The Future of Analytics: Predictive Insights for Business Success
The Evolution of Analytics
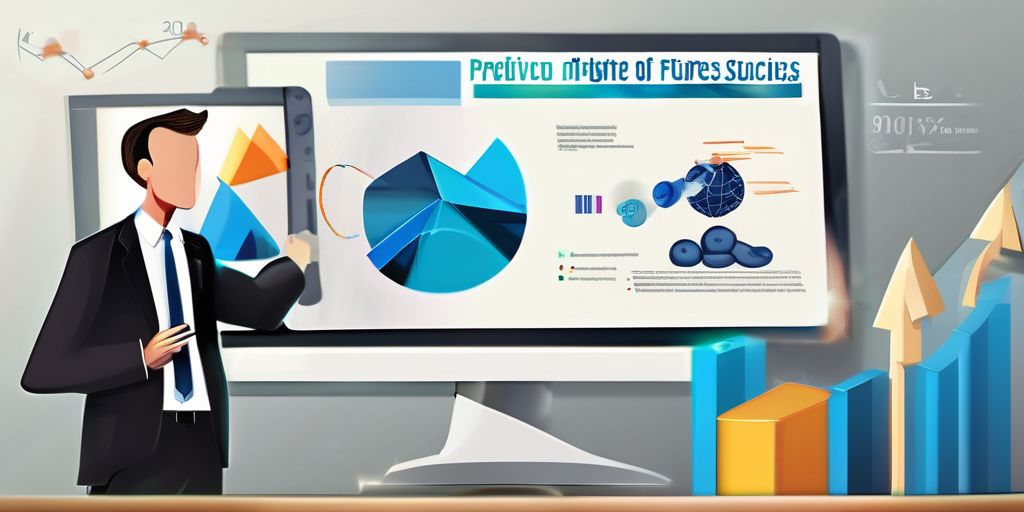
From Descriptive to Predictive Analytics
The field of analytics has evolved from descriptive to predictive analytics. Descriptive analytics focuses on analyzing historical data to understand what happened in the past. It provides insights into trends, patterns, and relationships in the data. However, it falls short in providing actionable insights for the future. Predictive analytics, on the other hand, uses statistical models and machine learning algorithms to make predictions and forecasts based on historical data. It goes beyond just analyzing the past and aims to provide insights into what is likely to happen in the future.
The Role of Machine Learning
Machine learning plays a crucial role in predictive analytics. It enables businesses to leverage the power of data and make accurate predictions for future outcomes. By analyzing large volumes of data, machine learning algorithms can identify patterns and trends that humans may not be able to detect. This allows businesses to gain valuable insights and make informed decisions. With the advancements in AI-driven software delivery, machine learning models can be trained to continuously learn and improve, providing more accurate predictions over time.
Challenges and Opportunities
As businesses continue to embrace predictive analytics, they face various challenges and opportunities. One of the challenges is ensuring MySQL database performance. With the increasing volume of data being processed, it is crucial to optimize the performance of the MySQL database to ensure efficient data retrieval and analysis. This can be achieved through techniques such as indexing, query optimization, and database tuning. By addressing these performance challenges, businesses can unlock the full potential of predictive analytics and derive valuable insights for decision-making.
Implementing Predictive Analytics
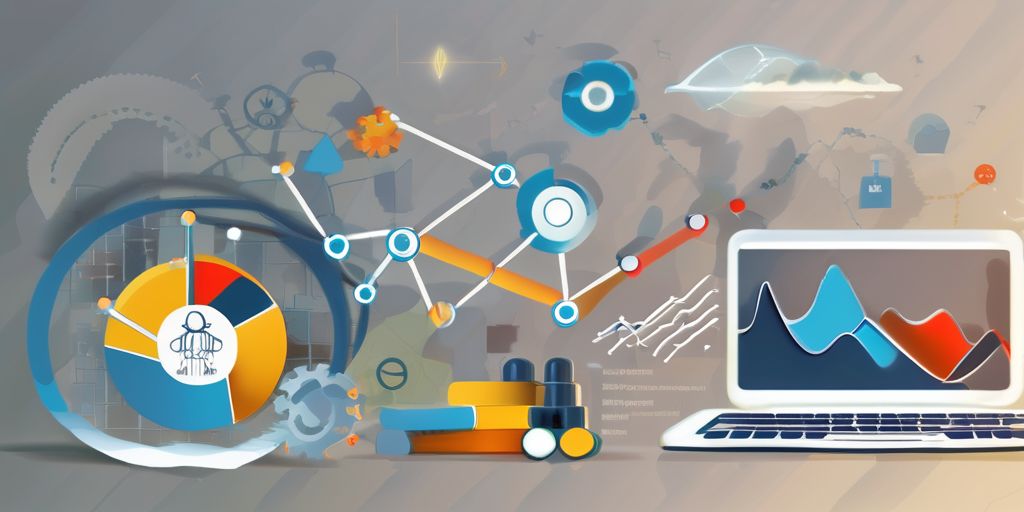
Data Collection and Preparation
Data collection and preparation is a crucial step in implementing predictive analytics. It involves gathering and organizing the necessary data to train the predictive models. This process requires careful consideration of the data sources, ensuring data quality and accuracy, and addressing any missing or incomplete data. Additionally, data preparation involves transforming the data into a suitable format for analysis, which may include cleaning, filtering, and aggregating the data. The success of predictive analytics heavily relies on the quality and preparation of the data.
Choosing the Right Algorithms
When it comes to choosing the right algorithms for predictive analytics, there are several factors to consider. One important factor is the type of data you have and the problem you are trying to solve. Different algorithms are better suited for different types of data and problems. For example, if you are working with structured data and want to perform regression analysis, linear regression algorithms like Ordinary Least Squares or Ridge Regression may be a good choice. On the other hand, if you are dealing with unstructured data and want to classify or cluster the data, algorithms like k-nearest neighbors or support vector machines may be more appropriate.
Building Predictive Models
Building predictive models is a crucial step in implementing predictive analytics. It involves using the collected and prepared data to create models that can make accurate predictions. The choice of algorithms plays a significant role in the success of these models. Different algorithms have different strengths and weaknesses, and selecting the right one is essential. Once the models are built, they need to be evaluated and refined to ensure their accuracy and effectiveness.
Evaluating and Refining Models
Once the predictive models have been built, it is crucial to evaluate and refine them to ensure their accuracy and effectiveness. This process involves analyzing the performance of the models and making necessary adjustments to improve their predictive capabilities. Evaluating the models involves comparing their predictions with actual outcomes and measuring metrics such as accuracy, precision, recall, and F1 score. By identifying any discrepancies or areas of improvement, businesses can refine their models and enhance their predictive insights.
Implementing Predictive Analytics is a crucial step for businesses looking to gain a competitive edge in today's data-driven world. At OptimizDBA Database Optimization Consulting, we specialize in helping companies optimize their databases to achieve faster transaction speeds. With our expertise and experience, we can ensure that your database performs at least twice as fast as before, with average speeds often reaching 100 times, 1000 times, or even higher! Our remote DBA services have been trusted by over 500 clients since 2001, making us a leader in the industry. If you're ready to experience a significant increase in performance, contact us today and let us help you unlock the full potential of your database.