The Evolution of Data Analytics in Business
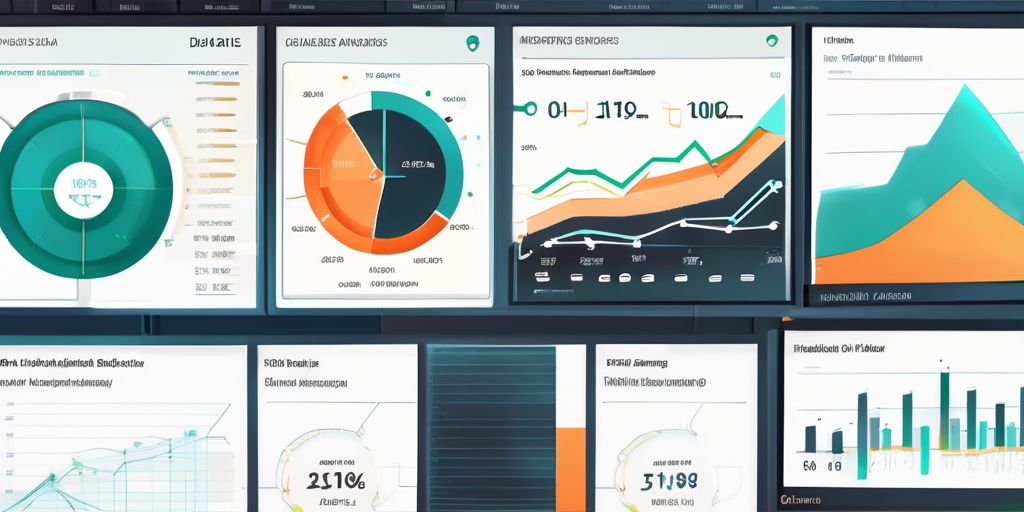
From Descriptive to Prescriptive Analytics
The journey from descriptive to prescriptive analytics marks a significant shift in the business intelligence landscape. Initially, organizations relied on descriptive analytics to understand past performance, summarizing historical data into understandable metrics. As the analytics maturity curve progressed, the focus shifted towards predictive analytics, which employs statistical algorithms and machine learning to forecast future outcomes.
However, the real game-changer has been the advent of prescriptive analytics. This advanced form of analytics not only predicts what might happen but also suggests actions to influence those outcomes. It leverages a combination of techniques, including simulation, optimization, and rules-based systems, to recommend the best course of action.
Adoption of data-driven decision-making is crucial in this transition. It involves integrating facts and insights from various data sources to inform business strategies. Here's a simplified process flow:
- Data Collection: Aggregating data from diverse sources such as internal databases, social media, and IoT devices.
- Data Analysis: Using predictive analytics to identify potential future scenarios.
- Decision-Making: Applying prescriptive analytics to determine the best actions to achieve desired business objectives.
Tip: Start small with prescriptive analytics by focusing on specific business processes where quick wins can demonstrate value and build momentum for wider adoption.
The Rise of Self-Service Analytics Platforms
Modern business intelligence tools like Tableau, Mode, and Looker emphasize visual exploration, dashboards, and self-service analytics. The movement to democratize data is in full swing, enabling more individuals within organizations to access and leverage data for decision-making.
No-Code Solutions
No-code and low-code tools are transforming the big data analytics space by removing the need for coding knowledge. These tools empower stakeholders to work with data without relying on data teams, freeing up data scientists for more complex tasks.
- Implement a table for presenting structured, quantitative data. Ensure it's succinct and formatted correctly in Markdown.
- Use a bulleted or numbered list for less structured content, like steps, qualitative points, or a series of related items.
"Be ready for the future of big data analytics"
- More accessible data: with the arrival of new functions, aimed at making data more accessible, BI had an advantage in terms of growth, because previously data analysis had remained in the hands of private profiles.
- Information cloud: one of the most notable trends in BI is its adoption of cloud software, which requires information technology (IT) teams to continue operating and providing income to key business applications.
- Rise of Analytics: The enhancement of analytics promises an exciting future for data-driven businesses.
The Impact of Big Data on Business Decisions
The advent of Big Data has been a game-changer for business intelligence, offering unprecedented insights into customer behavior, market trends, and internal processes. With the ability to process and analyze vast amounts of data, organizations are now equipped to make more informed and strategic decisions.
Big Data analytics has shifted the focus from gut-driven to data-driven decision-making, enabling companies to predict future trends and customer needs with greater accuracy. This shift has led to enhanced operational efficiency and the creation of more personalized consumer services.
Tip: Always ensure the accuracy and integrity of your Big Data sources to maintain the quality of insights derived.
Here are some key benefits of leveraging Big Data in business decisions:
- Identification of new revenue opportunities
- Optimization of marketing strategies
- Improved customer engagement
- Streamlined operational processes
As Big Data continues to evolve, its impact on business intelligence and decision-making processes will only grow stronger, paving the way for more agile and competitive business practices.
Artificial Intelligence and Machine Learning
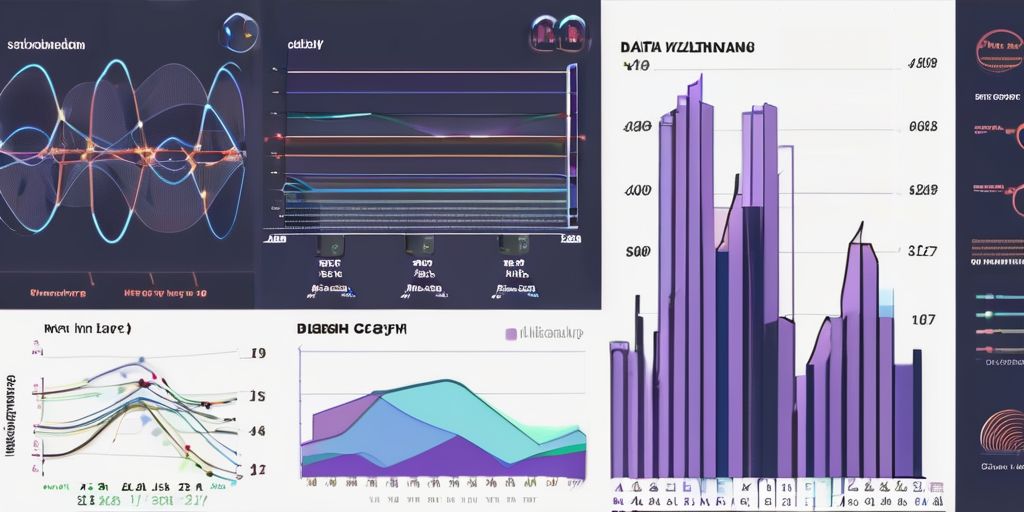
Predictive Analytics and Customer Insights
Predictive analytics and customer insights play a crucial role in understanding customer behavior and making informed business decisions. By leveraging predictive analytics, organizations can use historical data to forecast future outcomes, identify customer preferences, and preemptively address their needs. This enables companies to reduce the risk of customer churn and offer tailored solutions to retain customers. Additionally, predictive analytics involves techniques such as data mining and machine learning to uncover patterns and insights, ultimately leading to proactive decision-making.
- Data Mining: Involves exploring large data sets to find consistent patterns or systematic relationships between variables. It is used for segmenting customers, detecting fraud, and identifying potential market opportunities.
- Machine Learning: Focuses on developing algorithms that can make forecasts based on historical data, ultimately leading to better outcomes and informed decisions.
Tip: Implementing predictive analytics allows companies to reduce resource wastage, increase profitability, and make effective forecasts by leveraging historical data and customer insights.
AI-Driven Forecasting Models
Predictive analytics is a way to generate better outcomes, make more informed decisions that are based on data, and reduce the risk of failure and loss. Predictive analytics tools help companies to increase their profitability by reducing the wastage of resources and making effective forecasts.
Adoption of data-driven decision-making
Data-driven decision-making is the process of incorporating facts and various information extracted from multiple sources of data in making business decisions. It uses multiple parameters and metrics to make business plans and strategies in a structured and informed manner.
Machine Learning for Fraud Detection
The application of Machine Learning (ML) in fraud detection represents a paradigm shift in how businesses safeguard their operations. By leveraging algorithms that learn from data, ML can identify patterns indicative of fraudulent activity with a precision that traditional methods struggle to match. This adaptive learning capability means that the system continuously improves, becoming more adept at spotting complex fraud schemes over time.
Fraud detection models typically process vast datasets, looking for anomalies that signal potential fraud. These models are trained on historical data, which includes examples of both legitimate transactions and confirmed cases of fraud. As they learn, the accuracy of their predictions increases, which is crucial for maintaining trust and security in digital transactions.
Tip: Regularly updating ML models with new data is essential to keep pace with the evolving tactics of fraudsters.
The benefits of implementing ML for fraud detection are numerous:
- Enhanced detection accuracy
- Real-time fraud prevention capabilities
- Reduced false positives, improving customer experience
- Scalability to handle growing data volumes
As businesses continue to digitize, the role of ML in fraud detection will only become more integral, offering a robust defense against the ever-changing landscape of cyber threats.
The Integration of Business Intelligence and IoT
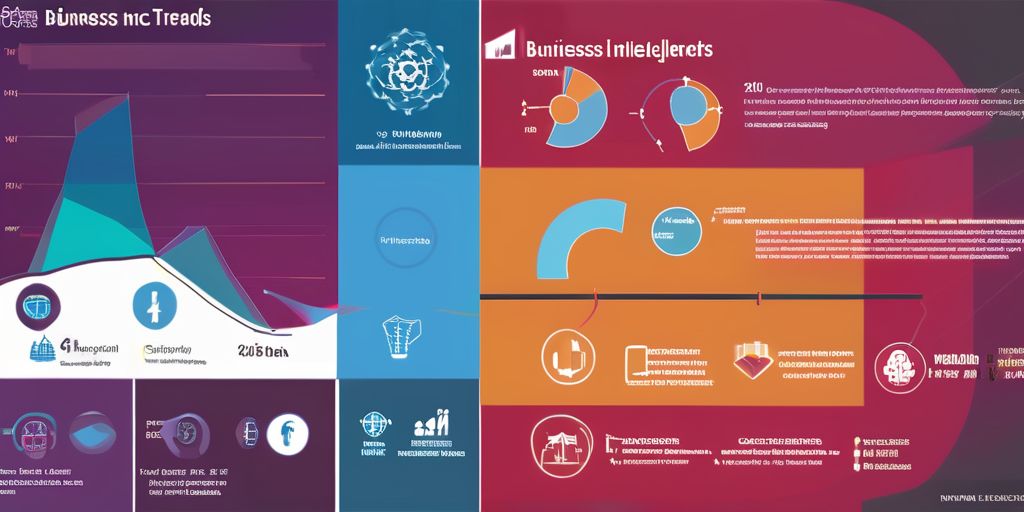
Real-Time Data Analysis from IoT Devices
Accessing real-time data for analysis has become a game-changer across various industries. Gone are the days when making decisions based on historical data was sufficient. Imagine trading Bitcoin based on last week's prices or crafting social media content based on trends from a month ago. Real-time data has already transformed industries like finance and social media, and its applications continue to expand. For example, Walmart has built a massive hybrid cloud infrastructure to manage supply chains and analyze sales in real time. This allows them to react swiftly to market changes and customer demands. Real-time, automated decision-making is becoming the norm, with machine learning and artificial intelligence playing a crucial role. The increasing velocity of big data analytics has shifted the focus to data freshness, enabling better-informed decisions and increased competitiveness. Implementing a table for presenting structured, quantitative data can enhance the analysis of real-time data, providing a clear visual representation of key metrics and trends.
Enhancing Operational Efficiency with IoT
The integration of IoT technology in business operations has led to significant improvements in operational efficiency. Real-time data analysis from IoT devices allows for quick decision-making and proactive maintenance. Additionally, IoT enables the aggregation of data from various sources such as internal databases, social media, and more, providing a unified view of critical information. This streamlined data processing and storage ensures that data is ready for analysis, ultimately enhancing operational agility and guaranteeing customer satisfaction. Implementing IoT technology reduces decision-making times, improves processes, and allows employees to focus on more important tasks. It also increases efficiency and operational agility. Machine Learning, a scientific discipline in the field of Artificial Intelligence, plays a crucial role in identifying complex patterns in the vast amount of data generated by IoT devices, thereby improving predictive models for proactive business strategies.
IoT-Enabled Predictive Maintenance
IoT-enabled predictive maintenance systems leverage data-driven insights to predict equipment failures before they occur. These systems play a crucial role in enhancing operational efficiency and preventing disruptions in manufacturing processes. By automatically rerouting production processes when equipment failures are predicted, businesses can minimize downtime and maintain seamless operations. Additionally, the use of machine learning and AI-driven systems in predictive maintenance is transforming industries by enabling proactive strategies and automated decision-making. Implementing a table for presenting structured, quantitative data may provide a succinct overview of the impact of predictive maintenance on operational efficiency and downtime prevention. Furthermore, a bulleted list can highlight the key benefits of IoT-enabled predictive maintenance systems, such as proactive strategies, automated decision-making, and operational resilience. It's important to note that ethical considerations and data privacy should be prioritized when implementing predictive maintenance systems to ensure responsible and transparent use of data. As organizations continue to adopt IoT-enabled predictive maintenance, they should focus on building trust with ethical data practices and ensuring compliance with privacy laws and regulations.
Cloud Computing and Business Intelligence
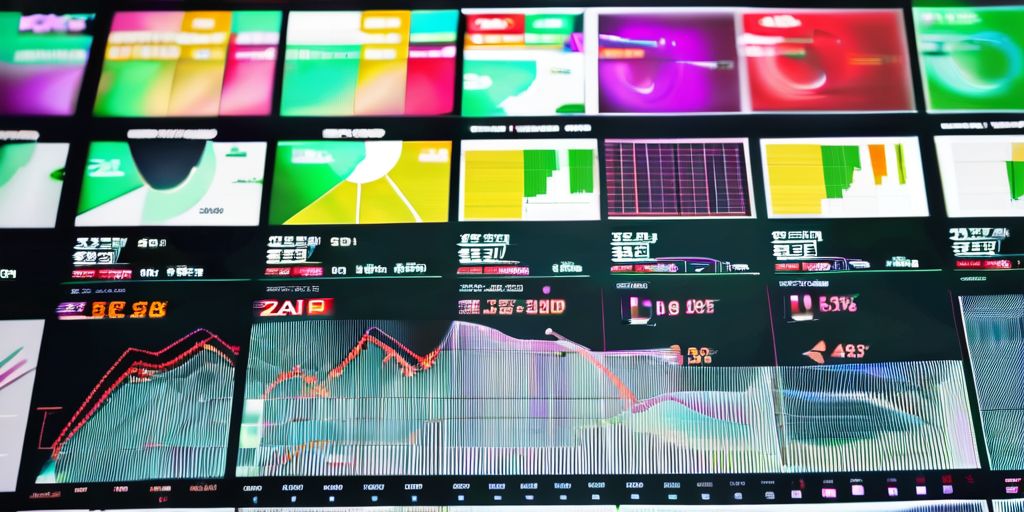
The Shift to Cloud-Based BI Solutions
The adoption of cloud software in business intelligence has transformed the way organizations handle data storage and processing. With cloud platforms like Snowflake, Redshift, and BigQuery, businesses now have access to virtually infinite storage and processing capabilities, eliminating the need to worry about physical storage limitations or acquiring additional hardware. This shift to cloud-based solutions enables multiple stakeholders to access data simultaneously without performance bottlenecks, leading to improved accessibility and security. Organizations can now deal with almost limitless amounts of new data, allowing for enhanced scalability and flexibility. Cloud-based services offer benefits such as cost savings, improved efficiency, and strategic decision-making, making it a pivotal trend in the evolution of business intelligence. The rise of cloud computing has also led to the optimization of data storage systems for all the V's of big data, further enhancing the potential for data-driven businesses to thrive.
Benefits of Scalable BI Infrastructure
BigQuery offer virtually infinite storage and processing capabilities. Cloud-based data processing enables multiple stakeholders to access data simultaneously without performance bottlenecks. This accessibility, combined with robust security measures, allows organizations to access up-to-the-minute data from anywhere, facilitating data-driven decision-making. Processing Data Variety With the surge in data volume comes an increase in data variety. Data can originate from various sources, and managing diverse data formats can be challenging. Fortunately, tools like Fivetran provide data quality monitoring and testing, empowering data producers and consumers to self-serve. Storage and Analytics Platforms Cloud technology has revolutionized data storage and processing. Businesses no longer need to worry about physical storage limitations or acquiring additional hardware. Cloud platforms like Snowflake, Redshift, and BigQuery offer virtually infinite storage and processing capabilities. Cloud-based data processing enables multiple stakeholders to access data simultaneously without performance bottlenecks. This accessibility, combined with robust security measures, allows organizations to access up-to-the-minute data from anywhere, facilitating data-driven decision-making. From enhancing operational efficiency to predicting future trends, BI empowers businesses to harness the true potential of their data. As the landscape evolves, staying abreast of Business Intelligence trends and continuously optimizing BI strategies will be key to maintaining a competitive edge. Why wait to transform your data into decisive power? Start with Grow’s 14-day free trial and explore the intuitive, customizable dashboards that make data analysis accessible and actionable for your dynamic business needs. And for added confidence, see how Grow stands out in the market by reading the user behavior and supply chain suspensions. More accessible data: with the arrival of new functions, aimed at making data more accessible, BI had an advantage in terms of growth, because previously data analysis had remained in the hands of private profiles. Information cloud: one of the most notable trends in BI is its adoption of cloud software, which requires information technology (IT) teams to continue operating and providing income to key business applications. Rise of Analytics: The enhancement of analytics promises an exciting future for data-driven businesses. Augmented analytics
Security Considerations for Cloud BI
As businesses increasingly adopt cloud-based Business Intelligence (BI) solutions, security remains a paramount concern. The shift to the cloud offers numerous advantages, such as scalability and remote accessibility, but it also introduces new challenges in safeguarding data.
Data encryption, both at rest and in transit, is a fundamental security measure that must be rigorously implemented. Additionally, robust access controls and identity management ensure that only authorized personnel can access sensitive information. Regular security audits and compliance with industry standards are essential to maintaining a secure cloud BI environment.
To illustrate the importance of security in cloud BI, consider the following points:
- Ensuring compliance with data protection regulations
- Implementing multi-factor authentication (MFA) for user access
- Utilizing advanced threat detection and response mechanisms
- Adopting a comprehensive data governance framework
Tip: Always have a clear incident response plan in place. This will minimize the impact of any security breach and ensure business continuity.
Data Visualization and Interactive Reporting
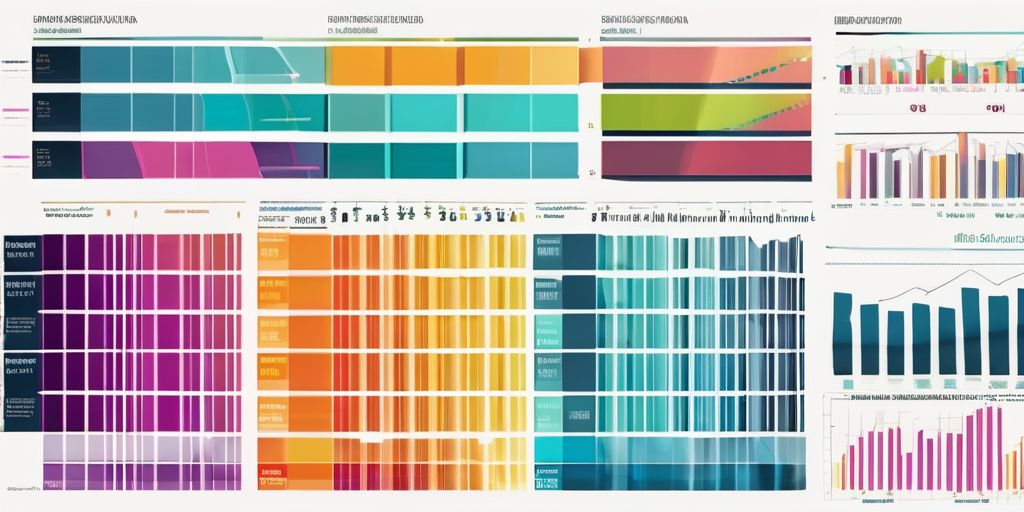
Advancements in Data Presentation
The landscape of data presentation is evolving rapidly, with a focus on making complex data sets more accessible and understandable. Data democratization is a key trend, enabling users across various levels of an organization to engage with data directly. This shift is supported by the development of intuitive visualization tools that allow for dynamic interaction with data.
Modern BI tools have made significant strides in dashboarding capabilities, which are crucial for managing and tracking large volumes of information. These dashboards are not just static displays; they are increasingly interactive, allowing users to drill down into specifics and uncover deeper insights. For instance, tools like Tableau and Domo offer features that facilitate visual exploration and self-service analytics.
No-code and low-code platforms are also gaining traction, simplifying the process of working with data for non-technical users. This empowers more stakeholders to make data-driven decisions without the bottleneck of relying on specialized data teams. Here's a brief list of benefits these advancements provide:
- Enhanced user autonomy in data analysis
- Reduced dependency on IT and data science teams
- Quicker turnaround times for insights and decision-making
Tip: Embrace the power of interactive dashboards to not only view but also to manipulate and explore your data, leading to more informed business strategies.
Interactive Dashboards and Real-Time Reporting
Data Visualization Tools: These are essential in BI for converting complex data sets into graphical representations. They make data more accessible and understandable. For instance, a business intelligence dashboard is a data visualization tool that aggregates and displays key metrics and KPIs in real-time, allowing managers to make quick, informed decisions.
- Implement a table for presenting structured, quantitative data. Ensure it's succinct and formatted correctly in Markdown.
- Use a bulleted or numbered list for less structured content, like steps, qualitative points, or a series of related items.
Streamline your strategy and surge ahead with Grow — your command center for real-time insights and market-leading solutions.
Grow’s BI tools come into play here, analyzing data with advanced techniques to draw out deep insights. The platform’s user-friendly interface on the business intelligence dashboard allows for complex data interaction, making analysis both accessible and interpretable to all business users.
Insight Generation and Decision Making: The final stage is where insights are translated into actionable business decisions or strategies.
The Role of Storytelling in Data Visualization
Effective data visualization tools and reporting platforms are essential for communicating findings to stakeholders. Analyzing data for business insights involves using structured and less structured content, identifying patterns and trends, and ensuring data accuracy. Modern business intelligence tools like Tableau, Mode, and Looker emphasize visual exploration, dashboards, and self-service analytics. The movement to democratize data is in full swing, enabling more individuals within organizations to access and leverage data for decision-making. No-Code Solutions No-code and low-code tools are transforming the big data analytics space by removing the need for coding knowledge. These tools empower stakeholders to work with data without relying on data teams, freeing up data scientists for more complex tasks. No-code solutions promote data-driven decisions throughout the organization, as data engagement with a data lake strategy that can aggregate a wide range of information across many sources. Enterprises are also seeing innovations in the area of data visualization. People understand the meaning of data better when it's represented in a visualized form, such as charts, graphs and plots. Emerging forms of data visualization are putting the power of AI-enabled analytics into the hands of even casual business users. This helps organizations spot key insights that can improve decision-making. Advanced forms of visualization and analytics tools even let users ask questions in natural language, with the system automatically determining the right query and showing the results in a context-relevant manner. Generative AI and large language models (LLMs) improve an organization's data operations even more with benefits across the entire data pipeline. Generative AI can
The Role of Blockchain in Business Intelligence
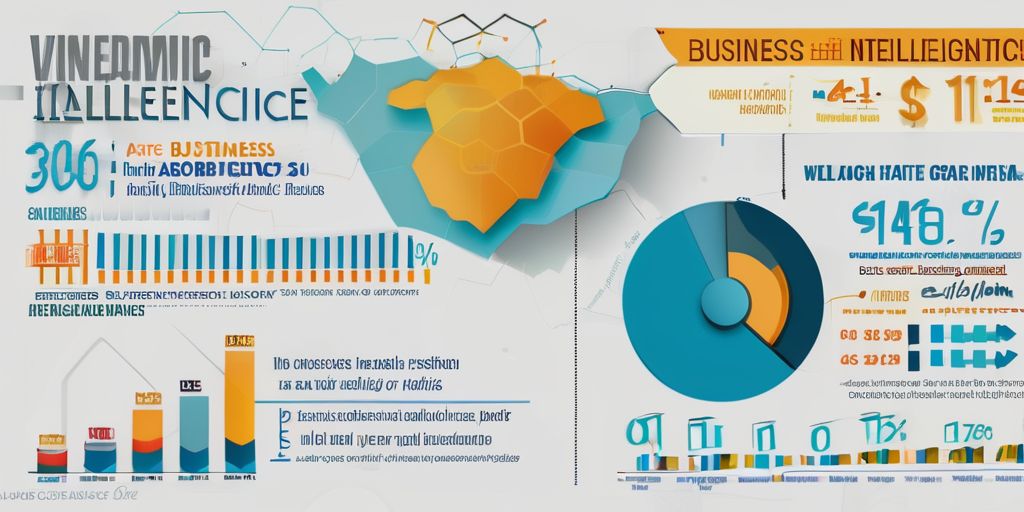
Enhancing Data Security and Transparency
In the realm of Business Intelligence (BI), data security and transparency are not just buzzwords; they are critical components that shape trust and compliance in the digital age. With the advent of stringent privacy laws like GDPR and CCPA, organizations are mandated to protect sensitive information and uphold the integrity of their data management practices.
To address these challenges, companies are adopting robust security measures and transparent processes. For instance, implementing a data certification program can significantly bolster data governance, ensuring that all stakeholders adhere to established data usage standards. Moreover, the use of data catalogs provides a clear outline of how data is to be handled, fostering an environment of accountability and control.
Tip: Always ensure that data is secured both at rest and in motion to maintain its integrity throughout its lifecycle.
As BI tools evolve, they now include features that identify and flag data anomalies, enhancing the veracity of big data analytics. However, it is imperative for businesses to not only rely on these tools but also to understand and maintain the integrity of their data pipelines. This involves selecting the right data sources, analysis methods, and defining user roles for each specific use case.
Blockchain for Supply Chain Analytics
Enhancing Data Security and Transparency
Blockchain for Supply Chain Analytics
Smart Contracts and Automated Compliance
Implement a table for presenting structured, quantitative data. Ensure it's succinct and formatted correctly in Markdown.
- Blockchain can enhance supply chains by enabling products to be delivered faster and more cost-effectively
- Improving the traceability of products
- Allowing for automated compliance and smart contracts
Blockchain can enhance supply chains by enabling products to be delivered faster and more cost-effectively, improving the traceability of products, allowing for automated compliance and smart contracts.
Smart Contracts and Automated Compliance
The advent of blockchain technology has paved the way for smart contracts, which are self-executing contracts with the terms directly written into code. These contracts automatically enforce and execute the terms of an agreement, reducing the need for intermediaries and minimizing the risk of disputes. For businesses, this means a more streamlined and efficient compliance process.
Automated compliance through smart contracts can significantly reduce the administrative burden associated with traditional contract management. Systems can now predict equipment failures and automatically reroute production processes to prevent disruptions, exemplifying the proactive capabilities of smart contracts in maintaining compliance.
While the potential for automation is vast, many organizations still incorporate a manual approval step for critical decisions. This hybrid approach ensures both the efficiency of automation and the oversight necessary for control.
Tip: When implementing smart contracts for automated compliance, it's crucial to maintain a balance between automation and human oversight to ensure that all regulatory and legal standards are met without compromising on decision quality.
Augmented Analytics and Natural Language Processing
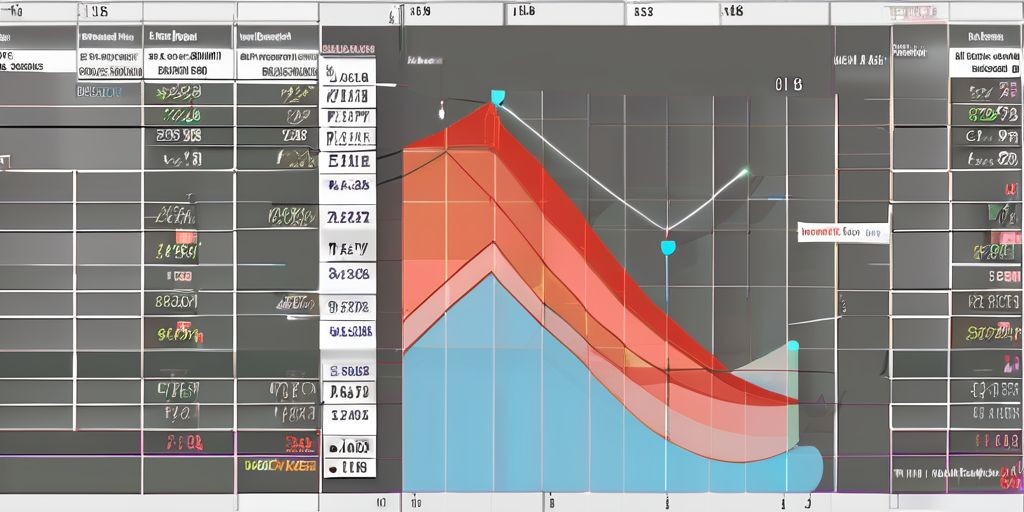
Conversational Analytics and BI Interfaces
The advent of conversational analytics has revolutionized the way businesses interact with their data. By leveraging natural language processing (NLP), users can now engage with BI tools through simple dialogue, making data analysis more intuitive and accessible. This shift towards conversational interfaces signifies a broader trend in making complex technology user-friendly.
Insight generation and decision-making have become more streamlined as a result. Users can ask questions in their own words and receive answers in the form of easy-to-understand visualizations and reports. For example, asking "What were our sales last quarter?" could instantly generate a sales report without the need for complex query languages.
Tip: Always phrase your questions clearly and specifically when using conversational analytics to ensure the most accurate and relevant results.
Generative AI and large language models (LLMs) further enhance this experience by automating data observability and providing proactive alerts. This not only improves the quality and efficiency of data operations but also supports a more dynamic and responsive BI strategy.
NLP for Data Querying and Interpretation
Natural Language Processing (NLP) capabilities play a crucial role in data querying and interpretation. These capabilities enable chatbots and voice and text analysis, as well as autonomous business process automation. Additionally, NLP supports personalization and recommendation features in websites and services. With the help of AI and machine learning, companies are leveraging NLP to identify data patterns, detect anomalies, and support predictive analytics. Here's a brief table showcasing the applications of NLP:
Application | Description |
---|---|
Chatbots | Conversational interfaces for customer interactions |
Voice and Text Analysis | Understanding and processing spoken and written language |
Autonomous Business Process Automation | Automation of routine business processes |
Personalization and Recommendation | Tailoring content and suggestions for users |
Furthermore, here's a valuable tip for leveraging NLP capabilities: Advanced forms of visualization and analytics tools enable users to ask questions in natural language, with the system automatically determining the right query and showing the results in a context-relevant manner.
The Future of Augmented Decision-Making
Real-time, automated decision-making is becoming the norm, with machine learning and artificial intelligence playing a crucial role. Machine learning (ML) and artificial intelligence (AI) are already revolutionizing industries such as healthcare and manufacturing. In healthcare, intelligent systems can detect and diagnose medical conditions, while in manufacturing, AI-driven systems can predict equipment failures and automatically reroute production processes to prevent disruptions. Beyond these examples, we see applications like email marketing software that can automatically determine the winning variant in an A/B test and apply it to other campaigns. The analysis of customer data is also becoming automated, enabling businesses to determine loan eligibility and make informed lending decisions. However, many organizations still retain a manual approval step for critical decisions to ensure oversight and control.
Ethical Considerations and Data Privacy
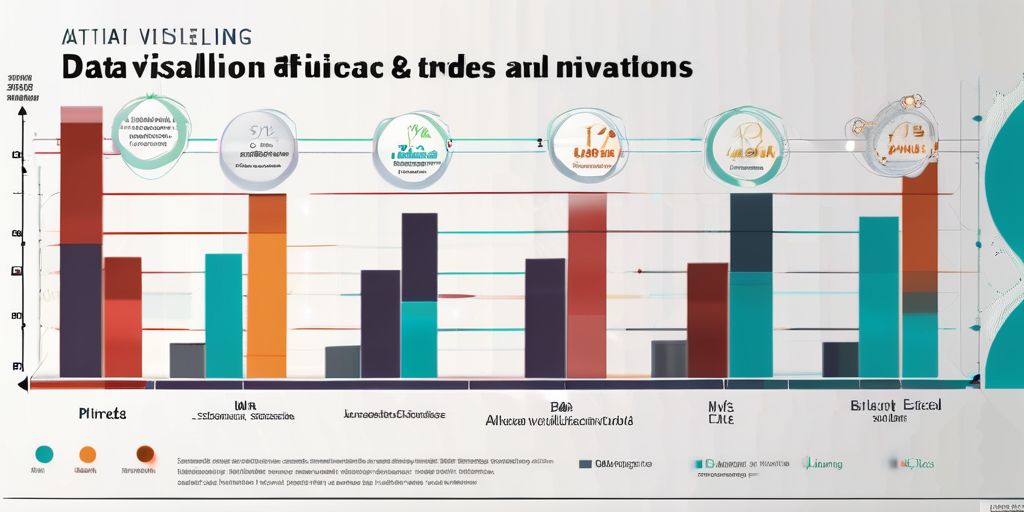
Navigating the Complexities of Data Governance
Understanding the right data sources, analysis methods, and user roles for each use case is essential for maintaining data health and reducing downtime. Data observability platforms, such as Monte Carlo, monitor data freshness, schema, volume, distribution, and lineage, helping organizations maintain high data quality and discoverability.
By establishing a central set of governance standards, organizations can maintain control over data usage while allowing multiple stakeholders access to data for their specific needs. Implementing a data certification program and using data catalogs to outline data usage standards can help ensure data compliance across all departments.
Cloud technology has revolutionized data storage and processing, enabling businesses to overcome physical storage limitations and acquire virtually infinite storage and processing capabilities. Cloud-based data processing also facilitates access to data for multiple stakeholders, but businesses must prioritize the integrity of their data pipelines.
Compliance with regulations like GDPR and CCPA is not only a legal requirement but also essential for protecting a company's reputation. Data breaches can have severe consequences, making data security a top priority.
Privacy Laws and Their Impact on BI
The impact of privacy laws on business intelligence cannot be overstated. As data collection and analysis become more sophisticated, the need for robust data governance and compliance with privacy laws becomes paramount. Organizations must navigate the complexities of data governance to ensure ethical data practices and build trust with their stakeholders. This involves understanding the legal requirements, implementing secure data handling processes, and fostering a culture of data privacy awareness.
Implementing a table for presenting structured, quantitative data is essential for demonstrating compliance with privacy laws and regulations. This table can outline the key provisions of privacy laws, the corresponding compliance measures, and the impact on business intelligence operations.
Organizations should also consider the following points:
- Conducting regular privacy impact assessments to identify and mitigate potential risks.
- Providing ongoing training to employees on data privacy best practices.
- Establishing clear procedures for handling data subject requests and consent management.
It is important to remember that compliance with privacy laws is not just a legal obligation, but also a strategic advantage. By prioritizing data privacy and ethical data practices, organizations can enhance their reputation, build customer trust, and mitigate the risks associated with data breaches and non-compliance.
Building Trust with Ethical Data Practices
Data stewardship and ethical data practices are becoming increasingly important in the business landscape. Organizations are now more focused on properly securing and managing data, especially in light of new regulations and widespread security breaches. Compliance with measures like the General Data Protection Regulation (GDPR) and California Consumer Privacy Act (CCPA) is vital to avoid fines and protect the company's reputation. Implementing a data certification program and using data catalogs to outline data usage standards can help ensure data compliance across all departments. By establishing a central set of governance standards, organizations can maintain control over data usage while allowing multiple stakeholders access to data for their specific needs. Proper data governance is crucial, especially with the ever-increasing volume of data. New tools are emerging to ensure that data stays where it needs to stay, is secured at rest and in motion, and is appropriately tracked over its lifecycle. These trends will continue to shape the big data landscape in 2024.
Collaborative Business Intelligence
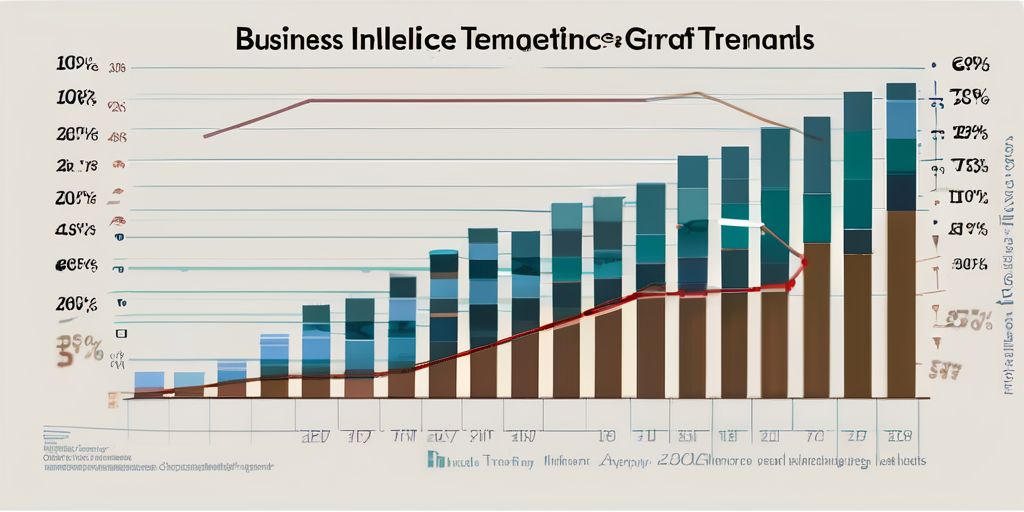
Fostering a Data-Driven Culture
In the era of digital transformation, the success of a business increasingly hinges on its ability to foster a data-driven culture. This requires not only access to data but also the development of organization-wide data literacy skills. By empowering cross-functional teams to independently manage and analyze their data, businesses can cultivate a sense of data ownership and collaboration. Data becomes a shared asset, contributing value across various business areas.
The democratization and decentralization of data have led to a surge in self-service analytics platforms. Tools like Tableau, Mode, and Looker facilitate visual exploration and enable stakeholders to engage with data directly. This shift is further supported by the rise of no-code and low-code solutions, which allow individuals to interact with data without extensive technical knowledge.
Tip: To maintain a unified approach to data, consider creating a data certification program. This ensures that all departments adhere to agreed-upon data standards.
Cloud technology has also played a pivotal role in this cultural shift. With platforms like Snowflake, Redshift, and BigQuery, businesses can leverage virtually infinite storage and processing capabilities, ensuring that data is accessible and actionable for decision-making across the organization.
Tools for Enhanced Collaboration and Sharing
In the realm of Collaborative Business Intelligence (CBI), the tools we choose can make or break the effectiveness of our data-driven initiatives. With the right set of tools, teams can seamlessly share insights, work on data models collaboratively, and make informed decisions together.
Microsoft SharePoint is a prime example of a platform that enhances collaboration. It offers both on-premises and cloud versions, allowing teams to manage content and create accessible documents for a wide range of users, including those using assistive technologies. SharePoint's core capabilities facilitate a shared environment where data and insights can be easily accessed and acted upon.
Another key player in this space is the suite of analytics tools such as Microsoft Power BI, Tableau, and Sisense. These tools not only provide advanced analytics and data visualization but also enable users to ask questions in natural language, making data more approachable and actionable for all team members.
Tip: When selecting collaboration tools, consider not only their individual features but also how they integrate with your existing systems and workflows to ensure a smooth and productive user experience.
The Importance of Cross-Departmental Data Access
Enabling multiple stakeholders to access data simultaneously without performance bottlenecks is crucial for data-driven decision-making. Robust security measures ensure up-to-the-minute data access from anywhere. Processing data variety is challenging due to diverse data formats from various sources. Tools like Fivetran simplify data integration with connectors to over 160 data sources. Snowflake's partnerships provide numerous benefits:
Advanced Analytics and Complex Event Processing
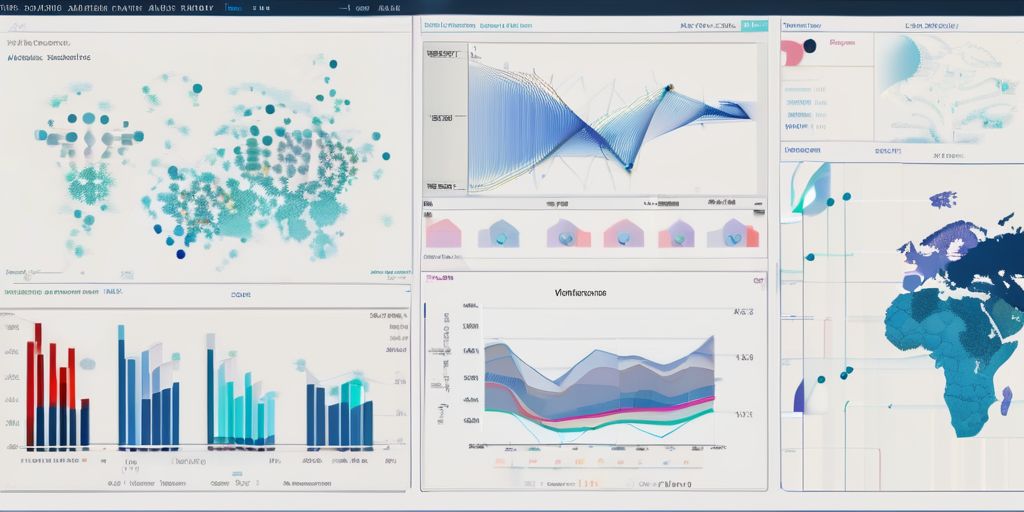
Real-Time Analytics for Dynamic Business Environments
In today's fast-paced business world, the ability to access and analyze data in real-time is a game-changer. Real-time analytics allow businesses to respond to market changes, customer behavior, and operational challenges as they happen. This immediacy transforms decision-making from a reactive to a proactive stance, significantly enhancing agility and competitiveness.
Real-time insights are not just about speed; they're about relevance. By leveraging streaming data, companies can monitor key performance indicators (KPIs) as events unfold, ensuring that strategies are informed by the most current information available. This shift from batch processing to streaming is crucial for maintaining data quality and freshness, which in turn drives better-informed decisions.
- Monitor KPIs continuously
- Adjust strategies based on current data
- Ensure data quality and freshness
Tip: To capitalize on real-time analytics, businesses should streamline their data infrastructure to support the rapid ingestion and processing of data streams.
The integration of machine learning and artificial intelligence further empowers real-time analytics. These technologies can analyze large volumes of historical and real-time data from disparate sources to find patterns, trends, and anomalies quickly and more efficiently. As a result, automated decision-making is becoming the norm, allowing companies to anticipate and address issues before they escalate.
Complex Event Processing in High-Velocity Data Streams
In the realm of business intelligence, the ability to process events in high-velocity data streams is becoming increasingly crucial. As organizations strive for real-time analytics, the focus shifts towards streaming data rather than batch processing. This shift ensures that decision-makers have access to the freshest insights, enhancing competitiveness and enabling more informed decisions.
Data observability plays a pivotal role in maintaining the integrity of this fast-moving data. With tools like Snowflake's Snowpipe streaming and Google's PubSub integration with BigQuery, organizations can now process and query data with significantly reduced latency. However, the challenge lies in ensuring that the rapid influx of data does not compromise quality.
Tip: Always balance the need for real-time data with robust data governance to prevent acting on inaccurate or incomplete information.
To address these challenges, businesses are turning to advanced solutions that offer both speed and reliability. For example, materialized tables serve as a midpoint between traditional databases and real-time streams, providing a snapshot of data that is readily available for querying without the wait associated with batch processing.
Predictive Models for Proactive Business Strategies
Predictive models play a crucial role in enabling businesses to make proactive decisions based on historical data and statistical algorithms. By identifying the likelihood of future outcomes, predictive analytics helps reduce risk, increase profitability, and drive better decision-making. Here's a brief overview of the key techniques used in predictive analytics:
- Predictive Analytics: This technique uses statistical algorithms and machine learning to identify the likelihood of future outcomes based on historical data. It enables businesses to make more informed decisions and reduce the risk of failure.
- Data-Driven Decision-Making: This process incorporates facts and information from multiple sources of data to make business plans and strategies. It empowers businesses to turn insights into actionable strategies and drive operational efficiency.
Predictive models are essential for uncovering hidden patterns, reducing resource wastage, and making effective forecasts. They enable businesses to harness the true potential of their data and transform decision-making processes.
Mansoor Mohammed, a dynamic Enterprise Agile Coach, emphasizes the importance of predictive analytics in improving operational efficiency and creating personalized consumer services. Cloud-based analytics and priority analysis further enhance the ability to predict future outcomes and understand customer needs.
Advanced analytics and complex event processing are essential components of modern database optimization. At OptimizDBA Database Optimization Consulting, we specialize in leveraging advanced analytics and complex event processing to deliver unparalleled performance improvements. Our industry-leading remote DBA services have been trusted since 2001, serving over 500 clients with a proven track record of significantly increasing transaction speeds. Experience the power of optimized database performance with OptimizDBA today!
The Future of Business Intelligence: Trends and Innovations
Business Intelligence technologies are challenging the status quo of decision-making with their cutting-edge predictive and proactive approach. From enhancing operational efficiency to predicting future trends, BI empowers businesses to harness the true potential of their data. As the landscape evolves, staying abreast of Business Intelligence trends and continuously optimizing BI strategies will be key to maintaining
Frequently Asked Questions
What are the key trends in business intelligence for the future?
The key trends in business intelligence for the future include the evolution of data analytics, artificial intelligence and machine learning, the integration of BI and IoT, cloud computing, data visualization, blockchain, augmented analytics, ethical considerations, collaborative BI, and advanced analytics.
How is business intelligence evolving in the era of big data?
Business intelligence is evolving in the era of big data by shifting from descriptive to prescriptive analytics, leveraging self-service analytics platforms, and making data-driven decisions based on the impact of big data.
What role does artificial intelligence play in business intelligence?
Artificial intelligence plays a crucial role in business intelligence by enabling predictive analytics, customer insights, forecasting models, fraud detection, and proactive decision-making.
How does IoT integration enhance business intelligence?
IoT integration enhances business intelligence by enabling real-time data analysis from IoT devices, improving operational efficiency, enabling predictive maintenance, and providing insights for proactive decision-making.
What are the benefits of cloud computing for business intelligence?
The benefits of cloud computing for business intelligence include the shift to cloud-based BI solutions, scalable infrastructure, and considerations for security in cloud BI.
What advancements are being made in data visualization and interactive reporting?
Advancements in data presentation, interactive dashboards, real-time reporting, and the role of storytelling are key areas of focus in data visualization and interactive reporting.
How does blockchain contribute to business intelligence?
Blockchain contributes to business intelligence by enhancing data security, transparency, supply chain analytics, and enabling smart contracts for automated compliance.
What is the future of augmented analytics and natural language processing in BI?
The future of augmented analytics and natural language processing in BI includes conversational analytics, NLP for data querying and interpretation, and the evolution of augmented decision-making.