Predictive Analytics: Unlocking Future Trends
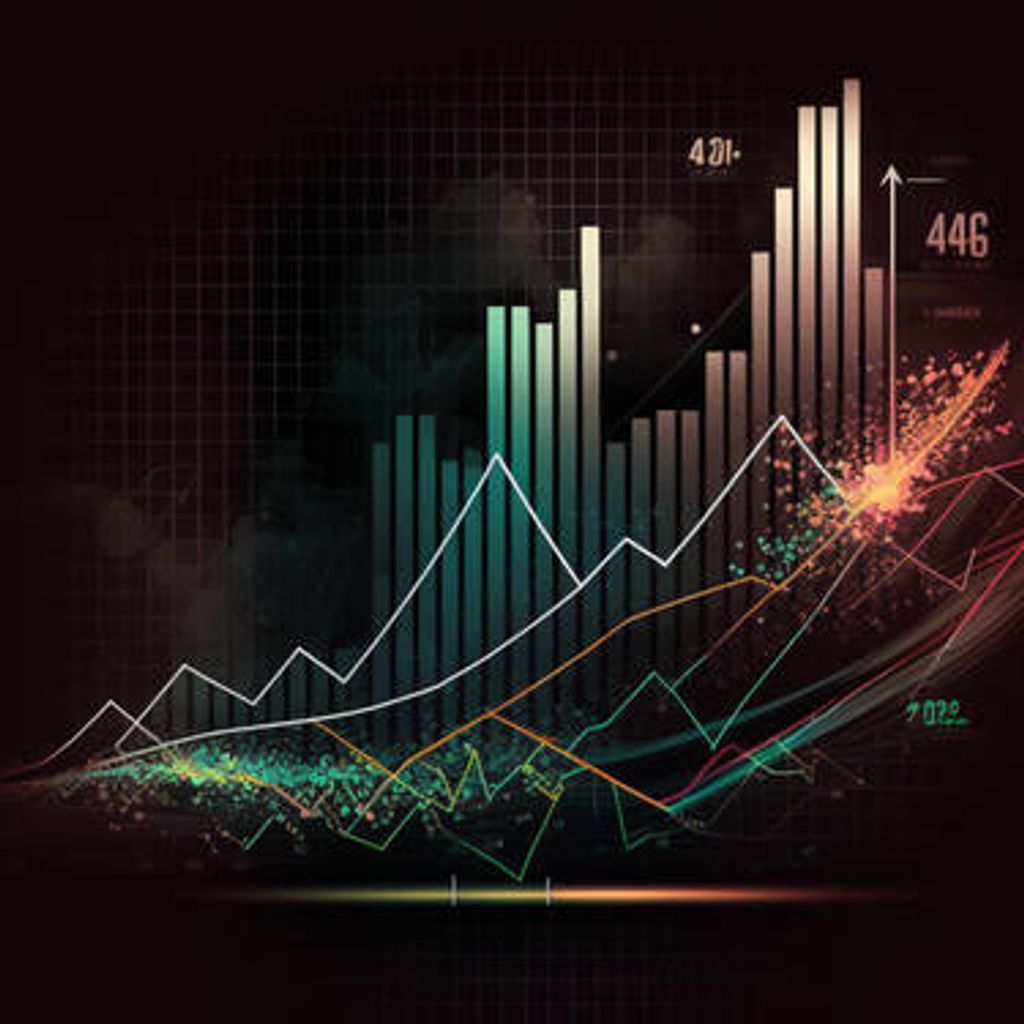
Harnessing Data and Algorithms
In the realm of predictive analytics, the power of data is harnessed through sophisticated algorithms that can identify trends and make forecasts. These algorithms are the engines that drive predictive models, turning raw data into actionable insights.
By leveraging historical data and machine learning, organizations can predict future events with greater accuracy, enabling them to make informed strategic decisions.
The process of harnessing data and algorithms involves several key steps:
- Collection of relevant and high-quality data.
- Cleaning and preprocessing to ensure data consistency.
- Selection and application of appropriate machine learning algorithms.
- Continuous refinement and adaptation of models to evolving data patterns.
Embracing these steps allows businesses to not only react to market changes but also to proactively shape their future strategies.
Proactive Decision-Making
In the realm of business strategy, proactive decision-making stands as a cornerstone, enabling organizations to steer their course with foresight and precision. By leveraging predictive analytics, companies gain the ability to not just react to market changes, but to anticipate and prepare for them. This forward-thinking approach is essential for maintaining a competitive edge and ensuring long-term success.
Predictive analytics plays a pivotal role in this process by providing insights into prospective outcomes, which guide businesses through complex choices and market possibilities. It empowers companies to forecast demand, manage inventory efficiently, and optimize marketing strategies, all while balancing the need for speed with thorough analysis to avoid impulsive decisions.
With predictive analytics, businesses can adapt swiftly to changing market dynamics, respond to emerging trends, and tailor their offerings to individual customer needs.
For instance, consider the following benefits that predictive analytics brings to proactive decision-making:
- Anticipating market trends and customer behaviors
- Minimizing risks and maximizing marketing effectiveness
- Enhancing operational efficiency and security
By integrating predictive analytics into their decision-making processes, businesses not only make informed decisions based on historical data but also stay flexible and responsive to immediate changes in customer behavior.
Resource Allocation and Optimization
In the realm of business, efficient resource allocation is pivotal for maximizing returns and minimizing waste. Predictive analytics plays a crucial role in this optimization process by forecasting where resources will be most effectively utilized. For instance, in marketing, predictive models can pinpoint which campaigns or customer segments are likely to yield the highest ROI, allowing for a strategic distribution of budget and effort.
Predictive analytics enables businesses to allocate resources proactively, ensuring that each decision is data-driven and aligned with future trends.
The benefits of such an approach are manifold, including:
- Proactive customer retention strategies, reducing churn rates and bolstering loyalty.
- Streamlined operations, where predictive maintenance can foresee equipment failures before they occur.
- Enhanced supply chain efficiency, by predicting demand and optimizing inventory levels.
By embracing predictive analytics, companies can transform their resource allocation from a reactive to a proactive stance, ensuring that every dollar spent is an investment in future success.
Forecasting Future Outcomes
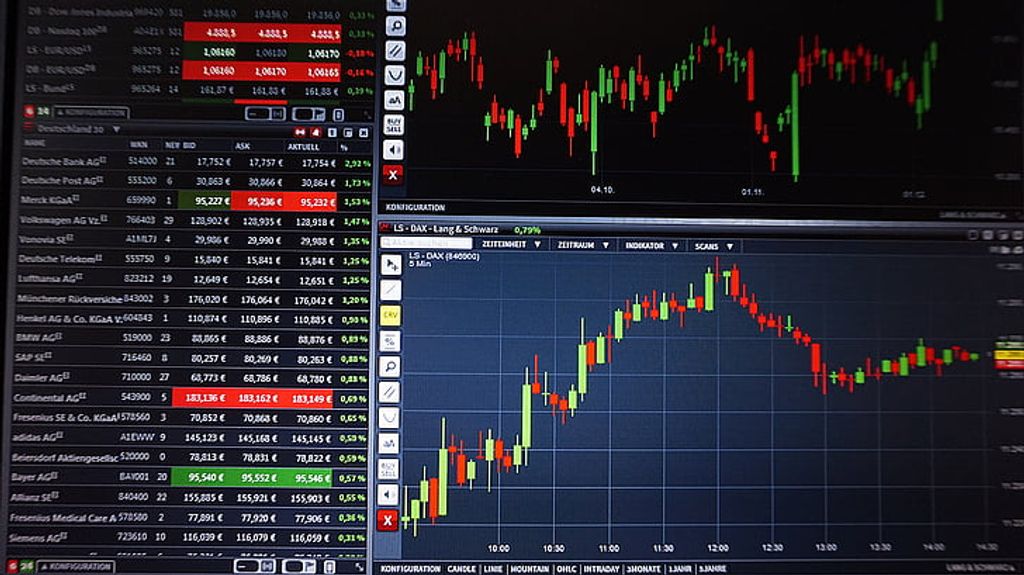
Statistical Algorithms in Action
At the heart of predictive analytics lies the power of statistical algorithms and machine learning, which together form the backbone of forecasting future trends. These tools are adept at sifting through vast amounts of historical data to identify patterns that can predict future events. Businesses can gain a competitive edge by understanding which customers may churn or which products will be in demand, thereby tailoring their strategies for maximum impact.
The predictive prowess of these algorithms is not just theoretical; it's practical and actionable. Companies like Netflix have harnessed this power to create highly personalized experiences, such as their content recommendation system, which keeps viewers engaged and subscribed.
To illustrate the effectiveness of statistical algorithms, consider the following benefits they bring to various business aspects:
- Improved decision-making: By anticipating future trends, businesses can make informed strategic choices.
- Security: Predictive models can detect potential threats and vulnerabilities.
- Risk reduction: Identifying potential risks before they materialize allows for proactive mitigation.
- Operational efficiency: Streamlining processes based on predictive insights can lead to significant cost savings.
In the realm of predictive analytics, investment in data governance, quality management, and talent development is crucial. These elements ensure that the insights derived are not only accurate but also actionable, driving growth and fostering a customer-centric business model.
Customer Behavior Prediction
Predictive analytics is revolutionizing the way businesses understand and interact with their customers. By analyzing past purchase history, browsing patterns, and demographic information, companies can create targeted marketing campaigns that resonate deeply with their audience. For example, an e-commerce platform might use predictive modeling to offer personalized product suggestions, enhancing the customer experience and boosting conversion rates.
Personalization at scale is made possible through customer segmentation, allowing marketing efforts to be more relevant to each individual, thus improving engagement and conversion rates. This approach not only fosters customer loyalty but also paves the way for efficient resource allocation.
Predictive models are instrumental in identifying customers likely to churn, enabling businesses to implement proactive retention strategies and significantly reduce churn rates.
Utilizing predictive analytics, businesses can also:
- Identify growth opportunities
- Optimize marketing strategies
- Improve operational efficiency
- Embrace uncertainty
- Track KPIs
- Analyze ROI
- Evaluate business success for continuous growth
Market Demand Alignment
In the realm of predictive analytics, aligning with market demand is crucial for businesses to stay competitive. By leveraging data on consumer buying patterns and preferences, companies can make informed decisions about inventory management and promotional strategies. This alignment leads to tangible outcomes such as increased sales and heightened customer satisfaction.
Personalized content recommendations are a testament to the power of predictive analytics in understanding market demand. Advanced algorithms now deliver hyper-personalized content, fostering a deeper connection between brands and their audiences. For instance, a clothing retailer can use predictive analytics to identify which customer segments are more likely to respond to discounts, crafting targeted campaigns that maximize return on investment (ROI).
The cloud revolutionizes business strategy, offering sophisticated tools for data analysis, scalability, and agility. The benefits are manifold, including cost savings, enhanced security, and fostering innovation for growth and success.
Optimizing marketing channels is another aspect where predictive analytics plays a pivotal role. With numerous channels available, it's essential to identify the most effective ones for reaching the target audience. Data analytics provides insights into channel performance, enabling marketers to allocate resources efficiently and enhance overall marketing effectiveness.
Analyzing Past Behaviors for Future Insights
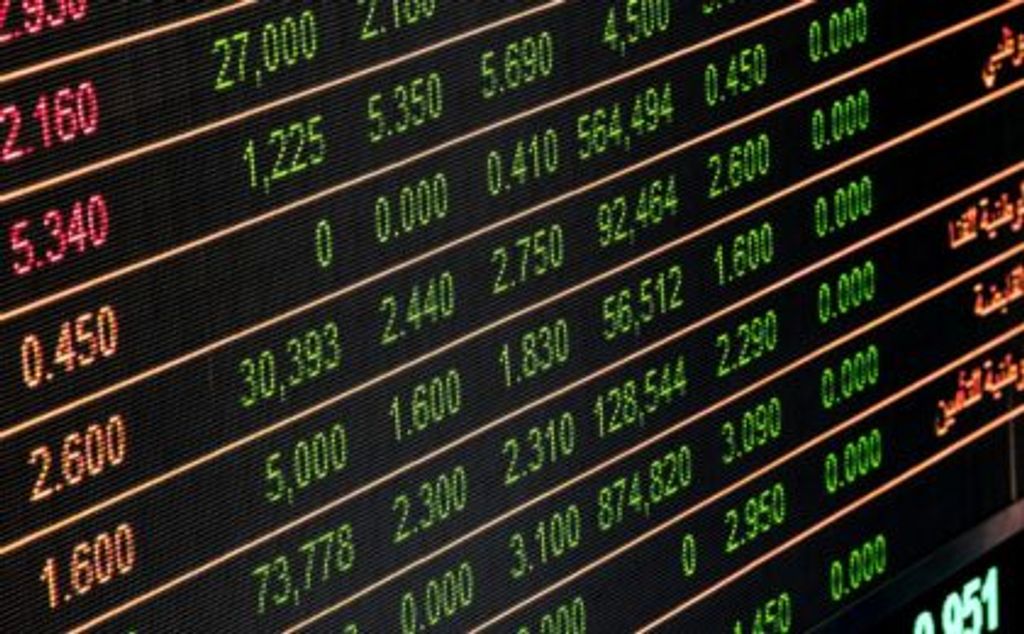
Pattern Identification
In the realm of predictive analytics, pattern identification is a cornerstone for forecasting future trends. By analyzing historical data, businesses can detect recurring sequences that may indicate future events or behaviors. This process often involves the use of anomaly detection techniques to identify outliers that could signify potential issues or opportunities.
- Historical Data Analysis
- Sequence Detection
- Anomaly Identification
Proactive predictive system monitoring improves software reliability by detecting issues early. AI-enabled DevOps collaboration enhances task assignment efficiency. Automated code review and optimization streamline software development.
The insights gained from pattern identification are not only valuable for predicting what might happen but also for understanding why it may occur. This understanding is crucial for crafting strategies that are both responsive and anticipatory.
Risk Mitigation Strategies
In the realm of business, predictive analytics plays a pivotal role in identifying and mitigating potential risks before they escalate into significant issues. By analyzing historical data and identifying patterns, businesses can predict and prevent fraud, detect anomalies, and assess risks. For instance, the use of automation and predictive analytics enhances security by triggering specific measures when suspicious patterns are detected.
The strategic application of predictive analytics allows for timely adjustments to strategies, mitigating risks, and capitalizing on emerging opportunities.
Furthermore, cloud computing contributes to risk mitigation by offering cost savings, scalability, and flexibility. It not only enhances collaboration and improves data security but also optimizes operations, leading to business success. Here's how a retail company applied these principles:
- Market Analysis: Evaluated customer behavior, competitors, and market conditions.
- Risk Identification: Pinpointed regulatory challenges and intense competition.
- Strategic Planning: Devised risk management strategies tailored to the new market.
Process Optimization
In the realm of predictive analytics, process optimization emerges as a critical component for enhancing operational efficiency. By scrutinizing historical data, organizations can pinpoint bottlenecks and streamline workflows, leading to a more efficient and productive environment. Optimization efforts often translate into tangible cost savings and heightened performance.
Predictive models serve as a compass for continuous improvement, guiding businesses to refine processes and eliminate inefficiencies.
For example, consider the impact of predictive analytics on inventory management:
- Identification of overstock and understock patterns
- Forecasting optimal stock levels
- Scheduling restocking to align with predicted demand
These steps not only ensure resource optimization but also prevent the loss of sales due to stockouts or the unnecessary capital tied up in excess inventory. As businesses embrace predictive analytics, they unlock the potential to transform their operations into a well-oiled machine, primed for the challenges of tomorrow.
Predictive Analytics: Meaning and Application
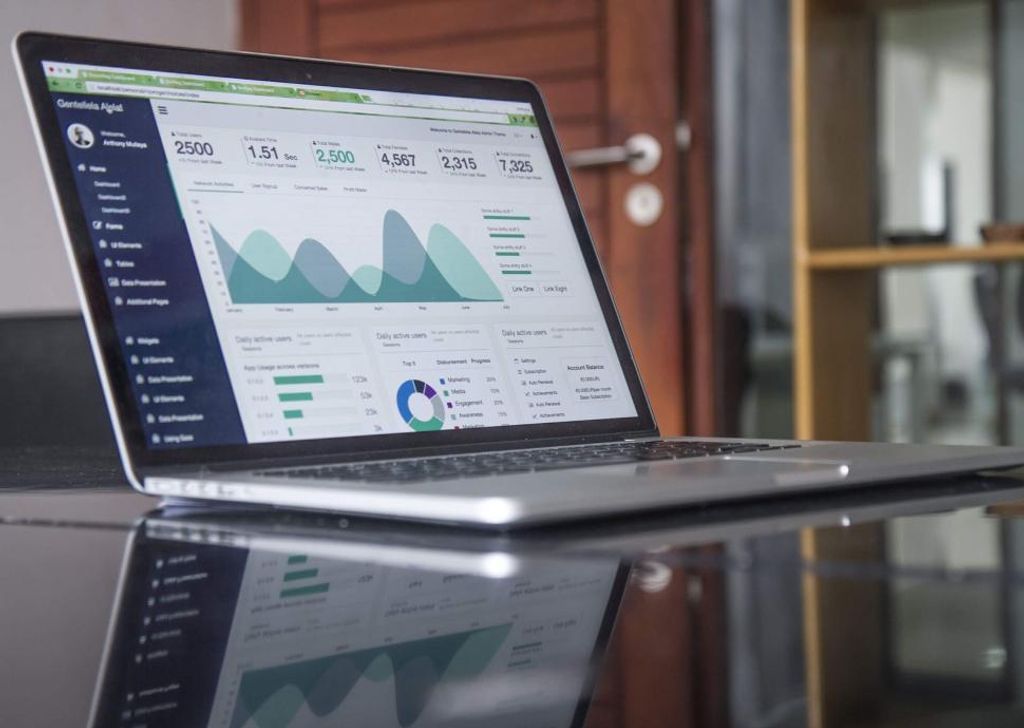
Extracting Valuable Insights
In the realm of predictive analytics, extracting valuable insights from data is the cornerstone of strategic decision-making. By sifting through the noise and identifying the signals, businesses can uncover patterns and trends that inform future actions. This process is not just about having data, but about understanding and interpreting it to make informed decisions.
The true power of predictive analytics lies in its ability to transform raw data into actionable knowledge.
For instance, consider the following steps a business might take to extract insights:
- Collect diverse data from various sources.
- Employ advanced analytics to process and clean the data.
- Utilize statistical models to identify trends and correlations.
- Translate findings into strategic business initiatives.
These steps underscore the importance of a methodical approach to data analysis, ensuring that the insights gained are both reliable and relevant to the business objectives at hand.
Forecasting Trends and Events
Predictive analytics leverages historical data and sophisticated algorithms to not only interpret past behaviors but also to forecast future trends and events. This forward-looking approach is crucial for businesses aiming to stay ahead of the curve in a rapidly changing market environment.
- Decision Trees: Used for classification and regression tasks, providing clear and logical decision paths.
- Regression Models: Estimate relationships between variables, often used for predicting numerical outcomes.
- Neural Networks: Mimic the human brain to recognize complex patterns and make predictions.
By employing these statistical models, companies can anticipate market demands, customer preferences, and potential risks, thereby enabling proactive strategic planning.
The application of predictive analytics extends across various domains, from anticipating customer churn to optimizing product offerings. It empowers organizations to tailor their strategies and operations to meet the evolving needs of their market and customers. The ultimate goal is to transform insights into actionable strategies that drive business growth and competitive advantage.
Statistical Models and Algorithms
The backbone of predictive analytics lies in the sophisticated use of statistical models and algorithms. These tools are designed to sift through historical data, identifying patterns that can forecast future events with a degree of certainty. Key to this process is the selection of the right model for the data at hand, which can range from simple linear regression to complex neural networks, each with its own set of strengths and applications.
Data preprocessing is a critical step in ensuring the quality of the predictive model. Techniques such as data imputation and outlier detection are employed to refine the dataset, addressing issues like missing values and anomalies that could skew the results. Once the data is prepared, various modeling techniques are applied, such as decision trees or random forests, to learn from the data and make informed predictions.
The choice of algorithm greatly influences the predictive power and accuracy of the model, making it a pivotal decision in the analytics process.
Here is an overview of some popular predictive modeling techniques and their typical use cases:
- Decision Trees: Categorizing data based on features; often used for classification problems.
- Regression Models: Estimating relationships between variables; ideal for forecasting numerical outcomes.
- Neural Networks: Recognizing complex patterns and relationships; suitable for image and speech recognition.
- Clustering Models: Grouping similar data points; used for market segmentation.
- Time-series Models: Analyzing time-ordered data; used for stock market predictions or weather forecasting.
Predictive Analytics for Future Planning
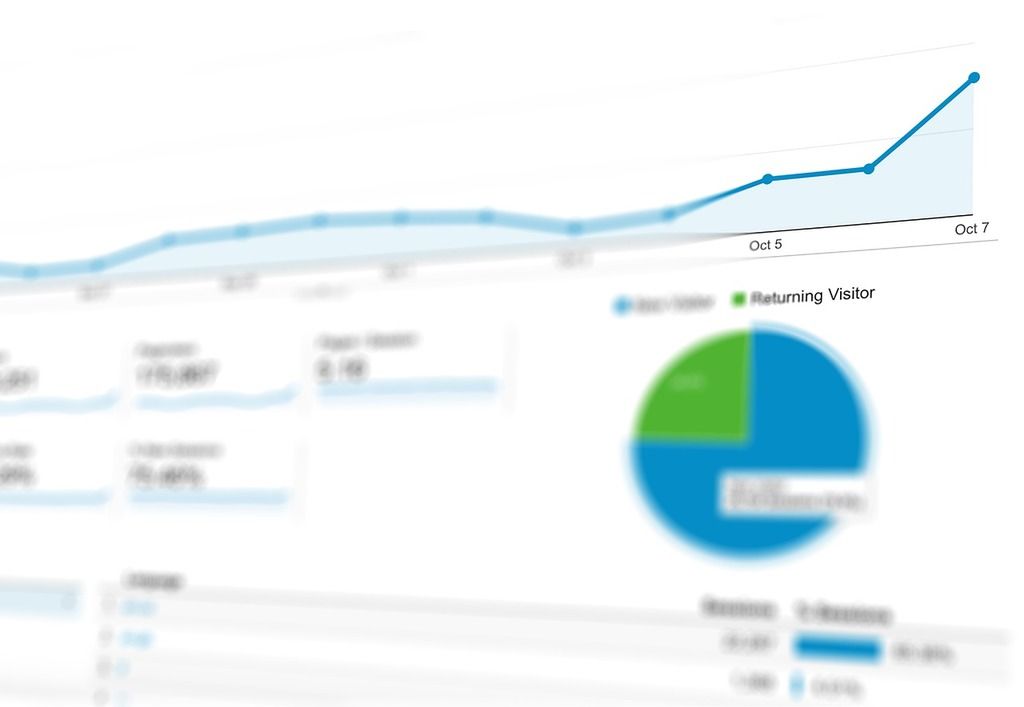
Insights for Marketers
Predictive analytics offers marketers a powerful lens through which they can foresee the impact of their strategies and optimize campaigns for maximum ROI. Enhanced decision-making becomes a reality as predictive modeling provides a forward-looking perspective, steering away from intuition and towards data-driven predictions.
Customer segmentation and personalization are at the forefront of predictive analytics benefits. By understanding and categorizing customers based on behavior and preferences, marketers can tailor their messages to resonate more deeply, leading to higher engagement and conversion rates.
Predictive models are not just about forecasting; they are about creating a strategic advantage in a competitive marketplace.
The integration of top BI tools simplifies data complexities and caters to businesses of all sizes. These tools transform raw data into actionable insights through APIs, automation, and AI, enabling marketers to:
- Securely transfer and integrate data from diverse sources
- Analyze data to discover actionable insights
- Organize data for easy access and analysis
- Maximize marketing ROI and prove the value of marketing strategies
By leveraging predictive analytics, marketers can future-proof their strategies, ensuring they are always one step ahead in anticipating customer needs and market demands.
Anticipating Customer Needs
In the realm of predictive analytics, anticipating customer needs is paramount for businesses aiming to stay ahead of the curve. By leveraging data and analytics, companies can gain a 360-degree view of their customers, enabling them to deliver personalized experiences that meet the evolving demands for privacy, convenience, and sustainability.
The mastery of data not only fuels advanced technology but is also fundamental in executing customer experiences that resonate on a personal level.
To effectively anticipate customer needs, businesses must focus on several key areas:
- Personalization: Crafting unique experiences tailored to individual preferences.
- Responsiveness: Quickly adapting to customer feedback and market changes.
- Predictive Engagement: Utilizing predictive models to foresee and act on customer desires.
These strategies, when implemented correctly, provide a competitive edge by enhancing customer satisfaction and fostering loyalty. The result is a more efficient, customer-centric approach that drives better business outcomes.
Forecasting Demand
Demand forecasting is a critical component of predictive analytics, enabling businesses to anticipate future customer needs and align their strategies accordingly. By leveraging historical data and predictive models, companies can estimate the demand for products or services with greater accuracy, ensuring they are well-prepared to meet market requirements.
The benefits of accurate demand forecasting are manifold:
- Enhanced Decision-Making: Data-driven predictions inform strategic choices, moving beyond intuition to a more reliable, analytical approach.
- Customer Segmentation and Personalization: Tailoring inventory and marketing efforts to distinct customer groups improves engagement and sales.
- Optimal Resource Allocation: Balancing supply with predicted demand minimizes excess inventory costs and maximizes efficiency.
Predictive analytics transforms raw data into actionable foresight, guiding businesses through the complexities of market demand.
Use cases across various industries illustrate the versatility of demand forecasting. Retailers adjust inventory for seasonal trends, healthcare providers anticipate patient needs, and manufacturers predict maintenance schedules. Each scenario underscores the value of predictive analytics in crafting a responsive and strategic business plan.
Redefining Decision-Making with Predictive Analytics
AI-Powered Strategic Decisions
Predictive analytics, now intertwined with artificial intelligence (AI), is revolutionizing strategic decision-making. By sifting through extensive datasets, businesses can forecast market trends and customer behaviors, thus identifying potential opportunities. This capability enables Small and Medium-sized Businesses (SMBs) to make decisions that are both informed and timely, reducing risks and enhancing the effectiveness of their strategies.
The agility afforded by real-time analytics allows for swift adaptation to market changes and optimization of marketing strategies. However, a balance must be struck between the speed of decision-making and the need for comprehensive analysis to prevent hasty, impulsive choices.
AI-driven personalization is a game-changer for SMBs, allowing them to tailor their offerings to meet individual customer preferences. This level of customization can provide a competitive edge, but it also raises ethical considerations around data usage and customer consent.
The following table highlights some key benefits of AI in predictive analytics:
Benefit | Description |
---|---|
Decision-Making | Enhanced by real-time data analysis and recommendations. |
Security | Ensures compliance and protects against data breaches. |
Cost Efficiency | Optimizes resources to reduce unnecessary expenditures. |
Collaboration | Improves teamwork and productivity through shared insights. |
Growth | Drives business expansion by identifying and capitalizing on trends. |
Market Trend Anticipation
Anticipating market trends is a pivotal aspect of business strategy, and predictive analytics serves as a powerful tool in this endeavor. By analyzing historical data and trends, businesses can forecast future market behaviors, enabling them to be proactive rather than reactive. For instance, a retail company might use past sales data to predict seasonal demand, adjusting inventory to meet customer needs while avoiding surplus.
Predictive analytics also plays a crucial role in investment strategies. Investors leverage this data-driven approach to anticipate growth, minimize risk, and achieve long-term sustainability. In the dynamic startup ecosystem, being able to foresee market shifts can be the difference between success and obsolescence.
The integration of predictive analytics into business operations allows for a nuanced understanding of the market landscape. Companies can benchmark performance, identify market gaps, and strategize to gain a competitive edge.
In the realm of B2B marketing, predictive analytics facilitates a machine learning-driven approach to adapt to evolving market dynamics. It empowers proactive decision-making, enabling businesses to anticipate customer needs and seize opportunities as they arise.
Risk Minimization Strategies
In the realm of predictive analytics, risk minimization is a cornerstone for ensuring business resilience and continuity. By leveraging historical data, companies can identify patterns that signal potential risks, allowing them to enact preemptive measures. For instance, creditors use predictive models to assess the likelihood of customer defaults, which informs their lending decisions and helps maintain financial stability.
Operational efficiency is another facet where predictive analytics plays a pivotal role in risk reduction. By streamlining workflows, businesses not only enhance their profit margins but also mitigate risks associated with process failures and inefficiencies. This dual benefit underscores the importance of integrating predictive analytics into business operations.
The strategic application of predictive analytics in risk management not only safeguards against potential threats but also fortifies a company's position in a competitive market.
The following table illustrates the impact of predictive analytics on various risk-related aspects of business:
Aspect | Impact of Predictive Analytics |
---|---|
Data Security | Identification of suspicious patterns triggers security measures |
Credit Risk | Assessment of customer default probabilities informs lending |
Operational Efficiency | Streamlined processes reduce risk and increase margins |
By adopting predictive analytics, organizations can transform their approach to risk management, moving from a reactive to a proactive stance, and thus securing a more predictable and controlled business environment.
Technologies for Predictive Analytics Solutions
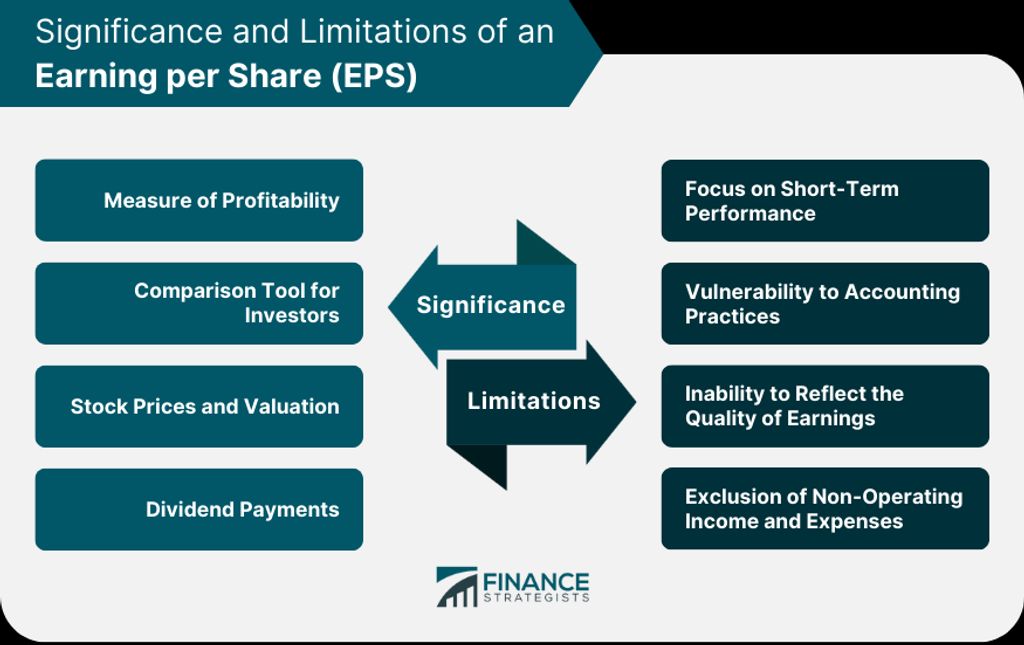
Data Processing and Feature Engineering
The foundation of predictive analytics lies in the meticulous preparation of data, a process known as data processing. This stage is crucial for ensuring the quality and consistency of the data before it enters the predictive models. It involves tasks such as cleaning, which removes errors and inconsistencies, and preprocessing, which includes normalization and handling of missing values.
Feature engineering is a creative step where data scientists transform raw data into meaningful features that significantly enhance the model's predictive power. This can involve generating new variables, such as interaction terms, or applying domain knowledge to extract relevant information.
The art of feature engineering not only enriches the dataset but also uncovers subtle patterns that might otherwise remain hidden.
Here's a simplified overview of the data processing and feature engineering steps:
- Data Cleaning: Addressing missing values, removing duplicates, and correcting errors.
- Data Preprocessing: Normalizing data, encoding categorical variables, and handling outliers.
- Feature Engineering: Creating new features, applying mathematical transformations, and selecting the most relevant features for the model.
Complex Pattern Recognition
In the realm of predictive analytics, Complex Pattern Recognition plays a pivotal role in deciphering intricate relationships within data. Machine learning algorithms excel at identifying these patterns, often hidden and non-linear, which traditional statistical methods may overlook. This capability is crucial for personalization and recommendation systems, where understanding nuanced customer behavior can significantly enhance user experience.
- Structured risk assessment
- Continuous monitoring
- Collaboration
- Resource optimization
These elements are essential for successful enterprise software delivery, and they are intricately linked with the ability to recognize complex patterns. By leveraging advanced analytics, businesses can optimize decision support systems and improve anomaly detection, leading to more informed strategic planning.
The precision of predictive models is heavily dependent on the quality of data. Ensuring data integrity and accuracy is paramount, as the outcomes of predictive analytics are only as good as the data they are based on.
Real-time Predictions
The advent of real-time predictions in predictive analytics has revolutionized the way businesses respond to dynamic market conditions. By leveraging artificial intelligence (AI) and machine learning algorithms, organizations can now make decisions based on data that is updated continuously, allowing for immediate action in areas such as stock market trading, fraud detection, and predictive maintenance.
- Stock Market Trading: Instantaneous analysis for better investment decisions.
- Fraud Detection: Real-time alerts to prevent fraudulent activities.
- Predictive Maintenance: Timely predictions to avoid machinery downtime.
Real-time predictive analytics enables a level of agility and precision that was previously unattainable, empowering businesses to adapt to changes as they occur and maintain a competitive edge.
Conclusion
In conclusion, predictive analytics is a powerful tool that enables businesses to unlock valuable insights, forecast future trends, and drive strategic decision-making. By harnessing the power of data and advanced algorithms, organizations can proactively identify opportunities, mitigate risks, and optimize operations. Predictive analytics goes beyond traditional analytics by focusing on what may happen in the future, allowing businesses to tailor their strategies to align with market demands. With its ability to anticipate customer behavior and forecast events, predictive analytics is reshaping the way businesses approach decision-making and planning for the future.
Frequently Asked Questions
What is predictive analytics and how does it work?
Predictive analytics is the use of data and algorithms to forecast future trends and outcomes based on historical patterns. It works by analyzing past data to make predictions about future events.
How can businesses benefit from predictive analytics?
Businesses can benefit from predictive analytics by making proactive decisions, mitigating risks, optimizing resource allocation, and aligning strategies with market demands. It enables organizations to anticipate customer behavior, forecast demand, and identify opportunities.
What are the key techniques used in predictive analytics?
Key techniques in predictive analytics include statistical algorithms, machine learning, pattern identification, risk mitigation strategies, and process optimization. These techniques help in identifying patterns, predicting outcomes, and optimizing operations.
How does predictive analytics differ from traditional analytics?
Predictive analytics goes beyond traditional descriptive and diagnostic analytics by focusing on forecasting future events rather than just explaining past occurrences. It empowers businesses to take proactive actions based on data-driven insights.
What role does AI play in predictive analytics?
AI plays a significant role in predictive analytics by enabling advanced data processing, complex pattern recognition, and real-time predictions. AI-powered algorithms help in making strategic decisions, anticipating market trends, and minimizing risks.
Can predictive analytics help in marketing strategies?
Yes, predictive analytics can help marketers in understanding customer needs, forecasting demand, and optimizing marketing campaigns. By analyzing data on customer behavior and preferences, businesses can tailor their strategies for better results.
How accurate are predictive analytics forecasts?
Predictive analytics forecasts are generally accurate as they are based on historical data analysis and advanced algorithms. While no prediction is perfect, predictive analytics can provide valuable insights and guidance for decision-making.
What technologies are essential for predictive analytics solutions?
Technologies essential for predictive analytics solutions include data processing, feature engineering, complex pattern recognition, real-time predictions, AI-powered decision support, customer insights, and text data processing. These technologies enable businesses to leverage data for strategic planning and decision-making.