Leveraging Advanced Analytics for Actionable Insights
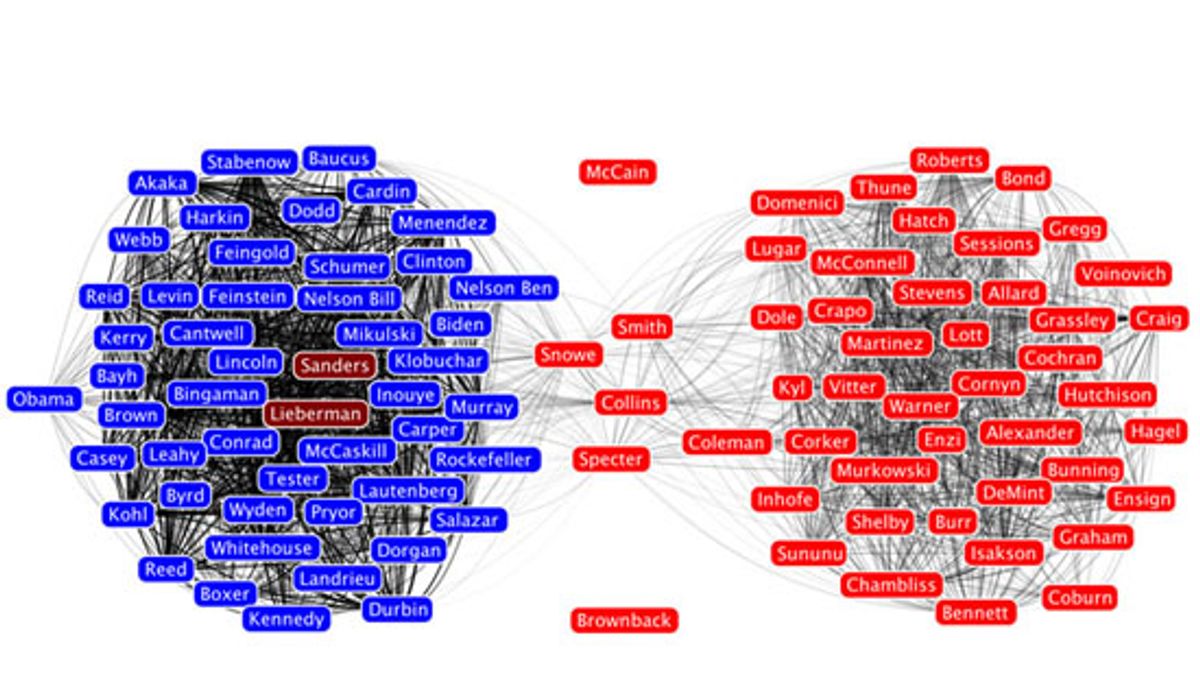
Understanding the Role of Advanced Analytics
The transformative power of advanced analytics lies in its ability to convert raw data into actual marketing performance reporting. For data analysts, these insights are the currency with which they deliver value, driving data-driven recommendations that enhance campaign performance.
Advanced analytics tools enable healthcare providers to uncover patterns, trends, and correlations within vast datasets. This leads to optimized workflows, resource allocation, and overall operational processes, streamlining day-to-day operations and contributing to a more cost-effective delivery of healthcare services.
Healthcare analytics represents a powerful approach to leveraging data within the intricate landscape of the health care sector. This specialized field employs advanced analytical techniques to sift through vast amounts of historical and real-time data, extracting valuable insights that can drive informed decision-making.
Furthermore, contextual intelligence is essential for addressing the limitations of traditional data analytics approaches. While traditional analytics tools excel at processing structured data and performing predefined queries, they often struggle with unstructured data and complex relationships. Contextual intelligence bridges this gap, incorporating advanced techniques to enhance data interpretation.
From Data to Decisions: The Analytics Process
The journey from raw data to strategic decision-making is both complex and critical. Analytics integrates data-driven insights into decision-making, enabling strategic choices, optimized resource allocation, and real-time responses for competitive advantage. Transforming data into actionable insights drives smarter and wiser decision-making.
The process begins with selecting an appropriate analysis method based on organizational goals. Data collection follows, often involving tagging events and features or gathering user feedback. With the data in hand, analysts employ tools like data visualization and AI to interpret both quantitative and qualitative aspects, revealing underlying patterns and opportunities.
The real value lies in the orchestration and interpretation of data to extract qualitative insights that inform strategic actions.
Once insights are identified, they must be prioritized in alignment with organizational objectives. Implementing solutions is an iterative process, with gradual releases and continuous refinement to ensure effectiveness and alignment with evolving goals.
Efficient Decision-Making is at the heart of this process, as it empowers organizations to act swiftly and with confidence, backed by the solid foundation of data-driven evidence.
Case Studies: Real-World Impact of Analytics
The real-world impact of analytics is best exemplified through case studies that demonstrate the practical application and outcomes of data-driven decision-making. One notable case in the healthcare sector involved the use of analytics to streamline preventative care, resulting in improved patient outcomes and increased health system efficiency.
Preventative care initiatives, powered by analytics, have shown a significant reduction in hospital readmissions and a better understanding of patient risk factors. This not only enhances the quality of care but also optimizes resource allocation within healthcare facilities.
The application of data analytics in healthcare is a testament to its potential to revolutionize the industry, leading to better patient care and more efficient operations.
The following table summarizes the impact of analytics on key healthcare metrics:
Metric | Before Analytics | After Analytics |
---|---|---|
Hospital Readmissions | High | Reduced |
Patient Risk Identification | Limited | Enhanced |
Operational Efficiency | Average | Improved |
These case studies underscore the transformative effect of analytics, moving beyond theoretical benefits to deliver tangible improvements in healthcare delivery.
Scalability and Flexibility in Data Analytics
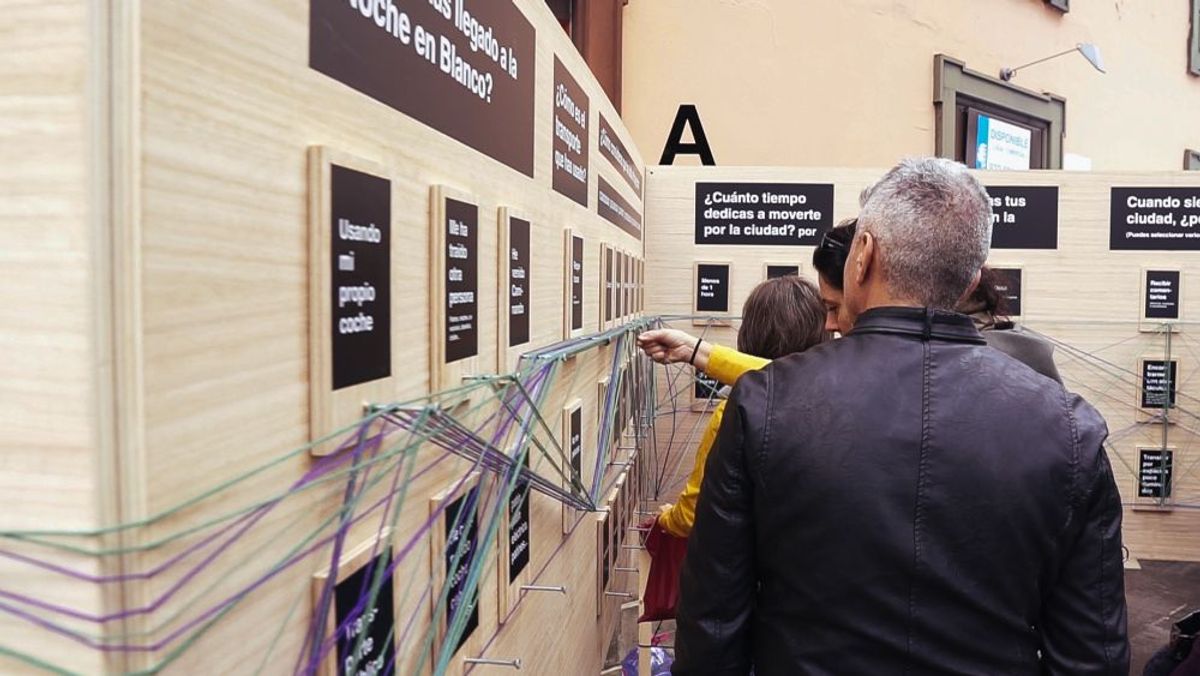
Designing for Scale: Strategies and Challenges
In the realm of data analytics, scalability is a cornerstone for success. As agencies and businesses expand, the volume and complexity of data they handle inevitably increases. A strategic approach to scalability ensures that the infrastructure and tools not only keep pace with this growth but also remain flexible enough to adapt to new demands.
To design for scale effectively, consider the following points:
- Functionality: Ensure the analytics tools support your strategic goals.
- Scalability: Select systems that can accommodate future growth.
- Pricing: Evaluate both current and future affordability.
- Complexity and learning curve: Opt for solutions that balance power with usability.
- Support: Assess the quality of customer service and onboarding resources.
Embracing an adaptive marketing data strategy is crucial. It must be deeply rooted in the agency's DNA, allowing for continuous refinement and optimization.
Iterative processes are key to scalability. By rolling out changes incrementally and testing continuously, agencies can reduce risk and fine-tune their analytics platforms to deliver more precise insights. This approach aligns with the broader organizational goals, ensuring that the data strategy remains relevant and effective over time.
Ensuring Flexibility in Analytic Approaches
In the dynamic landscape of data analytics, ensuring flexibility in analytic approaches is paramount. A scalable and flexible strategy is not just about expanding capacity; it's about adapting to new types of data, evolving business needs, and emerging technologies.
- Prioritize Data Quality: The insights derived from data are only as good as the data itself.
- Embrace Contextual Intelligence: Incorporate advanced techniques to handle unstructured data and complex relationships.
- Optimize Resource Allocation: Use analytics to drive efficiency and cost-effectiveness in operations.
Flexibility in analytics means being prepared to pivot and adapt strategies as new challenges and opportunities arise. It's about embedding adaptability into the very DNA of your agency's data strategy.
By fostering an environment where data strategies are built to change, organizations can remain agile in the face of growth and complexity. This ensures not only the scalability of data systems but also the continuous relevance and applicability of analytical insights.
Adapting to Evolving Data Landscapes
As the data landscape continues to evolve rapidly, businesses are compelled to stay abreast of emerging trends to maintain competitiveness. Scalability and flexibility are paramount in ensuring that data strategies are resilient to changes and growth. An adaptive approach to data management must be deeply rooted in the DNA of an organization, allowing for the seamless integration of new technologies and methodologies.
In the face of large, complex datasets, including unstructured data, the ability to derive meaningful insights is critical. This requires a robust infrastructure that can handle the volume and complexity of data generated by businesses and individuals.
To effectively navigate these challenges, organizations must focus on several key areas:
- Establishing robust data governance frameworks to ensure data quality and integrity.
- Investing in technology infrastructure capable of real-time processing and analysis.
- Adhering to regulatory requirements and industry standards to maintain data privacy.
By addressing these areas, businesses can adapt to the evolving digital landscape, where contextual intelligence will play an increasingly important role in shaping the future of data analytics and driving business success.
The Intersection of Health Data Analytics and Informatics
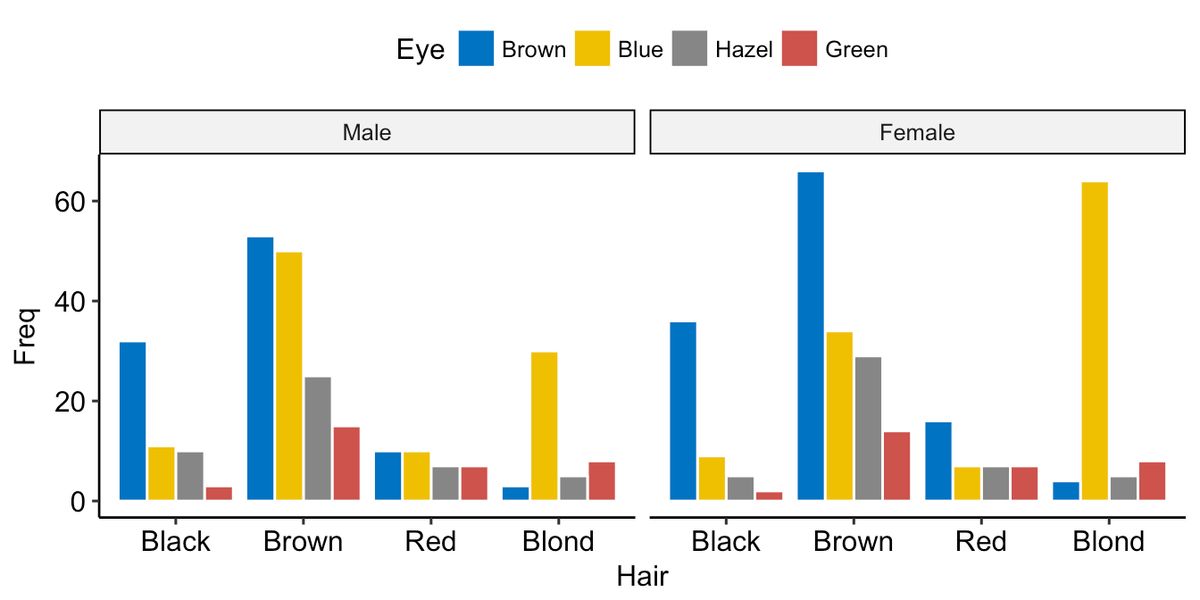
Improving Patient Outcomes with Data
The integration of healthcare data analytics is pivotal in enhancing patient care. By analyzing historical and real-time patient data, healthcare providers can deliver more effective treatment plans tailored to individual needs. Predictive health insights gleaned from analytics enable the early detection of diseases and the prediction of patient vulnerabilities, leading to proactive and personalized care.
The application of big data analytics in healthcare is not just about operational improvements; it's about revolutionizing patient care. Personalized treatment plans informed by data can significantly improve patient outcomes and satisfaction.
The transformative power of data analytics in healthcare is evident in its ability to turn insights into actionable strategies. These strategies not only benefit patients but also healthcare providers and the entire healthcare ecosystem. The following table illustrates some of the key benefits:
Benefit | Description |
---|---|
Personalized Care | Tailoring treatment plans based on patient data. |
Early Detection | Predicting diseases and conditions before they manifest. |
Preventive Strategies | Implementing interventions to prevent health issues. |
By focusing on the patient experience and leveraging data-driven insights, healthcare professionals can foster a more patient-centric approach. This leads to not only improved health outcomes but also a more positive experience for individuals within the healthcare system.
Informatics: The Backbone of Data-Driven Healthcare
Health informatics stands at the crossroads of technology and patient care, underpinning the entire healthcare analytics ecosystem. It is the discipline that ensures the strategic application of data, going beyond mere analysis to inform the development of technology solutions that enhance healthcare delivery and decision-making.
Health informatics professionals are pivotal in designing systems that not only collect and manage healthcare data but also make it accessible and actionable. Their expertise in information systems, databases, and technology facilitates the integration of analytics into healthcare processes, driving innovation and improving patient outcomes.
The challenges in the integration process often revolve around three core elements: people, process, and technology. To overcome these hurdles, a collaborative effort is essential, one that embraces data analytics for strategic thinking and leverages the data revolution for innovation and efficiency across sectors.
The synergy between health data analytics and informatics is a testament to the power of data in revolutionizing healthcare. It is a partnership that leads in innovation, optimizing processes, and ultimately, delivering better patient care.
Bridging the Gap Between Data and Clinical Practice
The integration of data analytics into clinical practice is pivotal for enhancing patient care and operational efficiency. Healthcare professionals are now equipped with tools that translate complex data into clear, actionable insights. These insights can lead to improved patient management and more effective disease prevention strategies.
The synergy between data analytics and clinical expertise is the cornerstone of a modern, evidence-based healthcare system.
To effectively bridge the gap, several key steps must be taken:
- Establishing a collaborative environment where data scientists and healthcare providers work together.
- Developing robust protocols for data sharing and privacy that align with ethical standards.
- Training clinicians in the use of analytics tools to ensure the seamless integration of data insights into patient care.
By addressing these areas, the healthcare industry can leverage the full potential of data analytics to inform decision-making and enhance the quality of care.
Optimizing Data Visualization and AI for Insight Extraction
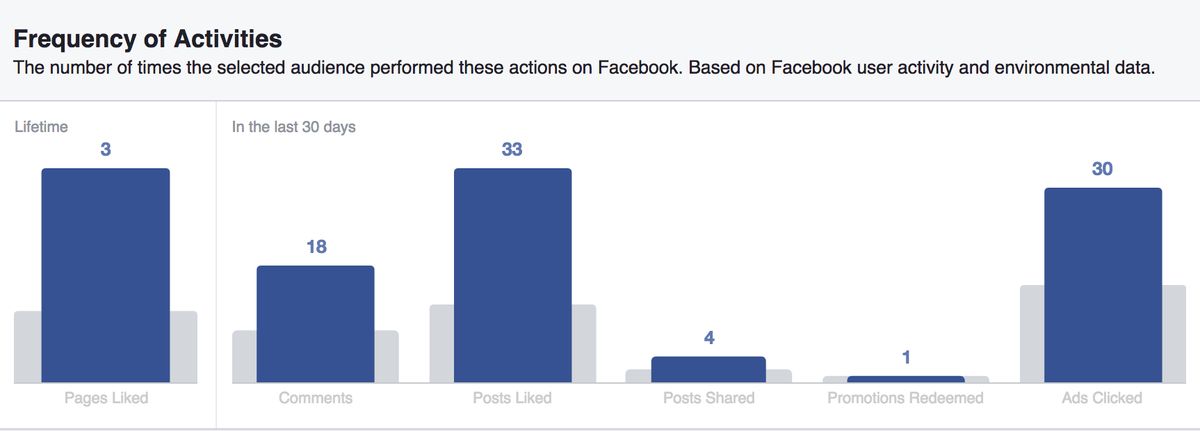
The Power of Visual Data Interpretation
The ability to interpret complex data visually is a cornerstone of effective analytics. Visualizations can transform abstract numbers into intuitive stories, making it easier for stakeholders to grasp the nuances of data and make informed decisions. For instance, a well-designed chart can highlight trends and outliers that might be missed in a spreadsheet.
Visual data interpretation is not just about aesthetics; it's a practical tool for uncovering insights. Consider the following table summarizing patient recovery times across different treatments:
Treatment Type | Average Recovery Time (days) |
---|---|
Medication A | 14 |
Medication B | 10 |
Physical Therapy | 12 |
This table succinctly conveys the effectiveness of treatments, guiding healthcare providers in optimizing patient care plans.
By focusing on the visual representation of data, analysts can convey complex information in a manner that is both accessible and actionable. This approach not only aids in the interpretation of data but also in the communication of findings to those who need it most.
Incorporating visual tools into the analytics process is essential. It allows for the rapid assimilation of information and supports the transition from insight to action. As such, organizations should invest in training and tools that enhance their team's ability to create and interpret visual data.
Integrating AI for Deeper Analytical Insights
The advent of Artificial Intelligence (AI) in the realm of data analytics has marked a significant shift towards more sophisticated and informed decision-making processes. AI's ability to process and analyze large volumes of data has transformed the landscape of business intelligence, moving beyond the hype to become a fundamental component in deriving actionable insights.
In the healthcare sector, the integration of AI and machine learning is revolutionizing patient care. By enhancing predictive capabilities and streamlining diagnostic processes, AI-powered analytics are enabling early disease detection and proactive interventions. This is particularly evident in the way AI can identify patterns and trends within vast datasets—tasks that are often too complex for human analysts.
- AI-driven predictive analytics
- Machine learning for pattern recognition
- Proactive healthcare interventions
The integration of predictive analytics and machine learning into data analytics platforms is not just a trend; it's a strategic move towards more informed and efficient decision-making.
As we continue to leverage AI in various aspects of data analytics, it's crucial to recognize the potential for these technologies to improve not only business operations but also patient outcomes in healthcare.
Balancing Quantitative and Qualitative Data Analysis
In the realm of data analytics, the harmonization of quantitative and qualitative analysis is paramount. Advanced analytics focus on quantitative and qualitative outcomes, ensuring a comprehensive view of data. While quantitative data offers structured insight, qualitative data provides context and depth, making the combination of both essential for nuanced decision-making.
- Based on your goals, select an analysis method.
- Collect user behavior data through event tagging and user feedback.
- Utilize data visualization and AI to enhance analysis.
- Identify and prioritize opportunities and challenges.
- Implement solutions iteratively and improve continuously.
Strategic data collection emphasizes accuracy, completeness, and relevance, ensuring a solid foundation for analytics. This focus on quality over sheer volume is crucial for deriving actionable insights.
Agencies that excel in data analytics understand the importance of not just reporting numbers, but also orchestrating and interpreting data to uncover qualitative insights. The synergy between data orchestration, analysis, interpretation, and reporting is what drives informed decision-making and ultimately, business intelligence.
Prescriptive Analytics in Healthcare
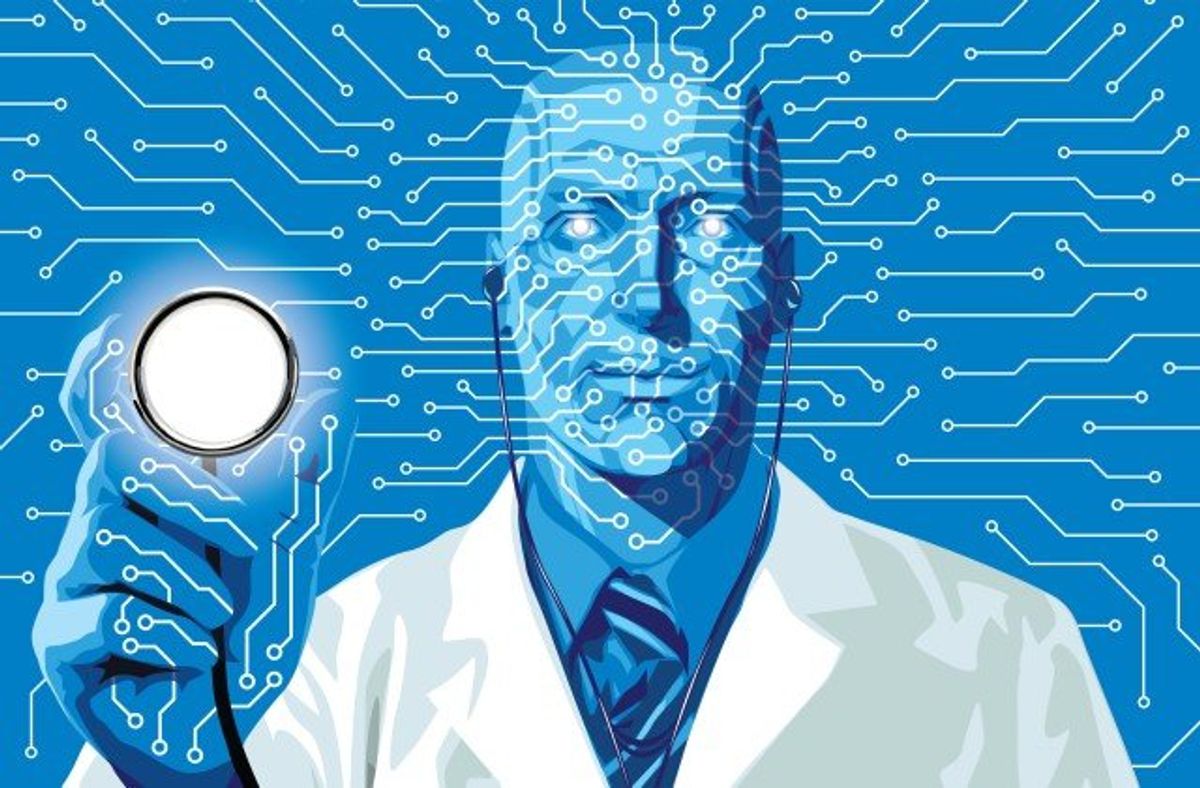
Beyond Descriptive and Predictive Analytics
Prescriptive analytics represents a significant leap forward from its descriptive and predictive counterparts. It harnesses the power of machine learning algorithms to not only interpret historical data but to also recommend actionable strategies for decision-making. This advanced form of analytics is transformative in healthcare, enabling providers to tailor patient care, allocate resources efficiently, and streamline operational processes.
By integrating prescriptive analytics, healthcare organizations can proactively address patient needs, anticipate potential health issues, and optimize treatment plans, leading to improved patient outcomes and operational excellence.
Discovery analytics, while akin to prescriptive analytics, zeroes in on clinical data to unearth patterns that yield actionable insights. It is instrumental in identifying previously unrecognized opportunities for enhancing patient care. Below is a list of benefits that these advanced analytics categories offer:
- Predictive Health Insights: Anticipating patient vulnerabilities to certain conditions for early intervention.
- Accurate Health Insurance Rates: Refining insurance rates by evaluating individual risk factors.
- Proactive Decision Making: Utilizing forecasts to prevent complications and personalize care.
The integration of these analytics into healthcare systems is not just about data processing; it's about transforming data into a roadmap for quality care and operational efficiency.
Machine Learning and Decision-Making
The integration of machine learning (ML) with healthcare analytics heralds a new era in medical decision-making. ML algorithms excel at identifying patterns and anomalies in large datasets, which can lead to breakthroughs in patient care and operational efficiency. For instance, ML can predict patient outcomes, prevent complications, and optimize treatment plans, thereby enhancing the delivery of personalized care.
The use of ML in healthcare is not just about data analysis; it's about transforming that data into actionable strategies that improve patient experiences and outcomes.
Healthcare organizations can leverage ML in several key areas:
- Predictive analytics for early disease detection
- Personalized treatment recommendations
- Resource allocation and operational management
By employing prescriptive analytics, healthcare providers can move beyond historical data to propose actionable strategies for decision-making. This proactive approach is crucial for optimizing patient care and resource management.
Operational Efficiency and Resource Optimization
In the realm of healthcare, operational efficiency and resource optimization are critical for delivering high-quality care while maintaining cost-effectiveness. Advanced analytics serve as a catalyst for identifying areas of waste and enabling the maximization of value across various healthcare processes.
Decentralized decision-making empowers those with the most relevant knowledge and proximity to day-to-day operations, fostering a more responsive and agile organizational structure. By leveraging data insights, healthcare providers can ensure that resources are allocated effectively, striking a balance between patient needs and operational capabilities.
thereby facilitating the development of more efficient and targeted strategies.
Here's an example of how analytics can prioritize improvements:
- Identify opportunities for enhancing the user experience.
- Use a scoring model to prioritize these opportunities.
- Focus on optimizations that align with both product and organizational goals.
- Iterate and refine strategies for continuous improvement.
Ultimately, analytics not only contributes to a smoother healthcare experience but also ensures that resources such as personnel, equipment, and facilities are utilized to their fullest potential, minimizing waste and improving patient care.
Contextual Intelligence: The Future of Data Analytics
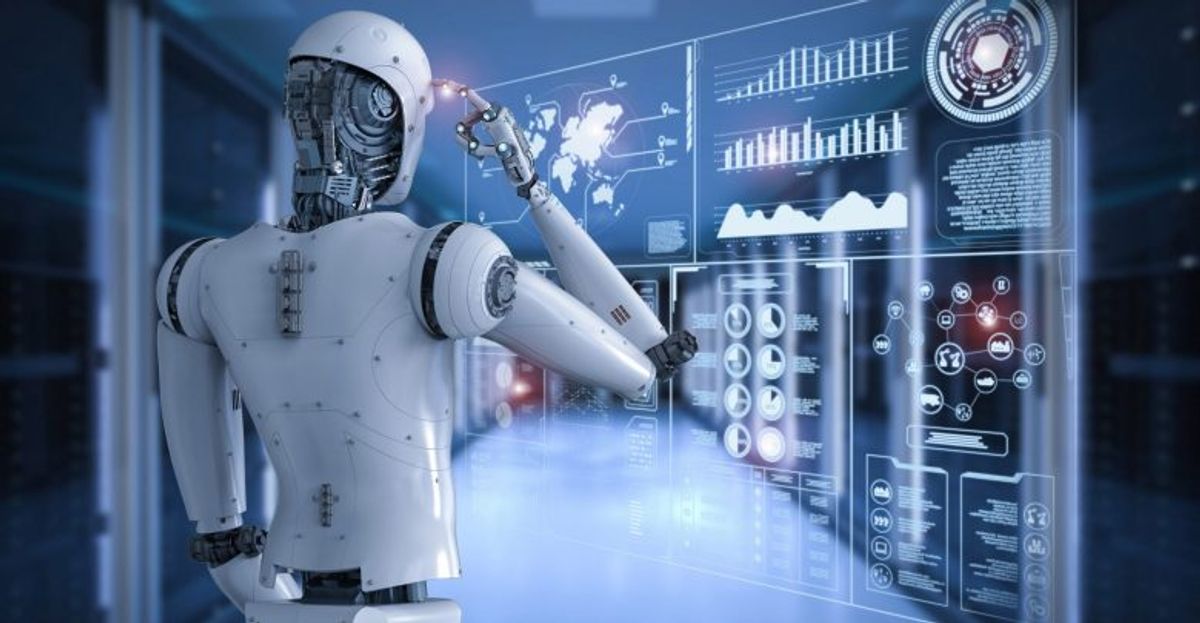
Defining Contextual Intelligence
Contextual intelligence is the analytical capability to process data not just on its own, but within the framework of its environment, relationships, and interactions. It represents a paradigm shift from traditional data analysis, focusing on the holistic understanding of data to uncover more profound insights and drive strategic decisions.
Contextual intelligence is increasingly vital in a world where data complexity is escalating. The integration of varied data sources, from structured databases to unstructured social media feeds, necessitates a method that can weave these disparate strands into a coherent narrative. This approach is not just about connecting dots but understanding the tapestry they form.
The essence of contextual intelligence lies in its ability to transform raw data into a rich tapestry of insights, enabling real-time decision-making and strategic foresight.
The applications of contextual intelligence are manifold, impacting sectors from healthcare to finance. Here's how it translates across different industries:
- Healthcare: Enhancing patient care by considering the full spectrum of patient history, lifestyle, and genetics.
- Finance: Detecting fraud by examining transactions within the broader scope of historical patterns.
- Retail: Tailoring customer experiences by understanding purchasing behaviors in the context of market trends.
Enhancing Data Interpretation with Context
In the realm of data analytics, contextual intelligence stands as a transformative force, enabling a more nuanced understanding of complex data sets. By analyzing data within the broader scope of its environment and interactions, organizations can uncover deeper insights that were previously obscured by the limitations of traditional analytics.
Contextual intelligence is not just about the data itself, but about the story it tells when viewed through the lens of its surroundings. This approach allows for a more holistic interpretation, which is crucial for making informed decisions and driving innovation.
- Understanding the environment in which data exists
- Recognizing the relationships and interactions within data
- Deriving meaning and significance from complex data ecosystems
Embracing contextual intelligence equips organizations with the ability to navigate the intricate web of data sources, transforming raw data into actionable strategies that align with their overarching goals.
As we venture further into an era where data complexity is the norm, the adoption of contextual intelligence will become a cornerstone for organizations aiming to leverage their data assets effectively.
Case Studies: Success Stories in Contextual Analytics
The advent of contextual intelligence in data analytics has led to a series of transformative success stories across various industries. Big data integration has been pivotal in enhancing decision-making, operational efficiency, and strategic planning. However, organizations often face challenges such as ensuring data quality and developing robust analytics capabilities.
One notable example is in healthcare, where contextual intelligence enables providers to offer personalized care by analyzing patient data alongside medical history and lifestyle factors. Similarly, in the financial sector, it aids in detecting fraudulent activities through the analysis of transactions within historical and behavioral contexts.
Embracing contextual intelligence allows organizations to transcend traditional analytics limitations, unlocking new opportunities for innovation and value creation.
The table below summarizes the impact of contextual intelligence in different sectors:
Sector | Improvement | Example |
---|---|---|
Healthcare | Personalized Care | Patient data analysis |
Finance | Fraud Detection | Transaction monitoring |
Retail | Customer Experience | Shopping pattern analysis |
Actionable Strategies from Data-Driven Insights
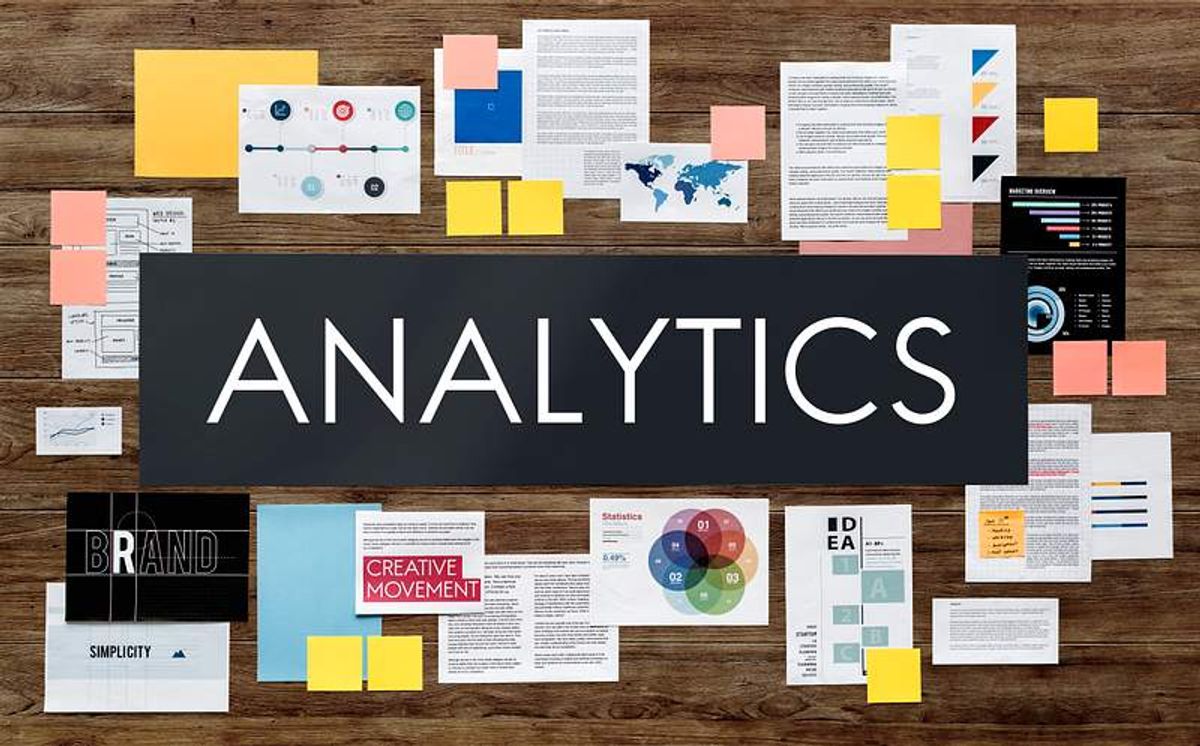
Identifying Opportunities and Challenges
In the pursuit of transforming analytics into action, the initial step is to sift through user data to uncover both obstacles that need to be surmounted and prospects that can be harnessed. Identifying a sequence of user actions that indicate a readiness to upgrade can serve as a catalyst for timely interventions, such as triggering in-app messages to encourage users to take the next step.
When faced with an abundance of potential enhancements to the user experience, it's crucial to employ a scoring model to prioritize these opportunities. This ensures that efforts are concentrated on the initiatives that most closely align with the product's vision and the organization's strategic objectives.
- Prioritize opportunities using a scoring model
- Align initiatives with product vision and organizational goals
- Trigger timely interventions based on user behavior
By meticulously analyzing both quantitative and qualitative user data, organizations can pinpoint the most impactful areas for improvement.
The integration of AI in analyzing qualitative user feedback further enriches the understanding of user needs, allowing for a more nuanced approach to addressing challenges. The iterative process of implementation and refinement is essential, as it enables organizations to adapt and evolve their strategies in response to new insights and changing market dynamics.
Prioritizing Actions Aligned with Organizational Goals
In the pursuit of transforming analytics into action, it is crucial to prioritize initiatives that are most aligned with the organization's strategic objectives. Fostering a data-driven culture is essential as it not only aligns analytics with business objectives but also overcomes cultural barriers and utilizes cutting-edge tools for advanced analytics implementation.
When faced with a multitude of potential improvements, employing a scoring model can be an effective way to rank these opportunities. This model should reflect the unique criteria that resonate with the product's vision and the organization's broader goals. For example:
- Impact on user experience
- Alignment with strategic priorities
- Feasibility and resource requirements
- Expected return on investment
By systematically evaluating each opportunity against these criteria, organizations can ensure that they are not just data-rich but also insight-driven.
Incorporating project management tools, such as Asana or Trello, can aid in the visualization and tracking of these priorities. Not all tasks are created equal, and understanding which ones are crucial to progress is key to maintaining focus on what matters most. This approach not only streamlines operational processes but also optimizes resource allocation, leading to enhanced efficiency and cost-effectiveness.
Iterative Implementation and Continuous Improvement
The journey from analytics to action is not a one-time event but an ongoing cycle of refinement. The iterative process is crucial for adapting strategies to real-world feedback and outcomes. By cyclically creating, reviewing, and updating initiatives, organizations can foster a culture of continuous improvement.
When faced with numerous potential enhancements, it's essential to prioritize. A scoring model can be employed to align improvements with organizational goals. This ensures that efforts are concentrated on the most impactful changes.
The key to successful iteration is not to overwhelm with changes but to introduce them incrementally. Testing updates in small segments allows for measured adjustments and risk mitigation.
Monitoring and measuring the effects of these changes is the final step in the cycle. Utilizing tools like trend and cohort analysis helps in identifying the impact of each iteration on product metrics, informing the next cycle of improvements.
- Identify opportunities for improvement
- Prioritize using a scoring model
- Roll out changes incrementally
- Monitor and measure impact
- Refine and iterate based on data
The Transformative Impact of Data Analytics in Healthcare
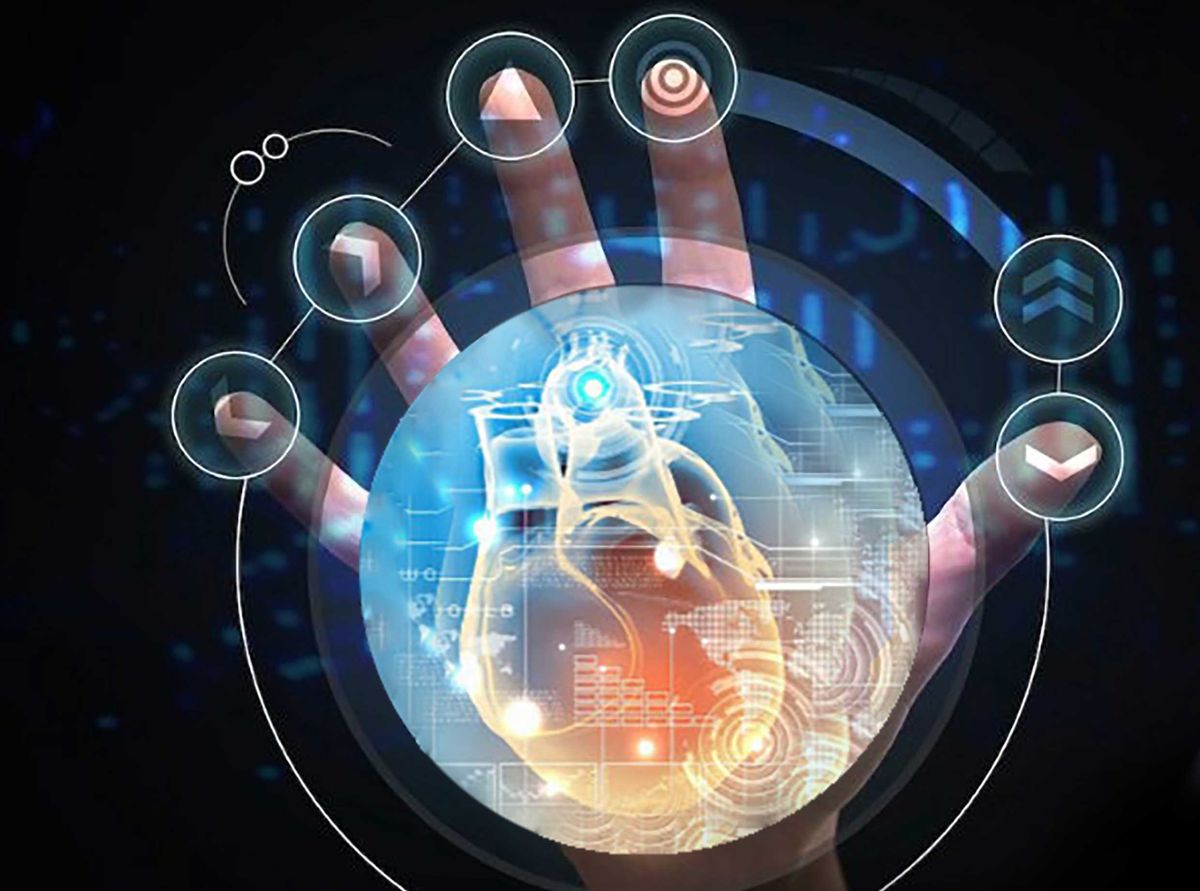
Quality of Care and Patient Management
Healthcare analytics is pivotal in enhancing not just organizational efficiency but also the quality of patient care. By leveraging data-driven insights, healthcare professionals can create personalized treatment plans, improve preventive care, and foster a patient-centric approach, leading to better health outcomes and a more satisfying healthcare experience.
Improved Patient Care and predictive health insights are at the forefront of this transformation. Analytics enables the prediction of patient vulnerabilities to specific conditions, allowing for preemptive and more effective interventions.
The integration of analytics into healthcare decision-making streamlines processes such as appointment scheduling, which benefits both patients and staff by reducing wait times and maximizing resource utilization.
Here's a glimpse at how analytics contributes to patient management:
- Personalized care plans based on historical and real-time data
- Enhanced preventive strategies
- Optimized scheduling for reduced wait times
- Fair and accurate insurance pricing
Embracing analytics in healthcare not only improves patient management but also sets the stage for a more efficient and patient-focused healthcare system.
Advancing Healthcare through Data-Driven Strategies
The integration of big data analytics in healthcare is not without its challenges, yet it is essential for the evolution of the sector. Implementing key strategies can facilitate a smoother transition and enable healthcare providers to harness the full potential of data-driven insights. These strategies are not just theoretical; they are practical pathways to innovation and improved patient care.
- Embrace technological advancements to enhance data collection and analysis.
- Foster collaboration between data scientists, healthcare professionals, and technology experts.
- Prioritize the personalization of patient care through data insights.
- Invest in training for healthcare staff to effectively utilize analytics tools.
Healthcare analytics represents a powerful approach to leveraging data within the intricate landscape of the health care sector. This specialized field employs advanced analytical techniques to extract valuable insights that can drive informed decision-making.
As technological innovations and data-driven insights converge, we witness the creation of a more effective, personalized, and sustainable healthcare ecosystem. The ongoing collaboration between these key stakeholders is instrumental in unlocking the full potential of data analytics for the benefit of patients and healthcare organizations.
The Role of Analytics in Healthcare Innovation
The integration of analytics into healthcare is not just a matter of processing large datasets; it represents a paradigm shift towards a more data-driven approach to innovation. Healthcare analytics is instrumental in uncovering patterns and correlations that lead to groundbreaking advancements in patient care and operational efficiency.
Healthcare analytics serves as the cornerstone for evidence-based decision-making, enabling providers to tailor treatments and optimize resource allocation. This analytical prowess is crucial for fostering a healthcare environment that is both effective and patient-centric.
- Identification of health trends and risk factors
- Personalization of patient care plans
- Streamlining of hospital operations
- Enhancement of disease surveillance
The true value of analytics in healthcare lies in its ability to convert complex data into clear, actionable insights that drive progress and innovation.
As we continue to witness the transformative impact of data analytics, it is clear that the future of healthcare will be increasingly informed by the intelligent interpretation and application of data. The synergy between analytics and healthcare not only improves current practices but also opens doors to new possibilities for enhancing patient outcomes and operational workflows.
The Transformative Impact of Data Analytics in Healthcare is undeniable. With the right expertise, healthcare organizations can unlock the potential of their data, leading to improved patient outcomes and operational efficiencies. At OptimizDBA, we specialize in database optimization consulting, ensuring your data solutions are not just faster, but also more reliable and effective. Don't let data challenges hinder your healthcare services. Visit our website to learn how we can help you experience transaction speeds that are at least twice as fast, with a performance guarantee that is unmatched in the industry. Take the first step towards transformation – contact us today!
Conclusion
In the journey from data to decision-making, the final destination is always actionable insight. Throughout this article, we've explored the myriad techniques that transform the overwhelming streams of big data into clear, actionable strategies. From the scalability and flexibility of modern analytics tools to the nuanced interpretation of qualitative data, we've seen how advanced analytics can inform and enhance every aspect of organizational operations. The healthcare industry serves as a prime example, where data analytics not only improves patient outcomes but also streamlines operational efficiency. As we embrace methods like contextual intelligence and employ advanced analytics, the potential to revolutionize industries and drive progress is immense. The key takeaway is clear: by harnessing the power of big data through strategic analysis and application, businesses can unlock unprecedented opportunities for growth and innovation.
Frequently Asked Questions
How does advanced analytics transform raw data into actionable insights?
Advanced analytics uses statistical methods and tools to interpret raw data and derive qualitative insights, which can be translated into data-driven recommendations for enhancing performance and driving campaign success.
What is the importance of scalability and flexibility in data analytics?
Scalability ensures that data analytics processes can handle growing amounts of data efficiently, while flexibility allows for adaptation to new data types and evolving analytical requirements.
How does health data analytics improve patient outcomes?
Health data analytics extracts meaningful insights from datasets to inform decision-making in clinical care, such as improving patient management, disease prevention strategies, and adherence to clinical protocols.
What role does data visualization and AI play in data analysis?
Data visualization aids in the interpretation of quantitative data, while AI analytics can uncover deeper insights, contributing to both quantitative and qualitative data analysis.
How can prescriptive analytics benefit healthcare providers?
Prescriptive analytics uses machine learning to analyze historical data and propose actionable strategies, optimizing patient care, resource allocation, and operational processes.
What is contextual intelligence in data analytics?
Contextual intelligence is an approach that adds context to raw data, enhancing the interpretation and understanding of data to extract more accurate and actionable insights.
What steps should be taken to transform analytics into action?
To transform analytics into action, select an analysis method based on goals, collect user behavior data, use data visualization and AI for analysis, identify opportunities and challenges, prioritize actions, and iteratively implement solutions.
What is the transformative impact of data analytics in healthcare?
Data analytics in healthcare leads to improved quality of care, operational efficiency, and advances in healthcare strategies, ultimately enhancing the healthcare industry.