Generative AI: The New Frontier in Business Intelligence
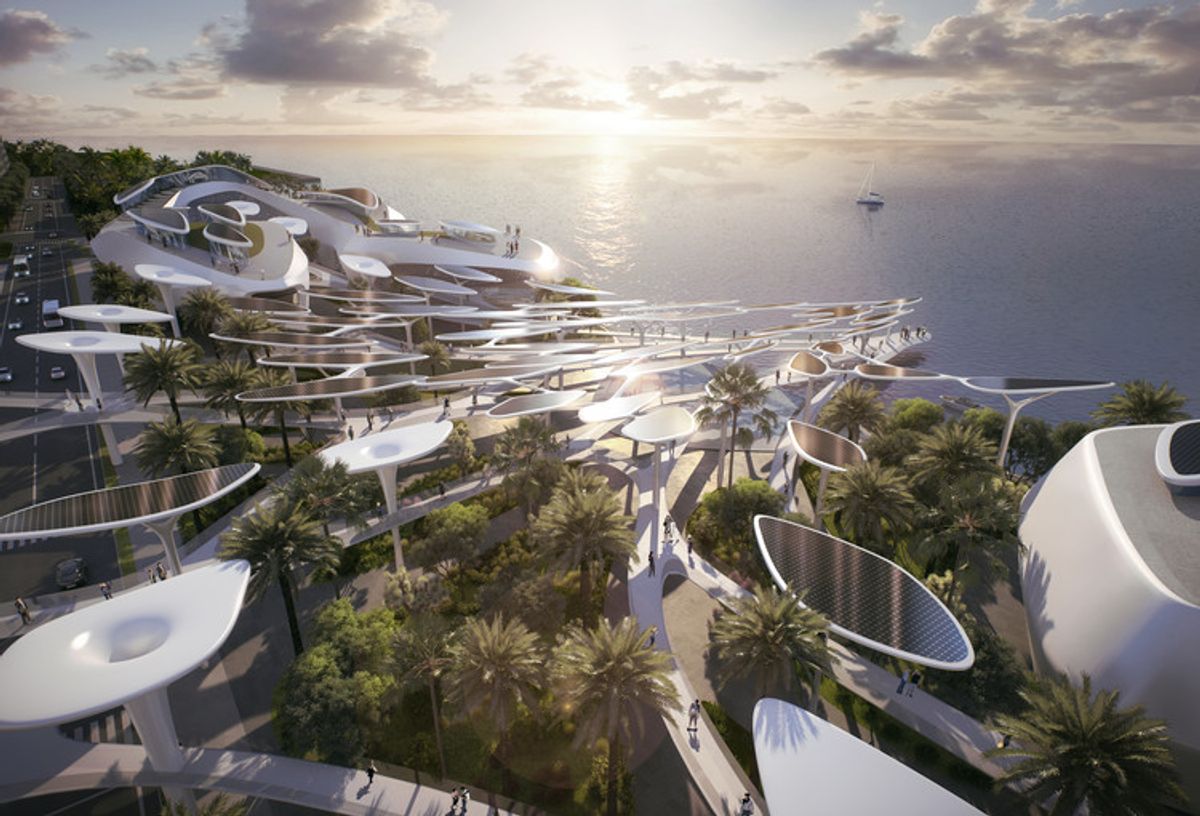
Bridging the Skills Gap with Generative AI
The advent of Generative AI is revolutionizing the way businesses approach the skills gap in their workforce. By automating complex tasks such as code generation and data analysis, these AI systems enable individuals without deep technical expertise to perform advanced functions. Generative AI is democratizing the creation and management of AI solutions, making them accessible to a broader range of users.
Generative AI is not only about automating tasks but also about enhancing human capabilities. For instance, synthetic data generation allows for effective training of machine learning models without the need for extensive real-world datasets. This innovation is pivotal in industries where data sensitivity or scarcity is an issue.
The integration of Generative AI into business processes is expected to significantly reduce the time and resources spent on training and upskilling employees, while simultaneously fostering innovation and efficiency.
The following table highlights the impact of Generative AI on workforce skills development:
Aspect | Impact of Generative AI |
---|---|
Accessibility | Simplifies complex tasks for non-experts |
Efficiency | Reduces time for training and upskilling |
Innovation | Encourages creative problem-solving |
Data Management | Enables use of synthetic data for model training |
As we move forward, the role of Generative AI in bridging the skills gap will likely become more pronounced, with a focus on fostering a collaborative environment where human intelligence is augmented by artificial capabilities.
The Rise of Self-Service BI and Data Democratization
The landscape of Business Intelligence (BI) is being transformed by the increasing demand for self-service tools that empower employees across organizational levels. Self-service BI platforms are becoming more user-friendly and highly automated, incorporating data science tools that are accessible without the need for advanced degrees. This shift is a testament to the broader trend of data democratization, where decision-making is supported by real-time insights and data visualization, enabling a competitive edge.
The future of Business Intelligence and Analytics is not just about providing data, but about equipping all users with the tools to explore and interpret that data effectively.
As the barriers to entry lower, the role of traditional data analysts is evolving. With the rise of citizen developers, there is a growing sentiment that specialized data skills will continue to be valuable, yet the democratization of data suggests a future where everyone can perform analyst roles to some extent. This leads to a critical question: Will data analysts become redundant, or will their roles simply transform?
- Data governance and privacy remain critical.
- Citizen data scientists are emerging, reshaping traditional analyst roles.
- Automation and IoT are key areas for software improvement and cost management.
Generative AI's Impact on the Role of Data Analysts
The advent of Generative AI is revolutionizing the role of data analysts by automating complex data tasks and enabling more strategic, insight-driven work. Data analysts are now evolving into roles that require deeper analytical thinking and creativity, as they shift from routine data processing to interpreting the sophisticated outputs of AI systems.
- Generative AI assists in creating synthetic data, which is crucial for training machine learning models without the constraints of sourcing real-world data.
- It fosters a new level of efficiency in tasks such as demand forecasting and supply chain optimization.
- Analysts are increasingly involved in setting up and refining AI models to ensure their outputs are aligned with business goals.
The integration of Generative AI into business intelligence tools is not just a trend; it's a transformative force that is reshaping the landscape of data analysis and decision-making processes.
Cloud Computing Innovations: Reshaping BI Infrastructure
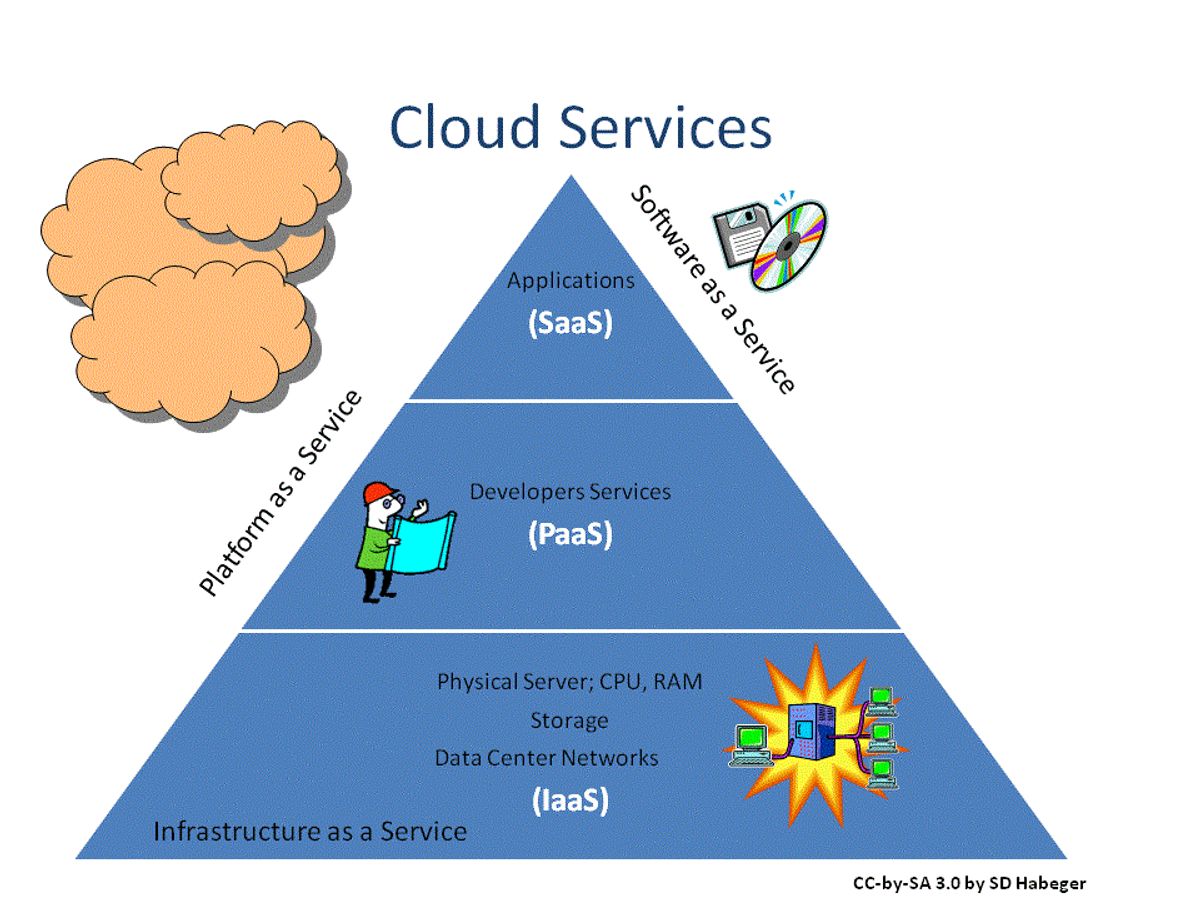
Cost-Effective Querying in the Cloud
In the era of cloud computing, cost-effective querying has become a cornerstone for businesses seeking to leverage their data without incurring prohibitive expenses. Cloud-based BI solutions offer cost-effective, scalable, and accessible data analytics tools, tailored to business needs for a competitive advantage. By opting for cloud services, companies can significantly reduce the overhead associated with maintaining on-premises hardware and software.
Cloud subscriptions are usage-based, meaning you only pay for what you use. This includes the number of queries, allocated RAM, concurrency, data volume, and server hardware.
To further optimize costs, some vendors provide in-database analysis, which eliminates the need to move large datasets. Usage reports can help identify peak consumption times, allowing for strategic scaling back of low-priority queries. Additionally, implementing access restrictions based on usage quotas or roles can effectively manage query volumes.
Strategy | Description |
---|---|
In-database Analysis | Avoids moving data for analysis |
Usage Reports | Identifies peak times to optimize query costs |
Access Restrictions | Manages query volumes based on quotas or roles |
As the cloud services market continues to grow, reaching an estimated $2.5 trillion by 2031, the demand for real-time analytics must be balanced with the costs of live querying. The trend towards cost-effective querying techniques is expected to persist, driven by the need to maintain agility and responsiveness in a data-driven business landscape.
Integration of BI Tools with Cloud Services
The integration of BI tools with cloud services marks a significant shift in how businesses approach data analysis and reporting. Cloud-based BI tools offer unparalleled flexibility and scalability, allowing organizations to adjust resources on-demand and manage costs effectively. The ease of access to BI capabilities across the organization fosters a culture of data-driven decision-making.
- Seamless collaboration and sharing of insights
- Rapid deployment and updates of BI applications
- Enhanced security with centralized data governance
The synergy between cloud services and BI tools is transforming the landscape of business intelligence, making advanced analytics accessible to a wider audience without the need for extensive IT infrastructure.
As cloud BI verticals continue to expand, we see a growing trend in sectors such as banking and insurance, where adoption rates have soared. The ability to integrate with various cloud services is not just a convenience; it is becoming a competitive necessity.
Cloud Verticals and Their Influence on BI
The advent of cloud verticals has ushered in a new era of industry-specific solutions, tailoring the cloud's versatility to meet the unique needs of different sectors. Connected cloud platforms are revolutionizing BI, offering unprecedented flexibility while also introducing new challenges.
- Digital assistants are streamlining language transcription, enhancing the accessibility of BI tools for a broader user base.
- Collaborative BI environments, empowered by AI, are setting the stage for future BI trends, emphasizing the importance of synergy between technology and human expertise.
The integration of cloud verticals into BI strategies is not just a trend; it's a transformative movement that is reshaping how businesses leverage data for competitive advantage.
Cloud verticals are not only changing the technical landscape but also influencing the human aspect of BI. The rise of citizen developers signifies a shift towards more inclusive data analysis, although specialized data skills remain crucial. With decision intelligence on the horizon, businesses are poised to enhance critical decision-making through advanced data science technologies.
Data Sharing and Monetization: Unlocking New Value
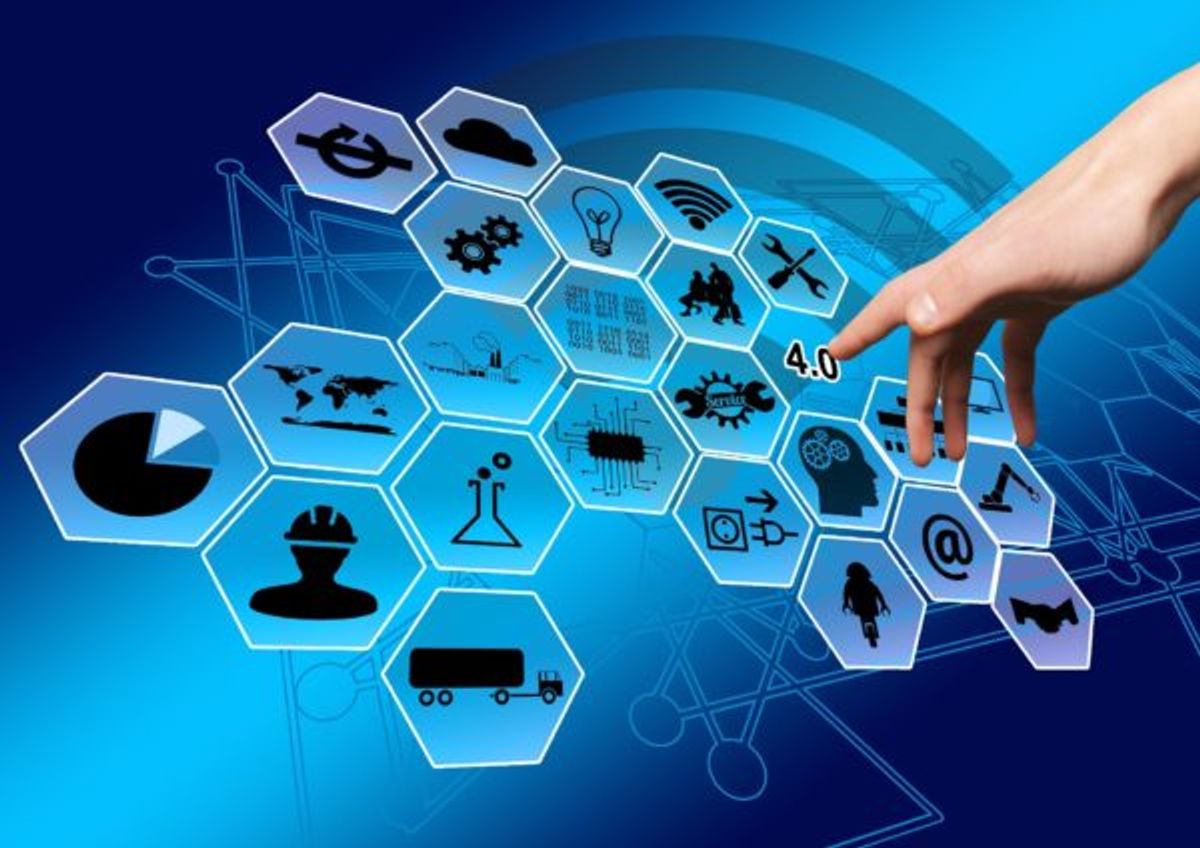
Breaking Down Information Silos
The evolution of business intelligence in 2023 is marked by a concerted effort to break down information silos. As organizations recognize the limitations of isolated data, the push towards a more interconnected and collaborative data environment intensifies. Data sharing and monetization are not just buzzwords but essential strategies for leveraging the full potential of business intelligence.
A key aspect of this trend is the adoption of data fabrics and data marketplaces, which facilitate the exchange of data within the boundaries of regulations such as GDPR. These platforms enable companies to buy, sell, or exchange data without the need for additional hardware or databases, thanks to modern processing technologies that can handle data even in encrypted form.
The demand for software that can encrypt and anonymize data for sharing will rise, ensuring that moving out of silos is a secure and controlled process.
However, the transition is challenging. Making data securely available and processing incoming encrypted data can be daunting for teams already burdened with tasks. Moreover, there is resistance from certain quarters, such as family-owned businesses and companies with entrenched data practices, who are hesitant to share their information externally. The journey towards dismantling information silos is complex but necessary for the future of business intelligence.
Emerging Data Marketplaces
The advent of data marketplaces marks a significant shift in the business intelligence landscape. Companies now have the ability to monetize their data assets, creating new revenue streams and fostering innovation. These marketplaces operate within the boundaries of regulations such as GDPR, ensuring that data exchange is secure and compliant.
Data marketplaces offer a platform for enterprises to buy, sell, or exchange data without the need for additional hardware or databases. This is made possible by modern processing technologies that can handle data even in its encrypted form. The benefits are twofold: companies can unlock the value of their data, and end users receive better products and services as a result.
The rise of data marketplaces is transforming how organizations approach data sharing and monetization, enabling a more fluid and dynamic BI ecosystem.
For instance, Snowflake's data clean rooms allow for the secure combination of proprietary data with third-party data, providing comprehensive customer insights. In regulated industries such as finance, healthcare, and insurance, this can lead to a deeper understanding of patients, loan applicants, and customers. Moreover, companies like John Deere have begun to sell data collected from sensors in their machinery, offering valuable information back to the users of their equipment.
Ethical Considerations in Data Monetization
As businesses venture into the realm of data monetization, ethical considerations take center stage. The commodification of data raises questions about privacy, consent, and ownership. It's crucial to balance the economic benefits with the responsibility to protect individual rights.
Data democratization empowers organizations by making data accessible to all, driving collaboration, informed decision-making, and innovation. However, monetizing data must be approached with caution to ensure that it does not compromise ethical standards or consumer trust.
The challenge lies in creating a framework that aligns data monetization strategies with ethical practices, ensuring that all stakeholders benefit fairly.
Here are some key ethical guidelines to consider:
- Establish clear data governance policies that define ownership and usage rights.
- Ensure transparency in how data is collected, used, and sold.
- Obtain explicit consent from individuals before using their data.
- Implement robust security measures to protect data from breaches.
- Comply with all relevant data protection regulations, such as GDPR.
Data Mesh: Decentralizing Data Management
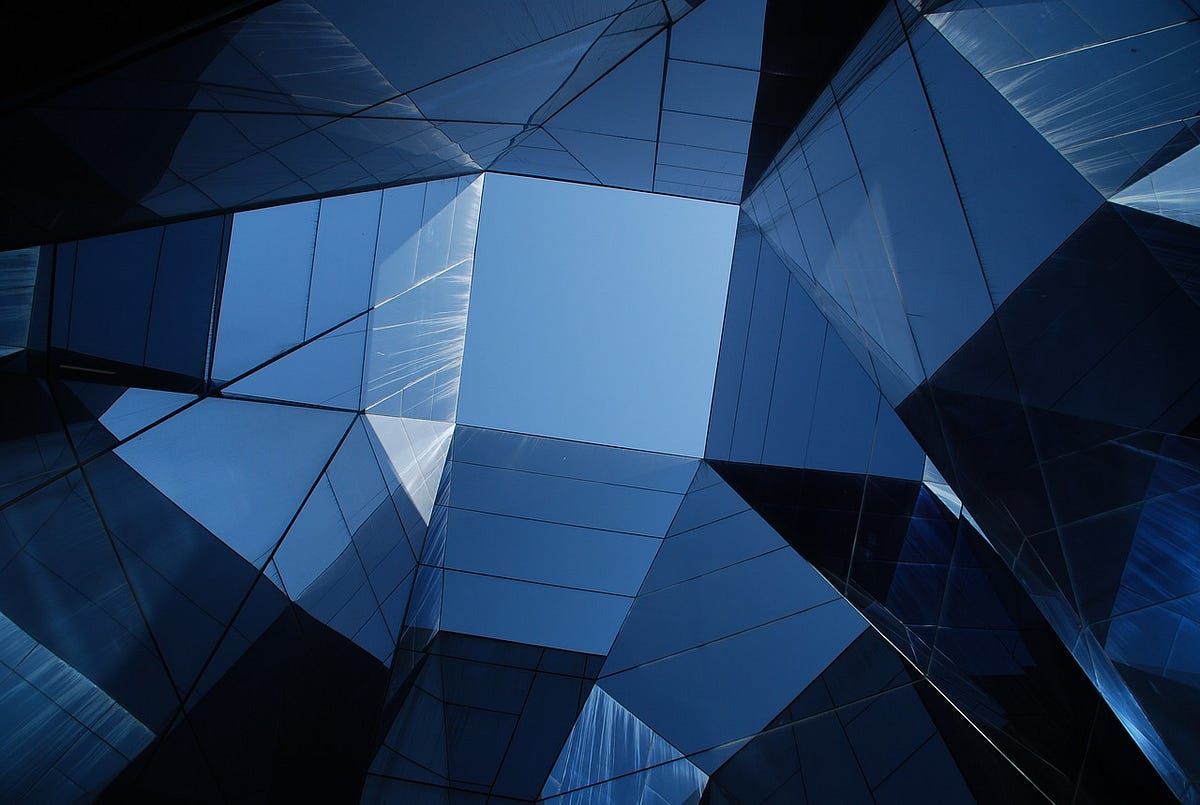
Principles of Data Mesh Architecture
The data mesh concept is transforming the landscape of data architecture by advocating for a decentralized approach to data management. Data mesh shifts the paradigm from monolithic, centralized systems to a distributed model where domain-specific teams are empowered with the ownership of their respective data products. This model promotes agility and scalability, as it aligns responsibility with the domain experts who best understand the data's context and use.
- Decentralization of data ownership
- Domain-oriented data control
- Self-serve data infrastructure as a product
- Federated computational governance
By embracing these principles, organizations can foster a more dynamic and responsive data environment. The data mesh effectively addresses the challenges of volume and complexity in data management, paving the way for operational and analytical data to coexist and inform business decisions more effectively.
The implementation of a data mesh architecture requires careful consideration of the organization's structure and the potential need for upskilling in areas such as metadata management. Autodesk's partnership with Atlan exemplifies a successful application of the data mesh, with 60 domain teams achieving clear visibility into data consumption. As with any strategic framework, it is crucial to evaluate whether the data mesh aligns with your company's specific needs and capabilities before fully committing to this approach.
Data Mesh in Practice: Case Studies
The implementation of data mesh architecture is gaining traction as businesses seek to enhance their data governance and management. Each domain team operates autonomously, ingesting, processing, and publishing data, which fosters a culture of responsibility and continuous improvement. Autodesk's partnership with Atlan exemplifies this approach, with 60 domain teams achieving full visibility into data consumption.
The shift towards decentralized data management is not just a trend but a strategic move to accommodate scalability and rapid change.
While the benefits are clear, the transition to a data mesh architecture can reveal skills gaps, particularly in metadata management, necessitating upskilling across teams. Moreover, the suitability of data mesh varies, and organizations must carefully assess their specific needs before adopting this model.
Challenges and Opportunities in Adopting Data Mesh
Adopting a Data Mesh architecture presents a paradigm shift in how organizations manage and utilize their data. Data is a strategic asset driving business growth and innovation. By decentralizing data ownership, Data Mesh allows domain-specific teams to manage their data autonomously, enhancing analytics efficiency, security, and insights for product development and digital transformation, thus unlocking business value.
However, this decentralization also introduces challenges. Cross-functional teams must collaborate effectively, which can reveal skills gaps, particularly in areas like metadata management. Upskilling across teams becomes essential to ensure that each unit can handle their data products competently.
The demand for software that can encrypt and anonymize data for sharing will rise, emphasizing the need for consistent security assessment and robust data governance.
While the opportunities for scalability and agility are significant, organizations must carefully consider their requirements before fully committing to a Data Mesh strategy. Autodesk's partnership with Atlan, resulting in 60 domain teams with complete visibility into data consumption, exemplifies a successful implementation, yet it's clear that the journey towards a fully functional Data Mesh is nuanced and requires a tailored approach.
Data Governance and Security: Prioritizing Protection
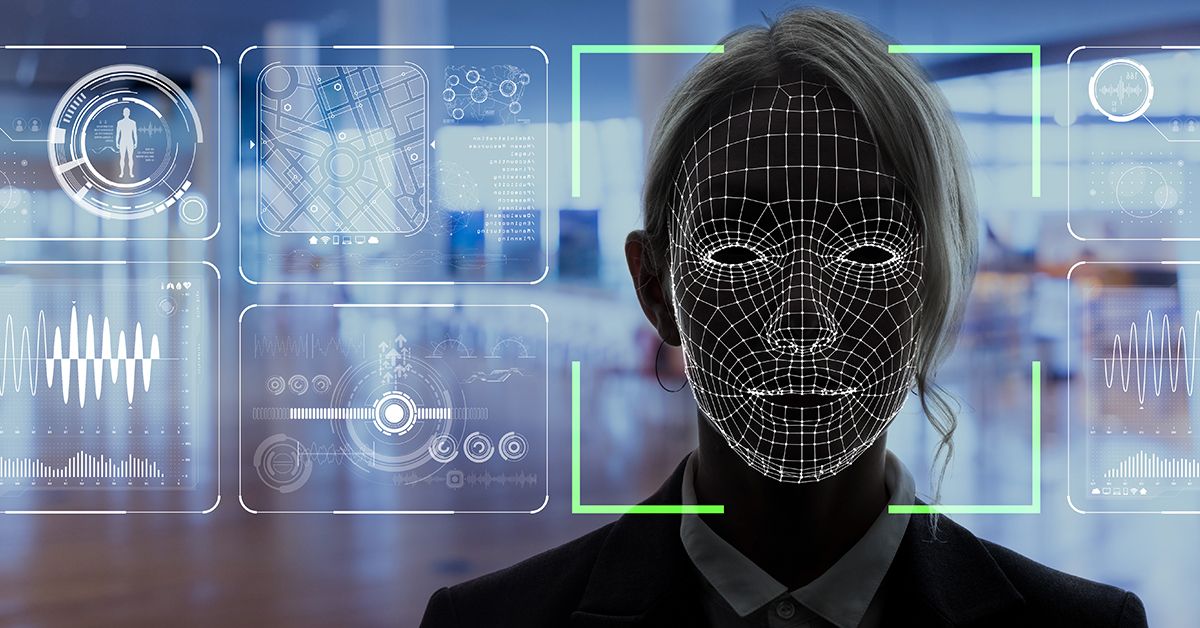
Evolving Data Privacy Regulations
In the dynamic landscape of business intelligence, data privacy regulations are becoming increasingly stringent. The General Data Protection Regulation (GDPR) has set a precedent, mandating robust security measures and governance to protect personal data from breaches and unauthorized access. Organizations are now compelled to implement technical and comprehensive strategies to comply with such regulations.
As the volume of big data escalates, an engineering-first approach is essential to tailor security features to an organization's specific needs. The integration of AI in data governance introduces new complexities, raising questions about AI's role in cybersecurity and the extent of its regulation.
Software solutions that offer built-in access control, compliance with legal requirements, and AI-driven risk assessment are becoming indispensable. These tools must facilitate smooth consent workflows and efficient data mapping to navigate the evolving landscape of data privacy.
- Insider security breaches pose a significant risk, often more damaging than external threats.
- Data governance protocols are crucial for maintaining data integrity and preventing unauthorized modifications.
- The balance between data accessibility, security, and compliance remains a critical challenge for organizations.
The key trends in Business Intelligence for 2023 highlight the importance of data privacy and governance, alongside the growing role of AI/ML in providing automation and insights for strategic decision-making.
Implementing Robust Data Governance Frameworks
In the realm of Business Intelligence, data governance is the cornerstone that ensures data integrity, security, and compliance. It is a multifaceted discipline that requires a harmonious blend of partnerships, best practices, privacy standards, and ethical frameworks. One of the pivotal elements in safeguarding sensitive data is encryption, which serves as a critical defense mechanism against unauthorized access.
The challenge lies in balancing the stringent requirements of data governance with the flexibility needed for business agility. Effective governance frameworks are not just about setting rules; they're about creating an environment where data can be used safely and effectively.
To achieve this, organizations must navigate a series of strategic steps:
- Establishing clear data ownership and defining quality standards.
- Implementing comprehensive data lifecycle management.
- Ensuring compliance with evolving regulations such as GDPR.
- Integrating technical measures with organization-wide policies to prevent security breaches.
Software solutions that offer built-in access control, consent workflows, and AI-driven risk assessment are becoming increasingly sought after. As regulations tighten and cyber threats evolve, an engineering-first approach is essential to tailor security features to an organization's specific needs.
Tackling Emerging Data Security Threats
In the face of emerging data security threats, businesses are compelled to balance the need for robust security measures with the demand for accessible data. The 2023 MOVEit attack, highlighting the widespread impact of a single vulnerability, serves as a stark reminder of the interconnected risks in our digital ecosystem.
Insider security breaches, often more damaging than external hacks, necessitate stringent oversight and sophisticated solutions. Software with built-in access control, compliance adherence, and AI-driven risk assessment is becoming increasingly vital.
As the digital landscape evolves, so too must our approach to data security. The integration of encryption and anonymization technologies is essential for safe data sharing, moving beyond silos to a more interconnected yet secure future.
The following points outline key considerations for tackling data security threats:
- Implementing engineering-first security customization
- Ensuring smooth consent workflows and efficient data mapping
- Adopting AI for cybersecurity and governance
- Addressing the challenges of securing data at the edge
Each measure represents a step towards mitigating the risks posed by sophisticated cyber threats and protecting the valuable assets within an organization.
Automation in BI: Efficiency and Scalability
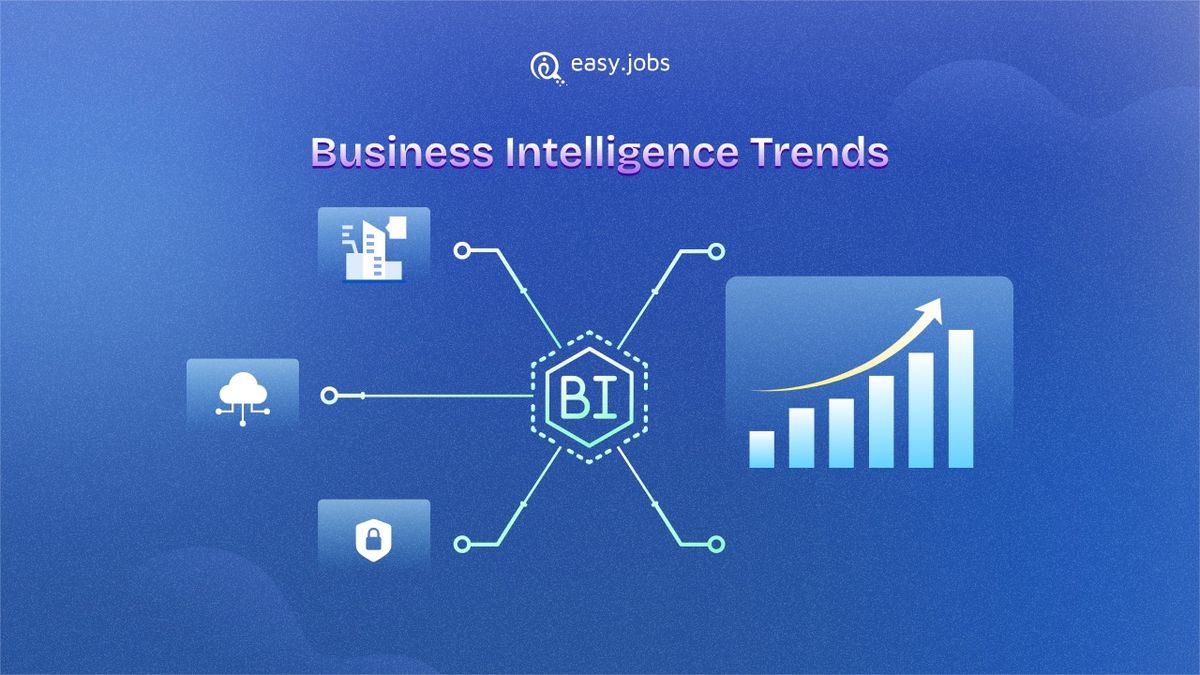
Automated Data Pipelines and Workflows
In the realm of Business Intelligence, automation is revolutionizing the way data pipelines and workflows are managed. By automating tasks such as system administration, monitoring, and database management, businesses achieve consistent, error-reduced operations. Automation extends to scalable and elastic cloud computing, exemplified by services like Oracle Autonomous Database, which adjusts resources automatically to meet demand.
Automation not only streamlines processes but also empowers citizen developers to construct and manage workflows, transforming them into reusable applications. This shift is pivotal as it aligns with the trend of data democratization, enabling faster insights and more agile go-to-market strategies.
However, transitioning to automated systems can present challenges. Legacy systems and manual configurations may hinder automation efforts. To facilitate a smoother transition, a dedicated data team is essential to drive adoption and mitigate onboarding difficulties. Here's a brief look at the areas impacted by automation:
- System administration and monitoring
- Task reviews and approvals
- Database and systems management
- Integration and OS patching
As automation becomes more prevalent, the role of data analysts is evolving, but not diminishing. The synergy between citizen developers and specialized data professionals is crucial for the future of BI.
The Role of AI in BI Automation
The integration of Artificial Intelligence (AI) in business intelligence (BI) automation is transforming the landscape of data analytics. AI-driven automation is not only enhancing efficiency but also redefining the roles within BI teams. Automation code provided by vendors allows for the deployment of entire systems with minimal human intervention, ensuring consistent, audited actions while reducing errors.
- Automated tasks include system administration, monitoring, task reviews and approvals, database management, integration, and systems management.
- AI algorithms are pre-programmed to identify risks, such as fraud, and can automatically deploy patches for network security.
- Machine learning programs record network behavior, aiding in disaster recovery and risk assessment.
The evolution of automation technologies is leading to a shift where dedicated data specialist roles may become less prevalent, as self-service BI tools become more sophisticated and accessible to non-technical users.
The trend towards self-service BI and data democratization is accelerating the adoption of AI in automation. As a result, non-technical employees are increasingly taking on analyst roles, supported by user-friendly BI platforms that incorporate advanced data science tools without the need for extensive expertise.
Measuring the Impact of Automation on BI Deployment
The integration of automation within BI systems has led to a transformative shift in how businesses approach data analysis and decision-making. Automation streamlines repetitive tasks, freeing up data professionals to focus on more strategic initiatives. By automating tasks such as system administration, monitoring, and database management, companies can achieve a higher level of efficiency and accuracy.
Automation's influence extends beyond mere time savings. It also plays a critical role in the scalability and elasticity of cloud computing services, like those provided by Oracle's Autonomous Database, which automatically scales to meet demand. This capability is crucial for businesses that need to adapt quickly to changing market conditions.
To truly understand the impact of automation on BI deployment, it's essential to consider both qualitative and quantitative measures. Here are some key aspects to evaluate:
- The reduction in time required to perform routine data management tasks
- The improvement in data quality and consistency
- The increase in the speed of deploying new BI solutions
- The ability to scale BI systems with minimal manual intervention
The potential generative AI productivity impact in 2023, as indicated by various business functions, underscores the importance of measuring automation's effectiveness in BI deployment.
As automation becomes more prevalent, it's vital to assess its impact comprehensively to ensure that it contributes positively to the organization's overall data strategy.
Citizen Data Scientists: Democratizing Analytics
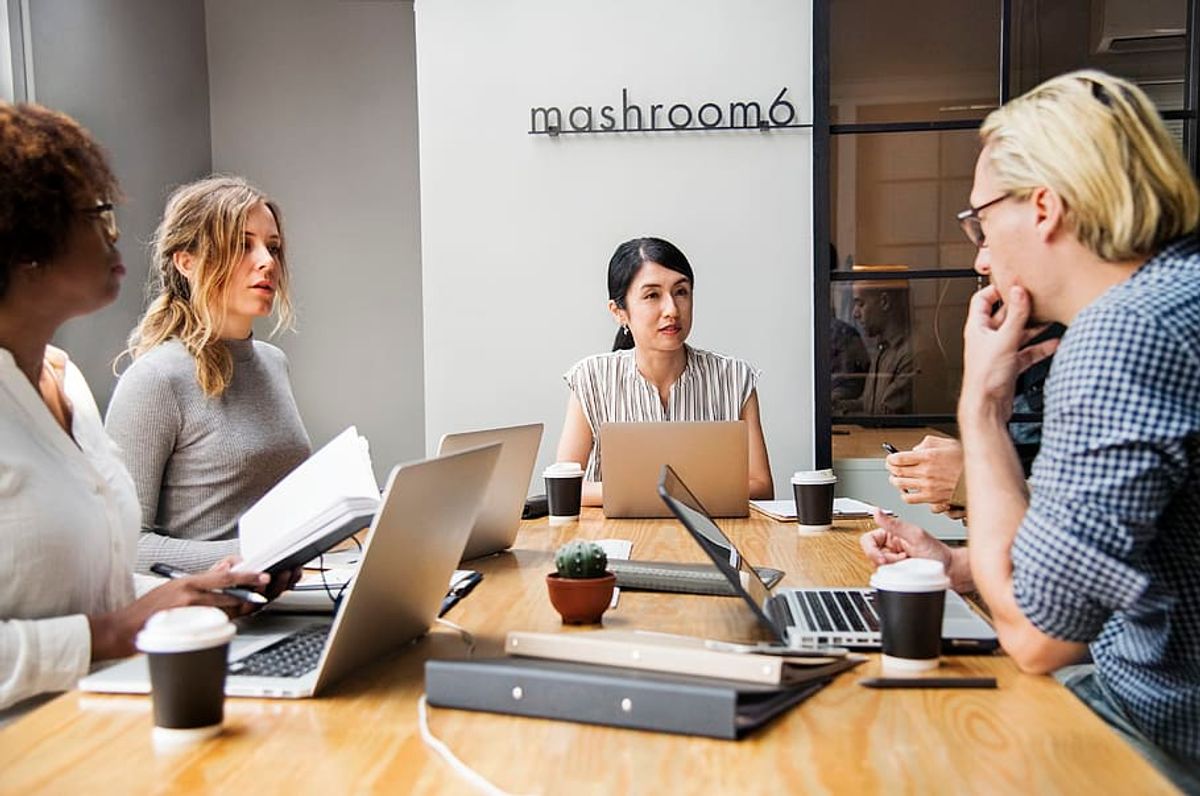
The Emergence of Citizen Developers
The landscape of business intelligence is rapidly evolving, and at the forefront of this transformation is the rise of citizen developers. These individuals, often without formal programming backgrounds, are leveraging user-friendly BI tools to perform complex data analysis and create applications that once required specialized skills. The empowerment of citizen developers is reshaping the BI ecosystem, making data more accessible and actionable for a broader range of users within an organization.
With the advent of intuitive platforms and visual programming environments, the barriers to entry for engaging with BI tools are lower than ever. This democratization of data is not only accelerating decision-making processes but also fostering innovation by enabling a diverse set of minds to contribute to problem-solving.
The trend towards citizen development is indicative of a larger shift in BI: the move towards self-service and automation. As these trends converge, they promise to redefine roles and workflows in the business intelligence domain.
While the rise of citizen developers is a boon for agility and efficiency, it also underscores the need for robust governance and oversight to ensure data integrity and compliance with regulatory standards.
Training and Tools for Citizen Data Scientists
As the role of citizen data scientists becomes more prominent, organizations are investing in training programs and tools tailored to empower these individuals. The goal is to equip them with the necessary skills to perform data analytics without the need for deep technical expertise traditionally associated with data scientists.
Essential tools for citizen data scientists range from intuitive data visualization software to automated machine learning platforms. These tools are designed to be user-friendly, enabling users to derive insights from data with minimal coding knowledge.
- Data visualization tools
- Automated machine learning platforms
- Self-service data preparation software
By providing access to these tools and comprehensive training, businesses are fostering an environment where data-driven decision-making can flourish across all levels of the organization.
Data governance is essential for informed decision-making. Steps include open data access, training, and clear policies. Collaboration tools enhance BI by integrating diverse data sources for competitive advantage.
Balancing Expertise and Accessibility in BI
The evolution of Business Intelligence (BI) tools has ushered in an era where the balance between expertise and accessibility is crucial. Non-technical users are now empowered to perform complex data analyses, thanks to intuitive platforms and automation. However, the sophistication of these tools does not eliminate the need for skilled data professionals; rather, it complements their work by allowing them to focus on more strategic tasks.
- The selection of BI software should be driven by the organization's specific needs, considering factors such as ease of use for non-technical users and the depth of analysis required.
- Training programs and continuous learning opportunities are essential to ensure that all users can effectively leverage the full capabilities of BI tools.
- Establishing a culture of data-driven decision-making involves both the democratization of data and the guidance of experienced analysts.
The demand for self-service BI and data visualization is indicative of a broader trend towards data democratization, enabling faster insights and more agile business strategies.
As the landscape of BI continues to evolve, organizations must navigate the delicate interplay between empowering citizen developers and maintaining a high standard of data literacy. The goal is to foster an environment where data is not only accessible but also utilized effectively to drive business success.
The Internet of Things (IoT): A Data Goldmine
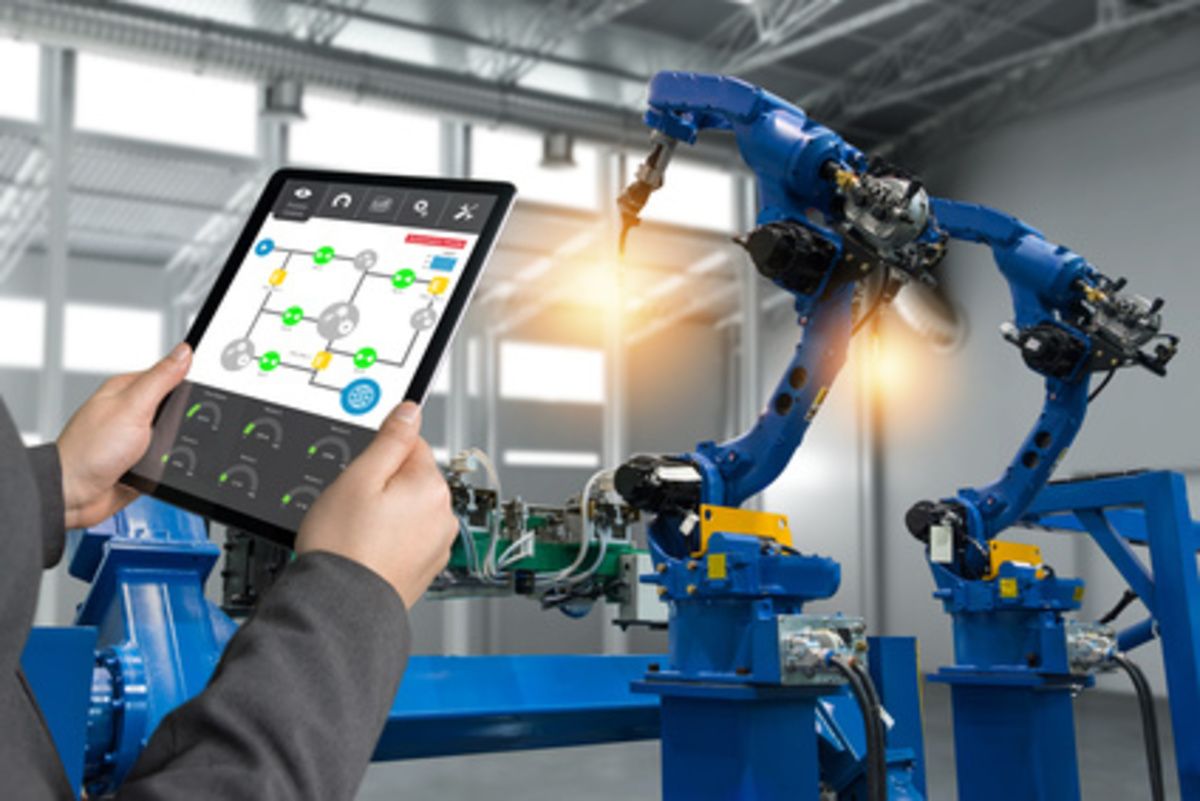
Integrating IoT Data with BI Systems
The integration of the Internet of Things (IoT) with Business Intelligence (BI) systems is a transformative trend that leverages the vast amounts of data generated by connected devices. The seamless flow of IoT data into BI platforms enables real-time analytics and insights, enhancing decision-making processes across various industries.
Security and cost management are pivotal when integrating IoT data, as they directly impact the scalability and sustainability of BI solutions. Software vendors are focusing on making IoT analytics more affordable and securing data at the edge, which are critical considerations for product owners.
- Ensuring data privacy and protection
- Optimizing data storage and processing costs
- Integrating real-time data streams with BI tools
The convergence of IoT and BI is not just about technology; it's about transforming data into actionable intelligence that drives business growth.
Selecting the right software is crucial for effective IoT integration. A data visualization tool is necessary for quick data exploration, while a comprehensive BI tool is essential for scalable reporting and analysis. Predictive analytics tools can further enhance the ability to adapt to market changes, creating a robust IoT-BI ecosystem.
Security Concerns in IoT for BI
As the Internet of Things (IoT) continues to expand, security concerns become increasingly critical for Business Intelligence (BI). The integration of IoT devices into BI systems introduces vulnerabilities that can be exploited by cyber threats. Ensuring the protection of sensitive data collected from a myriad of connected devices is paramount.
- Data Encryption: Vital for safeguarding data in transit and at rest.
- Access Control: Limits device and data access to authorized personnel.
- Regular Security Assessments: Essential for identifying and mitigating potential risks.
- Anonymization Techniques: Protect individual privacy when aggregating IoT data for analysis.
The demand for software that can encrypt and anonymize data for sharing will rise. Staying in silos helps no one, and the only way forward is to move ahead with guardrails and consistent security assessment.
Vendors and product owners must prioritize making IoT analytics affordable while securing data at the edge. This dual focus on cost-effectiveness and robust security measures will be a defining trend in the evolution of IoT for BI.
Cost Management for IoT in Business Intelligence
As the Internet of Things (IoT) continues to expand, businesses are increasingly integrating IoT data into their BI systems. Managing the costs associated with this integration is crucial for maintaining profitability. To achieve cost-effective IoT analytics, companies are exploring various strategies:
- Optimizing data streaming to ensure only relevant data is transmitted and stored, reducing unnecessary expenses.
- Implementing in-database analysis to minimize data movement and associated costs.
- Utilizing usage reports to identify peak consumption times, allowing for strategic scaling of resources.
- Restricting data access based on usage quotas or roles to control query volumes and costs.
The focus on making IoT analytics affordable and securing data at the edge is a testament to the industry's commitment to innovation and efficiency.
With IoT 2023 in review, it's evident that the developments in IoT have been significant, impacting both the cost structures and security considerations for BI. As businesses navigate the complexities of IoT integration, the emphasis on cost management will remain a top priority.
Decision Intelligence: Enhancing Business Decisions
Augmenting Decision-Making with Data Science
In the realm of business intelligence, decision intelligence represents a transformative approach that merges the analytical power of data science with the nuanced understanding of human decision-making processes. By integrating elements from psychology, neuroscience, and economics, decision intelligence enhances the quality and speed of business decisions.
Decision intelligence closes the gap between insights and decisions, effectively shortening the decision cycle and enabling more agile responses to market changes.
One of the key steps in leveraging decision intelligence is to establish clear decision pathways tailored to an organization's specific needs. This involves:
- Defining the decision-making framework.
- Mapping out the decision pathways.
- Linking relevant datasets to these pathways.
With these steps, businesses can harness predictive analytics and machine learning to generate actionable insights, ensuring that decisions are not only data-driven but also contextually informed. As this technology continues to evolve, we can anticipate a surge in its adoption, with leading vendors and developers focusing on enhancing its capabilities.
Case Studies in Decision Intelligence
The application of decision intelligence across various industries showcases its potential to revolutionize decision-making processes. Retail, healthcare, and fintech are notable sectors where decision intelligence has been instrumental in enhancing strategic outcomes. By integrating psychology, economics, and data science, businesses are able to make more informed and timely decisions.
Decision intelligence effectively closes the gap between insights and action, transforming how organizations approach complex decision-making scenarios.
For instance, a retail company may use decision intelligence to optimize inventory levels, predict consumer trends, and personalize marketing strategies. In healthcare, it can improve patient outcomes by predicting disease patterns and optimizing resource allocation. Fintech companies leverage it to detect fraudulent activities and tailor financial products to customer needs.
Here's a brief overview of decision intelligence applications in different sectors:
- Retail: Personalized marketing, inventory optimization
- Healthcare: Disease pattern prediction, resource management
- Fintech: Fraud detection, customized financial services
While the technology is still in its early stages, the following case studies exemplify the practical benefits and the growing interest from leading vendors and developers in the field.
Future Directions for Decision Intelligence in BI
As we look towards the horizon of business intelligence, decision intelligence stands out as a transformative force. By fusing decision science with data science, it promises to close the gap between insights and action, making the decision cycle more efficient and effective.
Decision intelligence is still in its early stages, with tech giants like Google and Alibaba pioneering dedicated labs and platforms. The integration of AI, machine learning, and social sciences is key to its evolution, offering a glimpse into a future where business decisions are significantly enhanced by technology.
The potential of decision intelligence lies in its ability to provide a decision map tailored to an organization's unique pathways, thereby optimizing outcomes.
Looking forward, we can anticipate a surge in software capabilities, with low-code platforms enabling even citizen developers to engage with decision intelligence tools. The trajectory is clear: as the technology matures, it will become an indispensable component of the BI ecosystem, reshaping how organizations approach and execute their decision-making processes.
In the fast-paced world of business, making informed decisions is crucial for success. At OptimizDBA, we specialize in Decision Intelligence, providing you with the tools and expertise to enhance your business decisions. Our proprietary methodologies and unparalleled experience in database optimization ensure that your transactions are not just faster, but potentially 1000 times more efficient. Don't let slow data processing hold you back. Visit our website to learn how we can transform your data solutions and propel your business forward. Let's optimize your potential together!
Conclusion
As we navigate through the evolving landscape of Business Intelligence (BI), the trends of 2023 offer a glimpse into a future where data is more accessible, decisions are more informed, and technology is more integrated into our daily business operations. Generative AI is set to democratize data analysis, enabling more individuals to engage with complex data without specialized skills. Cloud computing continues to revolutionize cost structures, while data sharing and monetization break down silos, fostering a more collaborative environment. The rise of the data mesh and the unwavering importance of data governance and security highlight the industry's commitment to managing and protecting data assets. Automation and the emergence of citizen data scientists are reshaping the workforce, and IoT remains a critical area for improvement. As these trends shape the BI landscape, businesses must stay agile and informed to leverage these advancements for competitive advantage. The future of BI is bright, and by embracing these trends, organizations can unlock new levels of efficiency, innovation, and growth.
Frequently Asked Questions
How is Generative AI shaping the future of Business Intelligence?
Generative AI is bridging the skills gap by enabling less technical users to generate complex data models and visualizations, democratizing data access and empowering a wider range of employees to make data-driven decisions.
What role does cloud computing play in Business Intelligence?
Cloud computing is essential for BI, offering cost-effective data storage and processing, seamless integration with BI tools, and specialized cloud services that enhance BI infrastructure and capabilities.
What is the significance of data sharing and monetization in BI?
Data sharing breaks down silos and fosters collaboration, while data monetization creates new revenue streams. Both trends are unlocking new value and transforming how businesses leverage their data assets.
Can you explain the concept of Data Mesh in BI?
Data Mesh is a decentralized approach to data management that emphasizes domain-oriented ownership and a self-serve data infrastructure, enabling organizations to manage data at scale more effectively.
Why is data governance and security a priority in BI?
With the increasing volume and sensitivity of data, robust data governance and security are critical to ensure compliance with evolving privacy regulations and to protect against emerging data threats.
How does automation impact Business Intelligence deployment?
Automation streamlines BI processes, from data pipelines to workflows, enabling faster deployment and scalable systems that can adapt to changing business needs with minimal human intervention.
Who are Citizen Data Scientists and what is their impact on BI?
Citizen Data Scientists are non-expert users who utilize BI tools to perform analytical tasks. They are democratizing analytics, though there remains a need for specialized data skills.
What challenges and opportunities does IoT present for BI?
IoT offers a wealth of data for BI, but it also raises concerns around data security and cost management. Addressing these challenges is crucial for businesses to fully capitalize on IoT's potential.