuable insights.
Ethical considerations in AI and data science are becoming increasingly important. As data engineers, we must ensure that the systems we build adhere to principles of fairness, transparency, and accountability. This is not just a technical challenge but a moral imperative that shapes the future of AI.
The requirement for engineering and AI prompt engineering skills marks a significant evolution in the role of the data analyst. Embracing these skills is crucial for those in the field, as it will distinguish the next generation of data analysts.
The landscape of data engineering is ever-changing, with new trends and best practices emerging regularly. Staying ahead of these developments is essential for data engineers to continue enabling AI advancements effectively.
Powering Modern Applications with Advanced Data Management
Strategies for Managing Data in Distributed Systems
In the era of distributed systems, managing data effectively is crucial for operational efficiency and business agility. The data-as-product strategy is becoming increasingly popular, as it enhances efficiency and drives innovation across the real-time data landscape. However, ensuring secure and contextual data sharing remains a significant challenge.
- Embed governance at the data source to maintain context and reduce costs.
- Prioritize data availability for fast, easy access across diverse environments.
- Utilize cloud-native databases to leverage the scalability and agility of the cloud.
As data environments grow more complex, the agility of data management systems becomes paramount to harness the full potential of distributed data.
Building resilient distributed systems is essential for businesses to realize long-term business outcomes. By integrating governance early in the data lifecycle, organizations can provide a clearer understanding of data's context and value, which is especially important as data travels downstream.
Emerging Technologies in Data Management for AI/ML Workloads
The integration of machine learning (ML) into SQL databases is a pivotal trend in 2024, driving the need for advanced analytics capabilities that are both scalable and compliant with global regulations. Organizations are rapidly adopting new technologies to ensure their data management strategies are AI-ready, aligning with the SQL 2024 trends.
- Streaming data platforms are becoming essential for real-time AI applications, moving away from traditional batch-oriented architectures.
- Modular data architectures facilitate the quick incorporation of new AI and ML analytics use cases.
- Data mesh and lakehouse concepts are being explored to enhance data governance and integration across hybrid and multi-cloud environments.
The quest for agility and innovation in the AI era necessitates a reimagined approach to data management, where flexibility and efficiency are paramount.
As the landscape evolves, companies must remain vigilant in their pursuit of technologies that not only bolster AI and ML analytics but also ensure responsible implementation in line with emerging global standards.
Case Studies: Innovative Data Management in Action
In the realm of healthcare analytics, the fusion of data science and medical expertise has led to a revolution in patient care. Bold strides in personalized treatment are being made as data-driven insights become integral to healthcare delivery.
The journey towards data democratization is marked by the challenge of providing seamless access to data across an organization. Achieving this unlocks unprecedented efficiency and innovation.
The finance sector has similarly seen a transformation, with data analysis driving strategic decisions. Below is a snapshot of the impact:
- Healthcare: Enhanced patient outcomes through data integration.
- Finance: Improved risk management and investment strategies.
As we navigate the complexities of modern data architectures, it's clear that the synergy between DataOps and traditional data management is bridging the gap between data producers and consumers, fostering a new era of accessibility and collaboration.
Navigating the Data-Driven Future: Opportunities and Challenges
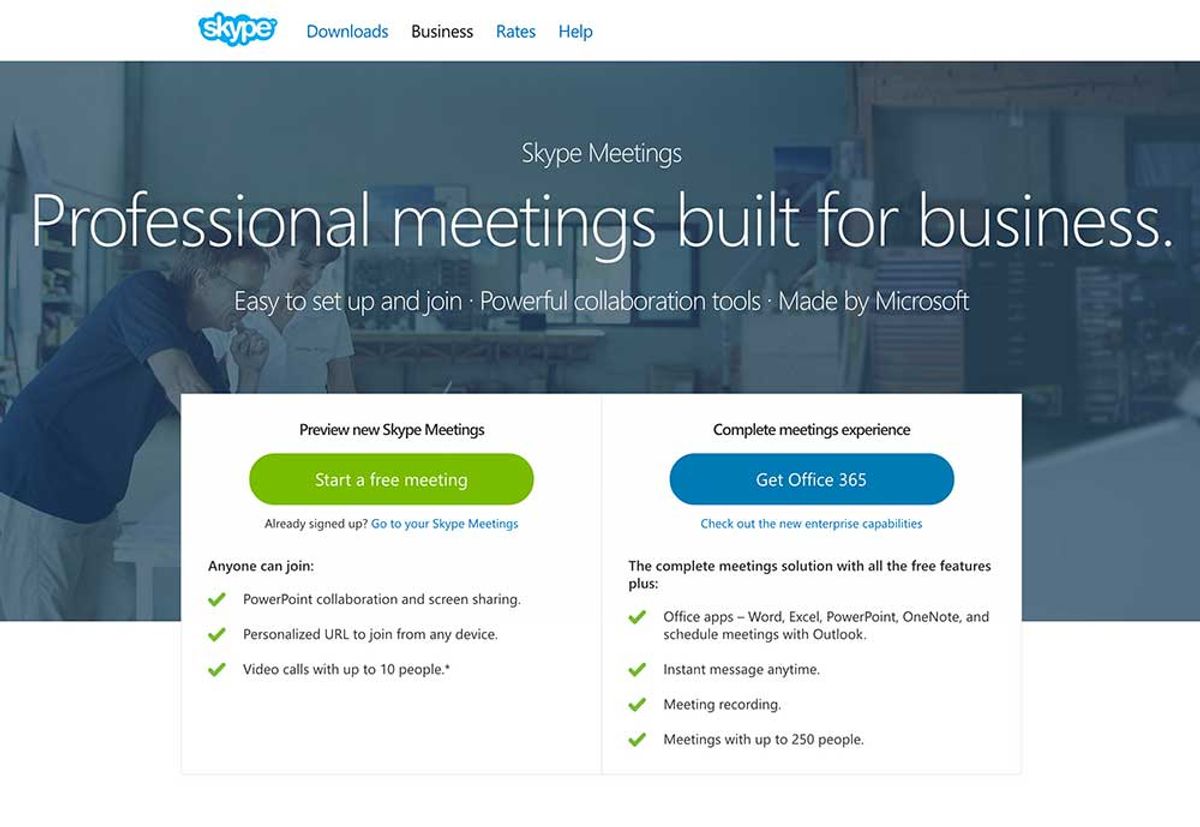
Identifying Growth Opportunities in a Data-Centric World
In the landscape of SQL trends in 2024, businesses are poised to leverage augmented analytics to gain a competitive edge. The integration of real-time and predictive analytics into strategic planning is not just a trend but a necessity for informed decision-making. Edge computing is another area where SQL's critical role is expanding, bringing data processing closer to the source and reducing latency.
- Augmented Analytics: Enhancing decision-making with AI-driven insights.
- Real-time Analytics: Providing immediate business intelligence.
- Predictive Analytics 2.0: Anticipating market trends and customer behavior.
- Edge Computing Integration: Streamlining operations with localized data processing.
Embracing these SQL trends will be crucial for businesses aiming to capitalize on data-driven growth opportunities. The ability to quickly interpret and act on data can set industry leaders apart from their competitors.
Overcoming Data Quality Challenges
In the quest to harness the full potential of AI, the elephant in the room is undoubtedly data quality. Ensuring the integrity and accuracy of data is paramount for AI systems to deliver reliable and actionable insights. To address this, organizations must identify and rectify the most common data quality issues.
- Establishing clear data governance policies
- Implementing robust data validation checks
- Utilizing advanced data cleaning tools
- Regularly auditing data for consistency and accuracy
By proactively tackling these challenges, businesses can significantly enhance their data's reliability, paving the way for successful AI implementations.
It's crucial to understand that data quality is not a one-time fix but a continuous process. As the volume and variety of data grow, so does the complexity of maintaining its quality. Embracing a culture of data excellence within the organization is essential for long-term success.
Strategic Planning for Data-Driven Business Transformation
In the era of data-driven decision-making, strategic planning is the cornerstone of successful business transformation. A well-planned strategy is the first step towards harnessing the power of data analytics to drive growth and innovation. Following this, the implementation of an automated data analytics platform can streamline processes and uncover valuable insights.
To remain competitive, businesses must adapt to the evolving landscape by integrating new data governance capabilities and modernizing their data infrastructure.
The journey of transformation involves several key stages:
- Assessing the current data architecture and its alignment with business goals
- Identifying areas where AI and ML analytics can be leveraged
- Modernizing data integration and governance to support a hybrid and multi-cloud environment
- Democratizing data to empower employees and enhance operational efficiency
- Continuously evaluating and refining data management strategies to meet emerging needs
Each of these steps is crucial for building a next-generation data architecture that is agile, scalable, and capable of meeting the demands of the AI era.
As we sail into the data-driven future, the need for optimized database solutions becomes paramount. At OptimizDBA, we pride ourselves on delivering faster data solutions and unparalleled database optimization consulting. Our clients, like Radio-Canada Inc., have experienced transaction speeds that are not just twice as fast, but often 100 times or more! Don't let data challenges slow you down. Visit our website to learn how our proprietary methodology can revolutionize your data processes and put you ahead of the curve. Let's talk about your challenges and unlock the full potential of your data!
Conclusion
As we navigate the dynamic landscape of SQL and data management in 2024, it's clear that the future is both challenging and ripe with opportunities. The shift towards cloud-based database management, the ubiquity of SQL Server across various platforms, and the increasing importance of data quality and real-time analytics are just a few of the trends reshaping the industry. The role of the DBA is evolving in response to these changes, with a greater emphasis on knowledge graphs, context-aware systems, and modern data architectures that support speed, scale, and flexibility. Organizations that embrace these trends, invest in modernizing their data management strategies, and adapt to the emerging best practices in data engineering will be well-positioned to lead and thrive in the AI era. As we conclude, it's essential for businesses to stay informed and agile, leveraging the insights from industry experts and reports like CB Insights' 2024 Tech Trends to make impactful decisions that drive growth and innovation.
Frequently Asked Questions
How is automation impacting the role of Database Administrators in 2024?
Automation in 2024 is streamlining repetitive tasks, allowing DBAs to focus on more strategic initiatives such as data governance, architecture, and security. However, it also requires them to adapt by gaining new skills in AI and automation tools.
What are the key considerations for businesses migrating databases to the cloud?
Businesses should consider data security, compliance, cost, performance, and the ability to integrate with existing systems. They must also decide between various service models like IaaS, PaaS, and SaaS.
How are real-time analytics changing business decision-making processes?
Real-time analytics provide instant insights into operations, enabling businesses to make quicker, data-driven decisions. This leads to improved customer experiences, operational efficiency, and competitive advantage.
What innovations in data storage technology are emerging to handle zettabyte-scale data?
Innovations include advancements in solid-state drives (SSDs), NVMe technology, and software-defined storage (SDS). These technologies offer improved speed, capacity, and scalability to manage massive volumes of data.
How is DevOps integrating with data management to enhance business agility?
Data management is adopting DevOps practices to automate and streamline data workflows, ensuring faster deployment, collaboration, and continuous improvement, which in turn enhances business agility.
What are knowledge graphs, and how do they contribute to data intelligence?
Knowledge graphs are data structures that connect data points in a contextual and semantic network, enabling complex queries and analytics. They enhance data intelligence by providing deeper insights and recommendations.
What are the benefits of modern data architectures like data lakehouses and data fabrics?
Data lakehouses and fabrics offer a blend of structured and unstructured data management, providing scalability, flexibility, and real-time analytics capabilities, which are essential for AI and ML applications.
Why is data engineering considered critical for successful AI implementation?
Data engineering is critical because it ensures the quality, availability, and structured flow of data, which are foundational for training accurate AI models and implementing AI solutions effectively.