Real-Time Analytics Revolution
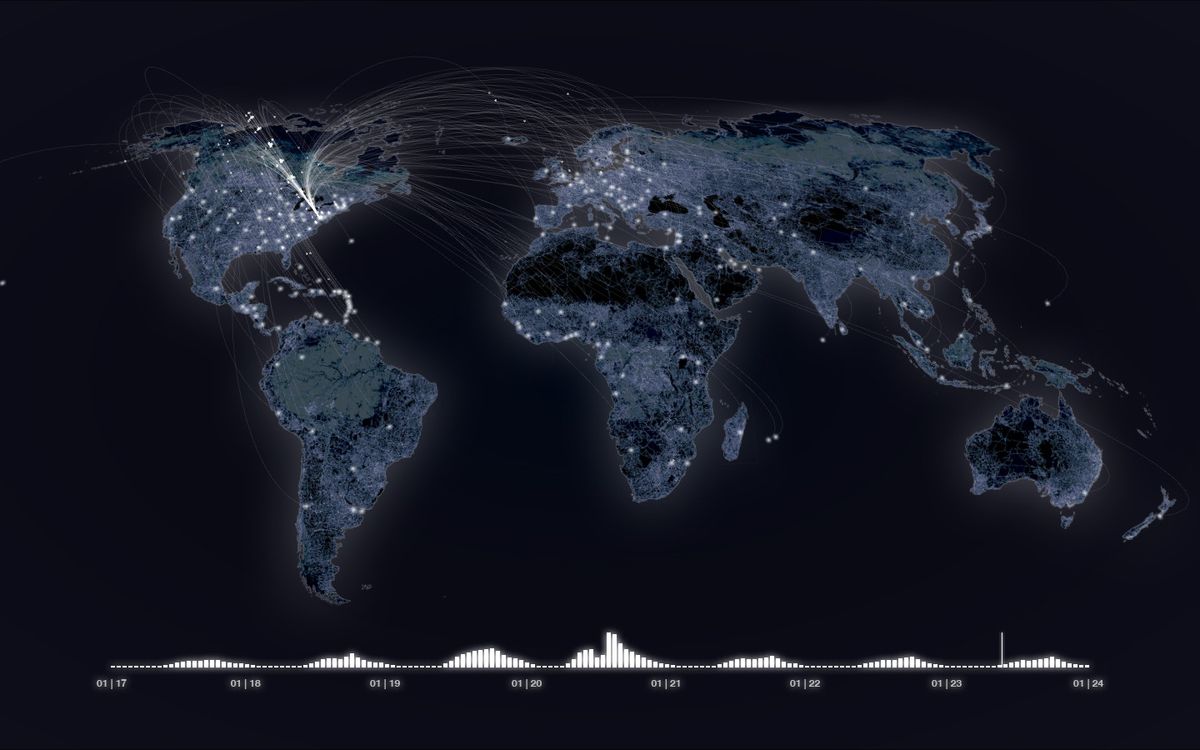
The Shift Towards Instant Insights
The corporate world is increasingly recognizing the value of real-time analytics. The ability to generate insights instantly is not just a convenience; it's becoming a critical component of competitive strategy. With tools that allow for natural language search and live-query capabilities, businesses can now connect to their data clouds or lakes in mere seconds, transforming the way they interact with data.
Instant insights enable organizations to respond to market changes with unprecedented speed. Here's how the process typically unfolds:
- Search: Use natural language to create insights in seconds.
- Connect: Live-query your data sources quickly and efficiently.
- Model: Build and refine data models to suit specific business needs.
- Visualize: Monitor business performance continuously with dynamic dashboards.
- Operationalize: Integrate insights into business workflows for immediate action.
The shift towards instant insights is not just about speed; it's about embedding analytics into the fabric of decision-making processes, ensuring that every business move is backed by data-driven intelligence.
Impact on Decision-Making Processes
The advent of real-time analytics has significantly altered the landscape of corporate decision-making. Real-time data analysis enables quick decision-making through in-memory databases and predictive analytics integration, setting new standards for data-driven decision-making in 2024. This immediacy allows businesses to respond to market changes with unprecedented speed, offering a competitive edge that was previously unattainable.
The integration of prescriptive analytics into real-time systems has been transformative, providing immediate recommendations that facilitate timely and effective responses to dynamic conditions.
Real-time decision-making is not just about speed; it's also about the precision and relevance of the insights provided. Advanced machine learning algorithms and optimization techniques are employed to sift through vast amounts of data, ensuring that the recommendations are both timely and contextually appropriate. The following points highlight the impact of real-time analytics on decision-making processes:
- Enhanced ability to predict and respond to market trends
- Improved operational efficiency through immediate insights
- Reduction in decision latency, leading to faster action
While the benefits are clear, organizations must navigate the challenges that come with the integration of these advanced systems. Ensuring data accuracy, managing the complexity of algorithms, and maintaining the privacy and security of information are critical considerations in the era of real-time analytics.
Challenges and Opportunities
The advent of real-time analytics has brought forth a plethora of opportunities for businesses to harness instant insights for strategic advantage. However, this transformation is not without its challenges. The integration of augmented analytics and the evolution towards Predictive Analytics 2.0 demand a robust infrastructure capable of handling vast data streams efficiently.
- Ensuring data accuracy and integrity
- Developing high-performance architectures
- Adhering to best practices in testing and monitoring
- Leveraging edge computing to minimize latency
The potential for innovation is boundless, yet the journey is complex. Companies must navigate these challenges with agility and foresight to capitalize on the opportunities presented by these analytical advancements.
The role of SQL remains critical in managing and querying data, emphasizing the need for continuous skill development in this area. As industries transform, staying ahead requires not just adopting new technologies but also fortifying existing systems and practices.
The Rise of Augmented Analytics
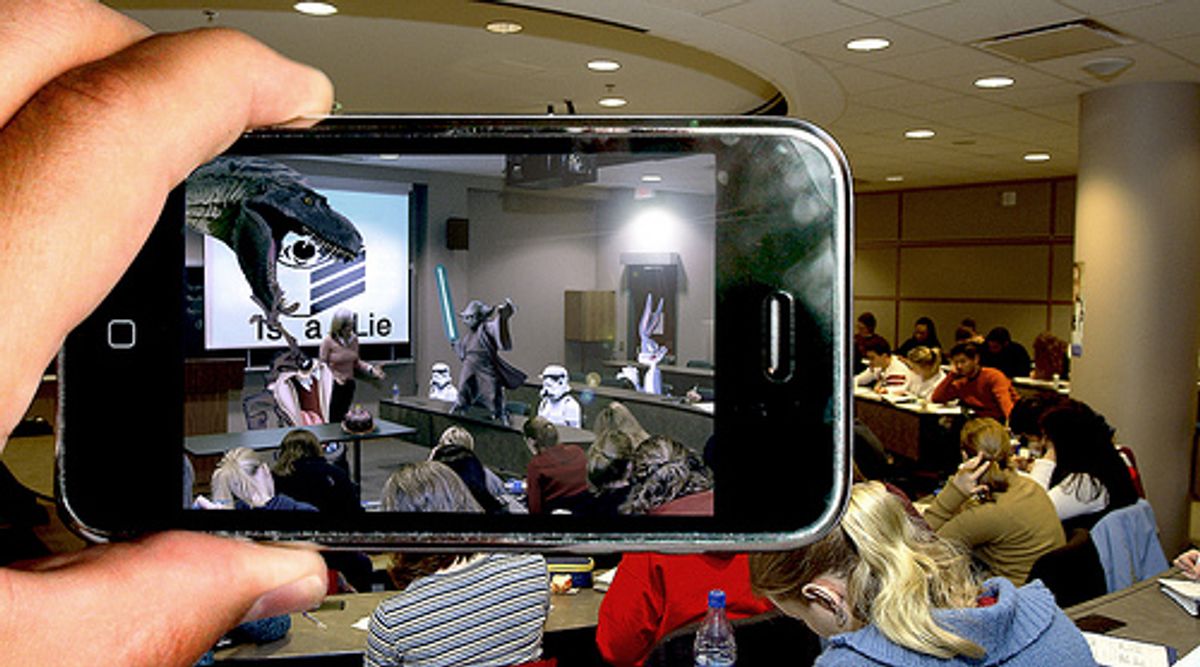
Integrating AI and ML in Data Analysis
The integration of Artificial Intelligence (AI) and Machine Learning (ML) is transforming the landscape of data analytics. Organizations are now able to uncover hidden patterns and forecast future trends with unprecedented precision. By harnessing the power of AI and ML, businesses are not only improving their predictive analytics but also automating complex decision-making processes.
Augmented analytics is redefining the role of data analysts by automating data preparation, analysis, and visualization. This shift is enabling both technical and non-technical users to focus on higher-value tasks, ensuring that actionable insights are derived with greater accuracy and efficiency.
The advent of generative AI is poised to further revolutionize business analytics, offering capabilities that extend beyond predictive and sentiment analysis to include trend detection and automated insight generation.
The following list highlights the key benefits of integrating AI and ML into data analysis:
- Enhanced predictive analytics capabilities
- Automation of routine data analysis tasks
- Increased efficiency in uncovering insights
- Improved accuracy in data-driven decision making
- Generation of actionable insights for strategic planning
Enhancing Data Literacy Across Organizations
In the corporate world, enhancing data literacy is a pivotal step towards harnessing the full potential of analytics. As organizations strive to become more data-driven, the ability to understand, interpret, and communicate data effectively becomes essential for all employees, not just data specialists.
By fostering a culture of data literacy, companies empower their workforce to make better decisions, innovate, and maintain a competitive edge.
To achieve this, a multi-faceted approach is often adopted, including:
- Emphasizing data quality through rigorous governance policies.
- Tailoring business intelligence (BI) reports to the audience's needs.
- Simplifying data presentation with effective visualizations to avoid complexity.
Moreover, the trend of data democratization is reshaping the landscape. Self-service analytics platforms and intuitive visualization tools are now accessible to a wider range of employees, promoting independent exploration and insight generation. This shift not only democratizes data but also embeds a data-driven ethos across the organizational fabric.
Future of Automated Insights
The future of automated insights is intertwined with the evolution of generative AI, which is poised to significantly enhance data democratization. Generative AI will drive the creation of sophisticated models that can process and analyze data with unprecedented efficiency, leading to more informed decision-making across various business sectors.
As cloud computing costs continue to influence the analytics landscape, organizations are seeking ways to optimize their data strategies. The integration of AI and ML in analytics is not just a trend but a necessity, enabling businesses to unlock deeper insights and predictive capabilities from their complex datasets.
The convergence of AI, ML, and analytics heralds a new era where automated insights become a staple in strategic business operations, ensuring that data-driven decision-making is more accessible, accurate, and actionable.
Data Fabric and Integration
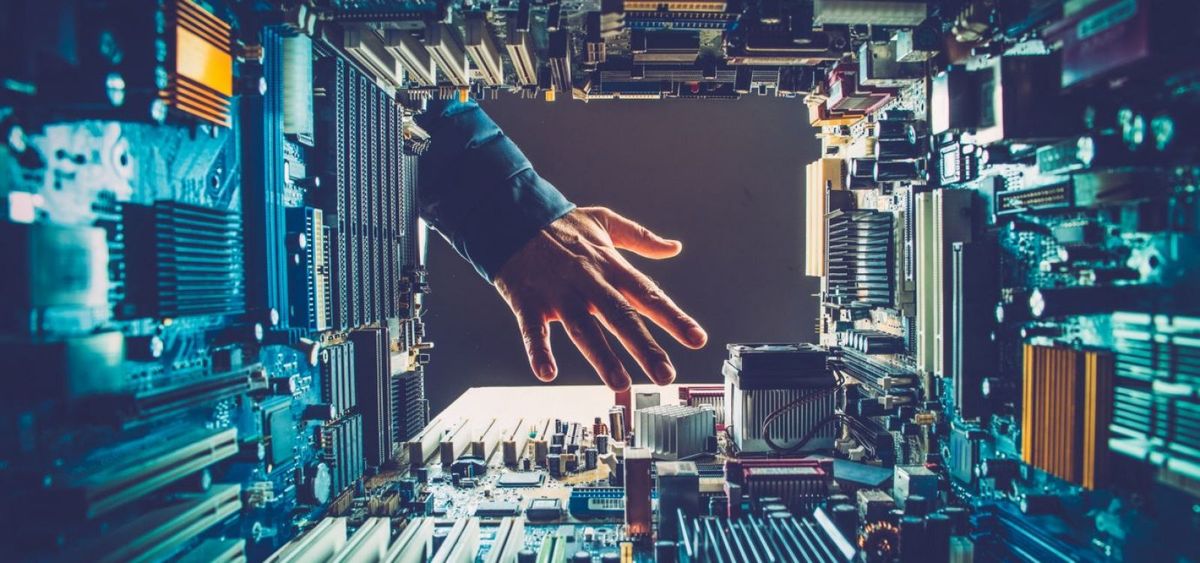
Streamlining Data Accessibility
In the rapidly evolving landscape of corporate analytics, streamlining data accessibility has become a cornerstone for businesses aiming to leverage data for strategic advantage. By simplifying the process of data access, organizations can ensure that valuable insights are readily available to decision-makers at all levels.
- Emphasize Data Quality: Rigorous data governance policies are crucial to maintain the accuracy and integrity of data.
- Know Your Audience: It's essential to tailor data presentations to the specific needs of the audience.
- Simplify and Clarify: Effective visualizations are key to presenting data in an intuitive and straightforward manner.
The democratization of data is not just a trend; it's a strategic imperative that frees up time and effort for both technical and non-technical users, allowing them to focus on higher-value tasks and make informed decisions. This approach aligns with the broader analytics trends that include the democratization of data, NLP, DaaS, and AI integration, with a future focus on advanced analytics, AI, ML, and predictive modeling for strategic decision-making.
Interoperability Between Diverse Data Systems
In the corporate world, interoperability is the linchpin that holds together the diverse systems within a data fabric architecture. It's not just about having a variety of data sources; it's about these sources communicating effectively to provide a cohesive data ecosystem. Seamless interoperability ensures that data flows freely between systems, regardless of their origin or format, enabling businesses to harness the full potential of their data assets.
Data fabric architectures are designed to support this level of integration, offering a flexible and scalable solution for managing complex data landscapes. With interoperability at its core, data fabric allows for:
- Consistent data quality and lineage across platforms
- Enhanced data security and governance
- Simplified data management and retrieval processes
By fostering an environment where data can be easily accessed and shared, organizations are better equipped to respond to market changes and make informed decisions. The strategic importance of interoperability cannot be overstated, as it directly impacts the agility and competitiveness of a business in today's data-driven world.
Building a Resilient Data Infrastructure
In the corporate world, the goal of a resilient data infrastructure is to minimize downtime and maintain business continuity. This involves protecting data integrity and ensuring that critical operations can persist under various conditions. A key component in achieving this resilience is the implementation of a data fabric architecture, which supports data governance activities to ensure data quality, lineage, and security across the entire landscape.
Ensuring data quality is foundational for successful analytics. Regular data validation and cleansing are crucial, and should be integrated into analytics tools and processes. As businesses continue to generate and rely on vast volumes of data, the importance of a resilient infrastructure becomes increasingly clear.
By creating a unified, integrated view of data without silos, data fabric simplifies the retrieval and analysis process, enabling seamless data access and processing across diverse sources and locations.
The future of data analytics hinges on the ability to control and secure data, reducing the risk of unauthorized use or misuse. As such, the data fabric approach will become more prevalent in the business analytics (BA) landscape, offering a competitive advantage in data governance.
Embedded Analytics in Business Operations
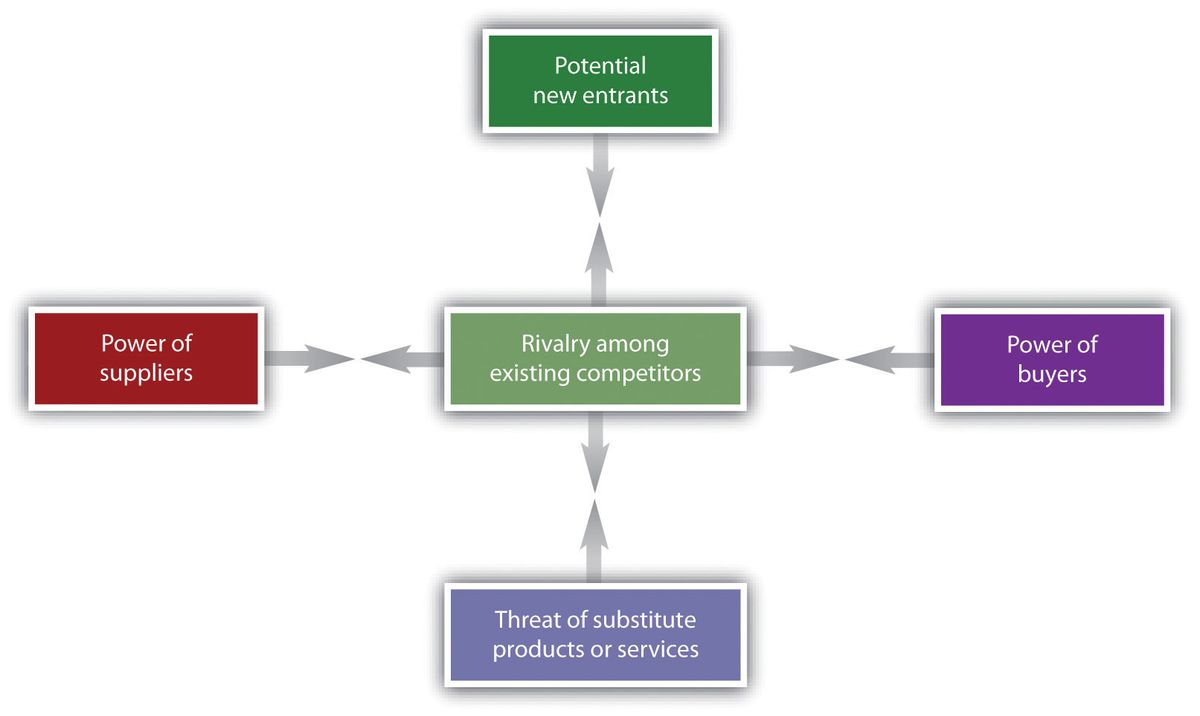
Seamless Integration with Applications
In the fast-paced corporate environment, seamless integration of analytics with everyday business applications is not just a convenience; it's a necessity. By embedding analytics directly into the tools employees use daily, companies can ensure that data-driven insights are readily available at the point of decision. This integration allows for a more intuitive and efficient workflow, where analytics become a natural part of the business process.
The goal is to create an ecosystem where analytics and operations are so intertwined that they become indistinguishable from each other.
For instance, CRM systems can now be enhanced with analytics to track sales performance, customer interactions, and pipeline progress. Here's a snapshot of how analytics can be integrated with various applications:
- Google Suite: Centralize email and calendar activities with analytics for better scheduling and communication insights.
- Microsoft 365: Access key analytics features directly within your email client.
- Slack: Receive real-time notifications on new leads and pipeline progress, leveraging AI-powered analytics for business agility.
- Zapier: Connect analytics with over 5,000 apps for comprehensive data insights and automation.
These integrations are crucial for businesses aiming to stay agile and maintain a competitive edge. As the landscape evolves, the fusion of analytics with applications will only deepen, leading to more sophisticated and democratized data usage across all levels of an organization.
Driving Operational Efficiency
Embedding analytics into business operations is a transformative strategy that frees up time and effort for both technical and non-technical users. By prioritizing resources for higher-value tasks, organizations can make more informed decisions without constraints. For instance, one company saved over $200k by increasing analyst efficiency and enabling front-line business users to find their own insights.
- Reduce costs by identifying areas where expenses can be minimized without affecting efficiency.
- Maximize marketing effectiveness through targeted audience segmentation, personalized messages, and success measurement.
Operational efficiency through data analytics enhances productivity and reduces costs. Innovation and adaptability are key for competitive edge, leveraging advanced analytics for business transformation.
Data-driven organizations are not only more adept at acquiring and retaining customers but are also significantly more likely to be profitable. The integration of analytics into daily operations is not just a technical upgrade but a fundamental shift towards a more agile and responsive business model.
Custom Analytics for Enhanced User Experience
In the realm of business operations, custom analytics are pivotal in crafting a user experience that is not only engaging but also deeply insightful. By tailoring analytics to the specific needs of users, companies can provide a more intuitive and personalized interface, which in turn fosters greater user satisfaction and loyalty.
Business analytics, with tools like Alttask, empowers companies to identify opportunities, mitigate risks, enhance end-user experience, integrate analytics into strategy, and transform market positioning through advanced analytics. The integration of analytics into applications allows for a seamless user journey, where data-driven insights become a natural part of the user's interaction with the platform.
The true power of custom analytics lies in their ability to adapt to the unique contours of each business, ensuring that every insight serves to enhance the user's interaction with the product.
To effectively implement custom analytics, consider the following steps:
- Evaluate user skill level to determine the appropriate complexity of the analytics tool.
- Leverage platforms like Mode Analytics for their user-friendly interfaces and advanced visualization libraries.
- Utilize Edge Analytics to embed engaging self-service analytics experiences into any application with minimal coding.
By embracing these practices, organizations can ensure that their analytics solutions are not just powerful, but also accessible and relevant to their users.
The Expanding Role of IoT in Analytics
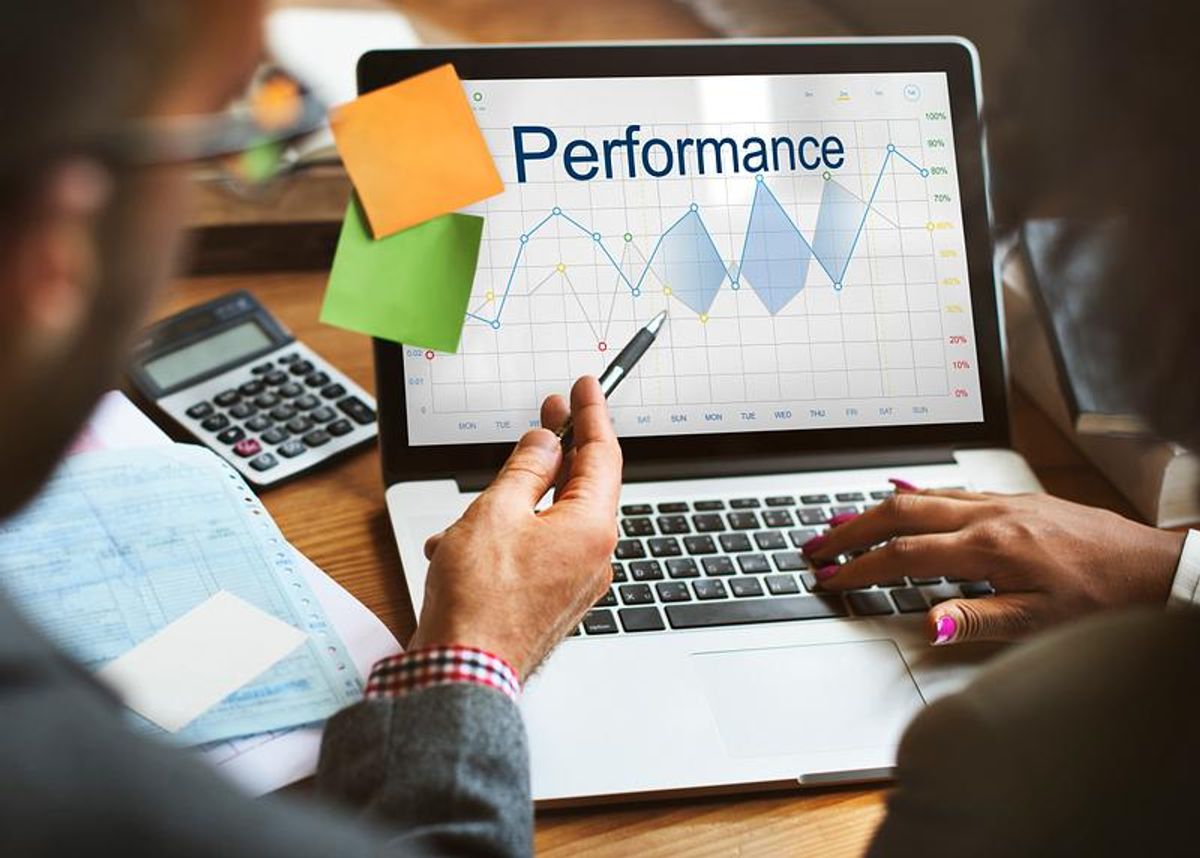
Harnessing IoT Data for Strategic Insights
The proliferation of the Internet of Things (IoT) has ushered in a new era of data collection and analysis. Edge analytics is a key component in this landscape, enabling the processing of data at the source, which is crucial for real-time insights and strategic decision-making. By analyzing IoT data, businesses can identify patterns and trends that inform operational improvements and innovative strategies.
Edge analytics not only conserves bandwidth but also reduces latency, making it an indispensable tool for connected environments like smart cities and autonomous vehicles. The main goal of IoT analytics is to extract valuable insights from the vast streams of data generated by connected devices.
The ability to quickly interpret and act upon IoT data can be a significant competitive advantage.
Here are some of the benefits of harnessing IoT data for strategic insights:
- Real-time operational intelligence
- Enhanced customer experiences
- Predictive maintenance and optimization
- Improved resource management
As the possibilities are limitless, organizations that embrace IoT data analytics position themselves to unlock new insights, drive innovation, and stay ahead in the increasingly data-driven corporate landscape.
Challenges in Managing IoT Data Streams
The proliferation of IoT devices has led to an explosion of data streams that organizations must manage efficiently. Edge analytics is becoming a critical solution, allowing data processing closer to the source and reducing latency. However, this approach presents its own set of challenges:
- Ensuring data integrity and quality across numerous devices.
- Balancing the computational load between edge devices and central systems.
- Addressing the security vulnerabilities inherent in a distributed network.
The sheer volume of IoT data necessitates robust data management strategies to harness its full potential while mitigating risks.
Data privacy and security remain paramount concerns, as the vast amounts of generated data increase the complexity of protecting it in cyberspace. The future of IoT analytics hinges on the ability to navigate these challenges, ensuring secure data storage and sharing practices to maintain data quality and integrity.
IoT Analytics in Predictive Maintenance and Optimization
The integration of IoT analytics into predictive maintenance strategies marks a significant leap forward in operational efficiency. By harnessing the power of IoT data, organizations can anticipate equipment failures and schedule timely maintenance, thereby reducing downtime and extending the lifespan of machinery. Predictive analytics plays a crucial role in this process, utilizing historical data to forecast potential issues before they arise.
Edge analytics is a key component in this evolution, bringing data processing closer to the source and enabling faster, more efficient decision-making. This approach is particularly beneficial in environments where immediate insights are critical and bandwidth is limited.
- Real-time decision-making
- Scenario analysis
- Machine learning and algorithms
The convergence of AI, IoT, and cloud technologies is revolutionizing the way enterprises approach growth and innovation. By integrating these powerful tools, businesses are able to transform vast amounts of data into actionable insights, driving improved efficiency and fostering a culture of continuous improvement.
Privacy and Ethical Considerations in Analytics
Navigating Data Privacy Regulations
In the corporate world, navigating data privacy regulations is becoming increasingly complex. As companies collect and analyze more data, the importance of adhering to stringent privacy laws and standards cannot be overstated. The interplay between innovation and privacy is a delicate balance that requires constant attention.
With the rise of digital technologies, the focus on data privacy and security is more critical than ever. Companies must adopt proactive compliance strategies to protect sensitive information and maintain consumer trust.
Businesses are now prioritizing robust cybersecurity measures and transparent data practices to sustain a positive brand image. This shift is not just about avoiding penalties but also about ensuring the integrity and quality of analytics outcomes. Here are some key considerations for companies:
- Ensuring analytics tools comply with industry-specific data security standards.
- Adopting secure data storage and sharing practices.
- Building trust with consumers through transparent data handling.
The future of analytics is inextricably linked to the ability of organizations to navigate these regulations effectively. As we move forward, the emphasis on data privacy will only intensify, making it a cornerstone of sustainable business operations.
Ethical Use of Predictive Analytics
In the realm of predictive analytics, the ethical implications of forecasting and influencing customer behavior cannot be understated. Organizations must navigate the fine line between personalization and privacy invasion. It's essential to use predictive models responsibly, ensuring that they do not perpetuate biases or infringe on individual rights.
Ethical considerations in predictive analytics include:
- Transparency in how data is collected and used
- Consent from individuals whose data is being analyzed
- Fairness in algorithmic decision-making to avoid discrimination
- Accountability for the outcomes of predictive models
The goal is to foster trust with consumers by being upfront about the use of their data and the measures taken to protect their privacy.
As predictive analytics becomes more intertwined with strategic decision-making, companies must establish clear ethical guidelines. These should be communicated across the organization and adhered to rigorously to maintain the integrity of data-driven initiatives.
Building Trust through Transparent Data Practices
In the age of big data, ethical data practices are crucial for building trust and ensuring transparency. By adopting a proactive approach to compliance with data protection regulations, businesses can safeguard sensitive information and maintain a positive brand image.
Transparency in data transactions not only enhances trust and security but also paves the way for the integration of augmented analytics. This integration promises to automate data preparation and insight generation, democratizing access to analytics capabilities.
The interplay between innovation and the safeguarding of sensitive information is key to staying ahead in an environment where data breaches can have far-reaching consequences.
As we look towards the future, the focus on data privacy and security becomes increasingly important. It impacts data quality and integrity, which in turn affects the outcomes of business analytics. A commitment to secure data storage and sharing practices is essential for raising awareness and building trust within organizations.
Cloud Analytics and Scalability
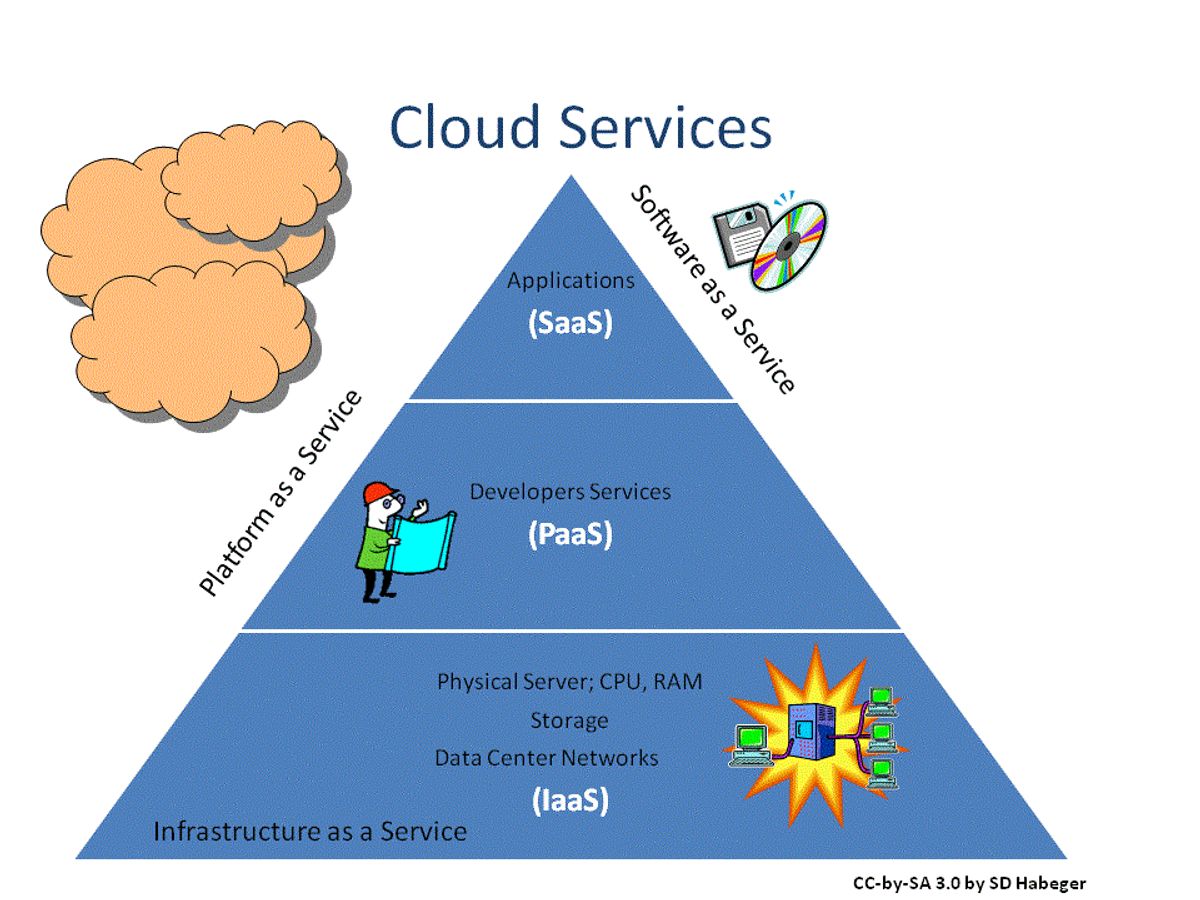
Advantages of Cloud-based Analytics Platforms
The transition to cloud-based analytics platforms has brought forth a paradigm shift in the corporate world. Increased scalability and flexibility are at the forefront, allowing businesses to adjust their data capabilities in line with fluctuating demands. Moreover, the cost-effectiveness of cloud solutions is a game-changer for companies of all sizes, enabling them to leverage advanced analytics without the hefty investment in on-premises infrastructure.
- Cost Savings: Cloud analytics often reduces the need for large upfront investments and lowers ongoing operational costs.
- Speed: Deployment of analytics solutions is faster, providing businesses with the agility to respond to market changes.
- Collaboration: Real-time collaboration and data sharing are enhanced, streamlining decision-making processes.
The ease of access to powerful analytics tools and the ability to process large volumes of data without the need for extensive hardware has democratized data analysis, making it an integral part of business strategy.
The flexibility offered by cloud platforms ensures that businesses can scale their analytics operations up or down as needed, without the constraints of physical infrastructure. This adaptability is crucial in a rapidly evolving market where the ability to pivot and innovate can be the difference between success and failure.
Scalability and Flexibility in Data Processing
In the realm of cloud analytics, scalability and flexibility are paramount. Businesses must evaluate the scalability of their analytics tools, ensuring they can accommodate growing data volumes and complexity. The ability to scale up or down based on business needs is a cornerstone of cloud data management.
Integration with existing systems is another critical aspect. Analytics tools must seamlessly integrate with current software, databases, and CRMs to maintain a smooth workflow. With around 20 Transforms available, users can leverage automated data cleansing and comprehensive integration checks, which are essential for accurate and reliable BI reports.
Understanding your data needs is crucial. Analyze the type, volume, and complexity of your data to select tools that meet your specific requirements. This strategic approach not only ensures data accuracy but also frees up resources, allowing for more informed decision-making.
Security Concerns and Solutions in the Cloud
As organizations increasingly adopt cloud-based analytics, security concerns have become a focal point. The transition to the cloud offers numerous advantages, but it also introduces vulnerabilities that can be exploited by cyber threats. To mitigate these risks, businesses are implementing a range of solutions.
Data encryption, both at rest and in transit, is a fundamental measure to protect sensitive information. Additionally, companies are enforcing stricter access controls and utilizing advanced threat detection systems to promptly identify and respond to potential breaches.
Ensuring robust security in the cloud is not just about technology; it's also about adopting a culture of security awareness and compliance with data protection regulations.
Here are some key steps organizations are taking to secure their cloud analytics platforms:
- Regular security audits and compliance checks
- Employee training on cybersecurity best practices
- Adoption of multi-factor authentication (MFA)
- Deployment of end-to-end encryption solutions
- Utilization of cloud services with built-in security features
By addressing security concerns proactively, companies can leverage the full potential of cloud analytics while maintaining the trust of their stakeholders.
The Convergence of Analytics and Business Strategy
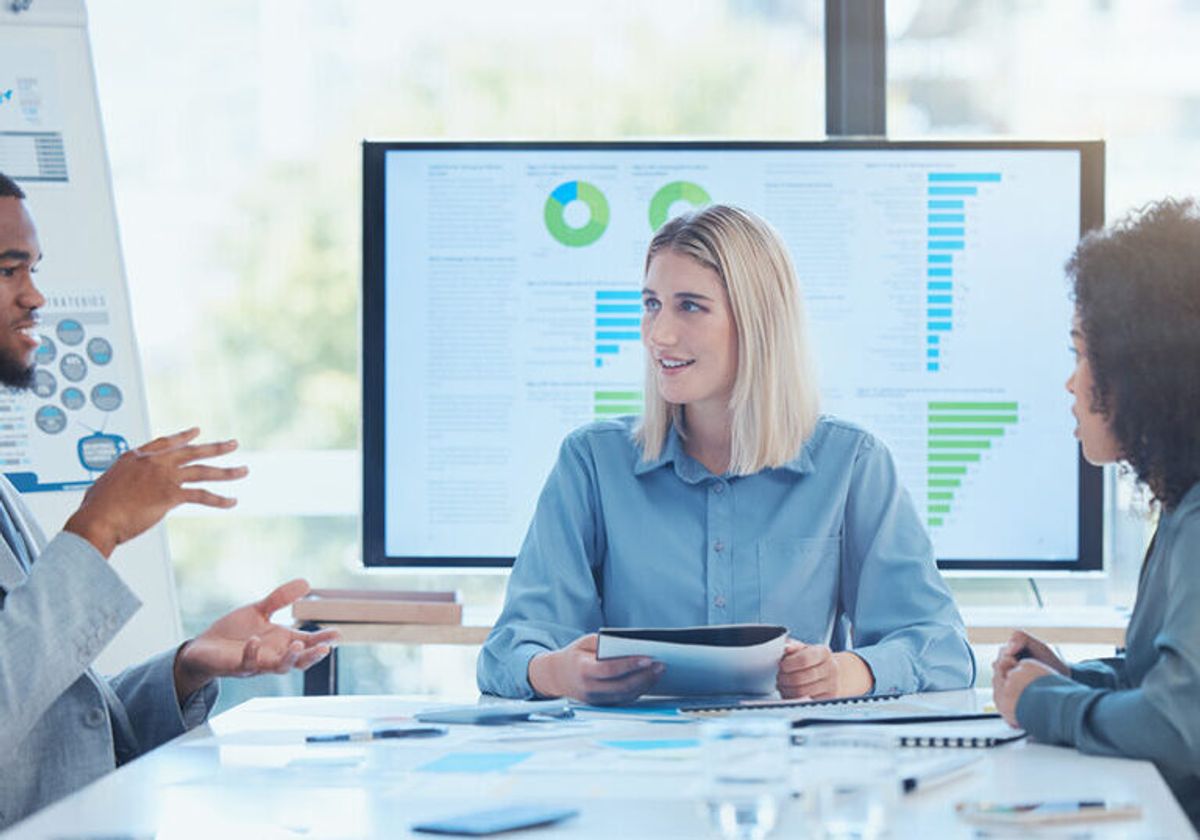
Data-Driven Decision Making
In the era of big data, data-driven decision making has become the cornerstone of competitive advantage. Organizations that harness the power of data analytics are more adept at understanding market dynamics and customer needs. By leveraging data governance, companies can ensure a structured approach to managing data across the organization, which is crucial for informed decision-making.
The impact of data-driven strategies is quantifiable. For instance, data-driven organizations are significantly more likely to acquire and retain customers, and to be profitable. This is supported by findings from the McKinsey Global Institute, which highlight the tangible benefits of a data-centric approach.
- Real-time decision-making enables immediate responses to changing conditions.
- Machine learning and algorithms provide sophisticated analysis for strategic recommendations.
- Scenario analysis evaluates potential outcomes, aiding in the selection of optimal decisions.
Embracing data-driven decision making is not just about adopting new technologies; it's about cultivating a mindset that values data as a critical asset for strategic planning and operational efficiency.
Aligning Analytics with Organizational Goals
In the pursuit of strategic alignment, analytics must be intricately woven into the fabric of an organization's goals. Data analytics is the art and science of examining raw data to extract actionable insights that propel informed decision-making. It's not just about having data; it's about having the right data aligned with the strategic objectives of the business.
Scalability and integration with existing systems are two critical factors that determine the success of analytics within an organization. Scalability ensures that the analytics tools grow with the company, handling increasing volumes of data and complexity. Seamless integration with existing software and systems, such as CRMs and databases, is essential for a smooth workflow and the realization of strategic goals.
By aligning analytics with organizational goals, companies can become data-driven entities that are more likely to acquire and retain customers, ultimately leading to increased profitability and market competitiveness.
To achieve this alignment, consider the following steps:
- Start with leadership commitment to a data-driven culture.
- Select an analytics model that complements the organization's strategic plan.
- Ensure clear communication of goals and the role of analytics in achieving them.
Cultivating a Culture of Analytics
In the corporate world, cultivating a culture of analytics is essential for staying competitive. It's about more than just having access to data; it's about integrating insights into every aspect of the organizational culture. This integration leads to a more informed and agile workplace, where decisions are made on a foundation of empirical evidence rather than intuition alone.
Democratization of data and analytics tools plays a pivotal role in this cultural shift. By equipping employees across all levels with the ability to analyze data, organizations foster a sense of ownership and accountability. Here's how a culture of analytics can manifest within a company:
- Encouraging curiosity and continuous learning about data.
- Providing training and resources for data analysis.
- Recognizing and rewarding data-driven achievements.
- Promoting transparency and sharing of insights across departments.
Success hinges on leveraging analytics and insights into organizational culture to shape the ideal working environment.
As the title suggests, 'Cultivating a Better Workplace Through Data-Driven Insights' is not just a goal but a strategic approach to achieving a robust blend of employee satisfaction and operational excellence. The journey towards a data-centric culture is ongoing, and it requires commitment at all levels of the organization.
Advancements in Predictive Analytics
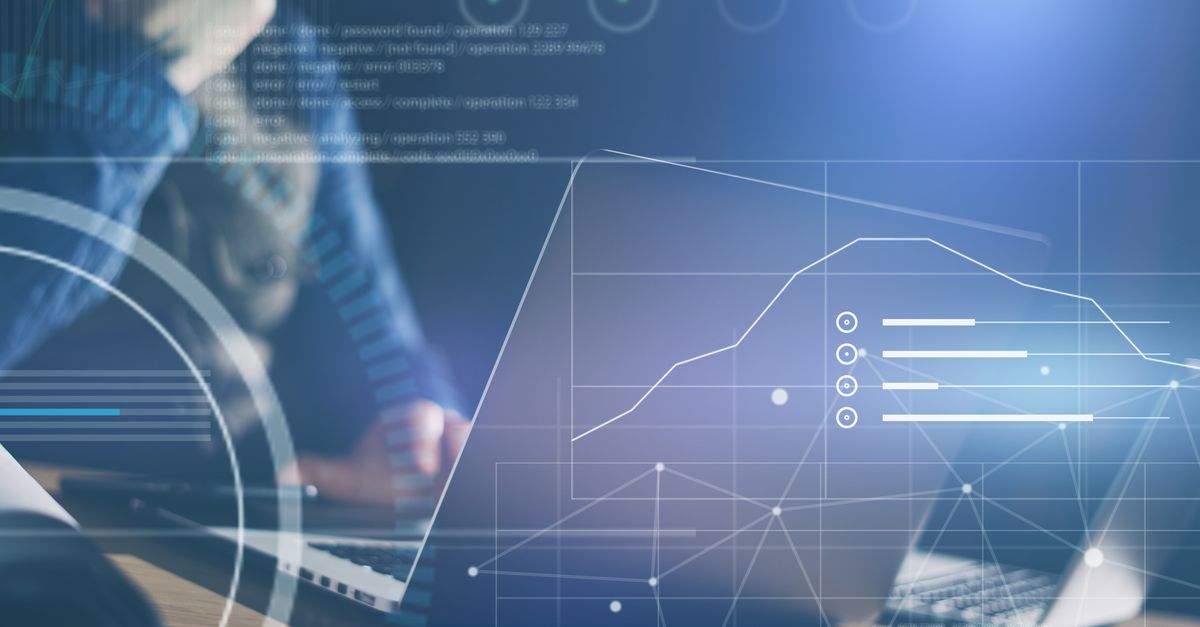
Improving Forecast Accuracy
The pursuit of improving forecast accuracy is a cornerstone in the realm of predictive analytics. By leveraging statistical models and machine learning algorithms, organizations can distill insights from historical data to anticipate future trends and behaviors. This capability is particularly crucial in sales forecasting, where accurate predictions translate directly into optimized resource planning and revenue predictability.
- Statistical models analyze past data to identify patterns.
- Machine learning algorithms refine predictions over time.
- Scenario analysis evaluates potential outcomes.
The integration of advanced machine learning and optimization techniques not only enhances the accuracy of forecasts but also enables a more nuanced understanding of various scenarios. This, in turn, empowers decision-makers to evaluate the consequences of different actions before committing to a strategy.
The key to unlocking the full potential of predictive analytics lies in the continuous refinement of models and algorithms, ensuring that they evolve in tandem with market dynamics and internal variables.
Applications in Risk Management
Predictive analytics has become a cornerstone in the realm of risk management, enabling businesses to anticipate and mitigate potential threats. Predictive analytics in business aids in forecasting trends, identifying risks, and making strategic decisions. It empowers organizations to proactively address challenges and seize opportunities, ensuring a competitive edge in the market.
In industries such as finance and insurance, risk assessment is critical. By leveraging data analytics, companies can identify potential issues and predict future trends. This proactive approach is essential for managing risks effectively. For example, in the services sector, financial data analytics plays a pivotal role in fraud detection by analyzing transaction data to identify unusual patterns or behaviors indicative of fraudulent activity.
The integration of machine learning algorithms and optimization techniques in prescriptive analytics allows for the analysis of data and generation of actionable recommendations. This advanced approach to data handling is instrumental in scenario analysis, evaluating the consequences of different actions to understand potential outcomes before making a decision.
Sales operations also benefit from predictive analytics, with applications such as sales forecasting and Customer Lifetime Value (CLV) analysis informing strategic decisions. These tools help in resource planning and optimizing customer acquisition and retention strategies.
The Future of Scenario Planning
As we look towards the future, scenario planning is evolving into a critical tool for navigating the uncertain waters of the corporate world. It's not just about making predictions; it's about preparing for a range of possible futures. The flexibility and foresight it provides are becoming indispensable for long-term strategic planning.
Scenario planning looks at different future scenarios and considers varied combinations of assumptions and events. These scenarios are not predictions but frameworks for understanding potential developments and their implications.
The role of data storytelling is set to rise, with a focus on translating complex analytical findings into compelling narratives. This skill will be crucial for data scientists and analysts to effectively communicate with stakeholders who may not have a deep understanding of data.
Here are some key aspects that will shape the future of scenario planning:
- Emphasis on data storytelling to bridge the gap between analytics and business strategy.
- Integration of advanced analytics and generative AI to create more nuanced and dynamic scenarios.
- Greater collaboration between data teams and business units to ensure scenarios are aligned with organizational goals.
The future of scenario planning is not just about the tools and technologies; it's about cultivating a mindset that embraces complexity and prepares for multiple outcomes.
Democratization of Data and Analytics Tools
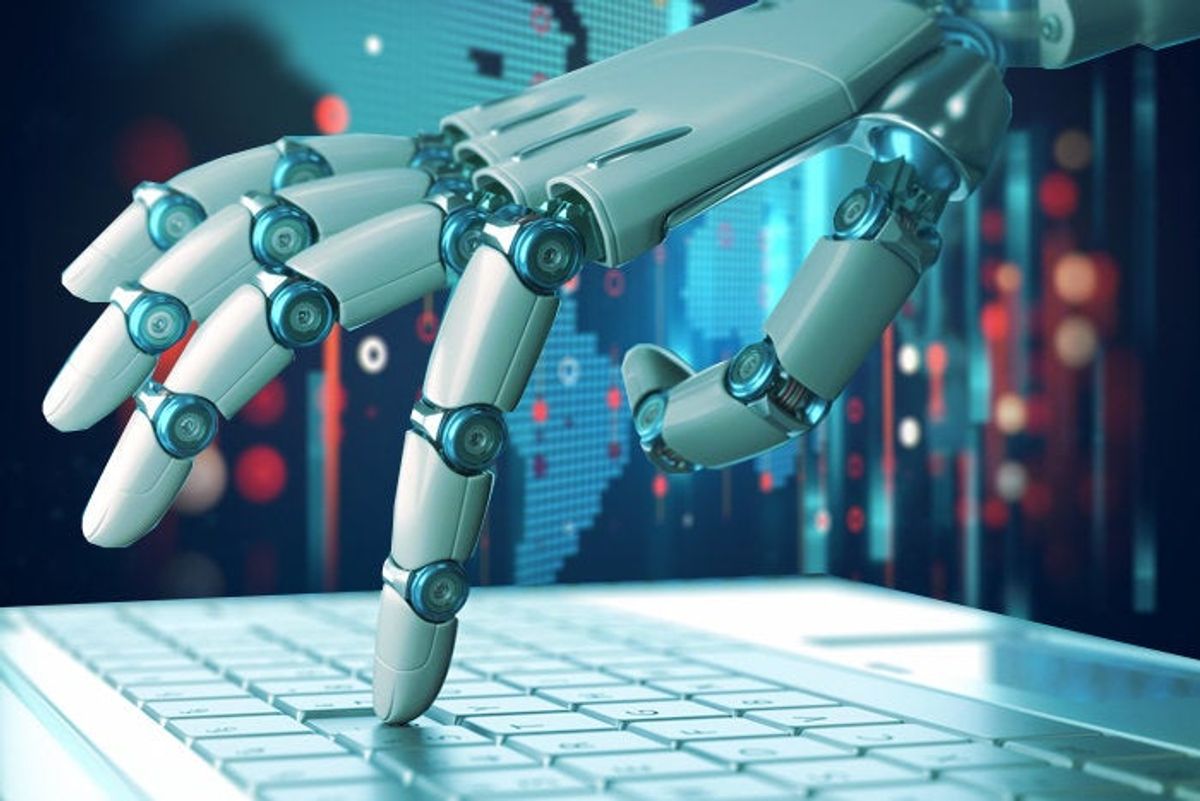
Empowering Non-Technical Users
The democratization of data and analytics tools is a pivotal trend in the corporate world, frees up time and effort for both technical and non-technical users alike. By prioritizing resources for higher-value tasks, organizations are enabling their workforce to make more informed decisions, regardless of their technical background.
- Evaluate user skill level
- User feedback and reviews
These steps are essential in selecting the right analytics tools that cater to various user skillsets, ensuring that even those with minimal technical knowledge can navigate the data landscape effectively. An active community around a tool can serve as a valuable resource for support and shared knowledge, further empowering users.
Admins can manage dashboard access to maintain data integrity, while still providing visibility to all users. This balance of access and control is crucial for adapting analytics tools to every user's needs.
With the integration of features like automated data cleansing and validation processes, analytics tools are becoming more accessible and user-friendly. This structured approach not only ensures the accuracy and reliability of data but also primes it for in-depth analysis by a broader range of users within an organization.
Bridging the Data Skills Gap
The corporate world is increasingly recognizing the importance of data literacy, and as such, is actively seeking ways to bridge the data skills gap. A skills gap analysis is a strategic approach to identify where employees may be lacking and to develop targeted training programs. Enhancing employee performance through such tailored education is crucial for businesses to stay competitive in a data-driven landscape.
To effectively bridge this gap, companies are exploring various avenues:
- Outsourcing to countries with a ready supply of trained data professionals.
- Encouraging the rise of citizen data scientists who, despite not having formal qualifications, can analyze data with the right tools.
- Emphasizing the need for data storytelling skills among data scientists and analysts.
The democratization of data analytics tools is empowering more individuals to contribute to data-driven decision-making, regardless of their technical background.
This approach not only fills the immediate need for data-savvy personnel but also fosters a culture where continuous learning and development are valued, preparing organizations for the future of analytics.
The Impact of Self-Service Analytics
The advent of self-service analytics has significantly altered the landscape of data-driven decision-making within organizations. By enabling employees across various departments to engage with data directly, a more democratic approach to analytics has emerged. This empowerment leads to a culture where informed decisions are the norm, not the exception.
- Self-service tools reduce reliance on IT and data experts.
- They foster greater data literacy among non-technical staff.
- There's an acceleration in the speed of insight generation.
The true value of self-service analytics lies in its ability to make data accessible and actionable for all, regardless of technical expertise.
However, this shift also brings challenges, such as ensuring data quality and governance. Organizations must balance the freedom of self-service with robust oversight to maintain data integrity and security.
In today's fast-paced digital world, the democratization of data and analytics tools is crucial for businesses to stay competitive. At OptimizDBA, we provide faster data solutions and unparalleled database optimization consulting to empower your team with the speed and efficiency they need. Our proprietary methodology guarantees significant performance increases, making us the trusted industry leader since 2001. Don't let data slow you down. Visit our website to learn how we can accelerate your data transactions and optimize your database performance today!
Conclusion
The landscape of corporate analytics is undergoing a profound transformation, driven by the integration of AI, real-time data processing, and the Internet of Things. As we have explored in this article, the future of analytics is not just about handling vast amounts of data but about extracting actionable insights with unprecedented speed and efficiency. The trends we have discussed—ranging from data fabric to embedded analytics—highlight the innovative directions in which the field is moving. For businesses to thrive in this new era, staying abreast of these trends and adapting to the evolving analytics paradigm is crucial. The potential for data to shape business strategies and outcomes is immense, and those who harness this potential will lead the charge into a data-centric future. As we continue to witness the growth and impact of analytics in the corporate world, one thing is clear: the ability to leverage data effectively will be a defining factor in the success of organizations across all industries.
Frequently Asked Questions
What is driving the Real-Time Analytics Revolution in the corporate world?
The need for instant insights and the ability to make timely decisions in today's fast-paced business environment are driving the Real-Time Analytics Revolution. As data is generated, real-time analytics processes and analyzes it, enabling businesses to act immediately on customer interactions, market trends, and anomalies.
How is Augmented Analytics transforming data analysis?
Augmented Analytics is transforming data analysis by integrating Artificial Intelligence (AI) and Machine Learning (ML) to automate data preparation, insight generation, and sharing. This enables organizations to enhance data literacy and provide actionable insights more efficiently.
Why is Data Fabric important for future analytics?
Data Fabric is important for future analytics as it streamlines data accessibility and ensures interoperability between diverse data systems. This creates a resilient data infrastructure that supports advanced analytics and data-driven decision-making.
What benefits do Embedded Analytics provide to business operations?
Embedded Analytics provides benefits such as seamless integration with applications, driving operational efficiency, and offering custom analytics for an enhanced user experience. This integration allows organizations to make data-driven insights part of their daily operations.
What role does IoT play in the future of analytics?
IoT plays a significant role in the future of analytics by providing vast amounts of data that can be harnessed for strategic insights. It poses challenges in managing data streams but offers opportunities in predictive maintenance and optimization.
How are privacy and ethical considerations impacting analytics?
Privacy and ethical considerations are increasingly important in analytics as organizations must navigate data privacy regulations and ensure the ethical use of predictive analytics. Building trust through transparent data practices is essential for maintaining consumer confidence and compliance.
What advantages do Cloud Analytics offer to corporations?
Cloud Analytics offers advantages such as cost-efficiency, scalability, and flexibility in data processing. While security concerns exist, solutions are being developed to ensure that cloud platforms can safely handle sensitive data.
How is the convergence of analytics and business strategy influencing organizations?
The convergence of analytics and business strategy is influencing organizations by promoting data-driven decision-making. Aligning analytics with organizational goals and cultivating a culture of analytics ensures that data insights support strategic initiatives and competitive advantage.