Transforming Supply Chains with AI
Optimizing Logistics with Predictive Analytics
The advent of AI-powered solutions is transforming the logistics landscape, enabling enterprises to anticipate and navigate the complexities of supply chain management. By leveraging predictive analytics, companies can now process vast amounts of data to forecast demand, manage inventory levels, and optimize delivery routes.
AI algorithms can process data from diverse sources, such as market trends and weather forecasts, to predict supply chain disruptions. This proactive approach ensures continuity and efficiency in operations.
The integration of AI into logistics not only enhances operational efficiency but also contributes to significant cost reductions. For instance, predictive maintenance can forecast potential equipment failures, allowing for timely interventions that prevent costly downtimes. Here's how AI is revolutionizing logistics management:
- Forecasting demand to adjust inventory and staffing
- Predicting supply chain disruptions to maintain smooth operations
- Scheduling maintenance to prevent equipment failure
- Optimizing delivery routes for faster and more efficient shipping
Enhancing Transparency and Traceability
In the realm of supply chain management, AI-driven solutions are pivotal in enhancing transparency and traceability. These technologies empower enterprises to monitor their supply chain in real-time, ensuring that every product can be traced back to its source. This not only boosts consumer confidence but also streamlines compliance with regulatory standards.
Key Benefits of AI in Transparency and Traceability:
- Real-time tracking of goods from origin to delivery
- Improved compliance with global standards
- Rapid identification and resolution of supply chain disruptions
By leveraging AI, companies gain the ability to swiftly adapt to changes in the supply chain, minimizing risks and maintaining the integrity of their operations.
Furthermore, AI facilitates the integration of heterogeneous data sources, which is essential for a comprehensive view of the supply chain. This integration is crucial for businesses to remain agile and aligned with business goals, despite the challenges of dealing with diverse datasets, including unstructured and dirty data.
AI-Enabled Inventory Management
The advent of artificial intelligence (AI) in inventory management has revolutionized how enterprises approach stock control and operational efficiency. AI-driven systems are now capable of predicting demand, ensuring that inventory levels are optimized to meet customer needs without excess waste. This predictive capability is not only about maintaining the right stock levels but also about reducing carrying costs and improving cash flow.
- Real-time data analysis for accurate stock predictions
- Automated reordering to prevent stockouts
- Dynamic pricing strategies based on inventory levels
By leveraging AI, businesses can transform their inventory management from a reactive to a proactive strategy, staying ahead of market demands and minimizing overstock scenarios.
The integration of AI into inventory management systems allows for a more nuanced understanding of stock patterns, leading to smarter purchasing decisions and better resource allocation. With AI, the entire supply chain benefits from increased transparency and responsiveness, making it a critical tool for modern enterprises.
Democratization of AI Tools in Manufacturing
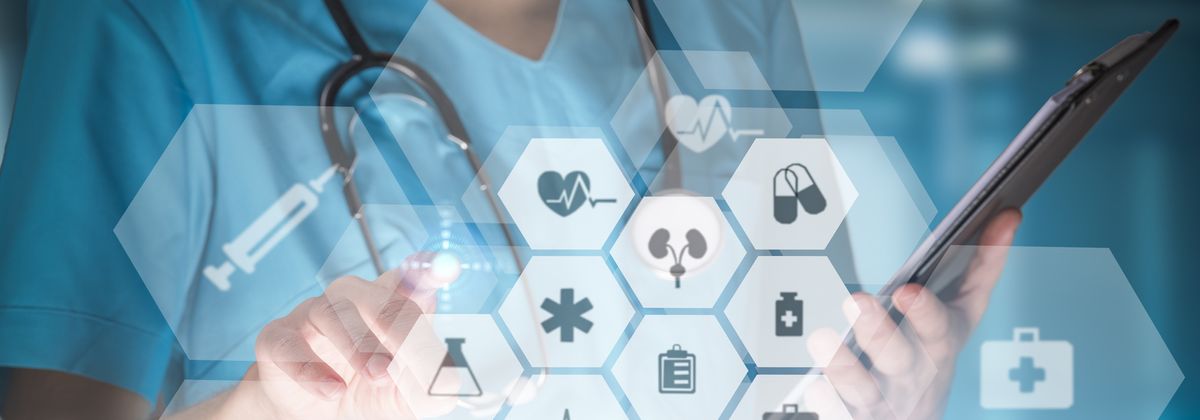
Bridging the Gap for Small and Medium Enterprises
The democratization of AI tools has leveled the playing field, allowing small and medium enterprises (SMEs) to adopt advanced technologies that were once the exclusive domain of larger corporations. AI revolutionizes industries for growth and efficiency, driving strategic decisions with predictive insights. For SMEs, this means an opportunity to innovate and compete on a global scale.
- Accessibility to AI platforms and tools
- Cost-effective solutions for AI integration
- Potential for innovation and global competition
Leadership is crucial for AI adoption and integration into business strategies. SMEs must recognize the importance of strategic planning and the role of AI in shaping their future. By embracing AI, these enterprises can optimize operations, enhance product offerings, and improve customer experiences.
The availability of AI technologies ensures that even the smallest players can harness the power of AI to make significant strides in their respective markets.
Platforms and Tools for AI Integration
The democratization of AI has led to an explosion of platforms and tools designed to facilitate the integration of artificial intelligence into manufacturing processes. Businesses leverage AI for innovation, development, and commercialization, which in turn revolutionizes industry standards in software delivery, driving efficiency and agility. The adoption of AI strategies is crucial for growth and innovation.
The path to successfully deploying AI extends across various domains, requiring collaboration in areas such as infrastructure setup, model training, quality assurance, and security measures.
To navigate this complex landscape, manufacturers can utilize a variety of resources:
- Infrastructure Providers: Offering robust computing power and storage solutions.
- AI Frameworks and Libraries: Simplifying the development of machine learning models.
- Integration Services: Ensuring seamless connection with existing systems.
- Support and Maintenance: Providing ongoing assistance for AI implementations.
Each component plays a vital role in building a cohesive, AI-ready infrastructure that can seamlessly integrate with legacy platforms and handle the diversity and volume of data generated in manufacturing environments.
Fostering Innovation Across the Board
The integration of AI within manufacturing is not just about adopting new technologies; it's about fostering a culture of innovation that permeates every level of the enterprise. Cultivating internal AI expertise is crucial for companies to not only understand the potential of AI but to also drive innovation from within. This expertise ensures that enterprises can effectively evaluate AI solutions and their applications, leading to more informed decision-making.
Partnerships with technology providers are equally important, as they bring in external knowledge and cutting-edge solutions. However, the true potential of AI is unlocked when these partnerships are complemented by a strong internal skill set. This dual approach allows for a nuanced integration of AI, balancing the benefits of external innovation with the empowerment of internal capabilities.
- Understanding the real benefits and applications of AI
- Scrutinizing costs, risks, and data-handling aspects
- Engaging in meaningful conversations with vendors
By embracing both external partnerships and internal capability building, enterprises can navigate the complexity of AI adoption and drive innovation across the board.
The Boundless Potential of AI Use Cases in Manufacturing
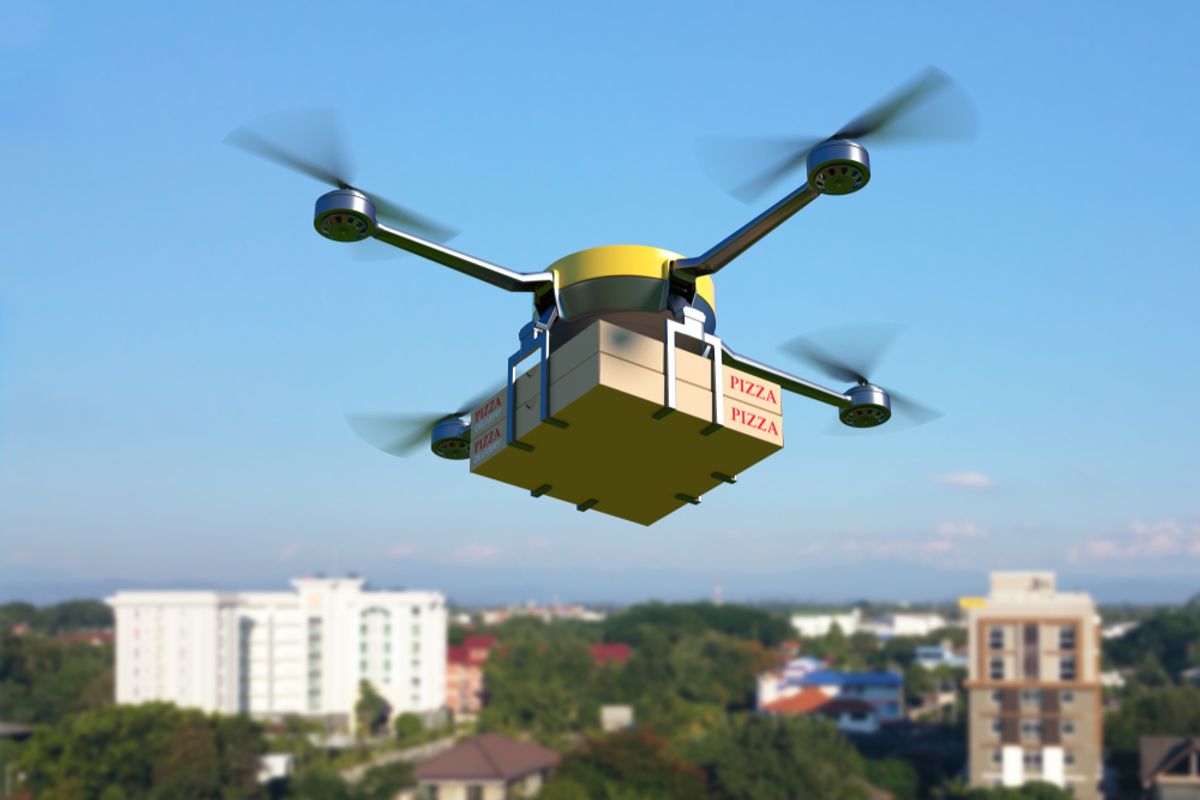
Predictive Maintenance for Equipment
The advent of AI-driven predictive maintenance represents a paradigm shift in manufacturing operations. Predictive failure and maintenance algorithms are not just about avoiding unplanned downtime; they're about ushering in an era of proactive strategy. By analyzing data from equipment sensors, these systems forecast potential failures and schedule maintenance, effectively turning the unpredictable into a planned event.
Predictive maintenance is often cited as the "low hanging fruit" of AI applications in manufacturing, due to its immediate impact on efficiency and cost savings. It's a prime example of how AI can transform reactive measures into a finely tuned anticipatory approach.
The use of AI in predictive maintenance enables a shift from reactive to proactive strategies, leveraging data-driven insights to optimize operations and prevent costly interruptions.
Here are some of the key benefits of implementing AI for predictive maintenance:
- Reduction in unplanned downtime: Minimizing the risk of sudden equipment failures.
- Cost savings: Lowering maintenance expenses by avoiding unnecessary repairs.
- Enhanced safety: Proactively identifying safety risks to prevent accidents.
- Improved equipment lifespan: Regular maintenance can extend the life of machinery.
Quality Control Through Machine Vision
The integration of machine vision into quality control processes represents a transformative leap in manufacturing efficiency. Specialized cameras, paired with AI software, enable real-time analysis of products to ensure they meet stringent quality standards. This synergy of technology not only accelerates inspection times but also enhances accuracy, surpassing the capabilities of human oversight.
Machine vision systems, through advanced image recognition and machine learning algorithms, provide a critical augmentation to the workforce. They uphold regulatory compliance and maintain a consistent standard of quality across production lines.
The implementation of machine vision in quality control involves several key steps:
- Selection of high-resolution cameras and appropriate lighting
- Integration with existing network systems and infrastructure
- Deployment of GPU-enabled edge devices for processing
- Continuous maintenance and updates of AI models
This strategic approach ensures that the impact of machine vision in manufacturing is maximized, leading to significant improvements in product quality and operational efficiency.
Customization and Personalization of Products
In the era of mass customization, AI is the driving force behind the ability to offer highly personalized products without sacrificing production efficiency. AI-driven systems analyze vast amounts of data to predict consumer trends, enabling manufacturers to stay ahead of the curve and offer innovative products that resonate with individual customer preferences.
Augmented reality (AR) and virtual reality (VR) technologies are transforming the customer experience by providing immersive platforms for product customization. This not only enhances customer satisfaction but also reduces the likelihood of returns due to mismatched expectations.
The integration of AI in manufacturing processes allows for a seamless transition from mass production to mass customization, where robots and 3D printing technologies are leveraged to produce custom orders efficiently and accurately.
AI's role extends beyond production, personalizing the delivery experience by optimizing routes and predicting demand patterns. This results in faster, more tailored service, meeting the modern consumer's expectation for quick and individualized delivery.
- Predictive trend analysis
- AR/VR for virtual customization
- Flexible manufacturing with AI automation
- Optimized delivery for personalized service
Advances in AI Algorithms and Infrastructure
Breakthroughs in Neural Networks and Machine Learning
The AI landscape is witnessing a paradigm shift with breakthroughs in neural networks and machine learning. These advancements are not just academic; they are practical, scalable solutions that are revolutionizing how enterprises approach complex problems.
- Enhanced Neural Architectures: New neural network designs are more efficient and accurate.
- Transfer Learning: Pre-trained models are adapted to new tasks, saving time and resources.
- AutoML: Automated machine learning empowers users with limited expertise to develop models.
For areas where data is scarce or sensitive, synthetic data can help simulate scenarios and train AI models without compromising real data. This approach requires careful design to ensure accuracy and avoid biases.
The path to successfully deploying AI extends across various domains, requiring collaboration in areas such as infrastructure setup, model training, and quality assurance. Even straightforward applications like camera vision technology require a detailed strategy for successful integration and ongoing maintenance.
Scaling Computing Power for AI Adoption
The adoption of AI in manufacturing hinges on the ability to scale computing power to meet the demands of sophisticated algorithms. Heavy-duty computing power is a cornerstone for AI applications, enabling the rapid processing of vast datasets and complex calculations. As AI becomes more integrated into manufacturing processes, the need for robust infrastructure becomes paramount.
Infrastructure setup is a critical step in scaling AI, involving the deployment of GPU-enabled edge devices and the integration with existing network systems. This ensures that AI models can be maintained and updated efficiently, facilitating continuous improvement and adaptation to new challenges.
The journey to AI adoption is strategic and multifaceted, requiring investment in new technologies and a commitment to organizational change.
To successfully navigate this journey, manufacturers must consider the following:
- Collaboration across domains, including model training and quality assurance
- Addressing data and integration challenges with legacy systems
- Standardizing and harmonizing data for a cohesive AI-ready environment
Reducing Barriers to AI Adoption
The journey to integrate AI into manufacturing is paved with challenges, but the destination promises significant rewards. Manufacturers must navigate obstacles such as initial investment costs and the complexities of integrating AI with legacy systems. A strategic approach to these challenges involves pilot projects and phased implementation, which can help mitigate disruption and ease the transition.
Integration complexities often stem from the need to harmonize disparate data systems. Creating a cohesive, AI-ready infrastructure requires standardizing data and ensuring compatibility with AI technologies. This process is crucial for leveraging the full potential of AI in manufacturing.
Ethical concerns, particularly around bias in AI algorithms, are also a barrier that must be addressed. Proactive measures, such as diverse data sets and robust testing protocols, are essential to prevent skewed outcomes and ensure fair decision-making.
The democratization of AI tools has made technology more accessible, allowing even small and medium-sized enterprises to compete on a global scale. The table below outlines the key steps to reduce barriers to AI adoption:
Step | Description |
---|---|
1 | Conduct a feasibility study to assess AI readiness |
2 | Implement pilot projects to test AI integration |
3 | Standardize data across systems |
4 | Train the workforce to work alongside AI |
5 | Address ethical considerations proactively |
Enhanced Processing Capability with AI
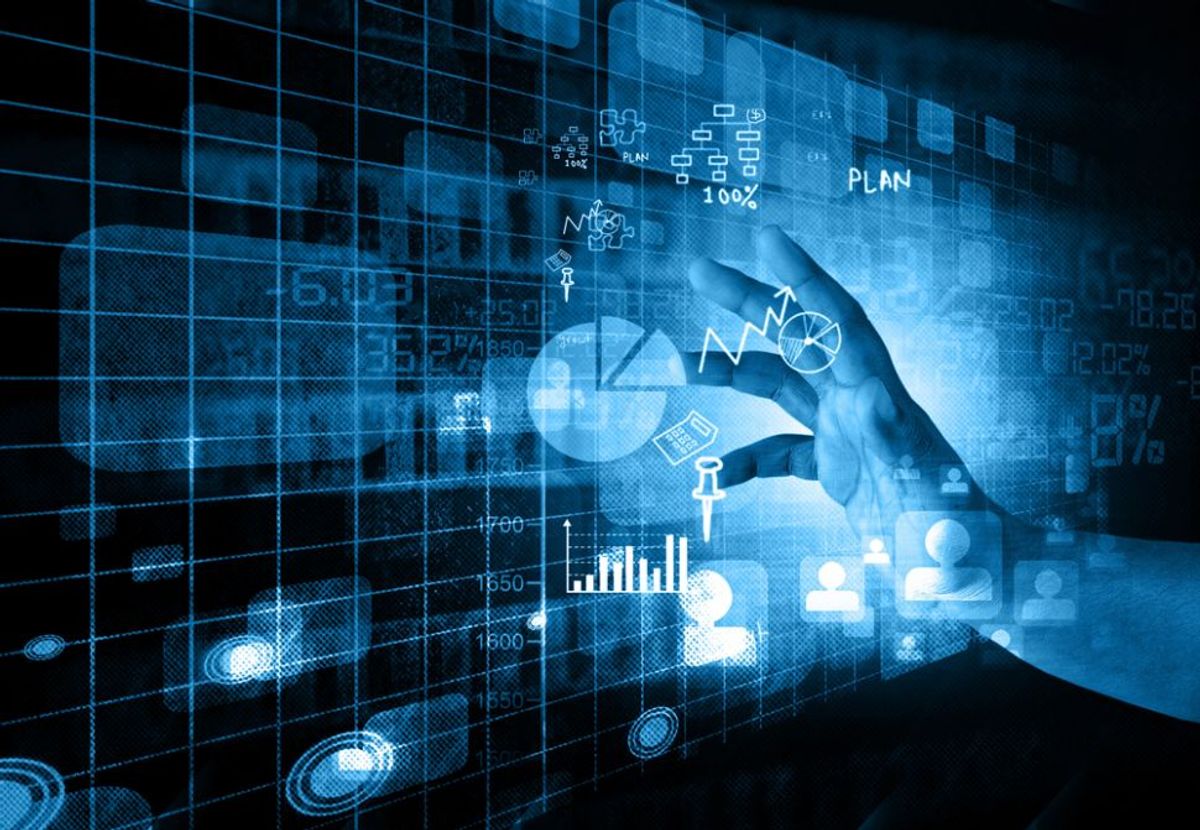
Accelerating Data Analysis for Operational Optimization
In the era of the data explosion, the ability to swiftly analyze and act upon the vast amounts of information generated by manufacturing operations is crucial. AI is transforming this landscape by collapsing the distance between data access and data analysis, enabling businesses to harness the full potential of their data.
AI-driven solutions are adept at navigating the complexities of data capture and analysis, providing manufacturers with the insights needed to optimize operations. These solutions can integrate with pre-existing databases, revealing hidden patterns and generating new information that is critical for informed decision-making.
By enhancing the processing capability of AI, enterprises can achieve operational optimization that is both effective and aligned with business goals.
The following table illustrates the impact of AI on operational efficiency:
Process | Before AI | With AI |
---|---|---|
Data Capture | Time-consuming | Automated |
Data Analysis | Manual | Real-time |
Decision Making | Delayed | Immediate |
Embracing AI not only accelerates data analysis but also ensures that the insights derived are meaningful, even from unstructured or 'dirty' data. This leads to a more flexible and responsive manufacturing environment.
Reducing Waste with Intelligent Systems
The integration of artificial intelligence (AI) into waste management processes is transforming the way enterprises approach sustainability. AI-driven systems are adept at identifying inefficiencies that lead to waste, enabling companies to make smarter decisions that contribute to a leaner, more eco-friendly operation.
By analyzing vast datasets, AI can pinpoint areas where resources are being overused or misallocated. This not only helps in reducing the physical waste but also minimizes energy consumption and lowers operational costs. The result is a significant step towards decarbonizing economies, aligning with global sustainability goals.
AI's predictive capabilities extend beyond just waste reduction, offering a comprehensive approach to resource management that is both proactive and preventive.
The following table illustrates the impact of AI on waste reduction in a manufacturing setting:
Year | Waste Reduction (%) | Energy Savings (%) |
---|---|---|
2021 | 15 | 10 |
2022 | 25 | 20 |
2023 | 35 | 30 |
Adopting AI for waste management not only addresses environmental concerns but also enhances the overall efficiency of manufacturing processes.
Informed Decision-Making in Real-Time
The integration of AI into manufacturing processes has ushered in an era of informed decision-making in real-time. By leveraging the power of AI algorithms, manufacturers can process and analyze vast amounts of data from diverse sources, such as market trends, weather forecasts, and geopolitical events. This analysis not only predicts supply chain disruptions but also informs critical operational decisions.
Real-time analytics enable proactive responses to changing conditions, ensuring that manufacturers maintain efficiency and competitiveness. The ability to anticipate and adapt quickly to market demands is crucial in today's fast-paced manufacturing landscape.
The strategic use of AI for real-time decision-making transforms how manufacturers operate, making them more agile and resilient.
Here are some of the key benefits of AI-driven real-time decision-making:
- Enhanced prediction of supply chain disruptions
- Optimization of inventory control and resource allocation
- Improved energy consumption and cost savings
- Increased ability to meet customer demands promptly
Navigating the Data Explosion in Manufacturing
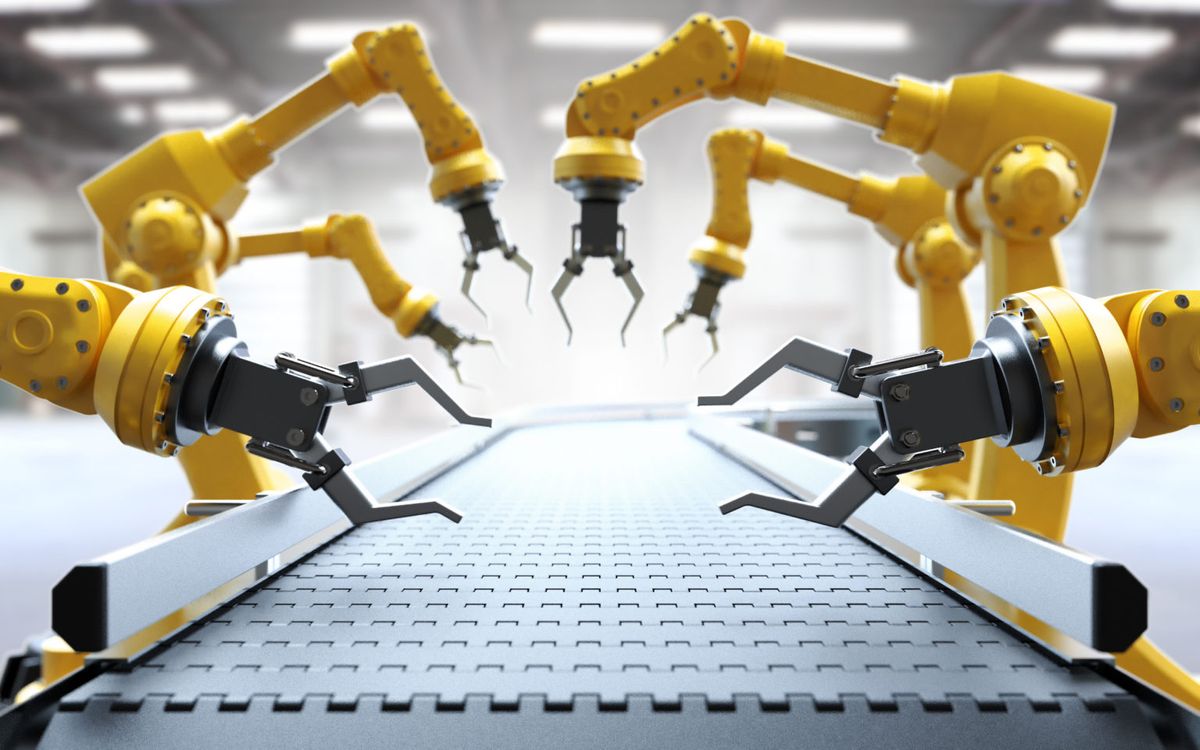
Unlocking Efficiencies with AI-Driven Data Analysis
The modern manufacturing landscape is awash with data, yet the sheer volume often leads to underutilization. AI-driven data analysis stands as a beacon of efficiency, transforming raw data into actionable insights. By harnessing the power of AI, enterprises can not only interpret complex datasets but also anticipate future trends and respond with agility.
AI's capacity to process and analyze data at unparalleled speeds is pivotal for operational optimization. It enables manufacturers to swiftly adapt to changing conditions, ensuring a competitive edge in a dynamic market.
Manufacturers face the challenge of turning the data explosion into a strategic advantage. AI-driven solutions offer a structured approach to this challenge:
- Data Quality Management: Ensuring the integrity and accuracy of data for reliable analysis.
- Pattern Recognition: Identifying trends and anomalies that can inform strategic decisions.
- Predictive Analytics: Using historical data to forecast future events and optimize processes.
The integration of AI into data analysis is not just a technological upgrade; it is a fundamental shift towards a more informed and responsive manufacturing paradigm.
Actionable Insights from Production Metrics and Logistics
The data explosion in manufacturing has led to an unprecedented opportunity for leveraging AI to transform raw data into actionable insights. AI systems can analyze vast amounts of data to identify patterns, predict outcomes, and suggest actions that can help improve manufacturing processes. This capability is not just about having data but about making it work for the enterprise.
By harnessing the power of AI, manufacturers can gain a comprehensive view of their operations, allowing for more informed decision-making. For instance, AI algorithms can process data from market trends, weather forecasts, and geopolitical events to anticipate supply chain disruptions. This foresight is crucial for maintaining seamless operations and optimizing various aspects of manufacturing such as inventory control, staffing, and energy consumption.
The integration of AI into manufacturing logistics goes beyond mere analysis; it empowers businesses to be proactive rather than reactive, adapting to changes with agility and precision.
Here are some ways AI delivers insights:
- Predictive analytics for demand forecasting
- Real-time monitoring of production metrics
- Optimization of supply chain logistics
- Enhanced inventory management through pattern recognition
Complexity Management in Data Capture and Analysis
In the face of the data explosion in manufacturing, the challenge of managing complexity in data capture and analysis is more pressing than ever. AI stands as a beacon of hope, offering the tools necessary to not only collect but also to make sense of the vast amounts of data produced. By leveraging advances in AI algorithms and infrastructure, manufacturers can transform raw data into actionable insights, aligning them with business goals.
The integration of AI into manufacturing systems, particularly legacy platforms, presents a significant hurdle. Yet, it is essential for creating a cohesive, AI-ready infrastructure. Manufacturers must navigate the integration challenges, standardizing and harmonizing data from diverse sources. This process is crucial for unlocking the full potential of AI in manufacturing, enabling the identification of bottlenecks, prediction of equipment failures, and enhancement of operational efficiency.
AI's role in data analytics is not just about processing power; it's about the intelligent interpretation of data, turning information into strategic assets. This requires a thoughtful approach to infrastructure design and optimization, ensuring that systems are robust, flexible, and capable of handling the intricacies of manufacturing data.
Why the Time for AI in Manufacturing is Now
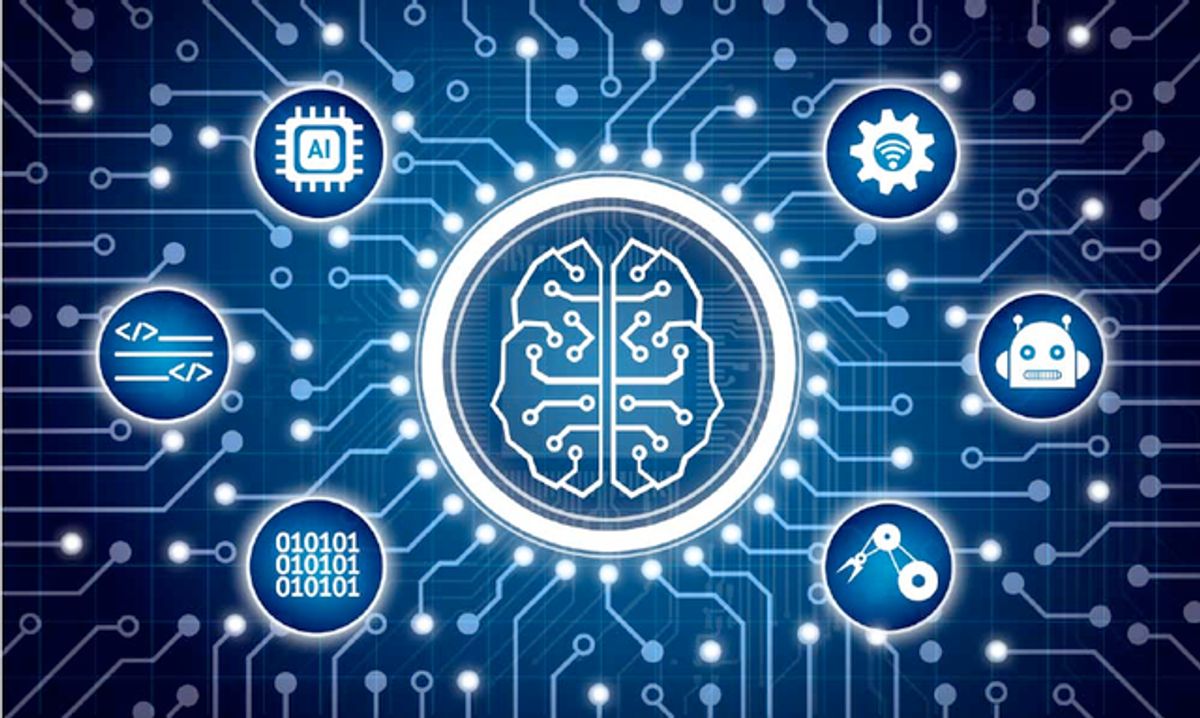
Responding to Economic and Workforce Pressures
In the face of economic and workforce pressures, manufacturers are turning to AI as a beacon of hope. The National Association of Manufacturers has highlighted a projected shortfall of 2.1 million jobs in the next decade, underscoring the urgency for innovative solutions. AI stands as a pivotal tool in bridging this gap, enhancing the capabilities of the existing workforce and driving efficiency.
The introduction of AI in manufacturing raises concerns about job displacement and the need for workforce upskilling. As AI automates routine tasks, the nature of jobs will evolve, necessitating a shift in the skills that workers need to thrive.
To address these challenges, a multi-faceted approach is essential:
- Prioritizing retraining and education initiatives to equip workers with the necessary skills for an AI-augmented environment.
- Implementing AI to automate routine tasks, allowing employees to focus on more complex and rewarding work.
- Fostering a culture of continuous learning and adaptation among the workforce to keep pace with technological advancements.
The integration of AI into manufacturing is not without its hurdles, particularly when it comes to legacy systems. Manufacturers must navigate the complexity of integrating AI with existing platforms, ensuring a seamless transition to a more automated and data-driven future.
Embracing Digital Transformation for Competitive Advantage
In the race to maintain a competitive edge, manufacturers are turning to digital transformation as a critical strategy. Digital transformation in manufacturing maximizes revenue, reduces costs, improves quality, and increases flexibility. It's a journey that intertwines technology with every facet of production, leading to a smarter, more connected, and AI-powered future.
The integration of AI into manufacturing processes is not just an opportunity—it's a necessity for those aiming to stay ahead. By leveraging AI, companies can unlock new levels of performance, fostering innovation and resilience. The strategic approach to this transformation involves a series of steps:
- Identifying key areas for AI application
- Developing a roadmap for technology integration
- Ensuring alignment with business objectives
- Continuously measuring and optimizing AI-driven initiatives
The future of manufacturing is smart, connected, and AI-powered. The time to embark on this journey is now.
However, the path to digital transformation is not without its challenges. Manufacturers must navigate data and integration hurdles, ensuring that their workforce is ready for the technological shift. The rewards, though, are substantial—opening the door to a future of limitless possibilities.
The Strategic Journey Towards AI Integration
The strategic journey towards AI integration in manufacturing is a multifaceted endeavor that requires a comprehensive approach. Collaboration is key in areas such as infrastructure setup, model training, quality assurance, and security measures. Even applications like camera vision technology necessitate a detailed strategy for successful deployment.
A key hurdle is the integration of AI into existing manufacturing systems, many of which are legacy platforms not originally designed for compatibility with AI technologies. Standardizing and harmonizing data across disparate systems is crucial to create a cohesive, AI-ready infrastructure.
While external partnerships are vital, developing internal AI expertise is equally important. Cultivating a well-developed internal skill set ensures meaningful engagement with vendors and a nuanced approach to evaluating AI solutions. This balance between external collaboration and internal proficiency paves the way for a strategic and informed AI adoption.
Here are some steps to consider on the journey towards AI integration:
- Establish strategic partnerships with technology leaders.
- Standardize and harmonize data for AI readiness.
- Develop internal AI expertise for effective vendor engagement.
- Ensure a detailed strategy for infrastructure and application integration.
- Continuously maintain and update AI models for optimal performance.
Overcoming the Hurdles to AI Adoption in Manufacturing
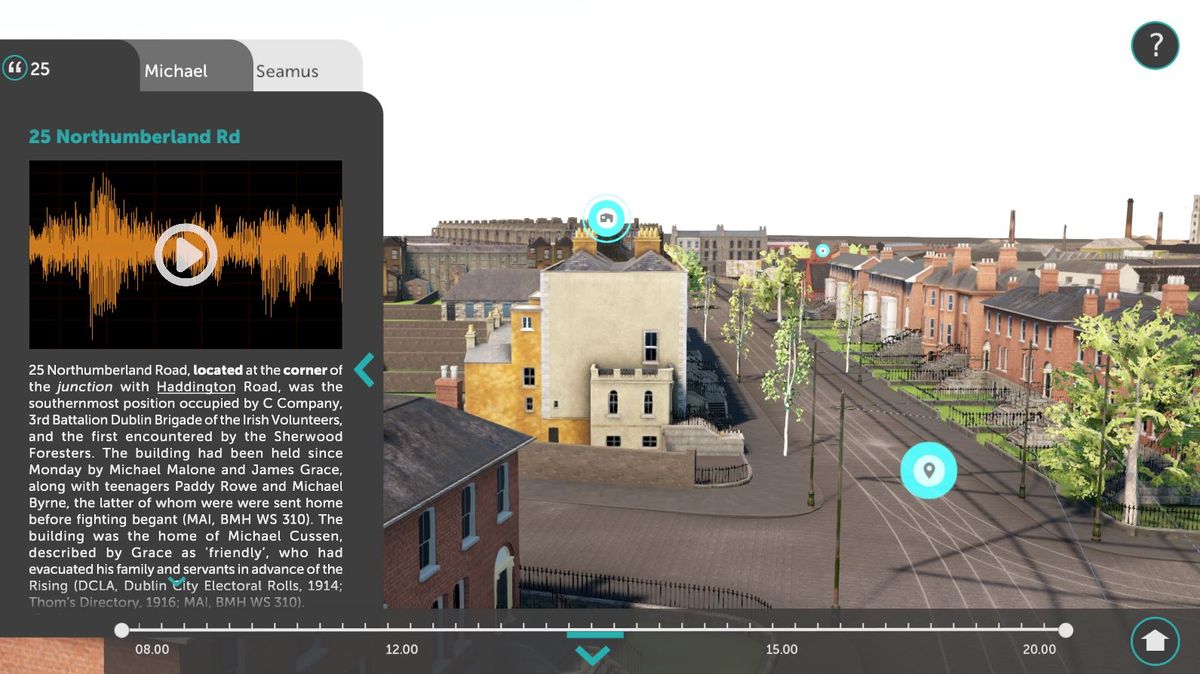
Addressing Data and Integration Challenges
The integration of AI into manufacturing systems presents a complex challenge, particularly when dealing with legacy platforms. Standardizing and harmonizing data is essential for creating an AI-ready infrastructure that can handle the diversity and volume of data in manufacturing.
Data orchestration is a critical step in this process, ensuring that data is accurate, relevant, and aligned with business goals. Manufacturers must navigate the intricacies of diverse datasets, including unstructured and dirty data, to extract meaningful insights.
The strategic approach to data integration involves not only technological solutions but also a focus on workforce training to leverage AI effectively.
By addressing these challenges, manufacturers can pave the way for smarter operations, optimizing efficiency and automation with the help of external expertise.
Customization vs. Efficiency: Striking the Right Balance
In the quest to tailor products and services to individual preferences, enterprises face the challenge of maintaining efficiency while offering customization. AI is pivotal in harmonizing these objectives, enabling businesses to innovate responsively and dynamically. For instance, AI-driven analytics can forecast consumer trends, allowing for proactive product development.
By leveraging technologies such as AR and VR, customers can engage in virtual customization, which not only enhances their experience but also streamlines the production process.
In the realm of production, AI's adaptability is showcased through its ability to adjust manufacturing operations for bespoke orders. This is achieved without sacrificing the pace of production, thanks to advanced robotics and 3D printing technologies. Moreover, AI's predictive capabilities extend to logistics, optimizing delivery routes for a more personalized and efficient service.
The balance between customization and efficiency is not just a technical challenge but also a strategic one, requiring a nuanced approach to data analysis and process design. The table below illustrates the impact of AI on both customization and efficiency in key areas of manufacturing:
Aspect | Customization Impact | Efficiency Impact |
---|---|---|
Product Development | Predictive trends | Streamlined design |
Production Process | Adaptable operations | Accelerated pace |
Logistics | Personalized service | Optimized routes |
Ensuring Workforce Readiness for Technological Change
The integration of AI into manufacturing is not just a technological upgrade but a transformative shift in the workforce dynamics. The need for upskilling is paramount, as AI automates routine tasks and creates new roles that require advanced competencies. Manufacturers must proactively address this by implementing comprehensive training programs.
Retraining initiatives are essential to bridge the skills gap and ensure that employees are equipped to handle the sophisticated systems and analytical tools that AI brings to the table. These programs should focus on both technical skills and the soft skills necessary for a more automated and data-driven work environment.
To mitigate job displacement concerns, it is crucial to foster a culture of continuous learning and adaptability among the workforce.
The following steps can guide enterprises in preparing their workforce for AI integration:
- Assessing the current skill levels and identifying gaps
- Designing tailored training modules for different job roles
- Partnering with educational institutions for specialized courses
- Encouraging cross-functional collaboration to promote knowledge sharing
- Monitoring progress and updating training needs as AI evolves
Customization and Personalization Through AI
Predicting Trends with Data Analysis
The advent of AI-powered predictive analytics is a game-changer for manufacturers, enabling them to anticipate market shifts and consumer demands with unprecedented accuracy. By analyzing diverse data sets, from market trends to geopolitical events, AI algorithms offer personalized insights that drive efficiency and cost reduction.
- Market Trends: Forecasting demand and supply chain needs
- Geopolitical Events: Anticipating disruptions
- Weather Forecasts: Planning for environmental impacts
AI's predictive capabilities are not just about avoiding risks; they're about seizing opportunities to optimize operations and gain a competitive edge.
Manufacturers who leverage AI for trend prediction can adjust their strategies proactively, ensuring they are always one step ahead. This strategic foresight is essential for maintaining a competitive advantage in a rapidly evolving marketplace.
Enhancing Manufacturing Flexibility with AI Automation
The integration of AI in the manufacturing sector has ushered in a new era of flexibility. Robots equipped with AI are now capable of performing complex tasks with precision, catering to custom orders and facilitating on-demand production through technologies like 3D printing. This level of automation not only maintains the speed of manufacturing but also adapts swiftly to changing demands.
AI-driven automation is transforming the delivery process as well. By predicting demand and optimizing delivery routes, manufacturers can ensure quicker and more personalized service to their customers.
The journey towards fully integrating AI into manufacturing is complex, yet the rewards are substantial. Manufacturers that seize the AI opportunity can unlock new levels of performance, fostering innovation and resilience in a competitive digital landscape.
Optimizing Delivery Routes for Personalized Service
The advent of AI in the logistics sector has brought about a significant shift in how delivery routes are planned and executed. Organizations embrace automation for efficiency and innovation, which not only enhances productivity but also elevates the customer experience. By leveraging AI-driven solutions, companies can predict demand patterns and optimize delivery routes, ensuring that services are both quicker and more personalized.
The integration of AI into delivery systems allows for dynamic routing adjustments in real-time, accounting for traffic conditions, weather, and sudden changes in customer preferences.
This level of customization is crucial in today's market, where consumers expect fast and tailored services. AI's predictive capabilities enable businesses to stay ahead of the curve, anticipating customer needs and aligning logistics accordingly. The result is a seamless delivery process that maximizes satisfaction and minimizes wait times.
Improving Workplace Safety with AI Innovations
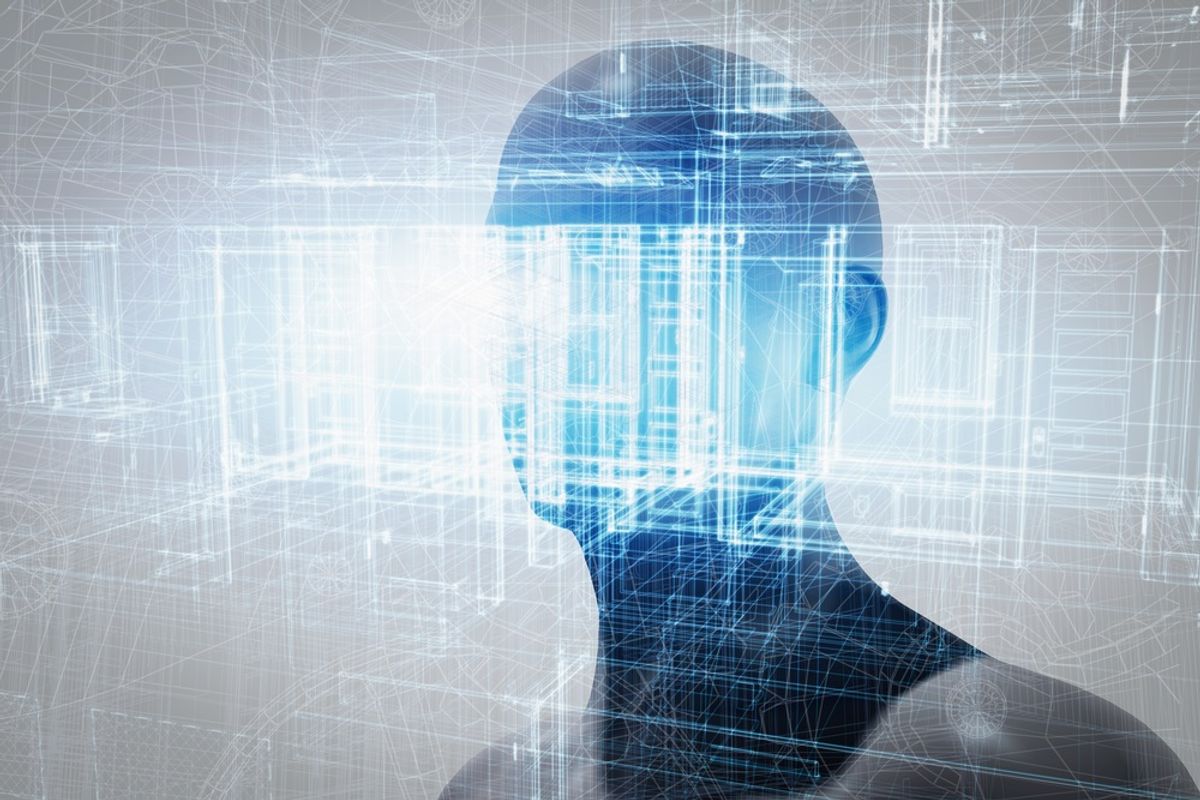
AI in Monitoring and Preventing Workplace Accidents
The integration of AI into workplace safety protocols has marked a significant advancement in preventing accidents and ensuring the well-being of employees. AI-driven systems are now pivotal in detecting whether workers are using personal protective equipment (PPE) correctly, which is crucial for their safety in hazardous environments.
AI also plays a critical role in safety zoning by monitoring restricted areas to prevent unauthorized access. This not only enhances safety but also ensures compliance with regulatory standards. Moreover, predictive maintenance facilitated by AI can anticipate equipment malfunctions, thereby preempting potential workplace accidents.
By analyzing real-time data, AI identifies patterns that could lead to safety risks, allowing companies to take proactive steps to avert incidents before they happen.
The implementation of these AI solutions is not without its challenges, but the benefits they offer in terms of accident prevention and overall safety are undeniable. As AI continues to evolve, its ability to enable smarter decision-making and optimize workforce distribution will become increasingly integral to manufacturing operations.
Robotics and AI in Ensuring Employee Safety
The advent of robotics and AI in the manufacturing sector has been a game-changer for employee safety. Robots are now performing tasks that are considered dangerous, repetitive, or physically demanding, thereby reducing the risk of injuries and accidents on the factory floor. AI-driven systems are also being deployed to monitor safety compliance, such as the use of personal protective equipment (PPE) by employees.
- Detection of PPE compliance
- Safety zoning to prevent unauthorized access
- Real-time monitoring of safety risks
AI augments human capabilities, reduces errors, and improves productivity, leading to cost reduction and efficiency gains across sectors.
Furthermore, AI's predictive capabilities are crucial in anticipating potential hazards. By analyzing data from various sensors and machines, AI can identify patterns that may lead to unsafe conditions, allowing for timely interventions. This proactive approach to safety is transforming how manufacturers address workplace risks, ensuring a safer environment for all employees.
Leveraging AI for Health and Safety Compliance
The integration of AI into health and safety compliance protocols has been a game-changer for manufacturing enterprises. AI-driven systems are now essential for monitoring compliance with safety regulations and standards. For instance, AI can automate the detection of personal protective equipment (PPE) usage, ensuring that workers are adequately protected at all times.
AI's predictive capabilities extend to equipment maintenance, foreseeing potential failures and reducing the risk of workplace accidents. This proactive approach not only enhances safety but also improves operational efficiency.
AI's contribution to safety is not limited to reactive measures. By analyzing workplace data in real-time, AI identifies patterns that may indicate safety risks, allowing for proactive mitigation. This level of surveillance and analysis is crucial in maintaining a safe working environment and adhering to strict safety regulations.
Cybersecurity is another critical aspect where AI bolsters defenses. In the context of enterprise software, particularly Cloud SaaS, AI-driven solutions drive efficiency, innovation, and revenue growth, while ensuring robust security protocols are in place to protect sensitive data and systems.
In the quest to enhance workplace safety, AI innovations are playing a pivotal role. By leveraging cutting-edge technology, businesses can predict potential hazards, automate safety protocols, and create a safer environment for all employees. Don't let your company fall behind in adopting these life-saving advancements. Visit OptimizDBA for unparalleled database optimization consulting and experience the transformative power of AI in safety management. Take the first step towards a safer workplace today!
Conclusion
As we stand on the brink of a new era in manufacturing, the integration of AI-driven software solutions is not just a trend but a transformative force reshaping the corporate tech landscape. The democratization of AI tools, advancements in algorithms and infrastructure, and the ability to process vast amounts of data are revolutionizing supply chains and production processes. Enterprises that seize this AI opportunity can expect to achieve unprecedented levels of efficiency, innovation, and customer personalization. While challenges remain, the potential for growth and competitive advantage is clear. The time for AI in manufacturing is now, and the enterprises that embrace this revolution will pave the way for a future of boundless potential and success.
Frequently Asked Questions
How is AI transforming supply chain logistics?
AI is revolutionizing supply chain logistics by leveraging predictive analytics to optimize routing and delivery schedules, enhance transparency and traceability across the supply chain, and manage inventory levels more effectively.
What does the democratization of AI tools mean for manufacturing?
The democratization of AI tools means that advanced AI technologies are now accessible to a broader range of manufacturers, including small and medium enterprises, enabling them to innovate and compete on a global scale.
Can AI improve equipment maintenance in manufacturing?
Yes, AI can significantly improve equipment maintenance through predictive maintenance techniques, which anticipate potential failures before they occur, thereby reducing downtime and maintenance costs.
What are the recent advances in AI algorithms and infrastructure?
Recent advances in AI include breakthroughs in neural networks and machine learning, as well as the scaling of computing power, which have collectively reduced barriers to AI adoption and made it more cost-effective for manufacturers.
How does AI enhance processing capability in manufacturing?
AI enhances processing capability by accelerating data analysis, which enables operational optimization, waste reduction, and supports faster and more informed decision-making processes.
How can manufacturers manage the data explosion with AI?
Manufacturers can manage the data explosion by using AI-driven data analysis to unlock efficiencies and gain actionable insights from production metrics and logistics, while managing the complexity of data capture and analysis.
Why is now the right time for AI integration in manufacturing?
Now is the right time for AI integration in manufacturing due to economic and workforce pressures, the need for digital transformation to maintain competitive advantage, and the strategic benefits of AI integration.
What are the main challenges to AI adoption in manufacturing?
The main challenges to AI adoption in manufacturing include addressing data and integration challenges, balancing customization with efficiency, and ensuring workforce readiness for technological change.