The Impact of Data-Driven Decision-Making
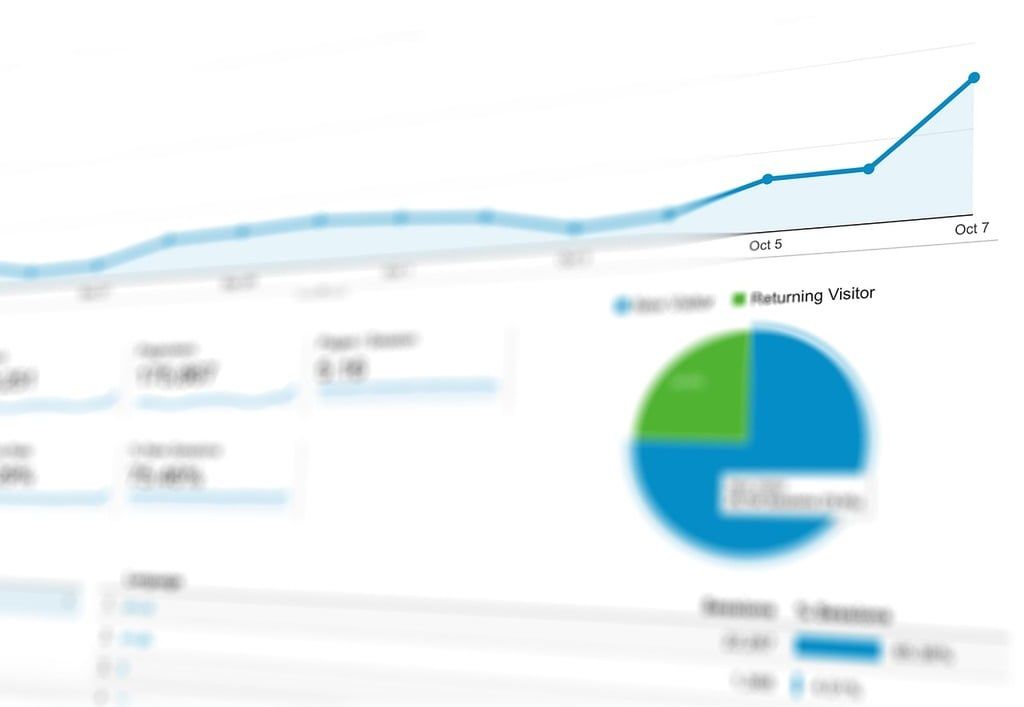
Benefits of data-driven decision-making
The adoption of data-driven decision-making marks a significant shift from the era of intuition-led strategies to one where decisions are anchored in empirical evidence. This approach leverages insights extracted from multiple data sources, aligning business plans and strategies with organizational goals. Here are some of the key benefits:
- Informed Decision-Making: Data replaces guesswork with hard facts, providing a clear understanding of customer needs and market trends.
- Resource Optimization: By identifying where to allocate resources effectively, businesses can reduce unnecessary spending.
- Empowered Employees: Access to data fosters a culture of autonomy and confidence, making businesses more agile and proactive.
- Risk Management: Early identification of potential risks and challenges is possible, allowing for proactive measures.
- Operational Efficiency: Analysis of operational data reveals inefficiencies, paving the way for improvements.
Data-driven insights not only illuminate the present but also forecast future trends, giving businesses a competitive edge. The ability to personalize customer experiences and measure performance over time equates to having a 'fitness tracker' for the business, ensuring continuous improvement and growth.
The shift towards data-driven decision-making is not just a technological upgrade but a cultural transformation that empowers businesses to act with precision and foresight.
Challenges in implementing data-driven decision-making
While the shift towards data-driven decision-making offers numerous advantages, organizations often encounter significant hurdles. Data quality is paramount; without clean, accurate, and relevant data, the foundation of decision-making is compromised. To address this, businesses must invest in robust data cleansing processes and validation techniques.
Integration of disparate data sources presents another challenge. Organizations must leverage sophisticated tools capable of harmonizing diverse data formats and structures. This is critical for a holistic view of business operations.
The skill gap is a notable barrier, with a pressing need for data scientists and analysts adept in predictive analytics. Overcoming this requires targeted training and strategic hiring.
Change management is also a crucial aspect, as resistance to new systems can stifle progress. Clear communication and demonstrating the value of analytics are key to fostering acceptance. Here's a concise list of common challenges and potential solutions:
- Data Quality: Invest in data cleansing and validation to ensure accuracy.
- Data Integration: Use integration tools to combine data from various sources.
- Skill Gap: Provide training or hire qualified personnel.
- Change Management: Implement strategies to manage resistance to change.
Future trends in data-driven decision-making
As the business landscape evolves, future trends in data-driven decision-making are becoming increasingly clear. The integration of generative AI is poised to significantly democratize data, enabling a broader range of users to engage with analytics tools. Despite this, less than half of company executives currently possess the skills to utilize data software independently, highlighting the need for upskilling and a supportive culture.
- Generative AI and data democratization
- Upskilling the workforce
- Developing a supportive culture
The Internet of Things (IoT) will also play a pivotal role, with security and cost management being key areas for improvement. Decision intelligence is set to bridge the gap in decision-making processes by merging social sciences with advanced AI and machine learning techniques.
Businesses can harness the power of predictive analytics by addressing challenges, prioritizing ethical considerations, and measuring success using KPIs and continuous improvement.
Predictive Analytics: Shaping the Future of Business
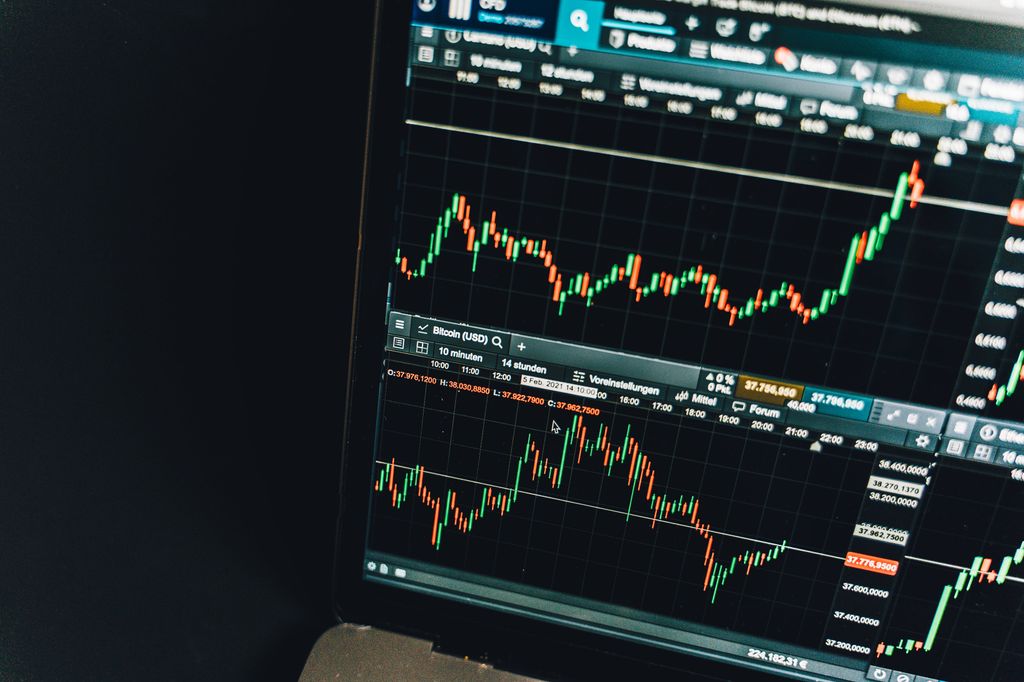
Understanding predictive analytics
Predictive analytics stands at the forefront of modern business strategy, enabling organizations to forecast future trends and customer behaviors with remarkable accuracy. By harnessing the power of statistical algorithms, machine learning techniques, and historical data, this approach goes beyond mere analysis of past events; it provides a probabilistic window into what lies ahead.
The process begins with Data Collection, where diverse data sources are tapped to gather the necessary information. This is followed by Data Analysis, where the collected data is scrutinized to identify patterns and relationships. The final step, Outcome Prediction, involves applying predictive models to make informed forecasts about future events or trends.
Predictive analytics is not a crystal ball, but it offers a significant edge in decision-making by reducing uncertainty and guiding strategic planning.
While predictive analytics can be a game-changer, it's important to remember that it's based on probabilities and not certainties. The margin of error, although present, is often outweighed by the benefits of being able to anticipate and prepare for future scenarios.
Applications of predictive analytics in business
Predictive analytics harnesses data, statistical algorithms, and machine learning to forecast future business scenarios. It's akin to a meteorological service for your company, predicting both prosperous times and potential challenges. Businesses leverage predictive analytics to anticipate customer needs, manage risks, and craft marketing strategies that resonate more effectively with their target audience.
Predictive analytics is not infallible, operating on probabilities and carrying a margin of error. However, it remains a valuable tool for informed decision-making. Here are some key applications in various business domains:
- Sales and Marketing: Tailoring campaigns to customer preferences and predicting future buying patterns.
- Inventory Management: Forecasting product demand to optimize stock levels, reducing both overstock and stockouts.
- Risk Management: Identifying potential risks and mitigating them before they impact the business.
- Operational Efficiency: Streamlining processes and predicting maintenance needs to minimize downtime.
Predictive analytics is transforming the landscape of decision-making, offering a glimpse into the future that enables businesses to act proactively rather than reactively.
Despite its benefits, integrating predictive analytics into existing business systems poses challenges, such as ensuring seamless operation and interpreting the data accurately. Nevertheless, the foresight it provides is invaluable for businesses of all sizes, from startups to large corporations, in navigating the complexities of the market.
Challenges and opportunities in predictive analytics
While predictive analytics offers a transformative potential for businesses, it comes with its own set of challenges. Identifying the right data sources is a fundamental hurdle, as the quality and relevance of data directly impact the accuracy of predictions. Moreover, building a team with the necessary skills to interpret and apply analytical techniques is essential for leveraging predictive analytics effectively.
The integration of predictive analytics into business processes requires a strategic approach, encompassing clear objectives, data preparation, and continuous monitoring to ensure success.
The opportunities, however, are vast. Companies that successfully navigate these challenges can harness predictive analytics for sales forecasting, customer segmentation, and inventory optimization, among other applications. To illustrate the process and common obstacles, here's a step-by-step guide:
- Define objectives and identify use cases.
- Gather and prepare data from relevant data sources.
- Develop predictive models.
- Deploy models into business operations.
- Monitor and refine models continuously.
Embracing these steps can lead to more informed decision-making and a significant competitive advantage in the data-driven world.
Digital Transformation in Business Analytics
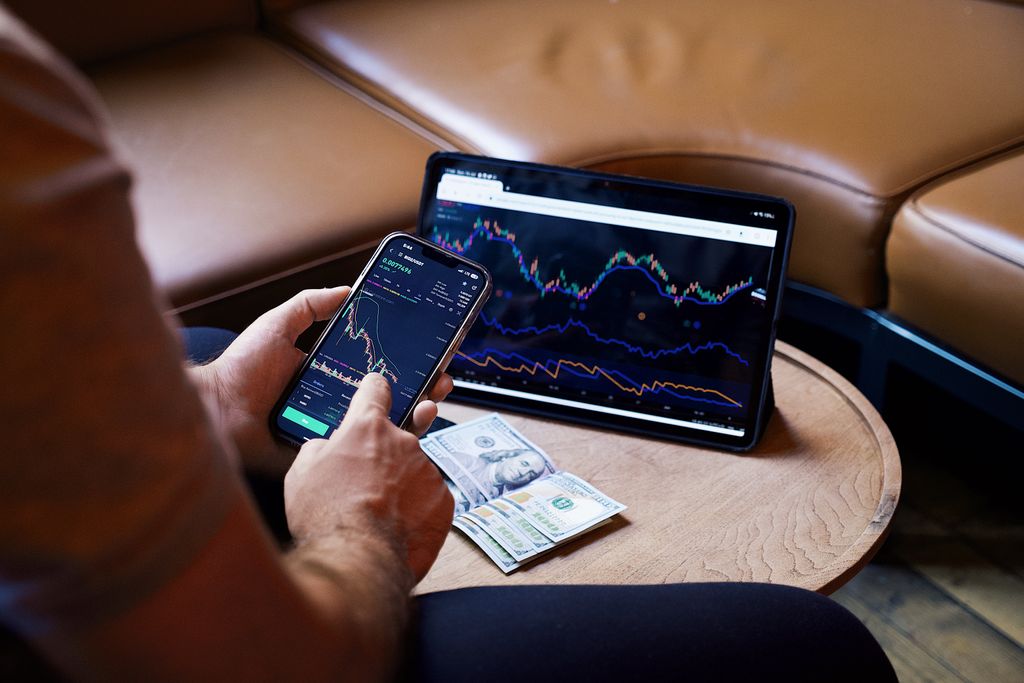
Adopting a data-driven decision-making system
In the era of digital transformation, adopting a data-driven decision-making system is crucial for businesses aiming to stay competitive. This approach leverages analytics to inform strategies and operations, aligning them with organizational goals. The process begins with defining the decision pathways unique to each organization, ensuring that the system can generate accurate and relevant insights.
- Define decision pathways specific to your organization.
- Link your datasets to the decision-making system.
- Utilize AI and machine learning to enhance accuracy.
By integrating decision intelligence, which combines decision science with data science, businesses can close the gap between insights and action, mitigating delays in the decision cycle.
The journey towards a data-driven culture doesn't end with implementation. It's a continuous cycle of improvement, requiring feedback and iteration to refine the system and adapt to evolving business landscapes.
The role of digital transformation in business analytics
Digital transformation is fundamentally altering the landscape of business analytics. It is not just about automating existing processes but about creating new, innovative business models that leverage technological advancements. Business analytics plays a pivotal role in this transformation, providing insights that drive these new models and optimize customer experiences.
Digital tools and techniques are now integral to business analysis, necessitating an expanded skill set for analysts. They must embrace agile methodologies and adapt to technologies like AI, blockchain, and IoT. This evolution is crucial for analyzing and interpreting data in our dynamic business environment.
The integration of digital tools into business analytics facilitates enhanced analysis and proactive decision-making, fostering a customer-centric approach.
However, the digital transformation journey is not without its challenges. Analysts face the need for continuous learning and must keep pace with rapid technological advancements to effectively utilize and interpret data. The table below summarizes the key aspects of digital transformation in business analytics:
Aspect | Impact on Business Analytics |
---|---|
Technological Advancements | Enables more sophisticated data analysis |
New Business Models | Drives innovation and optimization |
Skill Set Evolution | Requires continuous learning and adaptation |
Customer Experience | Becomes a central focus for analytics |
As AI is transforming software design and creation, it also becomes a game-changer for business analytics, offering potential benefits and shaping the nature of data interpretation and decision-making strategies.
Benefits and challenges of digital transformation
Digital transformation is a multifaceted process that reshapes the way businesses operate and interact with their customers. It leverages technological advancements to innovate business processes, products, and services, ultimately aiming to capture new market opportunities and maximize profits. The importance of business analytics is paramount in this transformation, as it provides the insights necessary to understand customer needs and identify emerging trends.
The adoption of digital tools and techniques is essential for business analysts to stay ahead in a rapidly evolving business landscape. This shift necessitates an expanded skill set and the embrace of agile methodologies. The integration of technologies such as AI, blockchain, and IoT into business analysis practices allows for more dynamic and complex data interpretation, enhancing the decision-making process.
Digital transformation, while offering numerous benefits, also presents significant challenges. Organizations must navigate the complexities of integrating new technologies, ensuring data privacy, and maintaining security. Moreover, the use of AI in software development raises ethical concerns, necessitating the adoption of ethical AI practices to mitigate risks and build public trust.
Despite these challenges, the future of digital transformation looks promising, with the potential to optimize operations and management across various industries. As businesses continue to adopt digital transformation strategies, they will need to balance the pursuit of innovation with the responsibility of ensuring ethical and secure practices.
Data Privacy and Security in Business Analytics
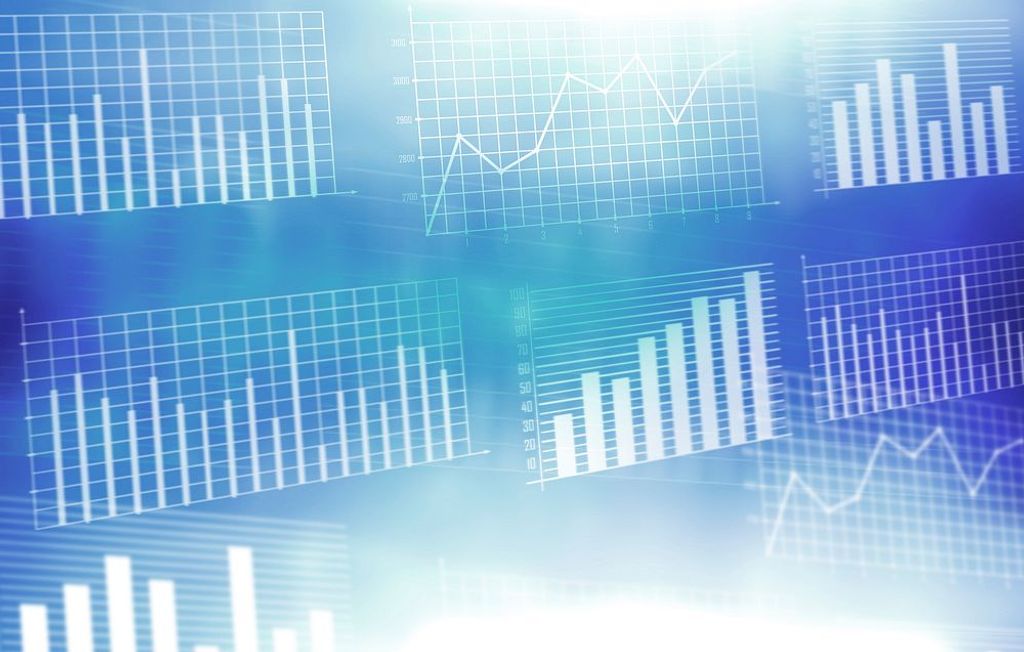
Trends in data privacy and security
In the realm of business analytics, data privacy and security have become paramount. As organizations navigate the complexities of regulations like GDPR and CCPA, they are compelled to implement robust measures to safeguard sensitive information. The focus is not only on preventing unauthorized access but also on ensuring that data handling practices are compliant with legal requirements.
- Implement robust data privacy and security measures.
- Comply with data protection regulations.
- Regularly update security protocols.
The landscape of data privacy is evolving, with an engineering-first approach becoming necessary to tailor security features to an organization's specific needs. As the volume of data grows, so does the sophistication of potential threats, making continuous assessment and improvement of security systems crucial.
Advancements in BI, including predictive analytics and cloud-based solutions, are impacting various sectors by offering tailored applications. This progress, however, brings forth new challenges in ensuring that the data remains secure amidst the technological evolution.
The impact of citizen data scientists
The rise of citizen data scientists is reshaping the landscape of business analytics, democratizing data and empowering a broader range of employees to engage in data-driven decision-making. Citizen developers are now becoming the new data analysts, leveraging automation to build, run workflows, and package them into reusable applications. This shift is not only about increasing accessibility but also about fostering a culture of continuous improvement and iterative development within organizations.
Despite concerns about redundancy, experts like Shaku Atre predict an increase in the demand for data scientists, albeit with a broader spectrum of specialized skills. The integration of generative AI and the focus on decision intelligence highlight the evolving nature of analytics roles, where technical expertise must be complemented by an understanding of social sciences and AI-ML technologies.
Continuous improvement through data-driven metrics is essential for business transformation. A cultural shift towards iterative improvement is crucial for success.
As we look to the future, the following trends are particularly noteworthy:
- Enhanced accessibility and democratization of data
- Emphasis on data quality and governance
- The growing importance of IoT data security
- The need for AI-related guardrails in light of generative AI integrations
Challenges and opportunities in data security
In the realm of business analytics, data security remains a paramount concern. Organizations must navigate a complex landscape of regulatory requirements and evolving threats. For instance, the General Data Protection Regulation (GDPR) mandates stringent measures to protect personal data from breaches and unauthorized access. Yet, these same organizations are expected to provide data on demand to users and submit to security audits.
The rise of big data has introduced new challenges in data governance. Poor management and oversight can lead to unregulated data handling practices, which in turn heightens the risk of security incidents. As highlighted by Keenethics, another issue for secure Big Data is data governance, emphasizing the need for robust management systems.
The intersection of AI and data security presents both challenges and opportunities. AI algorithms, while powerful in detecting potential threats, can also increase the risk of data leaks if not properly managed.
The following table outlines the cost impact of data breaches, as reported by IBM:
Year | Percentage of Cloud Data Breaches | Average Cost of Data Breach (USD) |
---|---|---|
2022-23 | 82% | 4.75 Million |
Despite the daunting challenges, there are opportunities for innovation in data security. The demand for encryption and anonymization software is growing, as is the need for consistent security assessments. Moving forward, businesses must adopt an engineering-first approach to customize security features, ensuring that data is protected at every point in its lifecycle.
The Future of Business Intelligence and Analytics
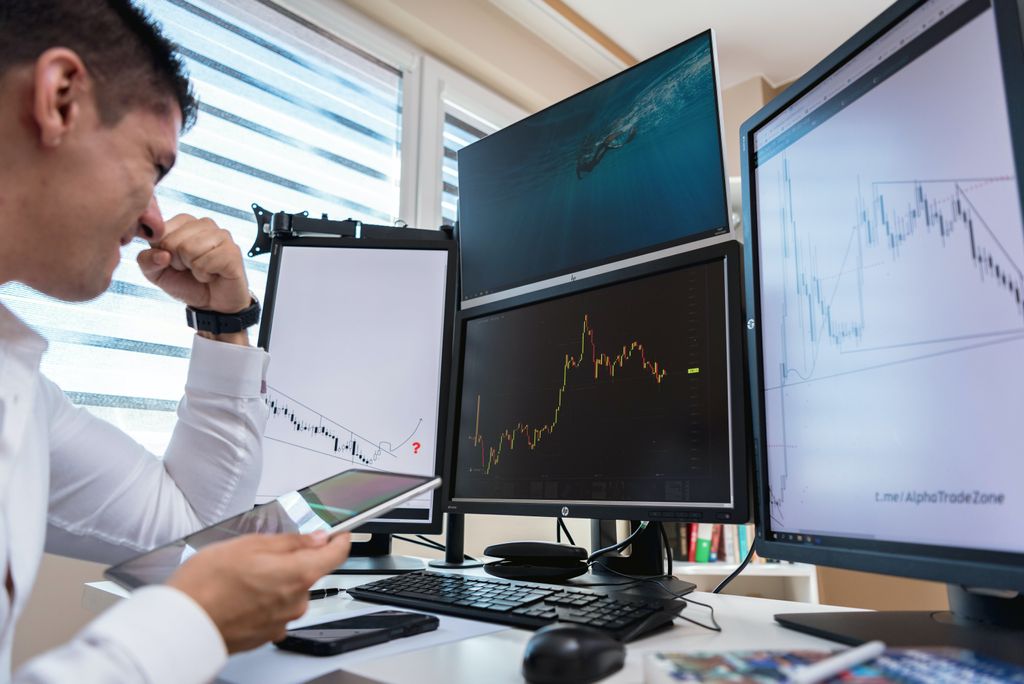
Emerging trends in business intelligence
The landscape of business intelligence is continuously evolving, with new trends emerging that promise to reshape the way organizations leverage data. Generative AI is one such trend, poised to significantly impact data democratization, enabling users across various levels of expertise to generate and interpret complex data sets. This trend underscores the importance of establishing strict usage guidelines to ensure ethical and responsible AI practices.
Another key trend is the rise of cybersecurity mesh architecture, which enhances the security of SaaS BI solutions. This approach allows for a more flexible and modular security infrastructure, addressing the increasing concerns around data privacy and security.
The integration of technology into business operations is not just a trend but a necessity. As businesses strive to stay ahead, they are adopting predictive analytics tools and other advanced technologies to make informed decisions. The following list highlights some of the major trends:
- Predictive Analytics Tools
- Data Sharing and Monetization
- Data Mesh and Governance
- Automation and IoT
- Decision Intelligence
As we embrace these trends, it's crucial to balance innovation with responsible and ethical data practices. The future of business intelligence will demand a harmonious blend of technological advancement and data stewardship.
The role of generative AI in data democratization
Generative AI is revolutionizing the way businesses approach data democratization. With less than half of company executives able to use data software independently, the complexity of data analytics has been a significant barrier. Generative AI, particularly through the use of large-language models (LLMs), is bridging this gap, enabling a more intuitive and accessible way for employees to engage with data.
Generative AI can connect employees with knowledge in a conversational style that builds new levels of comprehension and skill.
This transformative technology is not only enhancing personal productivity but also fostering a data-oriented culture within organizations. As a result, there is a notable surge in investment and interest in generative AI, with a McKinsey Global Survey revealing that 40% of respondents plan to increase their AI investment.
The impact of generative AI extends beyond technology adoption, influencing the very culture of organizations. It promotes a data and analytics mindset, crucial for the establishment of a data-driven culture. The table below highlights the cultural impact of generative AI based on recent surveys:
Year | Cultural Impact of Generative AI |
---|---|
2023 | Significant shift in organizational culture |
2024 | Emphasis on data-centric AI, focusing on quality and governance |
By improving data quality and governance, companies are poised to reduce biases and enhance the effectiveness of their AI initiatives. Gartner forecasts that by 2024, a significant portion of AI data will simulate reality to identify future scenarios, marking a shift towards a more reliable and sustainable AI utilization.
Cloud computing and its impact on business analytics
The advent of cloud computing has revolutionized the way businesses approach data analytics. By leveraging cloud-based platforms and services, organizations gain scalable computing power and storage capabilities, essential for handling the ever-increasing volumes of data. This shift has enabled businesses to access advanced analytics tools and embrace new methodologies, positioning them for a competitive edge in a data-driven landscape.
Cloud infrastructure not only facilitates efficient data processing but also paves the way for integrating sophisticated technologies like AI. The impact of AI on RDBMS integration and real-time analytics is profound, leading to scalable, intelligent, and integrated data processing systems. As cloud computing evolves, it promises to further enhance these capabilities, offering businesses unprecedented analytical power.
The future of business analytics is inextricably linked to cloud computing. With strict usage guidelines and a focus on cost-effective querying techniques, cloud computing is driving the development of new data management solutions like the data mesh, while ensuring data governance remains a priority.
The table below outlines the anticipated trends in cloud computing and their implications for business analytics:
Trend | Implication for Analytics |
---|---|
AI Integration | Enhanced real-time and predictive analytics |
Data Mesh | Improved data management at scale |
Cost Management | Drive for more efficient querying techniques |
Data Governance | Continued emphasis on privacy and security |
Conclusion
In conclusion, the future of decision-making is being transformed by the rapid advancements in business analytics and data science. As businesses integrate technology with everyday processes and operations, the need for data-driven decision-making becomes increasingly essential. Predictive analytics, digital transformation, and data-driven decision-making systems are among the emerging trends shaping the business landscape. These trends highlight the undeniable impact of data-driven decision-making in today's competitive business environment. It is clear that the world of AI and data analytics is in a state of dynamic change, demanding a balanced approach where innovation is coupled with responsible and ethical data practices. The future of business analytics holds great potential for businesses to make informed decisions, stay competitive, and drive innovation.
Frequently Asked Questions
What are the benefits of data-driven decision-making?
The benefits of data-driven decision-making include improved accuracy, faster decision-making, better insights, and increased efficiency in operations.
What are the challenges in implementing data-driven decision-making?
Challenges in implementing data-driven decision-making may include data quality issues, resistance to change, lack of skilled personnel, and the need for cultural transformation within the organization.
What are the future trends in data-driven decision-making?
Future trends in data-driven decision-making may involve the integration of AI and machine learning, enhanced predictive analytics capabilities, and the development of more user-friendly data visualization tools.
What is predictive analytics and how is it used in business?
Predictive analytics is the use of historical data and statistical models to forecast future trends and outcomes. In business, it is used to make informed decisions, identify potential risks, and optimize strategies based on predictive insights.
What are the applications of predictive analytics in business?
Predictive analytics is applied in business for customer segmentation, demand forecasting, risk management, fraud detection, and personalized marketing strategies.
What are the challenges and opportunities in predictive analytics?
Challenges in predictive analytics may include data privacy concerns, model accuracy, and interpretability. Opportunities include improved customer experiences, enhanced risk management, and competitive advantage through data-driven insights.
How does digital transformation impact business analytics?
Digital transformation impacts business analytics by enabling new and innovative processes, improving data quality, and aligning decision-making with organizational objectives through a data-driven approach.
What are the benefits and challenges of digital transformation in business analytics?
The benefits of digital transformation in business analytics include improved decision-making, enhanced operational efficiency, and better alignment with organizational goals. Challenges may include resistance to change, data security concerns, and the need for upskilling the workforce.