Strategic Alignment for AI-Driven Transformation
Bridging Business Goals with AI Capabilities
The integration of Artificial Intelligence (AI) into enterprise software is not just a technological upgrade; it's a strategic imperative that must be aligned with the core business goals. Ensuring that AI initiatives are closely tied to enhancing customer experiences, streamlining operations, or unlocking new revenue streams is crucial for achieving a high return on investment (ROI). This alignment guarantees that AI projects contribute to broader business objectives and do not operate in isolation.
Strategic business alignment is essential when investing in AI-powered software. It involves a collaborative effort across various departments, including product management, marketing, sales, and customer service. These stakeholders must work together to define clear objectives that AI can achieve, ensuring that the technology is not only technically viable but also commercially strategic.
- Outline your goals, strengths, weaknesses, and a three to six-month action plan.
- Clearly define how success will be measured.
- Present the AI strategy to stakeholders, ensuring it aligns with business objectives.
By integrating AI-driven data analysis tools into their operations, businesses can leverage the full spectrum of AI capabilities to propel themselves towards a data-informed future.
The Role of Leadership in AI Adoption
Leadership is pivotal in navigating the complex terrain of AI adoption. Setting a clear vision and strategy for AI within an organization is a fundamental responsibility of leaders. They must understand how AI aligns with the company's broader goals and ensure that ethical considerations are woven into the fabric of AI initiatives.
Ethical and privacy concerns are at the forefront of leadership challenges. The potential for misuse of AI technologies, such as privacy violations and biased decision-making, necessitates a responsible approach to AI adoption. Leaders must establish principles and frameworks that guide the deployment of AI, emphasizing aspects like fairness and safety.
The shift toward AI-centric operations requires new skills and roles, presenting leaders with the challenge of managing workforce impact and bridging skill gaps.
To ensure a responsible transition to AI-powered systems, leaders should prioritize seamless integration with existing systems and establish robust governance and oversight. This includes creating a governance framework to monitor AI performance and its impact on operations and workforce dynamics.
Aligning AI Initiatives with Market Demands
In the rapidly evolving landscape of enterprise software, aligning AI initiatives with market demands is paramount. The key is ensuring that AI initiatives are closely tied to core business goals, such as enhancing customer experiences, streamlining operations, or unlocking new revenue streams. This cross-functional alignment ensures that AI projects are technically viable and commercially strategic, maximizing ROI and serving broader business objectives rather than existing in a silo.
Strategic business alignment is crucial when investing in AI-powered software, extending far beyond the engineering team's purview. It requires a synergistic effort, where stakeholders across various departments collaborate to define clear objectives that AI can achieve.
To effectively align AI with market demands, consider the following steps:
- Evaluate potential AI use cases and prioritize initiatives that have the greatest potential to deliver value.
- Ensure privacy and security measures are integral to your AI initiatives.
- Invest in Talent and Training to address skill gaps and consider hiring AI specialists.
- Adopt a phased approach to AI integration, ensuring compatibility with existing systems and a smooth transition.
By focusing on these areas, businesses can ensure that their AI initiatives are not only technologically advanced but also deeply integrated with their market strategy and customer needs.
Technical Readiness for AI Integration
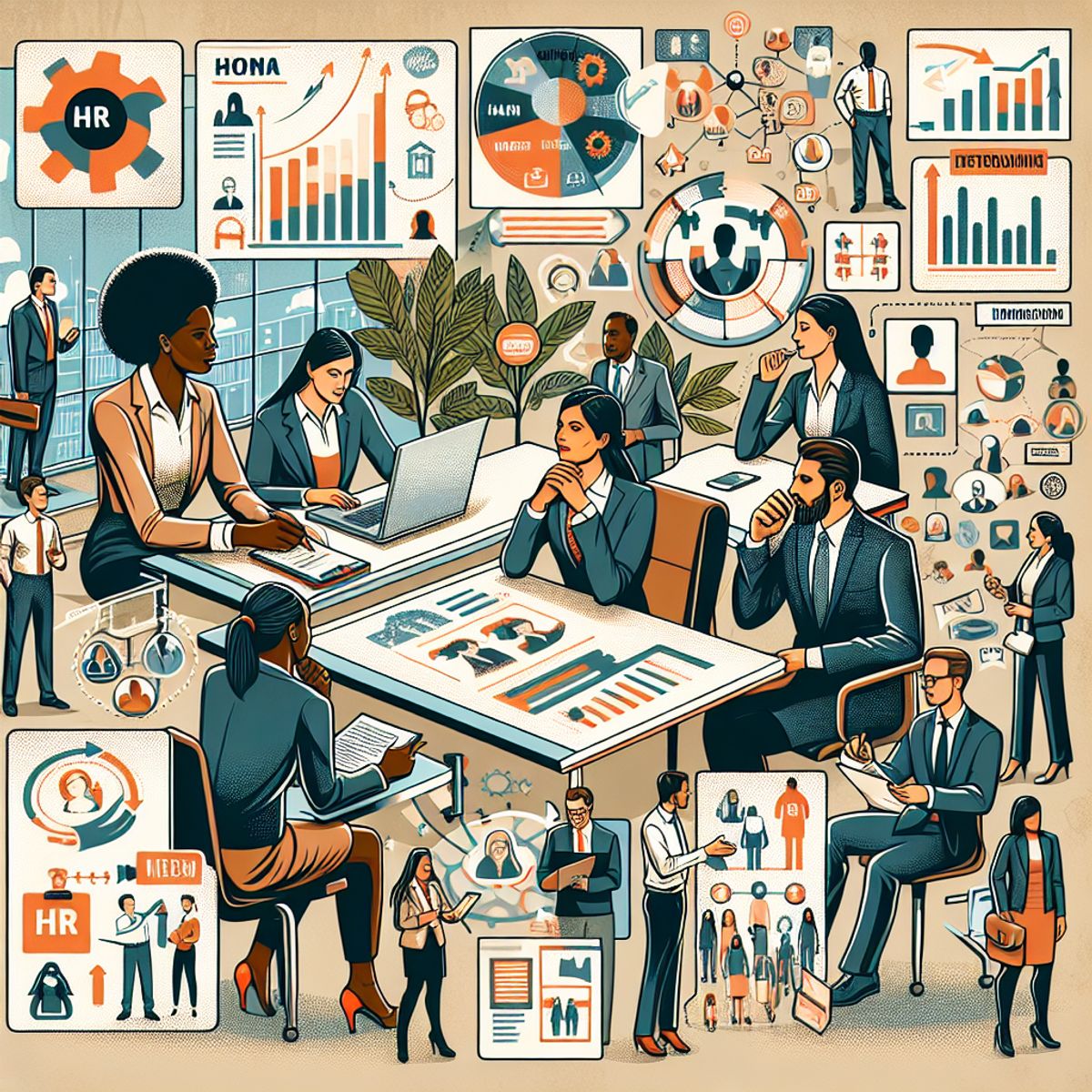
Evaluating Infrastructure for AI Deployment
The foundation of any AI initiative is the infrastructure that supports it. Evaluating the technical readiness of your systems is crucial for a successful AI deployment. This involves assessing the scalability of your current setup, the compatibility with AI technologies, and the ability to handle the increased data workloads that come with AI applications.
- Scalability: Can your infrastructure grow with your AI needs?
- Compatibility: Are your systems ready to integrate with AI technologies?
- Data Handling: Can your infrastructure manage the surge in data?
Ensuring that your infrastructure is AI-ready is not just about having the right hardware and software; it's about creating an environment where AI can thrive and deliver value.
It's also important to consider the human aspect of AI deployment. A robust framework that includes strategic alignment and quality control is essential for AI to transform enterprise strategies. This system acts as a quality gatekeeper, ensuring that AI-enabled applications meet stringent standards before making it to production.
Upskilling Teams for AI-Driven Development
In the era of AI transformation, upskilling teams is a critical step towards harnessing the full potential of AI in enterprise software development. A robust AI training program is essential, not only for the initial adoption but also for the ongoing evolution of AI tools within the organization.
Comprehensive training programs tailored to different roles ensure that all team members are proficient in the technical aspects of AI and can apply these tools effectively in their daily tasks. Moreover, fostering an AI-ready culture that values continuous learning and adaptability is vital for the long-term integration of AI methodologies.
By providing ongoing learning opportunities, such as workshops and webinars, teams remain abreast of the latest AI features and best practices, ensuring that the organization's AI initiatives are both innovative and ethically sound.
To truly embed AI into the fabric of enterprise software development, it is important to:
- Cultivate a culture of continuous learning and adaptability.
- Offer comprehensive and role-specific training programs.
- Provide ongoing learning opportunities to keep pace with AI advancements.
- Implement ethical AI guidelines to ensure transparency and fairness.
- Celebrate and incentivize small wins to encourage AI tool adoption.
Ensuring Data Quality and Accessibility
In the realm of AI, the adage 'garbage in, garbage out' holds significant weight. Ensuring data quality is not just about maintaining the integrity of datasets; it's about laying the foundation for AI systems that can be trusted to make sound decisions. Regularly cleaning and validating data sets is essential for reliable analysis, and this process should be ingrained in the enterprise's operational routine.
Data accessibility is equally critical. It's about making data available to the right people at the right time, while also safeguarding sensitive information. Here are key steps to achieve this balance:
- Implementing tracing data lineage to understand the data's journey and transformations.
- Guaranteeing accuracy and enriching metadata for better context and usability.
- Cataloging data securely to ensure it's both protected and retrievable.
By initiating data governance at the source, enterprises can not only reduce costs but also gain a superior understanding of the data's origin, value, and meaning.
Furthermore, compliance with data protection regulations such as GDPR or CCPA is non-negotiable. Secure data practices, including encryption and secure access controls, are imperative to protect against unauthorized access or breaches. Regular security audits of AI tools are necessary to detect potential vulnerabilities and safeguard data privacy.
The AI Software Delivery Challenge
Overcoming the Complexities of AI Implementation
The journey to integrate AI into enterprise software is fraught with complexities that can seem daunting at first glance. AI-powered software delivery solutions present challenges and opportunities. AI transforms logistics, enhances enterprise operations, and revolutionizes industries like transportation with its transformative power. To navigate these challenges, a strategic approach is essential.
Adoption complexity varies across different sectors and organizations. However, the key to a successful AI implementation lies in a step-by-step process that includes:
- Identifying user-friendly tools for non-technical users
- Designating leaders and projects for AI integration
- Offering robust AI training programs
- Creating incremental processes and documenting their effectiveness
By anticipating potential errors and establishing preventive measures, organizations can ensure a smoother transition to AI-powered systems.
It's crucial to recognize that while AI is powerful, it requires human input and quality control. This synergy of human creativity with AI capabilities can lead to a more effective and efficient enterprise environment.
Continuous Integration and Delivery (CI/CD) in AI
In the realm of AI-powered software development, CI/CD stands as a cornerstone, ensuring a streamlined and efficient delivery process. By automating the stages of development, from building to deployment, CI/CD pipelines significantly reduce manual intervention and the potential for errors, leading to a more reliable and consistent output.
Automation in CI/CD not only accelerates the development cycle but also enhances collaboration among teams. This is particularly crucial in AI projects where the integration of data science and software engineering practices is key. The following list outlines the core benefits of CI/CD in AI:
- Accelerated development timelines through automation
- Improved software quality with continuous testing and deployment
- Enhanced team collaboration and integration of cross-disciplinary expertise
- Greater reproducibility and reliability of AI applications
By embracing CI/CD, engineering leaders can transform potential into performance, turning technological challenges into opportunities for innovation and competitive advantage.
As AI continues to reshape the technology landscape, the readiness to evolve CI/CD practices will be a defining factor for companies looking to maintain a competitive edge. It's not just about adopting new tools, but also about fostering a culture that is agile and responsive to the dynamic nature of AI software delivery.
From Development to Deployment: Streamlining AI Workflows
The journey from development to deployment of AI applications is a critical phase where efficiency and precision are paramount. By leveraging CI/CD pipelines, teams can ensure a consistent and automated path for their AI-driven solutions, from initial coding through to production.
- Quality Gatekeeping: A robust CI/CD system acts as a gatekeeper, maintaining high standards for AI applications before they reach production.
- Rollbacks and Retraining: In case of performance issues, such as model drift, the system can rollback, retrain, and redeploy, keeping applications robust.
- Enhanced Collaboration: CI/CD fosters better team collaboration and accelerates development timelines.
AI-powered software delivery solutions enhance enterprise efficiency, productivity, and cost-effectiveness. Businesses benefit from automation, predictive analytics, and improved user experiences, gaining a competitive edge in the market.
The integration of AI tools into existing tech stacks requires not just external support from tool providers, but also internal efforts to promote adoption and celebrate incremental progress. This dual approach ensures that AI initiatives are not only technically sound but also aligned with business objectives.
AI and the Evolution of DevOps Practices
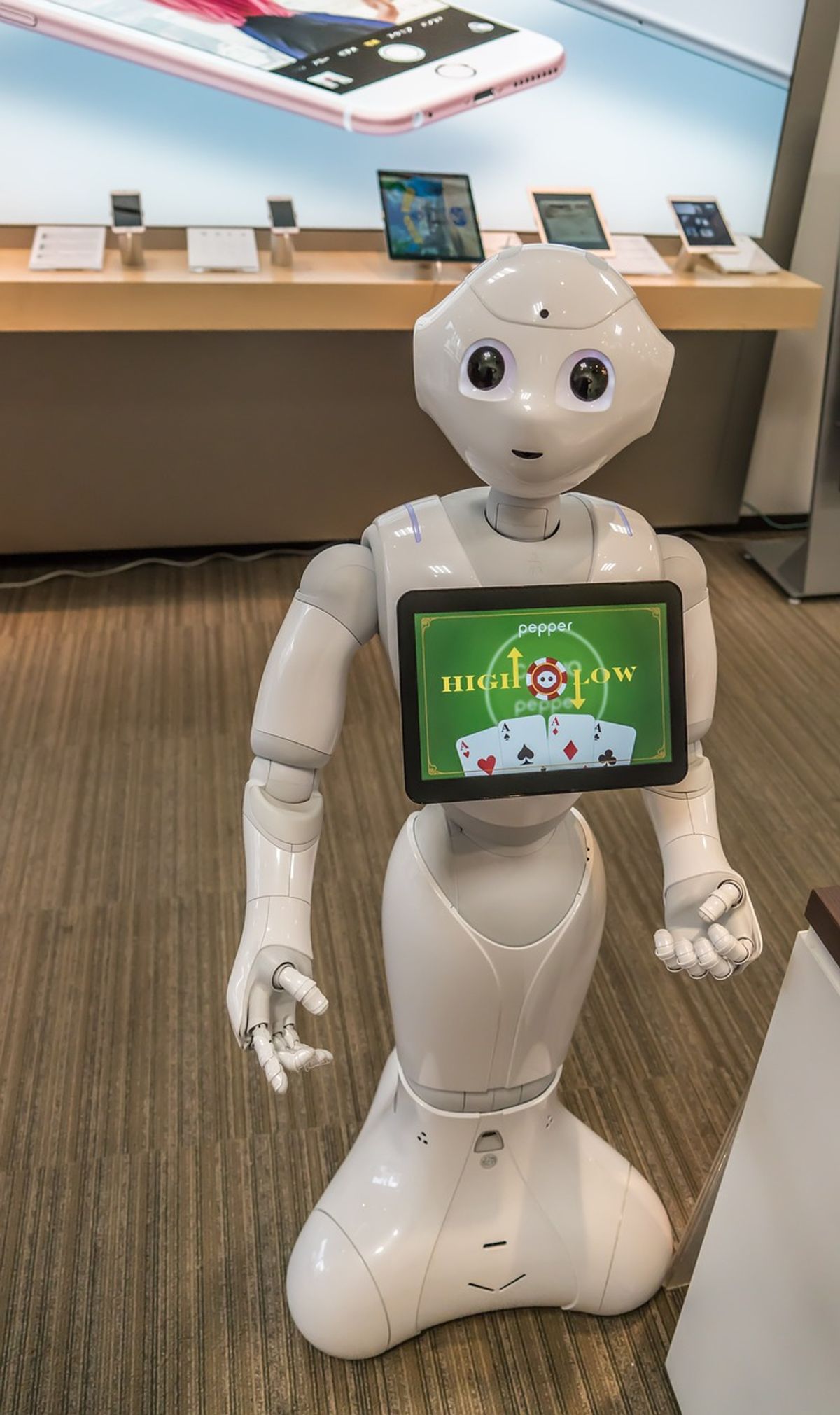
Incorporating AI into Continuous Testing
The integration of Artificial Intelligence (AI) into continuous testing is pivotal for enhancing the quality and reliability of AI-powered applications. Automated testing and fast feedback loops are essential for identifying and mitigating risks such as model drift, which can compromise the integrity of AI systems.
- Automated testing minimizes manual errors and enhances reproducibility.
- Fast feedback loops facilitate rapid issue identification and resolution.
- Integration with CI/CD pipelines ensures consistent application updates.
By integrating AI with robust CI/CD practices, engineering leaders can navigate the complexities of delivering AI-powered software, transforming potential into performance and vision into reality.
AI integration in DevOps revolutionizes software delivery with automation, predictive analytics, and specialized roles. However, challenges such as compatibility and the need for a cultural shift must be addressed. Strategic planning and the development of advanced skills are key for success in this new era of software delivery.
Leveraging AI for Enhanced DevOps Automation
The integration of artificial intelligence (AI) into DevOps practices is not just a trend; it's a paradigm shift that is redefining the landscape of software delivery. AI-driven automation is set to revolutionize the DevOps workflow, leading to faster deployment and enhanced performance. By leveraging AI tools, teams can automate complex tasks that were once manual, reducing the potential for human error and increasing efficiency.
The synergy between AI and DevOps is creating a new frontier for innovation. As AI becomes more sophisticated, it can predict potential issues before they arise, optimize processes, and even self-heal systems without human intervention.
The benefits of AI in DevOps are clear, but the journey to full integration is a path filled with both opportunities and challenges. Organizations that embrace this change will find themselves at a significant advantage, turning technological challenges into opportunities for innovation and competitive advantage.
The Impact of AI on DevOps Culture
The integration of Artificial Intelligence (AI) into DevOps is revolutionizing the way organizations approach software delivery. DevOps principles combined with AI improve software delivery, enhancing code quality, accelerating reviews, and positively impacting key metrics. This synergy is not just about efficiency; it's about fostering a culture of innovation where adaptive software delivery and strategic decision-making become the norm.
Embracing AI within DevOps goes beyond mere automation; it's about creating a dynamic environment where continuous learning and improvement are ingrained in the team's ethos.
As AI becomes a competitive edge, it's crucial for teams to adapt their practices to stay ahead. Here's how AI is reshaping DevOps culture:
- Encouraging a mindset of continuous experimentation and learning
- Automating routine tasks to free up creative problem-solving capacities
- Enhancing collaboration through data-driven insights and predictive analytics
- Fostering a proactive approach to incident management and resolution
In conclusion, the journey of integrating AI into DevOps is essential for staying relevant in the digital age. It requires careful navigation, but with the right approach, IT organizations can emerge more agile and better equipped to face the future.
Navigating the AI Testing Maze
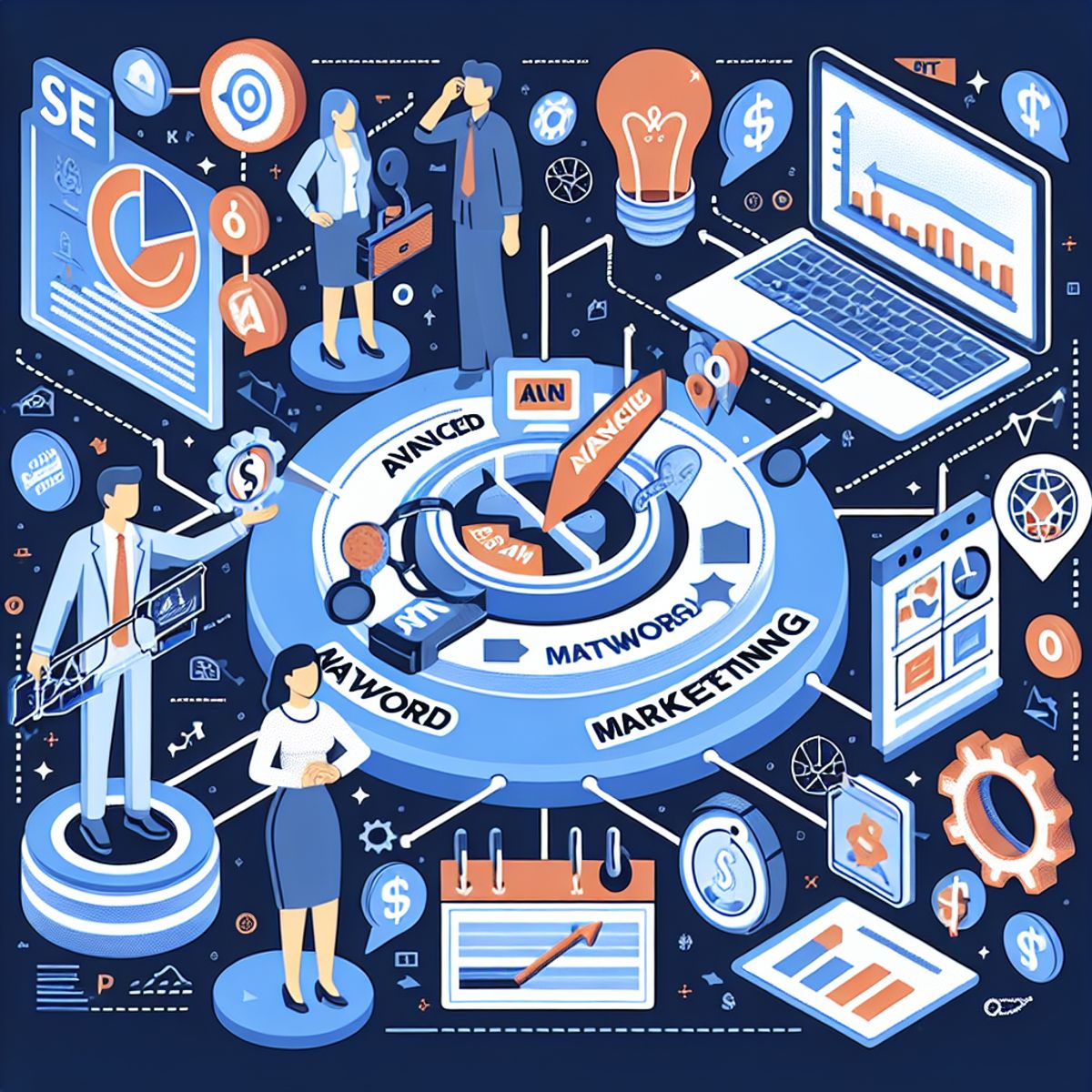
Addressing the Unpredictability of AI Systems
The advent of AI has introduced a new level of complexity to software delivery. AI systems, by nature, are probabilistic rather than deterministic, leading to outcomes that can be difficult to predict and control. This unpredictability poses a unique challenge for teams accustomed to traditional software development practices.
To navigate this maze, it's essential to establish robust testing frameworks that can handle the nuances of AI. These frameworks should be capable of addressing the infinite gray areas that AI systems present, moving beyond the binary yes/no testing approaches of the past.
Embracing the unpredictability of AI requires a shift in mindset and methodologies. Teams must learn to work with AI's inherent variability, turning it into an advantage rather than a stumbling block.
Here are key steps to address AI unpredictability:
- Define clear testing objectives that align with business goals.
- Develop a suite of tests that cover a range of probable scenarios.
- Implement continuous testing to capture and learn from unexpected behaviors.
- Utilize AI itself to predict and simulate potential outcomes.
Developing Robust Testing Frameworks for AI
The advent of AI in software development has necessitated a shift from traditional testing frameworks to more dynamic and robust systems. AI-driven feature testing and test automation are transforming software delivery, ensuring accuracy, efficiency, and real agility in development roles. This transformation is not without its challenges; AI systems introduce a level of complexity that defies the binary outcomes of conventional testing methods.
The complexity of AI systems requires a nuanced approach to testing, one that moves beyond the binary and embraces the probabilistic nature of AI outcomes.
To address this, organizations are turning to innovative CI/CD tools that integrate with evaluation platforms, automating the process and scaling quality assurance efforts. Here are some key steps to developing a robust testing framework for AI:
- Establishing clear testing objectives that align with AI's probabilistic behavior.
- Integrating automated testing tools that can handle the unpredictability of AI systems.
- Ensuring continuous feedback loops for iterative improvement of AI models.
By redefining testing protocols to accommodate the infinite gray of AI decision-making, teams can better prepare for the subjective outcomes that AI systems present.
From Yes/No to Infinite Gray: Testing AI Nuances
The transition from traditional software testing to AI-driven testing is marked by a shift from binary outcomes to a spectrum of possibilities. AI systems introduce a level of complexity that challenges the conventional yes/no logic, demanding a nuanced approach to testing. This complexity necessitates a framework that can handle the probabilistic nature of AI decisions, where outcomes are not just right or wrong but fall within a range of acceptability.
The key to effective AI testing lies in understanding the infinite gray areas and preparing to address them through comprehensive strategies.
To navigate this new landscape, several steps are essential:
- Embracing continuous learning and adaptation in testing practices.
- Developing metrics that capture the quality of AI decisions beyond accuracy.
- Implementing robust monitoring systems for ongoing validation.
These steps ensure that AI systems are not only functional but also aligned with the intended user experience and business objectives. As AI continues to evolve, so too must our methods for ensuring its reliability and effectiveness.
Human-Centric AI: Balancing Automation and Empathy
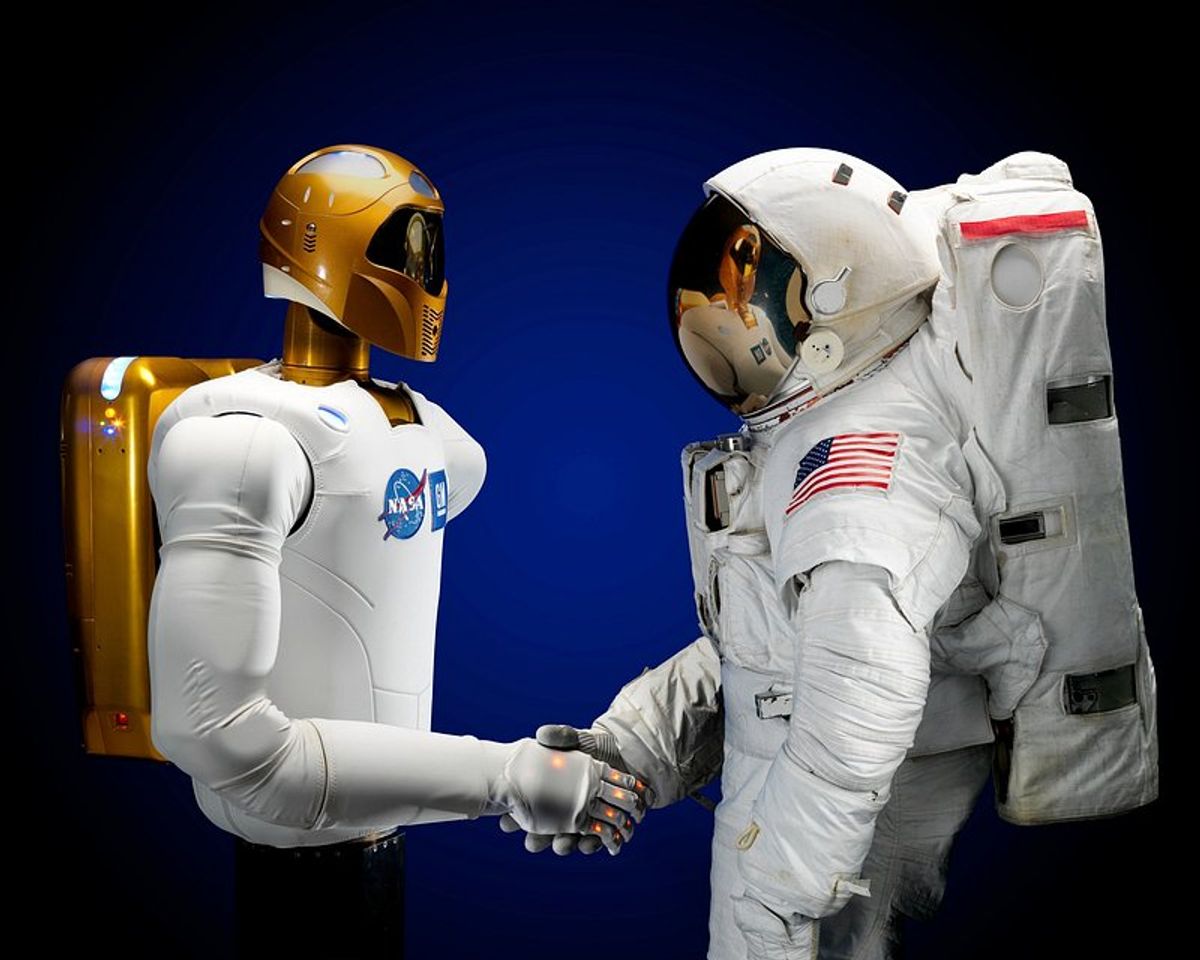
Maintaining the Human Connection in AI Services
In the pursuit of efficiency and automation, it's crucial to remember that AI services must complement, not replace, the human touch. The emotional connection and empathy inherent in human interactions are irreplaceable and must be woven into the fabric of AI-driven solutions.
AI is transforming customer experiences, but the integration of human empathy alongside AI's smart capabilities is what truly revolutionizes service delivery. This synergy ensures that technology amplifies rather than diminishes the value of human interaction.
To maintain a human-centric AI approach, it is vital to support staff through change management, addressing fears of job loss and ensuring high employee retention. This balance is key to sustaining both employee satisfaction and customer loyalty.
Here are some steps to ensure the human connection in AI services:
- Continuously monitor user interaction with AI features and gather feedback.
- Implement regular updates and optimizations based on user feedback.
- Prioritize ethics, data privacy, and the balance between automation and human interaction.
By adhering to these practices, enterprises can navigate the delicate balance of advancing technology while preserving the essence of human connectivity.
Ethical Considerations in AI Deployment
As enterprises embrace AI, ethical considerations must be at the forefront of deployment strategies. Ensuring AI ethics align with corporate values is not just a moral imperative but a business necessity. Ethical lapses can lead to reputational damage, which is why 80% of leaders recognize that AI security, ethics, and governance are crucial for future success.
Transparency, accountability, and inclusivity are key pillars of ethical AI. It's essential to establish principles and frameworks that guide the deployment of AI technologies. These should address concerns such as privacy violations and biased decision-making, which are top apprehensions among leaders.
The integration of AI into business operations demands a careful balance between technological advancement and ethical responsibility.
Here are some of the core ethical principles that leading enterprises and NGOs have begun to publish:
- Fairness
- Safety
- Explainability
- Social benefit
- Human oversight
Adopting AI responsibly means considering these principles not only to mitigate risks but also to foster trust and ensure that AI services contribute positively to society.
Designing AI Systems with User Experience in Mind
When designing AI systems, the end-user experience is paramount. AI-driven personalization in enterprise software not only enhances user satisfaction but also engagement and productivity. By focusing on the user, AI can also improve customer support, security, and compliance in software delivery, leading to better user experiences and business outcomes.
To ensure that AI systems are user-centric, it's essential to follow a structured approach:
- Identify User Needs: Engage with users to understand their challenges and expectations.
- Prototype and Iterate: Develop prototypes and iterate based on user feedback.
- Optimize AI Models: Test and refine AI models for accuracy and user relevance.
- Deployment: Integrate AI seamlessly, prioritizing security and user experience.
- Monitor and Adapt: Continuously gather user feedback and adapt the system accordingly.
Empathy is a key ingredient in the design process, ensuring that AI solutions are not only efficient but also resonate with the human aspect of enterprise operations. It's about striking the right balance between sophisticated technology and the nuances of human interaction.
AI in Enterprise Logistics: A Game Changer
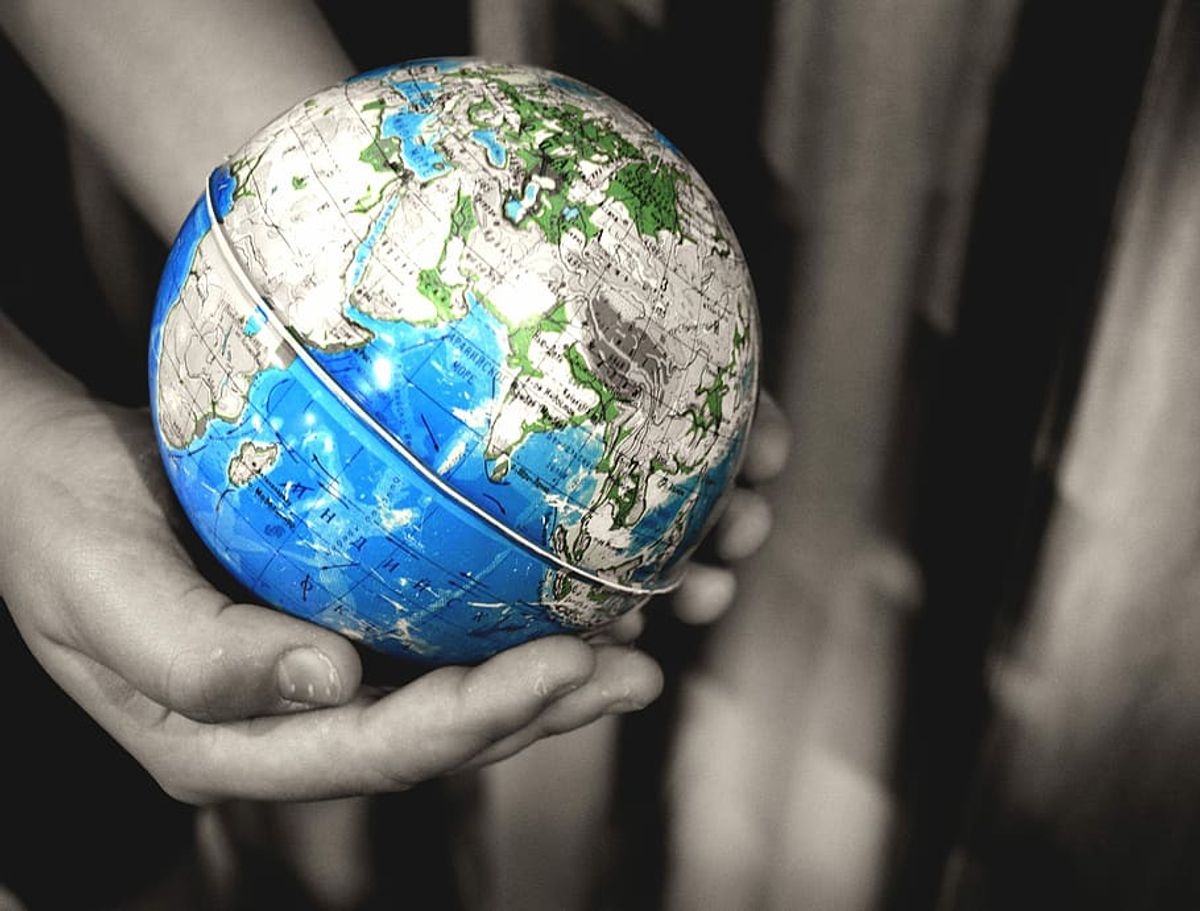
Optimizing Operations with AI-Driven Insights
The integration of AI into enterprise logistics marks the beginning of a new era, where data insights and analytics are not just supportive elements but core drivers of operational strategy. By harnessing the power of AI, businesses are able to transform their approach to data, turning vast amounts of information into actionable insights that drive efficiency and informed decision-making.
AI-driven transformation is not limited to the analysis of data; it also encompasses the automation of operations. This leads to streamlined processes and optimized performance, which are essential in today's fast-paced market. For instance, AI can enhance capacity utilization, minimize waste, and improve route optimization, leading to significant cost savings and increased competitiveness.
The proactive decision-making and adaptive planning enabled by AI are pivotal in maintaining operational agility. As AI solutions continuously monitor and optimize performance, businesses can stay ahead of the curve, ensuring they meet the ever-evolving market demands and regulatory requirements.
To fully leverage AI-driven insights, it's crucial to understand the technologies involved, their capabilities, and how to effectively utilize them in day-to-day operations. Here are some key areas to focus on:
- Monitoring and Performance Optimization
- Risk Management and Compliance
- Proactive Decision-Making and Adaptive Planning
Revolutionizing Customer Service through AI
AI is taking center stage when it comes to elevating customer service experiences. When we mix the smart capabilities of AI with the kind of empathy only humans can provide
AI boosts customer satisfaction and customer retention, with businesses using AI-powered tools like Zendesk bots witnessing a notable improvement in CSAT scores. This synergy of technology and human touch is not just a trend but a transformative force in customer service.
The integration of AI into customer service platforms such as Salesforce, Zendesk, and ServiceNow has been a game-changer. These platforms have become more effective and cost-efficient by leveraging GenAI, which is based on rich data and continuous learning.
AI transformation is making significant strides in reshaping customer support, placing it at the forefront of business processes.
The journey from legacy systems to innovative AI-driven customer experiences presents both opportunities and challenges. It requires a strategic approach to integrate AI into existing IT frameworks and a commitment to continuous improvement and adaptation.
Adaptive Strategies for Dynamic Market Conditions
In today's volatile business environment, characterized by high inflation, rising interest rates, and increasing data breaches, enterprises must navigate a labyrinth of challenges. AI-driven adaptive strategies are pivotal in maintaining a competitive edge under such dynamic market conditions. By leveraging AI, businesses can achieve a level of responsiveness that manual decision-making processes simply cannot match, allowing them to capitalize on emerging opportunities and mitigate risks with unprecedented agility.
Forecasting Market Trends
- Predicting changes in market demand and consumer behavior
Risk Management - Identifying and mitigating potential risks
AI solutions can dynamically adjust capacity levels based on evolving demand signals, market dynamics, and external factors such as weather patterns or economic trends.
The lack of scalability in manual processes is a significant hurdle for growing businesses. AI not only enhances scalability but also ensures that enterprises are not left behind due to inflexibility or delayed responses to market shifts. As the volume and complexity of data increase, AI's ability to process and analyze vast datasets becomes an invaluable asset for forward-thinking organizations.
The Competitive Edge of AI Adoption
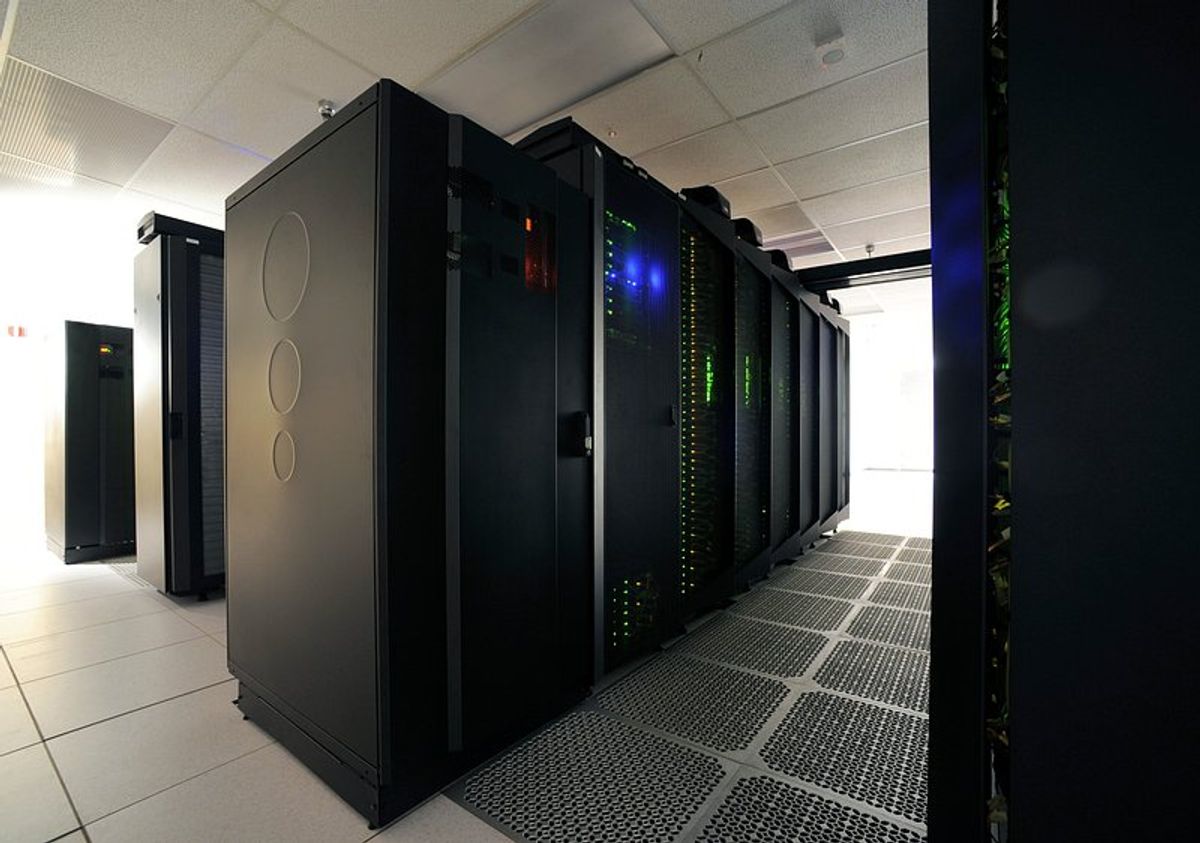
First-Mover Advantages in AI Technology
Enterprises that quickly adopt new AI technologies may secure significant first-mover advantages, setting the stage for enhanced revenue streams, improved customer satisfaction, and a more engaging employee experience. The competitive edge gained from being an early adopter can be substantial, as it allows businesses to outpace their rivals in innovation and market responsiveness.
However, the journey to integrating AI is not without its challenges. Similar to the early days of the internet, the promise of AI comes with the need for strategic implementation. Organizations must navigate the complexities of AI development, ensuring that the technology is not only adopted but also effectively incorporated into products and services.
The digital age demands that enterprises not only keep up with the latest technologies but also anticipate and shape future trends. AI offers a unique opportunity to do just that, by enabling organizations to deliver superior digital experiences and respond proactively to market changes.
To truly capitalize on AI, companies must identify early ROI opportunities and craft a smart expansion plan. This approach ensures a solid foundation for broader AI and automation implementation, future-proofing the enterprise against the relentless pace of technological change.
Enhancing Customer and Employee Experiences
The AI revolutionizes enterprise software delivery, enhancing user experience with personalization and predictive analytics, and serves as a catalyst for IT transformation. By leveraging technology to create simple, personalized, and intuitive experiences, AI is not just transforming customer interactions but also empowering employees. This empowerment boosts their knowledge, skills, and compensation, enabling them to win customers' hearts.
- Identifying high-value customers and understanding their needs
- Effectively cross-selling and upselling to maximize customer value
- Building strategies to retain customers and extend their lifespan
- Providing tailored solutions to increase customer retention
AI transforms experiences, enhancing customer satisfaction and loyalty through personalized interactions. It unlocks growth and customer success, evolving the ability to delight customers beyond just transactions.
In today's competitive market, a seamless and personalized journey that exceeds expectations is crucial. Organizations must combine the best technology with empathetic customer service to create experiences that truly differentiate and impact customer loyalty.
Maximizing Value from Existing Data and Systems
In the age of AI, the treasure trove of existing data within an enterprise can be the key to unlocking significant competitive advantages. Automating data processing not only minimizes manual errors but also frees up valuable human resources for more strategic tasks. By identifying patterns and anomalies, AI systems provide insights that can transform business operations and customer engagement.
- Ensure Data Integrity: Regular data cleaning and validation are essential to maintain the accuracy of AI outputs.
- Customize for Specific Needs: Tailoring AI tools to specific business challenges maximizes their effectiveness and value.
While multiple teams can benefit from shared access to data, the challenge lies in presenting it securely and contextually to non-originating users. Effective data governance initiated at the source ensures a deep understanding of the data's origin and value.
Leveraging predictive analytics is crucial for enterprises aiming to stay ahead. It allows for proactive strategies, such as identifying high-value customers and creating personalized experiences, which are instrumental in customer retention and satisfaction. The integration of AI into existing workflows should be done with an eye on data privacy and security, and teams must be trained to harness the full potential of these tools.
The Future of AI-Powered Innovation
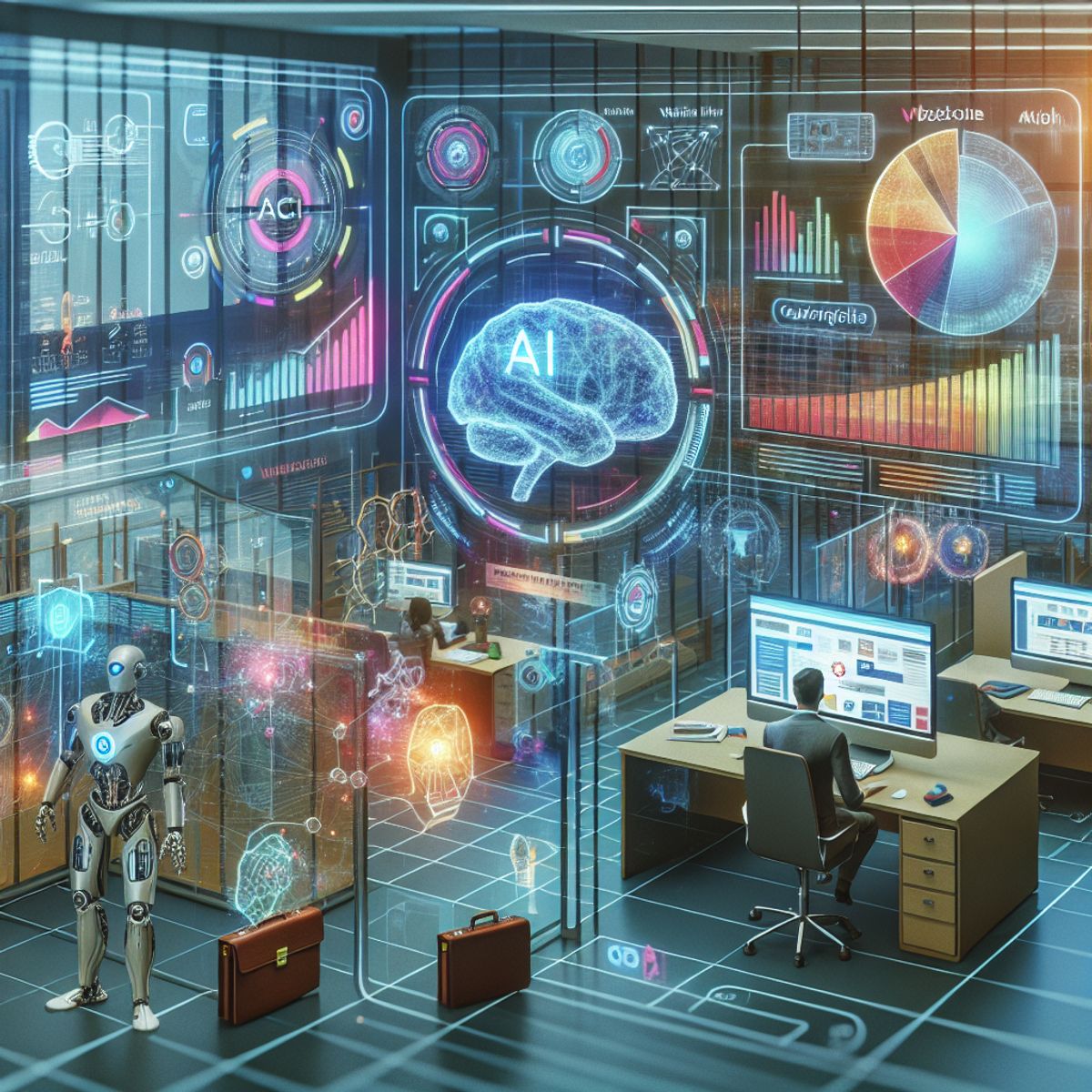
Predicting Market Trends with AI Analytics
The advent of AI analytics has revolutionized the way enterprises approach market trend prediction. Predictive analytics for demand forecasting is a prime example of how AI algorithms synthesize historical sales data, market trends, and external factors to anticipate future demand with unprecedented precision. This anticipatory analytics capability allows businesses to make proactive decisions, optimize resources, and seize opportunities ahead of competitors.
By applying machine learning algorithms to diverse data sources, AI-driven decision support systems generate insights that empower data-driven decision-making.
Real-time analytics further enhance an organization's agility, processing vast data streams to enable swift responses to market fluctuations. Here's how AI analytics contributes to different aspects of market trend prediction:
- Data Analysis and Insights Generation: Extracting patterns and insights from historical records and real-time data.
- Predictive Analytics for Forecasting: Improving forecasting accuracy by analyzing patterns, trends, and demand drivers.
- Real-time Market Response: Enabling immediate adjustments in strategies such as pricing and supply chain optimization.
AI as a Catalyst for New Business Models
The advent of AI is not just transforming existing business models; it's creating entirely new ones. AI's capacity to analyze vast datasets and uncover patterns is enabling businesses to predict customer behavior, personalize services, and enter markets previously inaccessible. For startups, AI acts as a catalyst, accelerating the journey from concept to market presence.
- AI-driven personalization: Tailoring products and services to individual customer preferences.
- Predictive analytics: Anticipating market trends and customer needs.
- Automation of routine tasks: Freeing up human talent for more creative and strategic roles.
AI is reshaping the competitive landscape by offering innovative ways to create value. It's not just about automating processes; it's about leveraging AI to identify and capitalize on new opportunities.
However, it's crucial to recognize that AI is a tool, not a panacea. While it can provide a competitive edge, it requires careful integration with business strategy and continuous refinement to deliver on its promise. The sustainability of these new business models depends on a deep understanding of both the technology and the market it serves.
Sustaining Success in an AI-First World
In an AI-first world, sustaining success requires a strategic approach that goes beyond initial implementation. Fostering an AI-ready culture is paramount, where continuous learning and adaptability become the norm. Encouraging teams to embrace AI tools and methodologies is essential, with a focus on upskilling and reskilling to maintain a competitive edge.
To ensure longevity, companies must also implement ethical AI guidelines. These should emphasize transparency, accountability, and fairness, integrating privacy and security measures as foundational elements of AI initiatives. This ethical framework not only supports sustainable AI practices but also builds trust with stakeholders.
Accelerating AI-powered innovation is key to winning tomorrow's market. The journey is demanding, but with incremental processes and a dedicated point person to assess effectiveness, companies can navigate the complexities of AI integration and emerge victorious.
Lastly, the ability to predict and adapt to market trends using AI analytics will be a distinguishing factor for companies. Those who invest in deep AI research acumen and foster a culture that celebrates small wins will be well-positioned to thrive in the dynamic AI landscape.
As we stand on the brink of a technological revolution that will fundamentally alter the way we live, work, and relate to one another, AI-powered innovation is at the forefront of this transformation. The potential for AI to drive efficiency, enhance scalability, and unlock new opportunities is immense. To ensure you're not left behind, visit OptimizDBA for unparalleled database optimization consulting and experience transaction speeds like never before. Embrace the future with confidence and let us help you accelerate your data solutions. Click here to learn how we can transform your business with our proprietary methodologies and extensive experience.
Conclusion
As we stand on the brink of a new era in enterprise software, the integration of AI into the delivery landscape is not just an option—it's an imperative. The insights and examples discussed throughout this article underscore the transformative power of AI, from enhancing CI/CD practices to driving innovation and maintaining a competitive edge. Enterprises that embrace AI and continuously adapt their software delivery models will not only survive but thrive, unlocking a future of possibilities and achievements. The journey towards AI-powered delivery is complex, yet it is clear that the readiness to evolve and the courage to embrace change are the hallmarks of tomorrow's industry leaders. The future is AI, and the time to navigate its landscape is now.
Frequently Asked Questions
What challenges do enterprises face in AI software delivery?
Enterprises face complexities in AI implementation, the need for continuous integration and delivery, and the necessity to streamline AI workflows from development to deployment.
How can leadership influence AI adoption in organizations?
Leadership plays a crucial role by aligning AI initiatives with business goals, fostering a culture of innovation, and investing in the necessary infrastructure and training.
What technical preparations are needed for AI integration?
Organizations must evaluate and upgrade their infrastructure, upskill their teams for AI development, and ensure high data quality and accessibility for effective AI deployment.
How does AI impact DevOps practices?
AI is reshaping DevOps by enabling continuous testing automation, enhancing DevOps automation, and influencing the culture towards more proactive and data-driven practices.
What are the ethical considerations in deploying AI?
Ethical considerations include maintaining transparency, ensuring fairness and non-discrimination, respecting privacy, and designing systems that prioritize user experience and trust.
How can AI transform enterprise logistics?
AI can optimize operations with insights, revolutionize customer service, and provide adaptive strategies for dynamic market conditions, leading to significant improvements in efficiency and service quality.
What competitive advantages does AI adoption offer?
Early adoption of AI can provide first-mover advantages, enhance customer and employee experiences, and maximize the value extracted from existing data and systems.
How can businesses sustain success in an AI-first world?
Businesses can sustain success by predicting market trends with AI analytics, leveraging AI as a catalyst for new business models, and continuously adapting to technological advancements.