The Role of Business Analytics in Strategic Planning
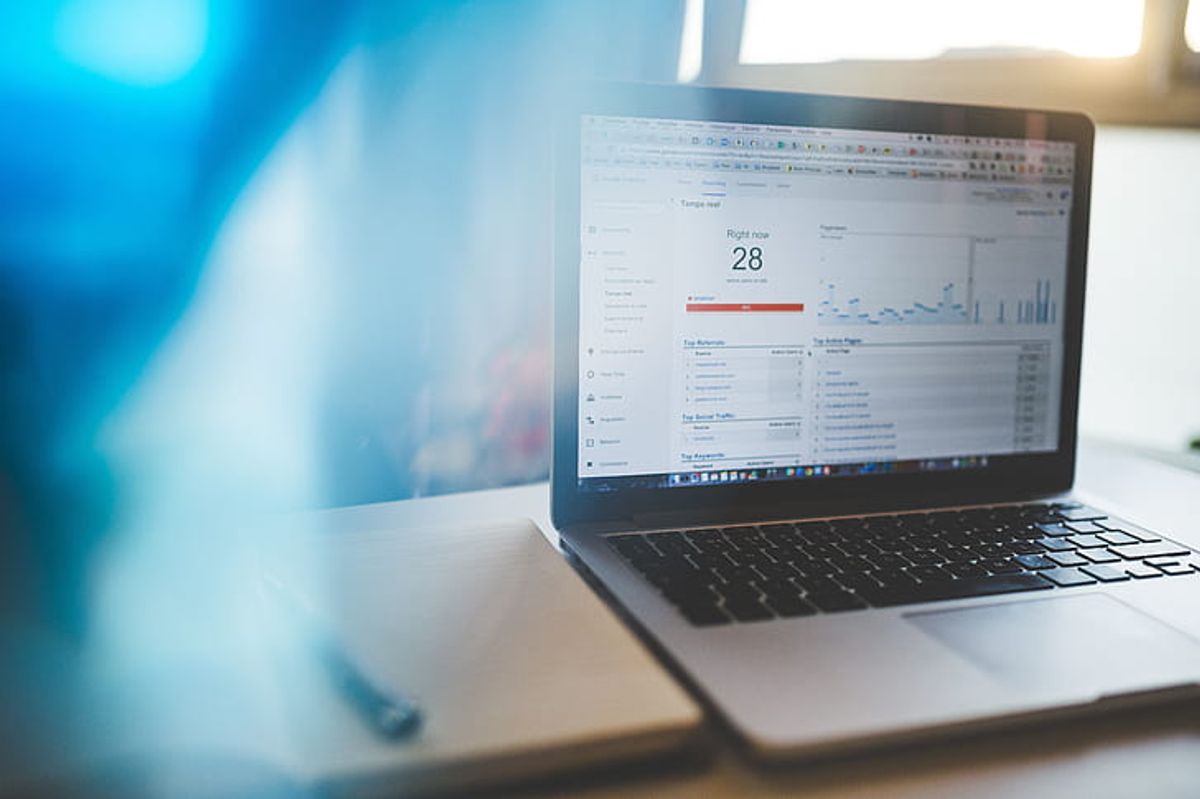
Utilizing Data Insights for Long-Term Goals
In the realm of strategic planning, leveraging data insights is crucial for setting and achieving long-term business objectives. By examining data sets for meaningful patterns, businesses can forecast trends, anticipate market changes, and align their resources accordingly. This proactive approach enables organizations to stay ahead of the curve and maintain a competitive edge.
Data analytics plays a pivotal role in this process, transforming raw data into actionable intelligence. For instance, a company might analyze customer behavior to determine potential areas for product expansion or to tailor marketing strategies that resonate with target demographics.
The integration of predictive analytics into strategic planning ensures that decision-makers are equipped with foresight, not just hindsight, empowering them to craft visionary goals that are informed by data-driven predictions rather than mere speculation.
To effectively utilize data insights for long-term planning, consider the following steps:
- Identify key performance indicators (KPIs) relevant to long-term objectives.
- Collect and analyze historical data to establish baselines and trends.
- Implement advanced analytics to project future scenarios.
- Regularly review and adjust strategies based on new data and insights.
Implementing Data-Driven Strategies for Growth
In the era of Big Data, implementing data-driven strategies is crucial for business growth. Companies that harness the power of data insights can not only maximize revenue but also gain a significant competitive advantage. To achieve this, businesses must invest in the right tools and talent, fostering a data-driven culture that permeates every level of the organization.
The process of integrating data analytics into business strategy involves several key steps:
- Identifying growth opportunities through data analysis
- Aligning data insights with business objectives
- Developing actionable strategies based on data findings
- Iterating the analytics process to refine strategies
By systematically analyzing customer behavior, market trends, and operational efficiency, businesses can make strategic decisions that propel them forward. Embracing data-driven decision-making (DDDM) provides a more objective foundation for these choices, moving away from intuition-based decisions.
Ultimately, the shift towards a data-centric culture is not just about adopting new technologies; it's about valuing data as a critical asset and making it accessible to decision-makers across departments. This methodical approach to strategic planning is the key to unlocking a business's full potential.
Measuring Performance Metrics for Decision-Making
In the realm of business analytics, measuring performance metrics is crucial for informed decision-making. A performance indicator, as the title '30 KPIs To Measure Performance (& How To Choose & Track Them)' suggests, tracks a measure related to your organization's performance. For example, manufacturing companies may focus on metrics such as production volume, defect rates, or supply chain efficiency.
Data analysis plays a pivotal role in enhancing decision-making by providing insights and statistical probabilities that guide critical choices based on empirical evidence rather than human bias. By understanding historical data, businesses can link it to future improvements in their models, identifying patterns, trends, and correlations that inform strategic decisions.
Decision analysis offers a systematic approach to evaluating options and making informed choices. It emphasizes post-solution analysis, which aids in identifying key success factors and competitive advantages.
To effectively measure and track performance metrics, consider the following steps:
- Define clear and relevant KPIs.
- Establish a baseline for comparison.
- Regularly collect and analyze data.
- Adjust strategies based on findings.
- Communicate results and insights to stakeholders.
Data Visualization Techniques for Effective Communication
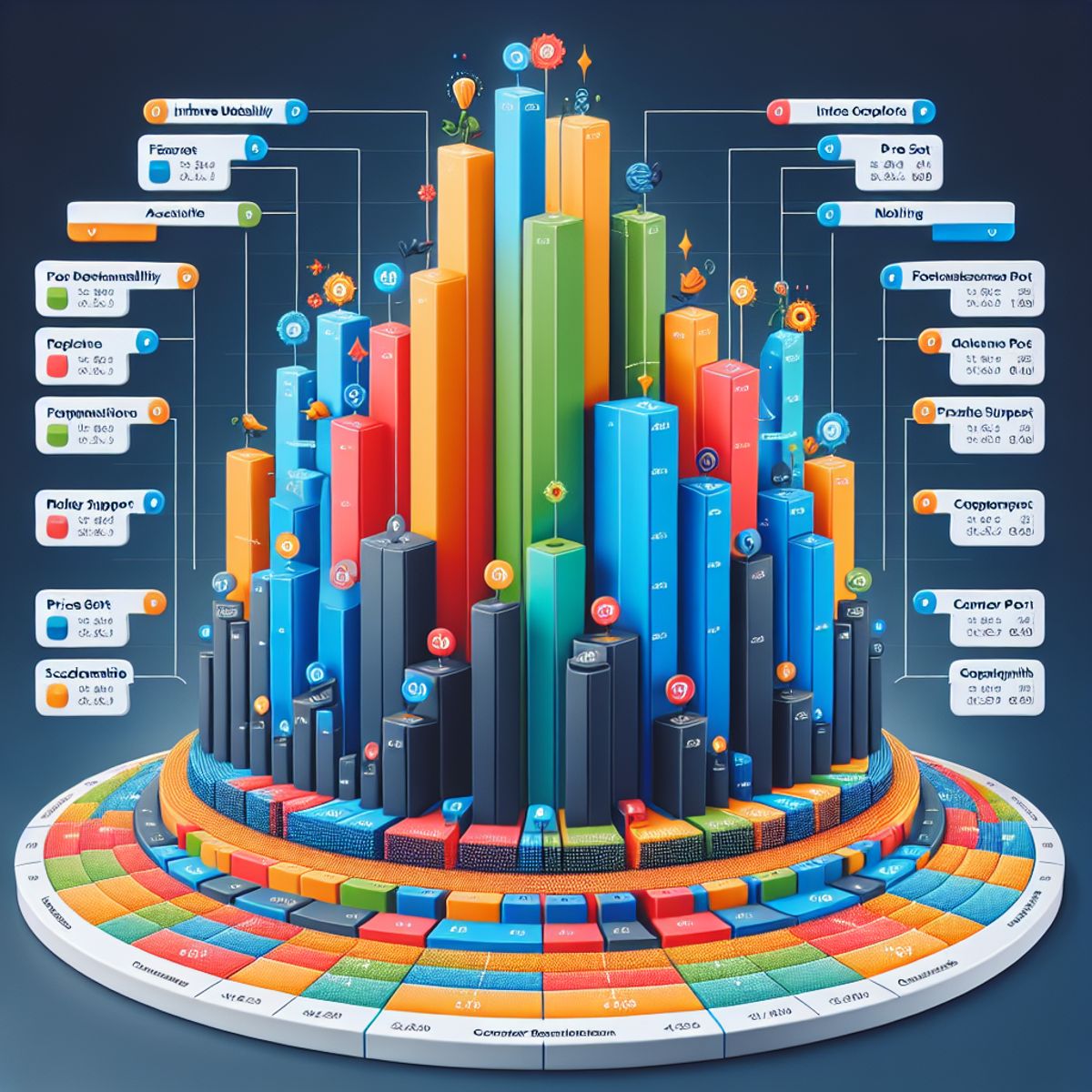
Creating Impactful Dashboards
In the realm of business intelligence, dashboards serve as the linchpin for presenting complex data in an accessible and actionable format. The design of these dashboards is critical, as they must not only be visually appealing but also intuitive enough to guide decision-makers towards key insights and trends.
To create an impactful dashboard, consider the following steps:
- Identify the key performance indicators (KPIs) that align with business objectives.
- Choose a layout that enhances the readability and allows for quick comparisons.
- Select appropriate chart types for the data being represented.
- Implement interactive elements to enable deeper exploration of the data.
By focusing on these elements, organizations can ensure that their dashboards effectively communicate the right information to the right people at the right time.
Remember, the ultimate goal of a dashboard is to distill large datasets into clear visualizations that drive informed decisions. With the right tools and approaches, dashboards can become a powerful asset in any organization's analytical arsenal.
Utilizing Infographics for Data Representation
In the age of information overload, infographics serve as a beacon of clarity, transforming complex data into digestible visual narratives. These visual tools are not just about aesthetics; they are a powerful means to encapsulate and convey insights with immediacy and impact.
Infographics synthesize data and concepts into a format that's both accessible and memorable, making them an invaluable asset in business analytics.
Here's why infographics are indispensable in data representation:
- They distill large amounts of data into a concise visual form.
- Infographics can highlight trends, patterns, and outliers at a glance.
- They are shareable and can effectively communicate findings to a broad audience.
As we navigate through the evolving landscape of data analytics, it's clear that the ability to present data visually will remain a cornerstone of guiding decision-makers. The future of data representation is bright, with advancements like mixed reality (MR) beginning to overlay data onto real-world scenarios, offering a more enriching and engaging way to interact with information.
Interactive Data Storytelling Approaches
Interactive data storytelling transcends traditional reporting by weaving data into a narrative that is both engaging and enlightening. Data storytelling and visualization are essential in business intelligence, empowering decision-makers with actionable insights and driving innovation. Tailoring narratives to engage and inform is key for success.
Often, surrounded by numbers, graphs, and charts, many might feel stuck in a data deluge. Descriptive analytics emerges as a reliable interpreter, transforming raw data into a compelling narrative.
In the realm of data analytics, the integration of technologies such as Extended Reality (XR) is revolutionizing the way we interact with data. Imagine exploring complex datasets as three-dimensional landscapes, pinpointing trends by navigating through interactive charts in a virtual environment. This immersive approach not only makes data more accessible but also more memorable.
Here are some key benefits of interactive data storytelling:
- Enhances understanding through immersive experiences
- Facilitates deeper engagement with data
- Encourages exploration and discovery
The future of data-driven decision making (DDDM) is bright, with advancements in technology continually expanding the possibilities for how we can visualize and interact with data.
Predictive Analytics for Future Forecasting
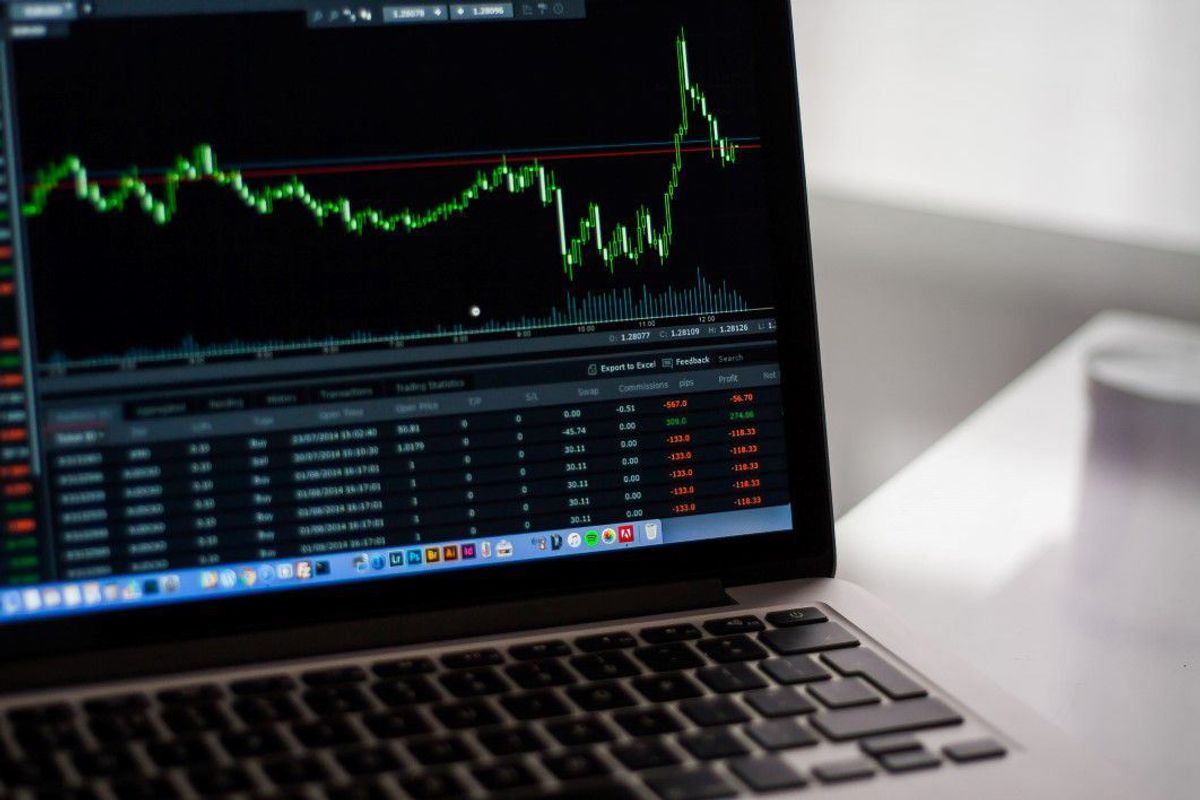
Utilizing Machine Learning Algorithms
In the realm of predictive analytics, the application of machine learning (ML) algorithms stands as a cornerstone for forecasting future trends and behaviors. These algorithms enable businesses to sift through vast datasets and uncover patterns that would otherwise remain hidden. ML techniques, such as decision trees and support vector machines, are not only adept at identifying these patterns but also at making predictions with a high degree of accuracy.
By integrating ML algorithms into their analytics framework, companies can automate complex tasks, enhance decision-making, and gain a competitive edge. The predictive power of ML is particularly valuable in sectors like finance, healthcare, and retail, where forecasting accuracy can have a significant impact on strategic outcomes.
To effectively leverage ML in business analytics, it is crucial to follow a structured approach:
- Identifying the business problem and relevant data sources
- Preprocessing and cleaning the data to ensure quality
- Selecting and training appropriate ML models
- Evaluating model performance and making necessary adjustments
- Deploying the model for real-time predictions and insights
This process ensures that the insights generated are not only accurate but also actionable, leading to informed decision-making and strategic planning.
Forecasting Trends and Patterns
In the realm of business analytics, forecasting trends and patterns is a cornerstone for strategic decision-making. By leveraging historical data, companies can anticipate market changes and pivot their strategies proactively. This shift from a reactive to a proactive stance is crucial for maintaining a competitive edge.
Predictive analytics has undergone a significant evolution, becoming more sophisticated with the integration of causal AI, deep learning, and machine learning models. These advancements have enhanced the ability to not only predict future events but also to understand their underlying causes and account for uncertainties, leading to more reliable forecasts.
The application of predictive analytics extends across various domains, including demand forecasting, fraud detection, and targeted marketing, proving its versatility and value in business operations.
Here are some key trends shaping the future of data analytics:
- Embracing advanced techniques for more accurate predictions
- Transitioning from basic forecasting to comprehensive predictive models
- Utilizing statistical modeling, machine learning, and deep learning
Understanding these trends is essential for businesses to stay ahead in a data-driven world.
Risk Assessment and Mitigation Strategies
In the realm of business analytics, risk assessment and mitigation are critical for safeguarding the future of an organization. Identifying potential risks is the first step in a proactive approach to risk management. This involves a thorough analysis of both internal and external factors that could impact the business.
Once risks are identified, the next step is to assess their potential impact. This assessment should be both qualitative and quantitative, providing a clear picture of the possible outcomes. Following this, it's crucial to treat the identified risks by implementing appropriate measures to either eliminate or reduce them. Continuous monitoring and reporting are essential to ensure that the risk mitigation strategies remain effective over time.
In the context of predictive analytics, systems like CryptoRLPM have been developed to manage portfolios with an emphasis on minimizing trading risk. By leveraging advanced algorithms, businesses can enhance their decision-making processes and stay ahead of potential threats.
To illustrate the process of risk mitigation, consider the following steps:
- Identify potential risks
- Assess the impact
- Treat the risks
- Monitor and report on the effectiveness
Ethical Considerations in Data-Driven Decision-Making
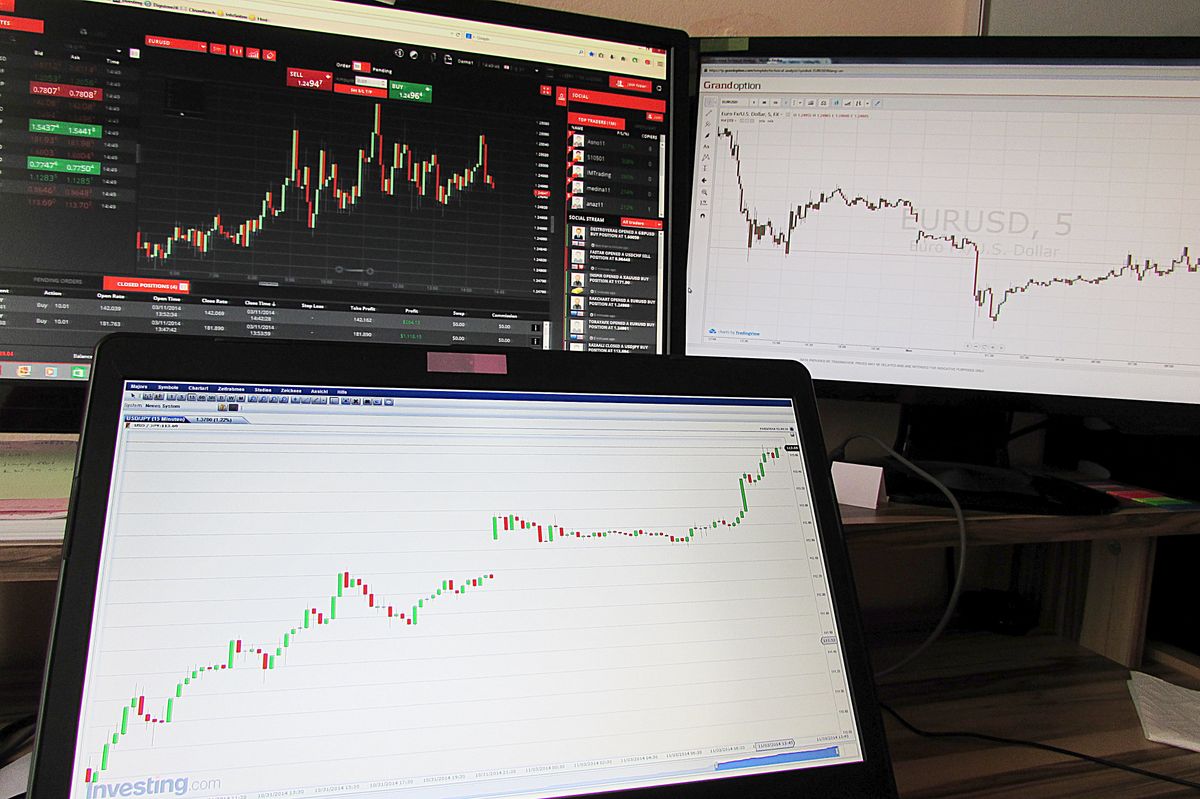
Ensuring Data Privacy and Security
In the realm of business analytics, ensuring data privacy and security is not just a regulatory requirement; it's a cornerstone of trust between a company and its stakeholders. Robust data governance frameworks are essential to protect sensitive information and maintain the integrity of business operations.
Ethical considerations in analytics include protecting user privacy, avoiding bias, and ensuring transparency. Robust data governance and diverse, monitored data analysis are key for ethical usage.
To effectively safeguard data, businesses must adhere to a multi-layered approach:
- Regularly update and enforce privacy policies.
- Implement strong encryption methods for data at rest and in transit.
- Conduct frequent security audits and risk assessments.
- Ensure that all employees are trained on data security best practices.
By prioritizing these practices, companies can navigate the complexities of data ethics and privacy, and uphold their commitment to responsible data management.
Addressing Bias in Data Analysis
In the realm of business analytics, overcoming biases is a pivotal challenge that can significantly impact decision-making. Bias in data analysis can skew insights and lead to erroneous conclusions, which in turn can affect the entire spectrum of business operations, from strategic planning to customer experiences. To address this, companies must adopt a multifaceted approach.
Data quality assessment is the first step in identifying potential biases. By evaluating the sources and methods of data collection, businesses can pinpoint areas where biases may occur. The following list outlines key actions to mitigate bias:
- Conducting regular audits of data collection and analysis processes
- Implementing diverse data sets to avoid over-representation of any single group
- Training data scientists and analysts on the importance of unbiased data interpretation
Ensuring that data-driven decisions are fair and objective is not just a technical necessity but also an ethical imperative.
It is essential to integrate robust data governance frameworks that support ethical data practices and adhere to privacy rules. As the landscape of data ethics evolves, staying informed and proactive is crucial for maintaining trust and integrity in data analytics.
Transparency in Data Usage
In the realm of business analytics, transparency in data usage is not just a legal imperative but a cornerstone of trust between a company and its stakeholders. Data democratization is a trend that underscores the ethical use of data, ensuring that the benefits of data analytics are accessible to all levels of users within an organization. This approach fosters an environment where data-driven decisions are the norm, and every team member is empowered to contribute to the company's strategic goals.
To maintain transparency, businesses must prioritize responsible data practices, which include compliance with regulations and adherence to data privacy rules. A robust data governance framework is essential to this end, as it provides clear guidelines on data access, usage, and sharing. Below is a list of key components that should be included in a data governance framework to ensure transparency:
- Clear data access policies
- Regular data audits
- Defined data usage protocols
- Training programs for data ethics
By embedding these practices into the organizational culture, companies can navigate the complexities of data analytics in business transformation, leveraging AI for insights while upholding ethical considerations for responsible use.
Implementing Business Intelligence Tools for Competitive Advantage
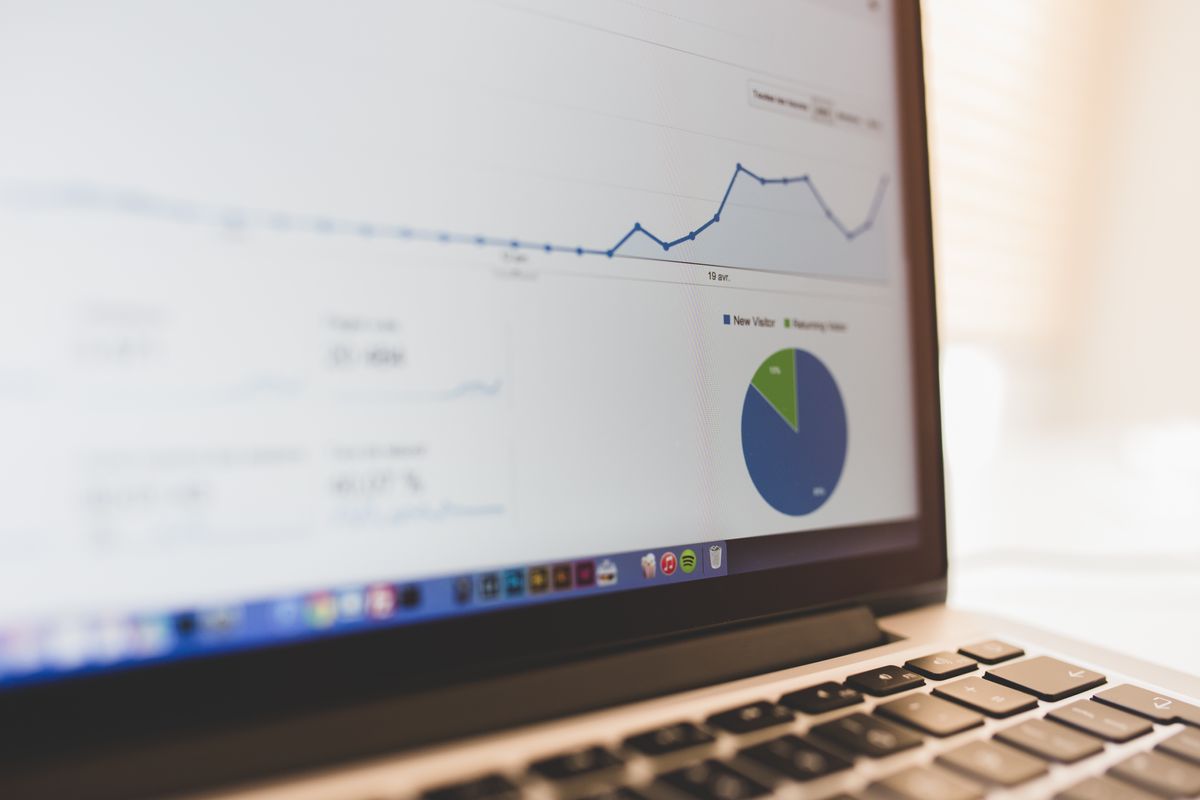
Utilizing AI for Market Analysis
In the realm of market analysis, artificial intelligence (AI) is a game-changer, offering unprecedented precision in understanding consumer behavior and market trends. By harnessing AI techniques, businesses can delve into vast datasets to uncover actionable insights that were previously inaccessible.
For instance, AI can be applied to predict business performance and identify key features to enhance prediction accuracy. This not only streamlines operations but also significantly reduces costs associated with IT personnel and hardware. Financial intelligence, augmented by AI, improves the integrity and timeliness of information, which is crucial for informed decision-making.
Current adoption rates of AI in various business operations are telling:
- 46% in Customer relationship management
- 47% in Digital personal assistants
- 40% in Inventory management
- 35% in Content production
Embracing AI in market analysis not only sharpens competitive edges but also equips businesses with the ability to anticipate market shifts and customer needs with remarkable agility.
Real-Time Data Monitoring for Quick Decisions
In the fast-paced world of business, real-time data monitoring is a game-changer. It enables organizations to observe and respond to changes as they happen, ensuring that decision-making is both timely and informed. By leveraging real-time analytics, businesses can gain a competitive edge, reacting swiftly to market trends, customer behaviors, and operational challenges.
- Timeliness of data is crucial in environments where decisions must be made quickly to capitalize on opportunities or mitigate risks.
- Machine learning models, such as J48 Decision Trees and Support Vector Machines (SVM), can process vast amounts of data, providing precise insights that drive strategic actions.
The integration of real-time data into business operations allows for immediate action, transforming insights into decisive outcomes.
Understanding the dimensions of data quality, including accuracy and timeliness, is essential for a reliable data pipeline that serves as a source of actionable business intelligence. The ability to notify concerned teams in real time can significantly enhance the effectiveness of decision-making processes.
Integration of BI Systems for Seamless Operations
The integration of Business Intelligence (BI) systems is a critical step in achieving a unified view of an organization's operations. Seamless integration ensures that data flows smoothly between systems, eliminating the risk of data silos that can obscure valuable insights. A well-integrated BI system empowers decision-makers with a comprehensive understanding of business performance.
Scalability is a key consideration when integrating BI systems. As businesses grow, their data and analytics needs evolve. It's essential to choose a BI solution that can handle increasing data volumes and complexity without compromising performance. Here are some factors to consider:
- Scalability: Can the BI system grow with your business?
- Ease of use: Is the interface user-friendly for all skill levels?
- Integration capabilities: Does the BI system work well with existing systems?
By focusing on these integration aspects, businesses can ensure that their BI systems provide a solid foundation for data-driven decision-making. Enhanced operational efficiency and a competitive edge are the rewards for those who successfully implement these tools.
Choosing the right BI tools involves evaluating various options against specific business needs and user capabilities. Factors such as data visualization, ease of use, and compatibility with current systems are crucial. Once the right tools are selected, designing logical data models and intuitive dashboards becomes the next step in harnessing the full potential of BI for strategic advantage.
Data Quality Management for Reliable Insights
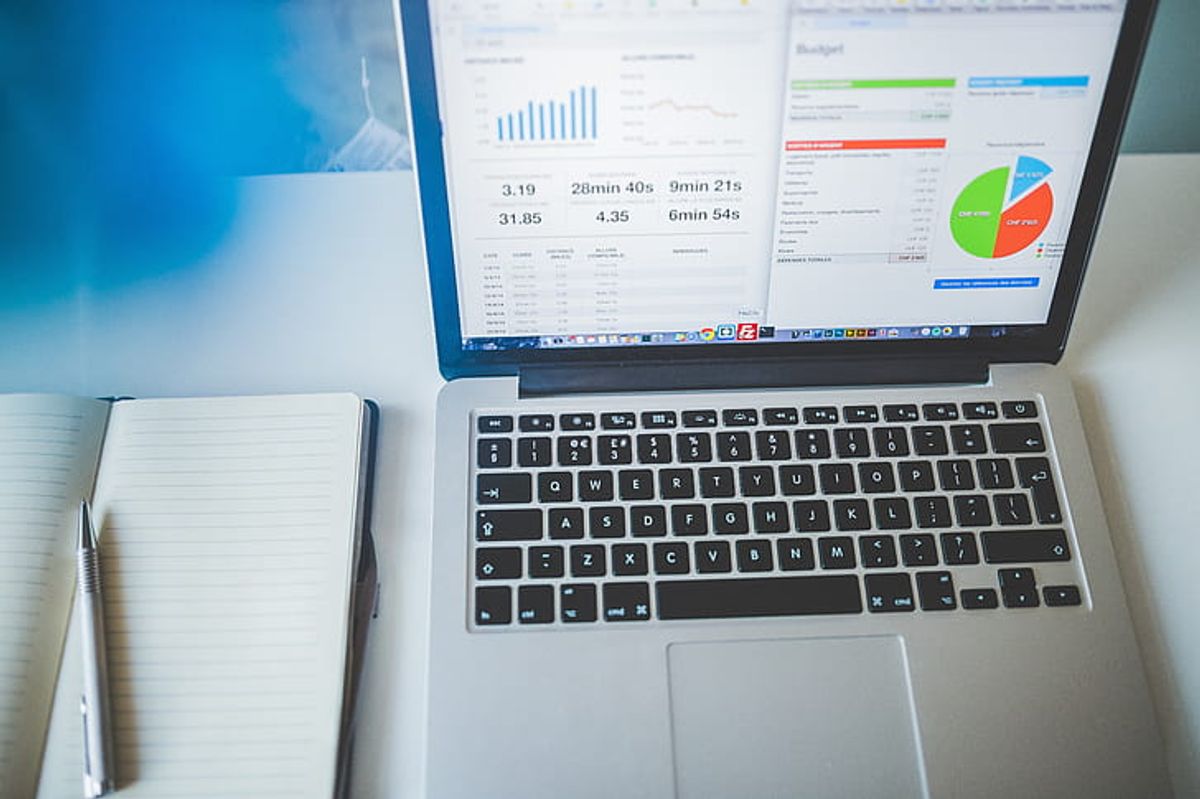
Ensuring Data Accuracy and Consistency
In the realm of business analytics, ensuring data accuracy and consistency is paramount. Data validation involves checking incoming data against predefined rules and criteria to ensure it meets quality standards. This could include verifying data formats, ensuring mandatory fields are not empty, and checking for logical consistency in data sets.
Implementing robust validation and verification strategies is essential to ensure data integrity in your pipeline.
Data cleansing is another critical step in improving data quality within your pipeline. This process involves identifying and rectifying data inaccuracies, inconsistencies, and redundancies. Start by removing duplicate entries to avoid skewed analysis and employ feasible automation tools to streamline this process.
Here are some best practices to maintain data quality:
- Regularly apply verification strategies as data flows through the pipeline.
- Unify date formats and categorize similar data types for consistency.
- Cross-verify data against reliable sources to address inaccuracies.
- Automate the cleansing process for continuous and efficient data management.
Data Cleaning Techniques for Improved Analysis
Data cleansing is a critical step in improving data quality within your pipeline. This process involves identifying and rectifying inaccuracies, inconsistencies, and redundancies. Start by removing duplicate entries to avoid skewed analysis. Address inaccuracies by cross-verifying data against reliable sources and correcting any errors found.
Standardize data formats to ensure consistency across the dataset. For instance, unify date formats, categorize similar data types, and align disparate data sets. Employ feasible automation tools to streamline this process, ensuring continuous and efficient data cleansing. Regularly implementing these techniques is critical to maintaining the integrity and reliability of data throughout its lifecycle in the pipeline.
Before enhancing the data quality in your pipeline, it's crucial to assess its current state. Begin by examining the data for accuracy, completeness, and consistency. Utilize tools to analyze data patterns, identify anomalies, and flag data deviating from established norms. This assessment should also review how current data management practices impact data quality, including data entry processes and automated data collection methods.
Identifying the primary sources of poor data quality is essential. Whether it's through human error or flawed data collection methods, understanding these sources can guide the implementation of effective data cleaning techniques. Here's a simple list to get started:
- Examine data for accuracy, completeness, and consistency.
- Analyze data patterns and identify anomalies.
- Flag data that deviates from established norms.
- Review and improve data entry and collection processes.
Data Governance Practices for Compliance
In the realm of business analytics, effective data governance is the cornerstone of ensuring compliance with regulatory requirements and maintaining the integrity of data-driven decisions. By implementing robust data governance frameworks, businesses can adhere to data privacy rules and uphold the highest standards of data ethics.
Data stewardship and security are integral to these frameworks, with a focus on prioritizing data quality for informed decision-making. It is not only about adhering to the present regulations but also about being prepared for future legislative changes. Descriptive analytics plays a role in examining past data for insights, while predictive analytics is leveraged to forecast future trends and ensure readiness for what lies ahead.
To maintain a competitive edge, it is essential to continuously monitor and refine data governance practices. This includes establishing clear data quality standards, regularly auditing data, and validating its accuracy and consistency.
Here are some best practices for ongoing data quality management:
- Establish a culture of continuous monitoring and improvement.
- Train staff on the importance of data accuracy and consistency.
- Encourage proactive reporting of data issues.
- Foster collaboration between teams to maintain high standards throughout the data pipeline.
Optimizing Business Processes through Analytics
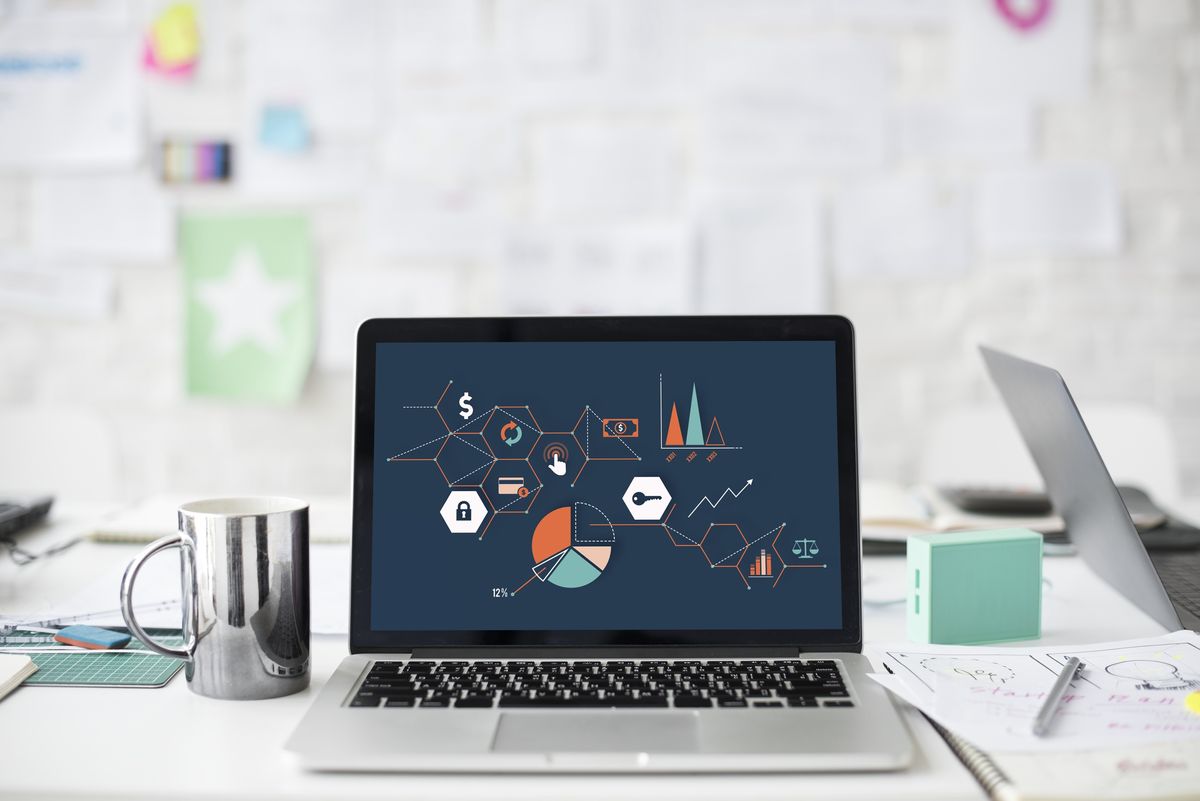
Identifying Process Bottlenecks with Data
In the pursuit of operational excellence, identifying and addressing process bottlenecks is crucial. Data analytics plays a pivotal role in pinpointing these inefficiencies. By analyzing workflow data, businesses can detect areas where processes slow down or become less effective. This analysis often reveals bottlenecks that, once resolved, can significantly improve overall performance.
To effectively identify bottlenecks, consider the following steps:
- Collect data from various stages of the process.
- Analyze the data to find patterns of delay or error.
- Prioritize issues based on their impact on the workflow.
- Implement solutions and monitor changes in process performance.
Ensuring that data is accurate and timely is essential for a true representation of process efficiency. Without high-quality data, efforts to streamline operations may be misguided.
Once bottlenecks are identified, it's important to address them systematically. Solutions may involve process redesign, technology upgrades, or changes in resource allocation. Continuous monitoring is key to ensuring that improvements are sustained and that new bottlenecks do not emerge.
Streamlining Operations for Efficiency
In the pursuit of operational excellence, streamlining operations for efficiency is paramount. By integrating data analytics into business strategy, companies can enhance their operational efficiency and foster a data-driven culture. This ongoing process requires investment in both tools and talent to transform business operations.
- Make production and purchasing decisions in real time
- Prevent stockouts and overstocking
- Improve supply chain efficiency
These steps are essential for businesses aiming to reduce waste and optimize resource allocation. The adoption of green technology and practices not only supports environmental sustainability but also contributes to a leaner, more cost-effective operation.
Consistency across the dataset is crucial. Employ automation tools to streamline processes, ensuring continuous and efficient data cleansing.
By focusing on these areas, companies can achieve a more agile and responsive operational framework, ready to adapt to market demands and customer needs.
Continuous Improvement through Data Feedback
In the realm of business analytics, continuous improvement is pivotal. By leveraging automation, organizations can ensure that data quality processes are not only efficient but also consistently adhere to high standards. This approach minimizes human error and maintains the integrity of the data pipeline.
Best practices in data quality management involve establishing a culture of ongoing monitoring and improvement. Teams should be trained in recognizing the importance of data accuracy and consistency, and a proactive stance should be taken in reporting and addressing data issues. Collaboration is key in sustaining high data quality standards.
Enhancing data quality is an ongoing commitment that requires regular assessment, cleansing, validation, and verification. By embedding these practices into the organizational culture, data becomes a more reliable and valuable asset for decision-making.
Despite the clear advantages, the path to effective data-driven decision-making (DDDM) is fraught with challenges. Data quality and integrity are paramount; decisions can only be as sound as the data upon which they are based. Robust data management and continuous investment in data governance are essential to overcome these hurdles.
In today's fast-paced business environment, leveraging analytics to streamline your operations can be a game-changer. At OptimizDBA, we specialize in DATABASE OPTIMIZATION CONSULTING, ensuring your data processes are not just faster, but smarter. With our proprietary methodology and a track record of significantly boosting performance, we're the partners you need to surge ahead of the competition. Don't just take our word for it; our clients, like Radio-Canada Inc., have experienced the transformative impact of our expertise. Ready to unlock the full potential of your business processes? Visit our website and let's discuss how we can elevate your data strategy to the next level.
Conclusion
In conclusion, the power of data analytics in business decision-making cannot be overstated. By leveraging data insights, organizations can make informed decisions, identify trends, and drive strategic initiatives. Embracing a data-driven culture and investing in the right technologies are crucial steps towards unlocking the full potential of data-driven decision-making. The rewards of enhanced strategic agility, improved operational efficiency, and competitive advantage make the journey towards data-driven decisions well worth the effort.
Frequently Asked Questions
How can business analytics help in strategic planning?
Business analytics can provide valuable insights and data-driven strategies for setting long-term goals, implementing growth strategies, and measuring performance metrics to support decision-making.
What are the key data visualization techniques for effective communication?
Key data visualization techniques include creating impactful dashboards, utilizing infographics for data representation, and employing interactive data storytelling approaches to communicate insights effectively.
How can predictive analytics contribute to future forecasting?
Predictive analytics can contribute to future forecasting by utilizing machine learning algorithms, forecasting trends and patterns, and implementing risk assessment and mitigation strategies based on data analysis.
What are the ethical considerations in data-driven decision-making?
Ethical considerations include ensuring data privacy and security, addressing bias in data analysis, and maintaining transparency in data usage to uphold ethical standards in decision-making processes.
How can business intelligence tools be implemented for competitive advantage?
Business intelligence tools can be implemented for competitive advantage by utilizing AI for market analysis, enabling real-time data monitoring for quick decisions, and integrating BI systems for seamless operations.
Why is data quality management important for reliable insights?
Data quality management is important for reliable insights as it ensures data accuracy and consistency, employs data cleaning techniques for improved analysis, and follows data governance practices to comply with regulations and standards.
How can business processes be optimized through analytics?
Business processes can be optimized through analytics by identifying process bottlenecks with data, streamlining operations for efficiency, and fostering continuous improvement through data feedback to enhance operational performance.
What is the significance of data-driven decision-making in business?
Data-driven decision-making in business harnesses the power of data to guide strategic decisions, offering a clear path to unlocking business potential by providing a more objective foundation for critical business choices rooted in data and empirical evidence.