The Strategic Value of Data Analytics
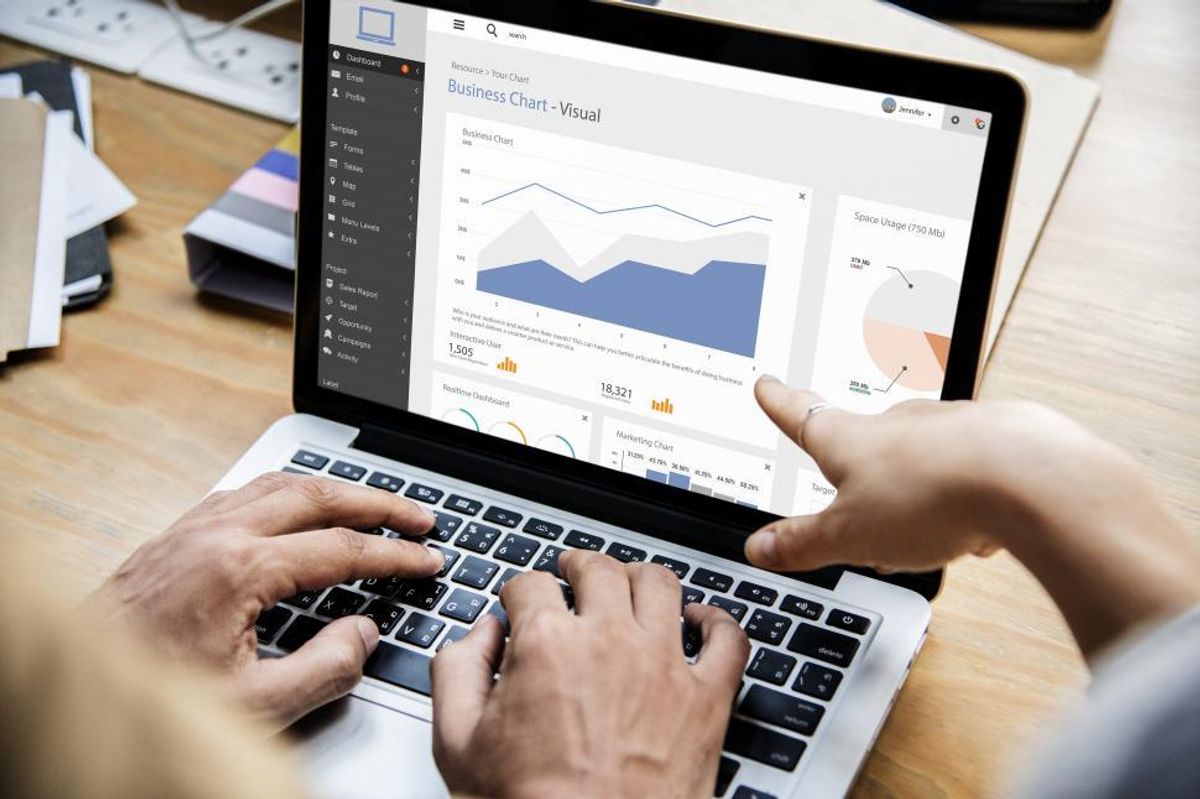
Driving Informed Decision-Making
In the realm of business, data-driven decision making is paramount, serving as the backbone for strategic actions that propel companies forward. By leveraging analytics, organizations can distill vast amounts of data into actionable insights, ensuring that every decision is supported by empirical evidence rather than mere intuition.
The systematic analysis of data not only clarifies the path to business growth but also instills a level of certainty in an otherwise unpredictable market.
While the benefits are clear, the path to effective data-driven decision making is fraught with challenges. Ensuring the integrity and quality of data is essential, as the value of decisions hinges on the accuracy of the underlying data. This necessitates robust data management and a commitment to data governance.
The universal value of data-driven decision making is evident across all business domains. It has the power to not only streamline operations but also to enhance customer experiences through deeper insights and personalized services. For businesses aiming to harness the full potential of their data, the imperative is to embrace a culture that prioritizes data-driven strategies.
Streamlining Operational Efficiency
Operational efficiency is a cornerstone of business success, and data analytics plays a pivotal role in enhancing this aspect. By analyzing workflow data, companies can identify bottlenecks and implement solutions to streamline processes, ultimately boosting productivity.
Automation is a key strategy in improving operational efficiency. For instance, integrating chatbot support can significantly reduce customer wait times by addressing common questions instantly, freeing up human specialists for more complex issues. This not only reduces functional expenses but also ensures a consistent and responsive customer experience.
Integrating data analytics into business strategy is an ongoing process. Invest in tools and talent, foster a data-driven culture, and enhance operational efficiency for business transformation.
Here are some practical steps to consider:
- Simplify processes to reduce customer effort.
- Automate manual tasks to increase efficiency and speed.
- Implement chatbot support to manage common customer queries.
- Automate billing or shipping notifications to reduce complaints.
Achieving a Competitive Edge
In the race to stay ahead of the competition, data analytics plays a pivotal role. It's not just about having data; it's about transforming that data into actionable insights that can drive strategic decisions and create a sustainable competitive advantage.
- Early adopters of AI and analytics are often the frontrunners in their industries.
- By analyzing market patterns and customer needs, businesses can respond swiftly and effectively.
- Predictive maintenance and product innovation are direct outcomes of data-driven strategies.
By leveraging customer insights and analytics, companies can stay attuned to evolving market demands and customer preferences, ensuring they are always one step ahead.
This data-driven decision making enables businesses to make informed choices, reducing reliance on guesswork and intuition. The strategic use of analytics fosters a culture of continuous improvement and adaptability, essential for maintaining a competitive edge in today's fast-paced market.
Harnessing Customer Insights for Growth
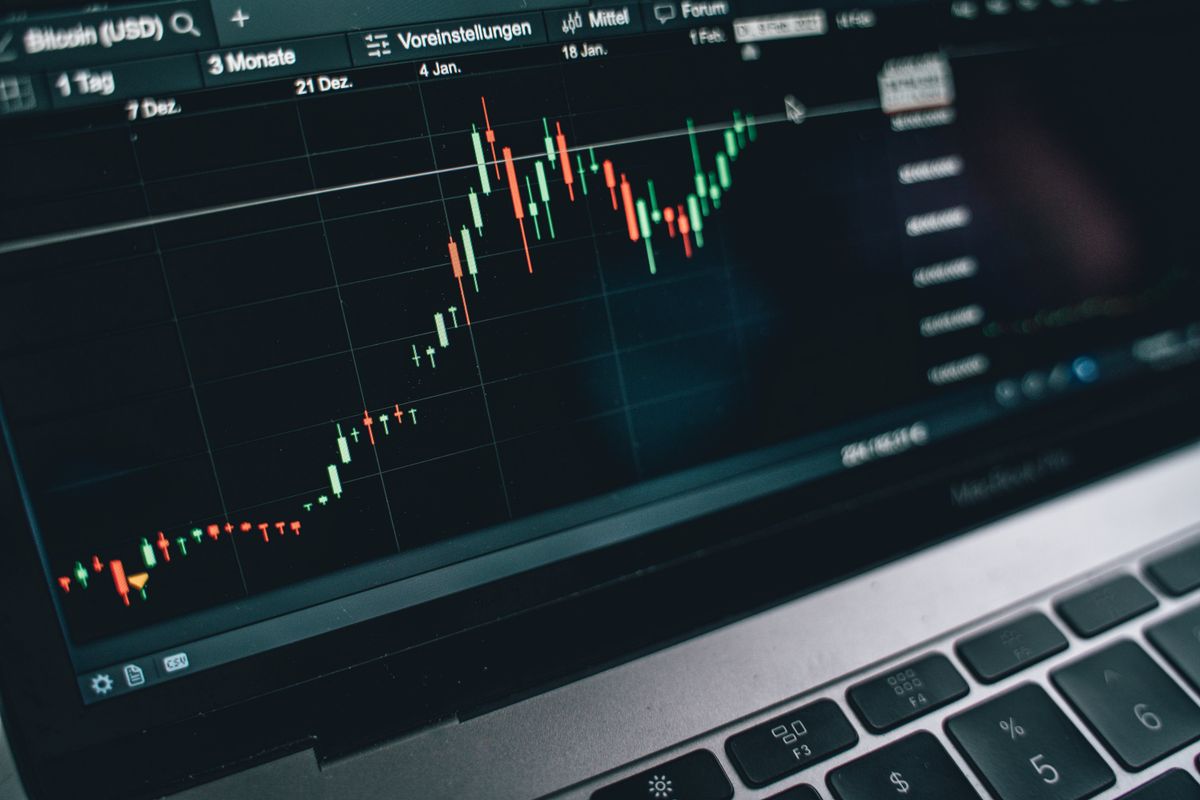
Understanding Consumer Preferences
In the dynamic landscape of consumer markets, data analytics stands as a pivotal tool for understanding and predicting customer behavior. Retailers, in particular, are turning to sophisticated analytic techniques to stay ahead in 2024, despite the challenges in predicting consumer behavior. By analyzing patterns and trends, businesses can tailor their offerings to meet the evolving demands of their customers.
Consumer preferences can be complex and multifaceted, but through the strategic use of data, companies can decipher the nuances of customer desires. Conducting surveys and focus groups remains a staple in gathering qualitative insights, while the integration of AI technologies enhances the depth and accuracy of data interpretation.
The acceptance and adoption of AI-based technologies not only depends on their perceived usefulness but also on the investment in capital and education to fully leverage their potential.
To effectively harness customer insights for growth, it is essential to consider various factors that influence consumer behavior:
- Perceived usefulness and ease of use
- Investment in capital and education
- Data quality and organizational resources
- Digital business model integration
Understanding these elements allows companies to not only meet but exceed consumer expectations, ultimately driving growth and competitiveness in the market.
Enhancing Customer Retention
Customer retention is pivotal for sustainable company growth. Leveraging data analytics can significantly improve customer loyalty by providing insights into customer behavior and satisfaction. Surveys are a powerful tool to gauge customer satisfaction and identify areas for improvement. By analyzing survey data, companies can pinpoint drop-off points in the customer journey and implement targeted strategies to enhance the overall experience.
Customer experience is not static; it requires continuous refinement. Data-driven insights enable businesses to set clear goals and track progress, ensuring that strategies are effectively converting insights into tangible actions. A well-structured rewards program, informed by customer data, can further incentivize repeat business and foster brand loyalty.
The key to enhancing customer retention lies in the ability to transform data into actionable strategies that resonate with customers and address their needs.
Here are some steps to consider for improving customer retention:
- Send out customer satisfaction surveys regularly.
- Analyze feedback to identify critical points in the customer journey.
- Implement changes to address identified issues.
- Introduce a rewards program tailored to customer preferences.
- Continuously monitor and adjust strategies based on ongoing data analysis.
Personalizing Marketing Strategies
In the realm of marketing, personalization is the key to unlocking customer engagement and loyalty. By leveraging analytics to understand individual preferences and behaviors, companies can tailor their marketing efforts to resonate with each customer. Personalization is not just about addressing the customer by name; it's about delivering relevant content at the right time, creating a unique experience for each individual.
Personalization has proven to be incredibly effective at driving sales and increasing ad ROI. A study by Google reveals that 90 percent of leading marketers acknowledge the significant impact of personalization on business growth. To implement a successful personalization strategy, consider the following steps:
- Identify key customer segments based on data analysis.
- Develop targeted marketing campaigns for each segment.
- Utilize A/B testing to refine and optimize personalized content.
- Continuously gather and analyze customer feedback to enhance personalization efforts.
Embracing personalization in marketing strategies is not just a trend; it's a transformative approach that can lead to substantial growth. Companies that prioritize personalized experiences are more likely to see an increase in customer satisfaction and retention, ultimately driving revenue impact.
Optimizing Business Operations with Analytics
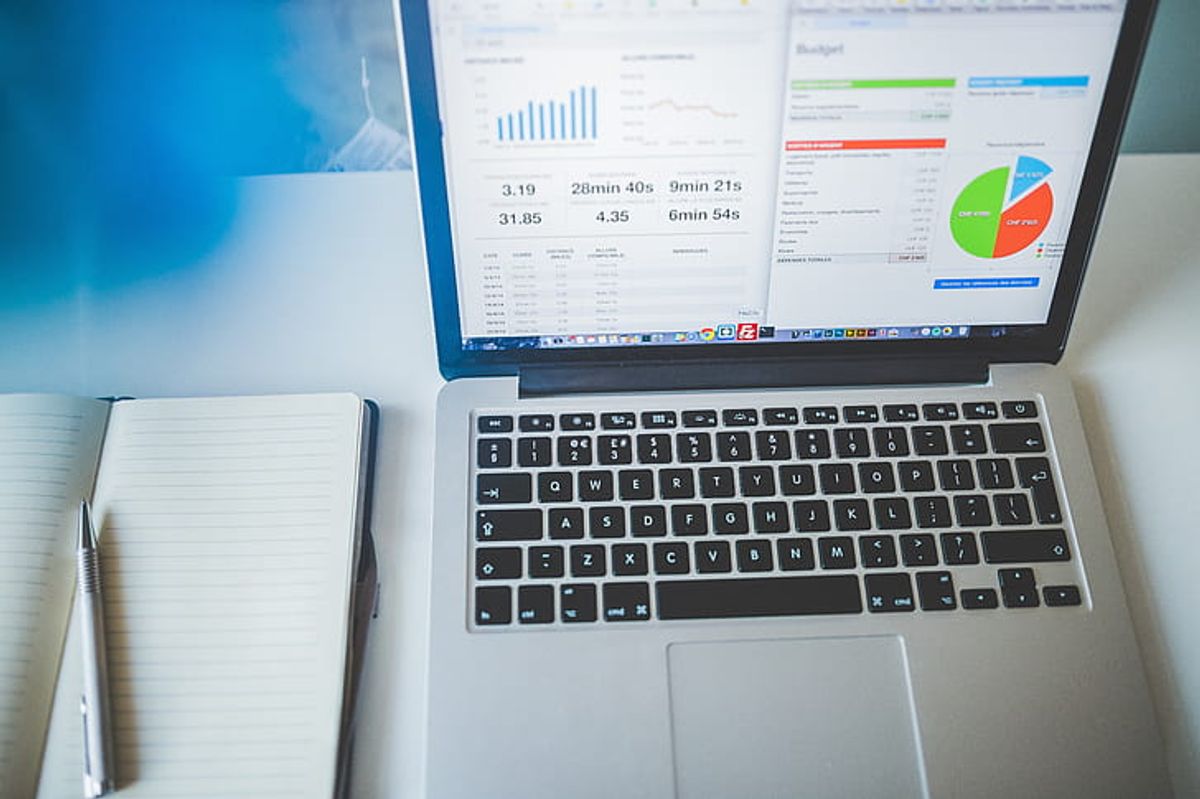
Improving Production Processes
In the realm of production, data analytics plays a pivotal role in enhancing efficiency and quality. By analyzing production data, companies can identify bottlenecks and areas for improvement, leading to more streamlined operations. For instance, data can reveal patterns in machine usage that suggest the need for maintenance or adjustments to prevent downtime.
By leveraging analytics, businesses can not only react to issues but also anticipate them, ensuring that production processes are continuously optimized.
Here are some key benefits of using data analytics in production:
- Predictive maintenance: Using historical data to forecast when machines will require servicing, thus preventing unexpected breakdowns.
- Quality control: Monitoring production in real-time to detect deviations from quality standards, allowing for immediate corrective actions.
- Resource optimization: Allocating resources more effectively based on data-driven insights into production demands and workflow efficiencies.
It's essential to not only gather and analyze the data but to also implement changes based on these insights to realize tangible improvements in production processes.
Predicting Equipment Maintenance
The integration of analytics into equipment maintenance has revolutionized the way businesses approach their operational health. Predictive maintenance strategies, powered by machine learning algorithms, enable companies to anticipate and address potential issues before they escalate into costly downtimes. By analyzing historical data and identifying patterns, these systems can forecast when maintenance is needed, ensuring that equipment operates at peak efficiency.
- Assess current maintenance schedules
- Implement predictive models
- Schedule maintenance proactively
- Monitor results and adjust as necessary
Predictive maintenance not only enhances the reliability of machinery but also optimizes the allocation of resources, leading to significant cost savings and improved operational performance.
To fully harness the benefits of predictive maintenance, it is crucial to have a clear understanding of the objectives and to choose the right tools and partners. Advanced analytics and automation play a pivotal role in this process, streamlining operations and contributing to overall efficiency.
Reducing Operational Costs
Data analytics serves as a powerful tool for reducing operational costs by identifying inefficiencies and areas where expenses can be trimmed without sacrificing quality. By analyzing patterns in data, companies can pinpoint wasteful practices and implement more cost-effective solutions.
Operational efficiency is not just about cutting costs; it's about optimizing resources to achieve better outcomes with less. For instance, predictive maintenance analytics can forecast when equipment is likely to fail, allowing for repairs before costly breakdowns occur. Similarly, process mining can reveal bottlenecks in workflows that, once addressed, can significantly lower operational expenses.
By leveraging data analytics, businesses can make strategic decisions that not only reduce costs but also enhance overall operational performance.
Here are some key areas where analytics have proven effective in cost reduction:
- Streamlining supply chain management
- Optimizing inventory levels
- Reducing energy consumption
- Minimizing downtime through predictive maintenance
- Automating repetitive tasks to free up human resources
Leveraging Market Analytics for Strategic Planning
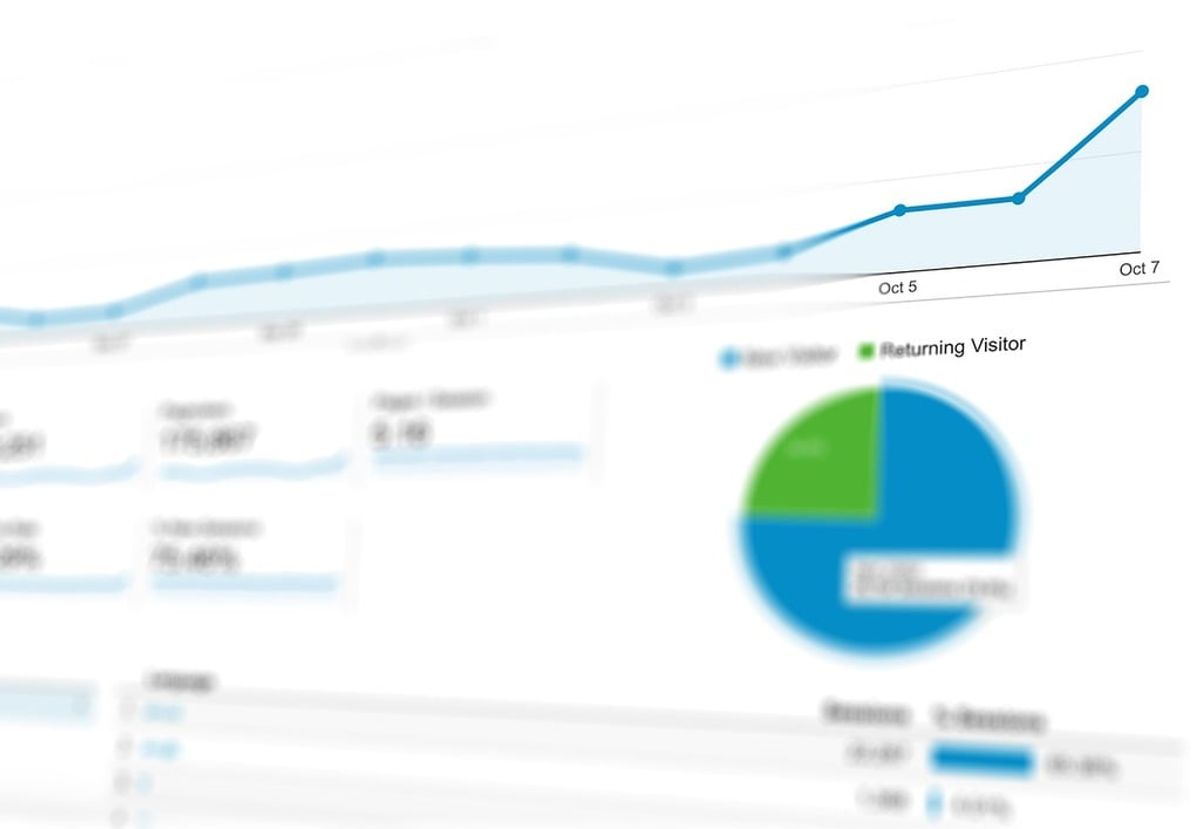
Identifying Market Trends
In the dynamic landscape of business, identifying market trends is pivotal for staying relevant and competitive. Utilizing data analytics, companies can detect patterns and shifts in consumer behavior, enabling them to anticipate changes and strategize effectively.
One effective strategy is to leverage trend discovery tools which track subtle yet significant movements within an industry. For instance, Exploding Topics is a platform that uncovers under-the-radar trends, providing businesses with early signals of emerging shifts.
By staying attuned to these trends, organizations can pivot their offerings to align with evolving market demands, ensuring they are not left behind as new preferences emerge.
Understanding these trends is not just about observing the present; it's about forecasting the future. Companies that excel in this area are often the ones that lead their industries, shaping the market rather than just responding to it.
Adapting to Consumer Demands
In today's dynamic market, adapting to consumer demands is crucial for business growth. By leveraging customer analytics, companies can uncover growth opportunities and ensure they meet the evolving needs of their customers. A data-driven approach allows businesses to be nimble, adaptable, and customer-centric.
Customer insights play a pivotal role in shaping business strategies. Through surveys, focus groups, and social media monitoring, businesses can gather valuable feedback and adjust their offerings accordingly. This continuous loop of feedback and improvement is essential for staying relevant in a competitive landscape.
By putting the customer in the driver's seat, businesses can make informed decisions that lead to increased satisfaction and loyalty. Happy customers are the cornerstone of a thriving business, and their feedback can unlock the door to new opportunities and sustained growth.
To effectively adapt to consumer demands, consider the following steps:
- Keep learning from customer feedback and market research.
- Innovate and improve products and services based on insights.
- Test and optimize strategies using performance metrics.
- Balance quantitative data with qualitative human perspectives.
Embracing these practices will help businesses stay ahead of the curve and shape a prosperous future.
Forecasting Future Market Developments
In the realm of business analytics, forecasting future market developments stands as a pivotal function that can significantly influence strategic planning. By leveraging predictive analytics and machine learning models, businesses can transition from merely reacting to market changes to proactively shaping their strategies. This shift not only enhances decision-making but also positions companies to capitalize on upcoming opportunities.
Forecasting is not just about predicting the future; it's about creating a roadmap for sustained growth. The following list outlines the key benefits of forecasting:
- Anticipating consumer trends and preferences
- Identifying potential new markets or products
- Adjusting supply chain management to meet future demand
- Allocating resources more effectively
By understanding and acting on these forecasts, businesses can align their operations with future market conditions, ensuring they remain competitive and agile in a rapidly changing environment.
While the benefits are clear, the process of forecasting is complex and requires a deep understanding of both historical data and current market dynamics. Companies must invest in the right tools and talent to harness the full potential of market forecasting.
Data Analytics as a Catalyst for Innovation
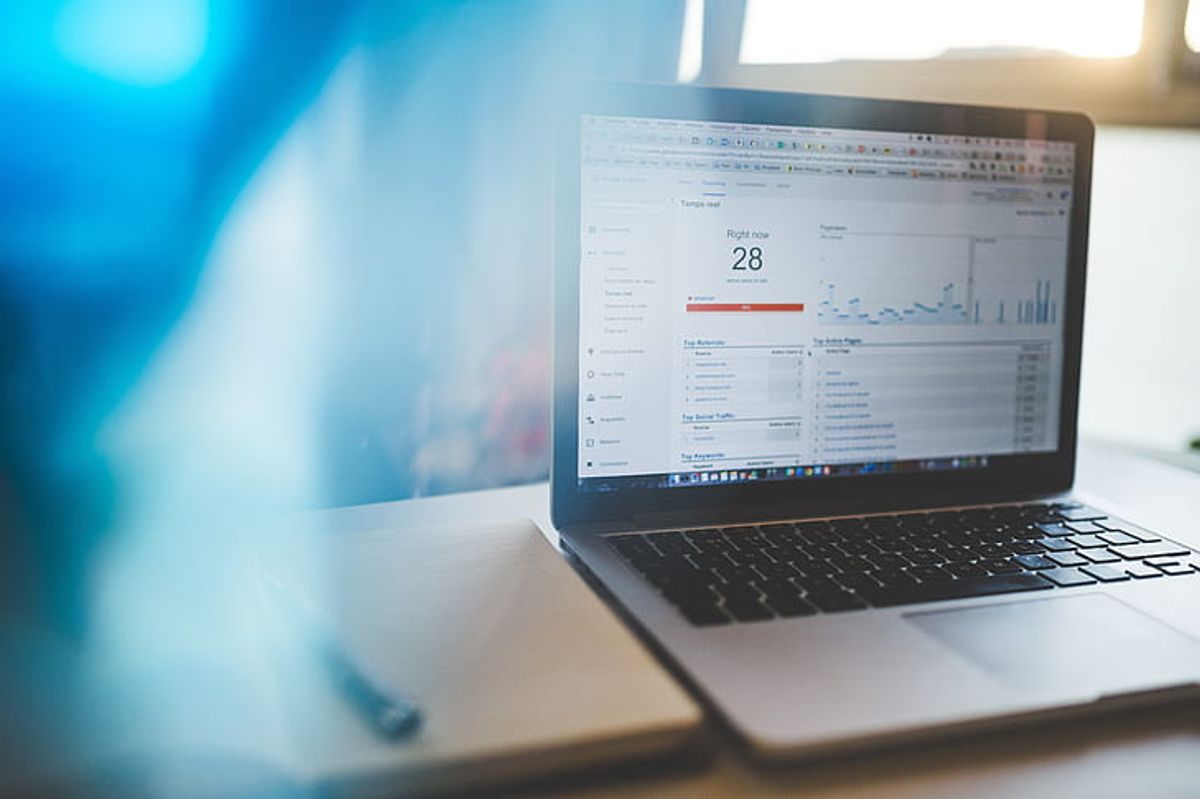
Fostering a Data-Driven Culture
To truly become a data-driven organization, you need to foster a culture that values data and insights. This starts at the top with executives who understand the power of data to drive better decision making and business outcomes. They must communicate a clear vision for becoming data-driven and empower teams to use data in their day-to-day work.
Investing in data literacy is crucial for building a data-driven culture. Employees at all levels should be equipped with the skills to understand and analyze data. This commitment to continuous learning and improvement enables teams to test, fail fast, and adapt based on the data.
A data-driven culture depends on continuous learning and improvement.
Leaders should encourage experimentation and frame failures as learning opportunities, not reasons to assign blame. With time and practice, data-driven decision making will become second nature. Here are some steps to guide the process:
- Assess Your Current Data Maturity Level
- Secure Leadership Buy-in
- Invest in the Right Infrastructure
- Build Data Literacy
Encouraging Experimentation and Creativity
In the realm of data analytics, fostering a culture of experimentation is crucial. Teams that embrace a growth mindset are more likely to innovate and drive the company forward. Leaders play a pivotal role in this process, by encouraging team members to test new ideas and view failures as valuable learning experiences rather than setbacks.
- Encourage risk-taking within the bounds of strategic goals.
- Promote a safe environment for sharing creative ideas.
- Implement rapid prototyping and iterative development.
By embedding these practices into the organizational fabric, companies can ensure that creativity and experimentation become a part of their DNA, leading to sustained innovation and growth.
Regular review and refinement of these experimental approaches are essential. This iterative process helps in fine-tuning strategies and maintaining a competitive edge in the ever-evolving business landscape.
Identifying New Business Opportunities
In the quest to identify new business opportunities, data analytics plays a pivotal role. By leveraging customer analytics, companies can unearth a wealth of growth areas. Analyzing customer insights, behaviors, and feedback reveals opportunities to enhance service and increase revenue.
- Analyze customer demographics: Identify untapped audience segments.
- Evaluate customer feedback: Discover areas for product or service improvement.
- Monitor market trends: Stay ahead by adapting to emerging demands.
By embracing data analytics, businesses can not only adapt to current market conditions but also anticipate and shape future trends.
Furthermore, data analytics enables businesses to identify market trends and customer preferences, which are crucial for developing effective business strategies. This insight-driven approach ensures that companies can enter new markets and extend their offerings, thereby driving revenue and market share.
The Impact of Big Data on Decision-Making
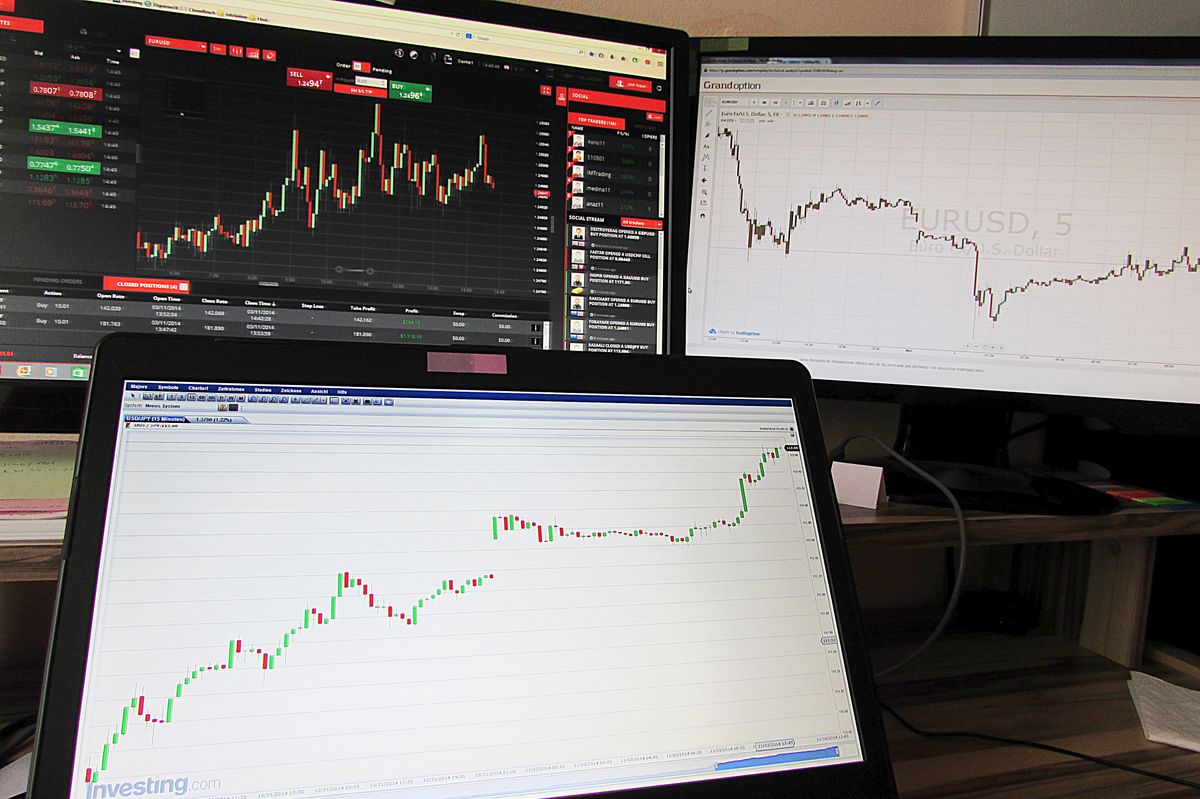
Enhancing Decision Quality
In the realm of business, data analytics serves as a cornerstone for enhancing decision quality. By dissecting vast amounts of information, companies can uncover hidden patterns and insights that lead to more informed and strategic choices. This process not only bolsters the accuracy of decisions but also minimizes the risks associated with uncertainty.
Analytics is pivotal in transforming raw data into actionable intelligence. The following points illustrate how analytics contributes to decision-making:
- Identifying key performance indicators (KPIs) to track success
- Analyzing customer behavior to tailor products and services
- Assessing market trends to stay ahead of the competition
- Predicting future outcomes to prepare proactive strategies
The integration of analytics into decision-making processes ensures that businesses are equipped to navigate the complexities of a dynamic marketplace. It is a powerful tool that drives growth and efficiency, ultimately leading to a competitive advantage.
Businesses leverage data analytics for quality improvement, decision-making, risk assessment, and personalized customer experiences. These elements are crucial in a landscape where agility and precision are paramount to success.
Accelerating the Decision-Making Process
In today's dynamic business landscape, accelerating the decision-making process is paramount. By leveraging data analytics, organizations can swiftly interpret vast amounts of information, leading to quicker and more effective decisions. The integration of analytics into decision-making processes not only speeds up these processes but also enhances their accuracy.
- Rapid data analysis allows for immediate response to market changes.
- Data-driven insights enable proactive problem-solving.
- Real-time analytics facilitate on-the-fly strategic adjustments.
The agility afforded by accelerated decision-making gives businesses the ability to adapt quickly and maintain a competitive stance in the ever-evolving market.
Furthermore, the use of predictive analytics tools can forecast future trends, allowing companies to stay one step ahead. This proactive approach minimizes risks and capitalizes on opportunities, ultimately driving growth and innovation.
Utilizing Predictive Analytics for Proactive Strategies
The integration of predictive analytics into business operations marks a transformative shift from reactive to proactive management. By analyzing historical data, businesses can forecast future trends and adapt their strategies in advance. This foresight is not just about avoiding risks; it's about seizing opportunities that lie ahead.
Predictive analytics empowers organizations to anticipate market changes, optimize resource allocation, and enhance customer experiences. It's a tool that, when used effectively, can redefine the competitive landscape by enabling companies to act before the curve, rather than simply reacting to it.
Predictive analytics is not a crystal ball, but it provides the next best thing: a data-driven glimpse into the future.
The benefits of utilizing predictive analytics are manifold, including improved risk management, fraud detection, and a deeper understanding of customer behavior. Here's a snapshot of how predictive analytics can be applied across different business areas:
- Risk Management: Mitigate potential threats before they materialize.
- Fraud Detection: Identify and prevent fraudulent activities proactively.
- Customer Interaction: Engage with customers based on predictive insights.
By harnessing the power of predictive analytics, businesses are not only enhancing their decision-making processes but are also paving the way for innovative growth strategies.
Integrating Advanced Analytics and AI
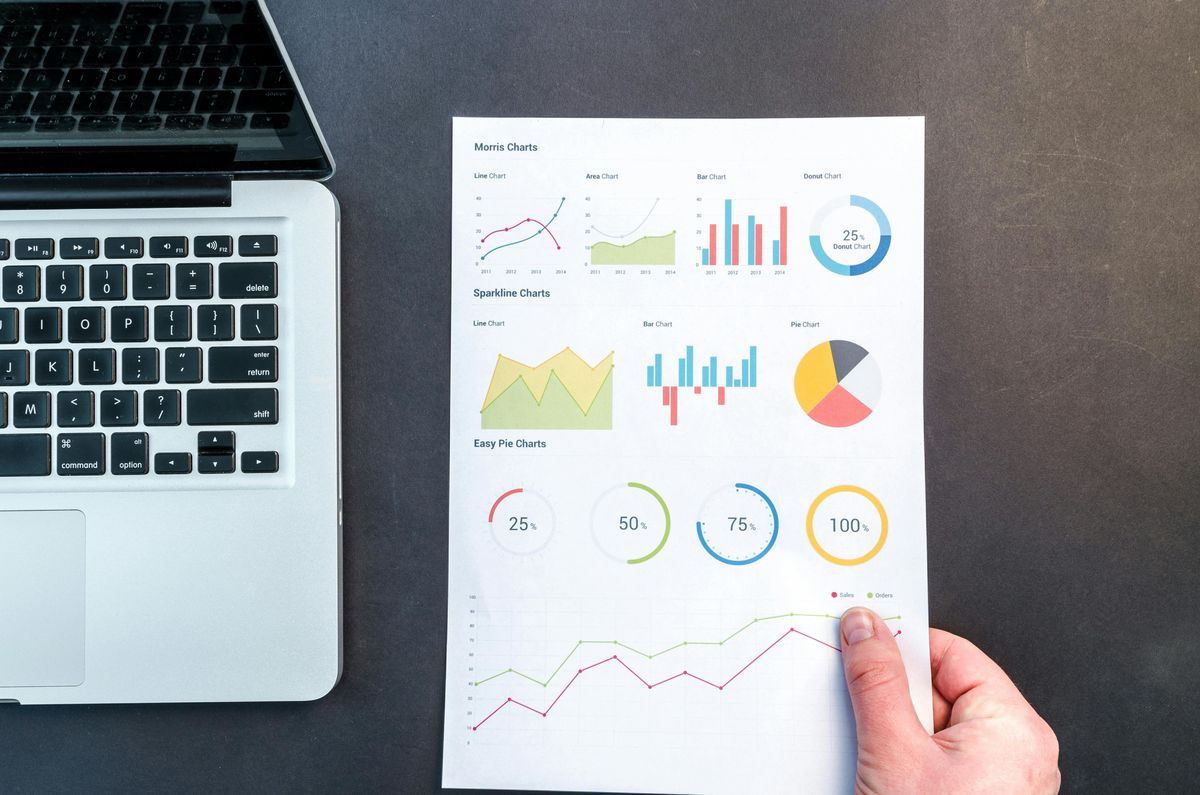
Improving Predictive Capabilities
The integration of artificial intelligence (AI) with predictive analytics is revolutionizing the way businesses forecast and plan for the future. Machine learning algorithms, which adapt and improve over time, are at the forefront of this transformation. They enable organizations to transition from a reactive stance to a proactive approach, effectively anticipating market shifts and customer needs before they occur.
The strategic deployment of AI technologies has led to remarkable outcomes, including enhanced decision-making and the opening of new market opportunities.
Here are some of the key benefits of improving predictive capabilities:
- Anticipating customer behavior to tailor products and services
- Identifying potential risks and mitigating them in advance
- Optimizing supply chain management to prevent shortages or overstock
It's important to note that the successful implementation of AI in predictive analytics requires a well-defined strategy. Companies must choose the right tools and partners to harness the full potential of these advanced technologies.
Automating Data-Driven Processes
The integration of advanced analytics and artificial intelligence (AI) has revolutionized the way businesses approach data-driven decision-making (DDDM). Automation in DDDM not only increases efficiency but also ensures consistency in executing complex analytical tasks. By automating routine data analysis, companies can allocate human resources to more strategic activities, enhancing overall productivity.
Automation is particularly effective in areas where data is voluminous and decisions need to be made rapidly. For instance, in customer service, automated processes can analyze customer interactions to identify trends and suggest improvements. This leads to a more dynamic and responsive business environment, where data-driven revenue generation becomes a pivotal aspect of digital transformation.
The use of data to drive decision making has a positive impact on business performance, enabling organizations to deliver efficient services and improve managerial decisions.
Here are some benefits of automating data-driven processes:
- Consistent and error-free data analysis
- Rapid response to market changes
- Enhanced ability to predict customer behavior
- Streamlined operational workflows
As businesses continue to embrace DDDM, the role of automation will become increasingly important, ensuring that data is not just collected, but also effectively utilized to drive growth and innovation.
Gaining Deeper Insights from Complex Data
In the realm of data analytics, the integration of advanced technologies like Artificial Intelligence (AI) has revolutionized the way businesses interpret complex datasets. By leveraging AI, companies are now able to unearth patterns and correlations that were previously obscured by the sheer volume of information. This enhanced capability to analyze data leads to more nuanced and comprehensive insights, which are critical for strategic decision-making.
The ability to gain deeper insights from complex data is not just about having the right tools; it's about asking the right questions and knowing how to interpret the answers.
For instance, AI-driven analytics can provide a granular understanding of customer behavior, enabling businesses to tailor their offerings and improve customer experiences. Additionally, predictive models can forecast demand with greater accuracy, informing inventory management and resource allocation. The table below illustrates the impact of AI analytics on various business aspects:
Business Aspect | Without AI | With AI |
---|---|---|
Customer Insights | Surface-level | Deep and actionable |
Demand Forecasting | General trends | Precise predictions |
Strategic Planning | Reactive | Proactive |
By decoding the complexities of big data, organizations can transform information into actionable intelligence, driving growth and maintaining a competitive edge in today's data-driven landscape.
Measuring the ROI of Data Analytics Initiatives
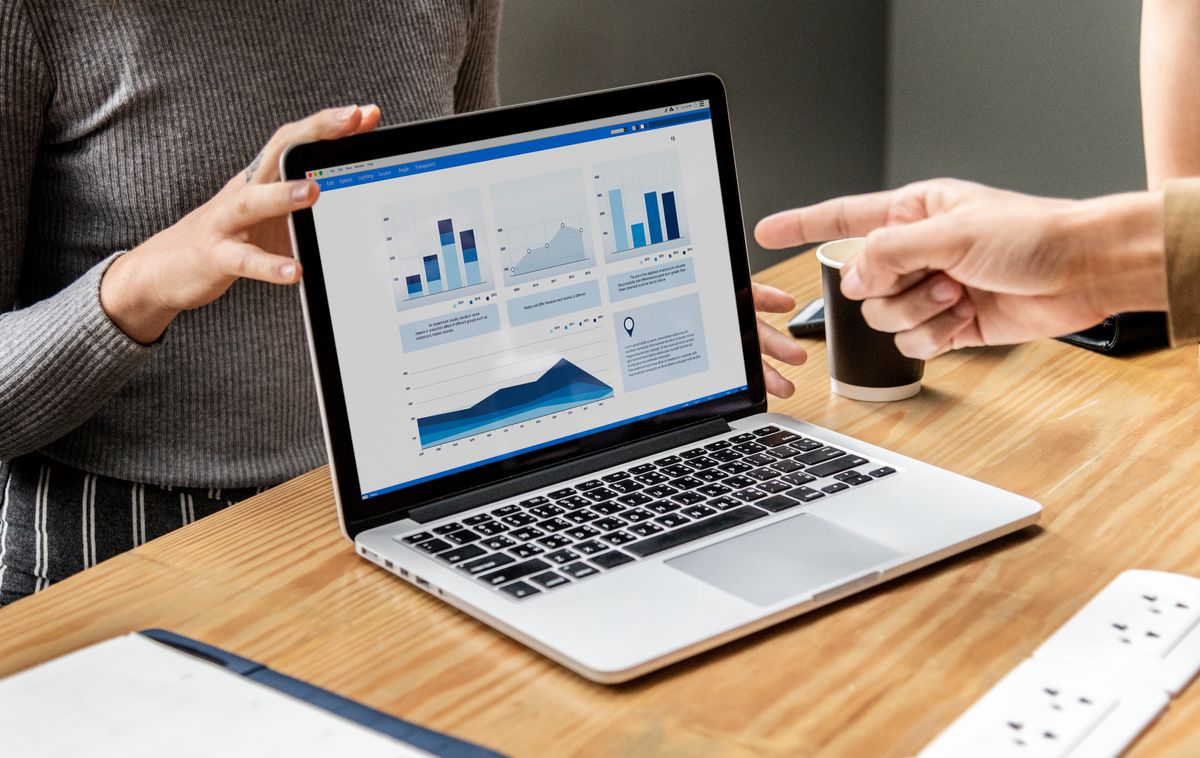
Quantifying the Impact on Revenue Growth
The democratization of data within organizations has paved the way for more agile decision-making, which is crucial for driving business growth. By measuring the impact of analytics on revenue, companies can prioritize features and strategies that maximize this growth potential.
By leveraging customer analytics, businesses uncover new growth opportunities, identifying areas to better serve customers and consequently boost revenue.
Understanding the direct correlation between analytics initiatives and revenue increase is essential. A structured approach to quantify this impact involves:
- Tracking and prioritizing features that maximize revenue impact.
- Tailoring roadmap visibility to align with public plans or private strategies.
- Converting customer conversations and feedback into actionable product insights.
Embracing analytics not only fosters innovation but also cultivates a data-driven culture that is a significant competitive advantage.
Assessing Cost Savings and Efficiency Gains
The integration of data analytics into business operations has proven to be a catalyst for cost savings and efficiency gains. By leveraging insights from data, companies can streamline processes, reduce waste, and optimize pricing strategies, leading to a more profitable bottom line.
Efficiency and productivity are at the heart of these improvements. For instance, AI in software development has enabled organizations to automate routine tasks, resulting in cost savings of up to 25%. This automation not only cuts down on manual labor but also accelerates the pace of work, allowing for quicker turnaround times and higher output.
The strategic application of data analytics facilitates a reduction in operational costs while enhancing customer satisfaction.
Here's a snapshot of the potential impact on efficiency and cost savings:
- Reduction in materials waste
- Improved product recall efficiency
- Enhanced customer satisfaction
- Optimized product pricing
By meticulously analyzing various data sources, such as sales and supply chain metrics, businesses can pinpoint areas ripe for improvement. This data-driven approach ensures that resources are allocated where they can generate the most significant return, ultimately leading to a sustainable competitive advantage.
Evaluating Customer Lifetime Value Improvements
Understanding and improving Customer Lifetime Value (CLV) is pivotal for long-term revenue growth. By evaluating CLV improvements, businesses can discern the effectiveness of their customer retention strategies. Investing in tools and talent is essential to foster a data-driven culture and iterate the analytics process.
Aligning analytics initiatives with business objectives is crucial. A structured approach to enhancing the customer experience can lead to increased loyalty and retention. For instance, sending surveys to gauge customer satisfaction and adjusting pricing strategies are actionable steps that can be taken based on data insights.
Enhancing the customer experience is an iterative process that requires turning insights into action. By setting concrete goals and monitoring progress, businesses can make meaningful changes that positively impact CLV.
Here is a simple framework to guide the evaluation of CLV improvements:
- Identify key customer touchpoints and assess satisfaction levels.
- Analyze the impact of loyalty programs on repeat purchases.
- Monitor changes in customer behavior following pricing adjustments.
- Implement feedback loops to refine business processes based on customer input.
Challenges and Solutions in Data-Driven Decision Making
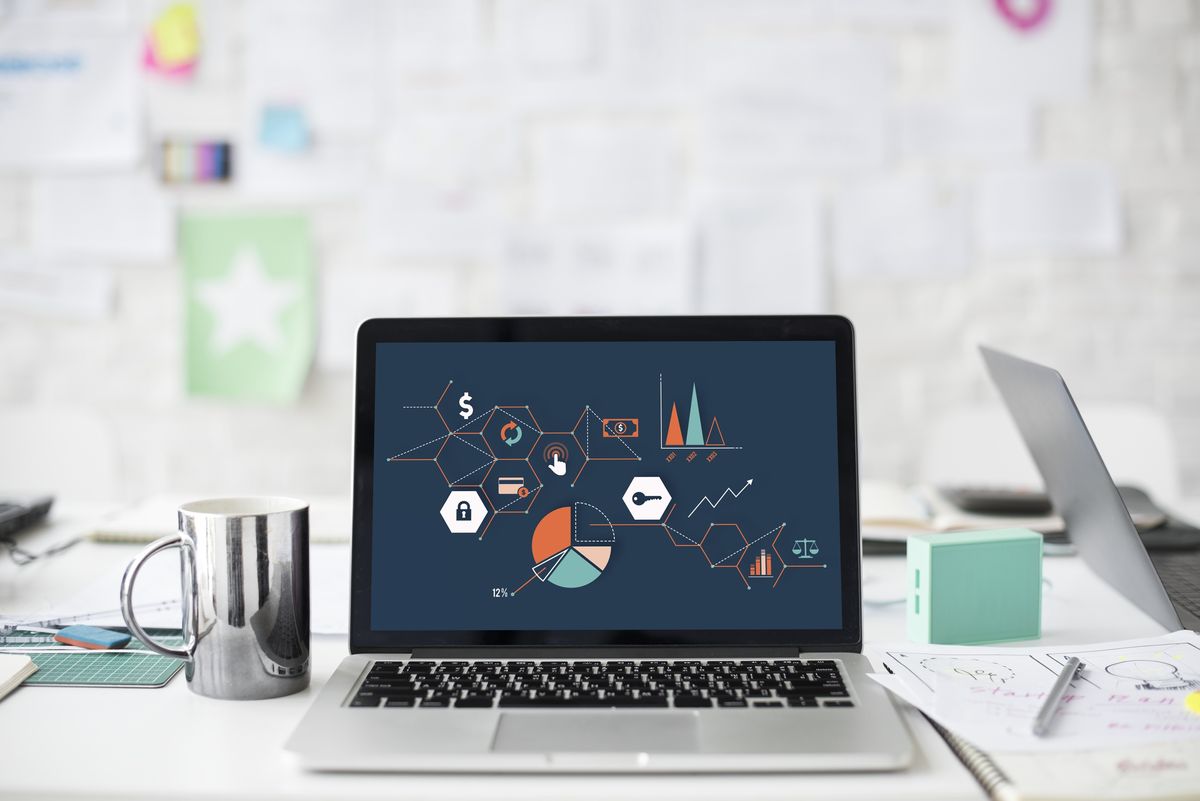
Overcoming Data Quality Issues
The strategic importance of data in decision-making is undeniable, yet data quality issues can significantly undermine this process. To ensure the integrity of data-driven decisions, businesses must adopt robust data management practices. These practices are not just about maintaining data; they're about enhancing its accuracy, consistency, and timeliness.
Effective Data Quality Management (DQM) is now at the forefront of business intelligence. It combines technology, processes, and the right people to deliver data that is accurate and useful. Here are some steps to address common data quality challenges:
- Establish a clear data governance framework.
- Implement regular data quality checks.
- Utilize advanced tools for data cleansing and validation.
- Foster a culture that values data integrity across the organization.
The goal is not to achieve perfect data, but to maintain a level of quality that ensures reliable analytics and business insights.
Poor data quality is not just an inconvenience; it represents a significant cost to businesses. In the US alone, it's estimated that poor data quality results in a loss of $3.1 trillion annually. This staggering figure highlights the need for ongoing investment in DQM to safeguard profitability and business value.
Ensuring Data Privacy and Security
In the digital age, data privacy and security are paramount for maintaining customer trust and complying with regulations. As data analytics becomes increasingly central to business operations, the need for robust security measures intensifies. Companies must prioritize protecting personal information to prevent data breaches that can lead to significant financial and reputational damage.
To ensure data security, organizations are adopting comprehensive strategies that include:
- Regular security audits and updates
- Employee training on data handling and privacy policies
- Implementation of advanced encryption methods
- Strict access controls and authentication protocols
The passage of regulations like GDPR and the California Consumer Protection Act has made data governance non-negotiable. Businesses are investing more in securing their data assets, recognizing that a breach could be catastrophic.
Having a dedicated team to drive data analytics initiatives is essential. This team should focus on data governance, ensuring the quality and security of data, while fostering a data-driven culture within the organization. Clear objectives and a balance between data-driven insights and intuition are key to leveraging analytics while maintaining privacy and security.
Bridging the Talent Gap in Data Science
The scarcity of skilled professionals in data analytics is a pressing challenge for businesses aiming to leverage data for growth. Data scientists and analysts are crucial for interpreting complex data and driving strategic decisions, yet they are in short supply. To address this, companies must be proactive in developing their workforce's capabilities.
Investing in data literacy is a foundational step. Training programs in data analysis, visualization, and storytelling empower employees to engage with data confidently, fostering a data-driven culture. Sharing success stories can further encourage the adoption of data-centric approaches.
Assessing your data readiness is vital. It helps identify gaps in your AI initiatives and is a critical step towards successful implementation.
Forming a multidisciplinary team that includes members with diverse skills can also help bridge the talent gap. This team can tackle various aspects of data science, from technical analysis to ethical considerations of data use.
Future Trends in Business Analytics
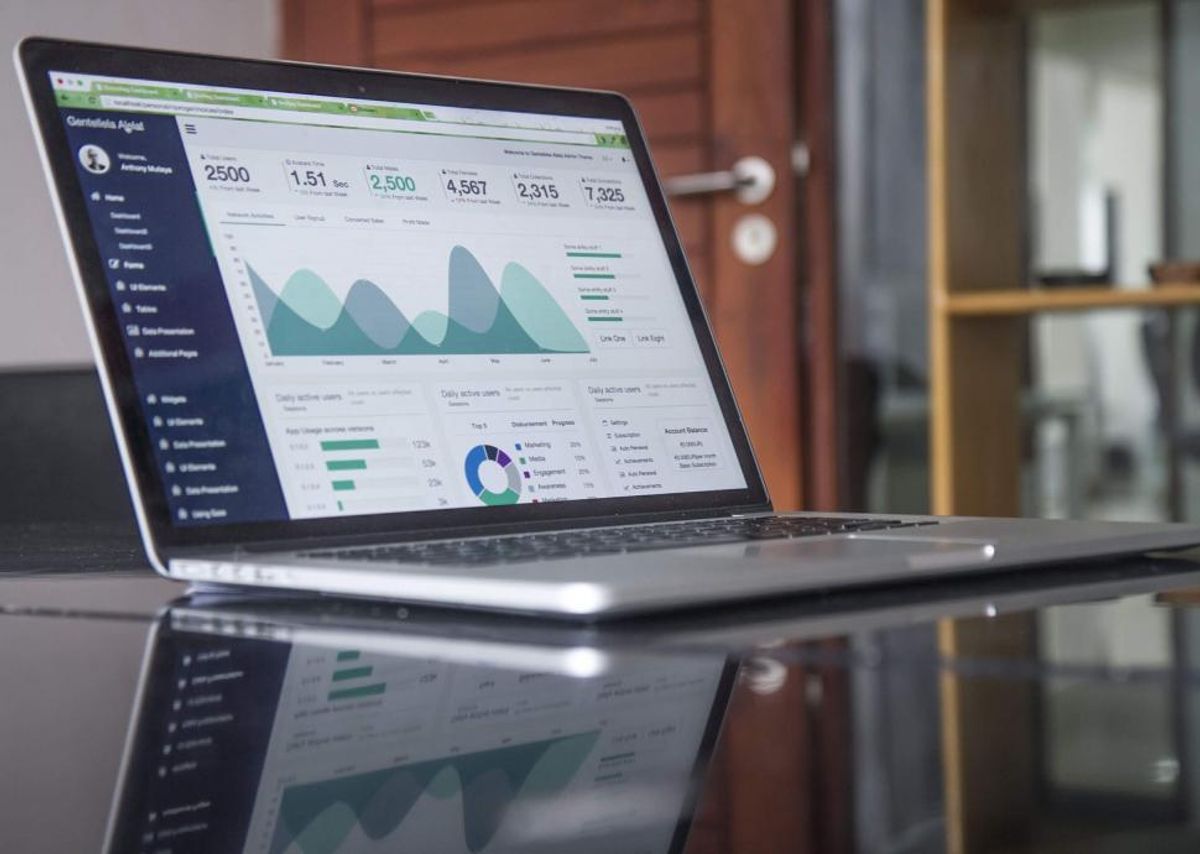
The Rise of Real-Time Analytics
In the fast-paced business environment, real-time analytics are becoming increasingly crucial. They enable organizations to make swift decisions based on the most current data available. This immediacy can be the difference between capitalizing on an opportunity and missing it entirely.
Real-time analytics drive revenue growth and help businesses overcome data management challenges by ensuring data quality, integrity, privacy, and security. By integrating these analytics into their operations, companies can respond to market changes with agility and precision.
The integration of real-time analytics into business processes is not just a trend; it's a transformative shift that empowers companies to act on insights as they unfold.
Here are some key benefits of real-time analytics:
- Immediate insight into customer behavior
- Enhanced ability to detect and respond to security threats
- Streamlined operational decision-making
- Improved customer experiences through timely personalization
The Integration of IoT with Analytics
The Internet of Things (IoT) has revolutionized the way we collect and analyze data. By integrating IoT with analytics, businesses can unlock a new dimension of insights, driving operational improvements and innovative services. Real-time data from IoT devices enables companies to respond swiftly to changing conditions, optimize resource allocation, and predict maintenance needs before issues arise.
The synergy between IoT and analytics paves the way for smarter decision-making and enhanced business models.
To fully capitalize on IoT analytics, organizations must navigate a complex ecosystem of devices, data formats, and platforms. A structured approach to this integration involves:
- Establishing a robust data infrastructure to handle the influx of IoT data.
- Implementing advanced analytics tools to process and interpret the data.
- Developing clear strategies to act on the insights gained.
As the IoT analytics market continues to grow, driven by advancements in data analytics and the integration of IoT with other technologies, businesses that embrace this trend will find themselves at the forefront of innovation.
The Evolution of AI in Data Analysis
The evolution of artificial intelligence (AI) in data analysis marks a significant milestone in the journey towards more sophisticated and efficient decision-making processes. AI's ability to process vast amounts of data with speed and accuracy has revolutionized the way businesses approach analytics. Predictive analytics, for instance, leverages AI to forecast market trends and consumer behavior, providing a crucial advantage in adapting to market shifts.
By integrating AI into their analytics frameworks, companies can enhance their agility and improve the accuracy of their predictions, leading to superior business outcomes.
The following list outlines key steps for businesses to maximize the benefits of AI in data analysis:
- Define clear problems that AI can address.
- Ensure high data quality for reliable AI outputs.
- Select appropriate AI models for specific tasks.
- Integrate AI seamlessly with existing systems.
- Consider ethical implications of AI applications.
- Scale AI initiatives gradually to manage risks.
- Regularly improve AI models and processes.
- Identify and utilize suitable AI techniques.
- Foster a culture of continuous learning and adaptation.
As AI continues to transform organizational operations, it enables a reduction in manual tasks and a significant boost in productivity. Companies that effectively harness the potential of AI in data analysis are poised to lead in innovation and strategic growth.
As the business world evolves, staying ahead of the curve in business analytics is crucial. At OptimizDBA, we pride ourselves on offering faster data solutions and unparalleled database optimization consulting. Our proprietary methodology has been trusted by over 500 clients since 2001, ensuring transaction speeds that leave competitors in the dust. Don't let your business fall behind; visit our website to learn how we can help you experience a significant increase in performance and stay at the forefront of future trends in business analytics.
Conclusion
In the journey of transforming data into strategic assets, businesses have witnessed a paradigm shift in decision-making. The insights gleaned from this article underscore the pivotal role of analytics in driving company growth. By harnessing the power of data analytics, organizations gain a comprehensive understanding of market trends, customer behavior, and operational efficiencies. This intelligence not only informs more accurate and timely decisions but also fosters a competitive edge that is essential in today's fast-paced market. As we have seen, the integration of advanced analytics and AI further propels businesses towards innovation, agility, and a sustained increase in performance. With a staggering 97% of businesses employing data analytics and its proven impact on revenue growth and customer retention, the message is clear: embracing data-driven decision-making is not just advantageous—it is imperative for future success and growth.
Frequently Asked Questions
How does data analytics enhance decision-making in businesses?
Data analytics provides insights, predictions, and actionable intelligence by analyzing data sets, leading to more informed and accurate decisions that improve business performance.
In what ways can data analytics streamline operational efficiency?
Data analytics helps businesses optimize production processes, predict equipment maintenance needs, and reduce operational costs, resulting in streamlined and efficient operations.
What competitive advantage can businesses gain from data analytics?
Businesses leveraging data analytics can outperform rivals by anticipating market trends, understanding customer behavior, and making timely, informed decisions.
How does analyzing customer data contribute to business growth?
Analyzing customer data allows businesses to understand preferences, improve customer retention, personalize marketing, and identify new growth opportunities.
What role does big data play in organizational decision-making?
Big data analytics significantly impacts decision-making by accelerating the process, enhancing decision quality, and providing a competitive edge through timely, informed strategies.
How can advanced analytics and AI transform business decision-making?
Advanced analytics and AI enable businesses to sift through vast data, identify patterns, automate processes, and extract actionable insights, leading to more strategic and effective decisions.
What are the key metrics for measuring the ROI of data analytics initiatives?
Key metrics include the impact on revenue growth, cost savings and efficiency gains, and improvements in customer lifetime value.
What future trends in business analytics should companies be aware of?
Companies should keep an eye on the rise of real-time analytics, the integration of IoT with analytics, and the evolution of AI in data analysis to stay competitive.