Unlocking Business Growth with Advanced Analytics
Overview
Introduction to advanced analytics
Advanced analytics is a powerful tool that allows businesses to gain valuable insights from their data. By utilizing advanced statistical techniques and machine learning algorithms, businesses can uncover hidden patterns and trends in their data that can drive decision-making and improve business performance. With the ability to analyze large volumes of data quickly and accurately, advanced analytics enables businesses to make data-driven decisions and stay ahead of the competition. In this article, we will explore the benefits of using advanced analytics in business and discuss key components of advanced analytics.
Benefits of using advanced analytics in business
Advanced analytics provides numerous benefits to businesses. One of the key benefits is the ability to make data-driven decisions. By analyzing large volumes of data, businesses can gain valuable insights that can inform their strategic planning and decision-making processes. Database management is another important benefit of advanced analytics. With advanced analytics tools, businesses can efficiently store, organize, and analyze large datasets, ensuring data accuracy and accessibility. Additionally, advanced analytics can help businesses identify trends and patterns in customer behavior, allowing them to tailor their products and services to meet customer needs. Overall, the use of advanced analytics in business can lead to improved efficiency, increased revenue, and a competitive advantage in the market.
Key components of advanced analytics
One of the key components of advanced analytics is Demystifying SQL. SQL, or Structured Query Language, is a programming language used for managing and manipulating relational databases. It allows businesses to extract valuable insights from large amounts of data by querying databases and retrieving specific information. By understanding and utilizing SQL, businesses can gain a competitive edge by uncovering hidden patterns and trends in their data. With the increasing availability of data and the growing importance of data-driven decision making, Demystifying SQL has become an essential skill for businesses looking to unlock the full potential of advanced analytics.
Data Collection and Preparation
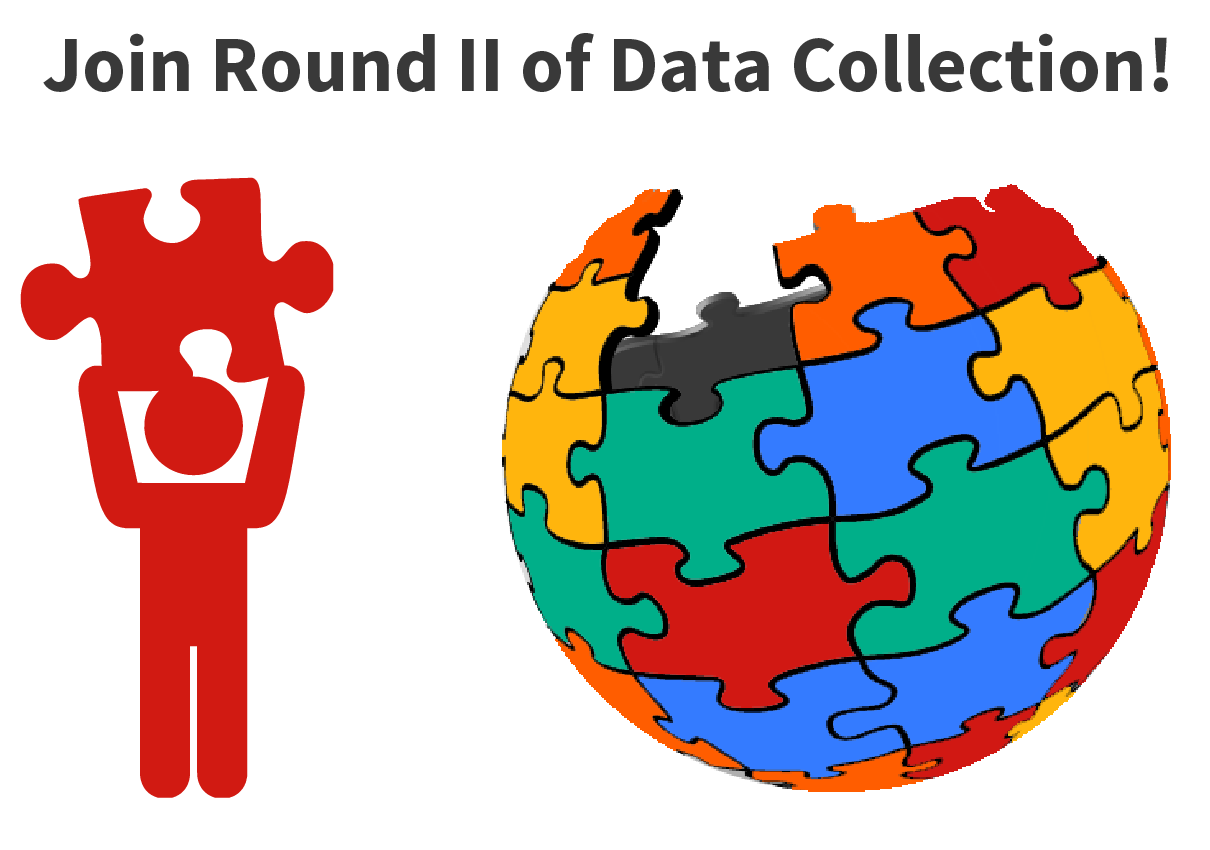
Importance of data collection
Data collection is a crucial step in the process of unlocking business growth through advanced analytics. Without accurate and comprehensive data, businesses cannot make informed decisions or identify valuable insights. Collecting relevant data allows organizations to gain a deeper understanding of their customers, market trends, and internal operations. It enables them to identify patterns, correlations, and anomalies that can drive strategic decision-making. Moreover, data collection is essential for SQL Server optimization as it provides the necessary inputs for improving database performance and efficiency. By collecting and analyzing data effectively, businesses can gain a competitive advantage and unlock new opportunities for growth.
Methods for collecting data
There are several methods available for collecting data in business. One of the most common methods is surveys, where businesses can directly ask customers or target audiences for information. Another method is observation, where businesses can gather data by observing customer behavior or market trends. Additionally, businesses can also collect data through web scraping, which involves extracting data from websites. Each method has its own advantages and disadvantages, and businesses should choose the method that best suits their needs and resources.
Data cleaning and preprocessing
Data cleaning and preprocessing are crucial steps in the data analysis process. Database management plays a key role in ensuring that the data is accurate, consistent, and reliable. It involves removing duplicate records, handling missing values, and standardizing data formats. Additionally, data preprocessing techniques such as normalization and feature scaling are applied to prepare the data for analysis. By effectively managing and preprocessing the data, businesses can improve the quality of their insights and make more informed decisions.
Data Analysis and Modeling
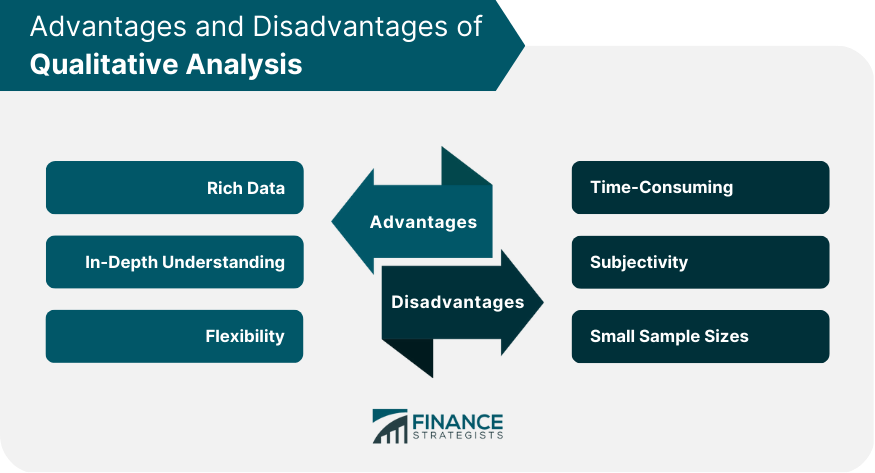
Exploratory data analysis
Exploratory data analysis is a crucial step in the advanced analytics process. It involves examining and visualizing the data to gain insights and identify patterns, trends, and outliers. During this stage, various statistical techniques and data visualization tools are used to explore the data and understand its characteristics. Exploratory data analysis helps in identifying data quality issues and understanding the relationships between variables. It also helps in identifying potential problems that may arise during the modeling phase. By analyzing the data, businesses can make informed decisions and uncover hidden opportunities for growth.
Statistical modeling techniques
Statistical modeling techniques are powerful tools for analyzing data and making predictions. These techniques involve the use of mathematical models to describe relationships between variables and to make inferences about the population from which the data was collected. By applying statistical models to business data, organizations can gain valuable insights into customer behavior, market trends, and other factors that can drive business growth. Some commonly used statistical modeling techniques include linear regression, logistic regression, and time series analysis. These techniques can help businesses identify patterns, forecast future outcomes, and make data-driven decisions.
Machine learning algorithms
Machine learning algorithms are a key component of advanced analytics. These algorithms enable businesses to make data-driven decisions by automatically learning patterns and making predictions from large datasets. There are various types of machine learning algorithms, including supervised learning, unsupervised learning, and reinforcement learning. Each type has its own strengths and applications. For example, supervised learning algorithms are used for classification and regression tasks, while unsupervised learning algorithms are used for clustering and dimensionality reduction. Reinforcement learning algorithms, on the other hand, are used for sequential decision-making problems. By leveraging machine learning algorithms, businesses can gain insights from their data and optimize their operations for improved performance and profitability.
Conclusion
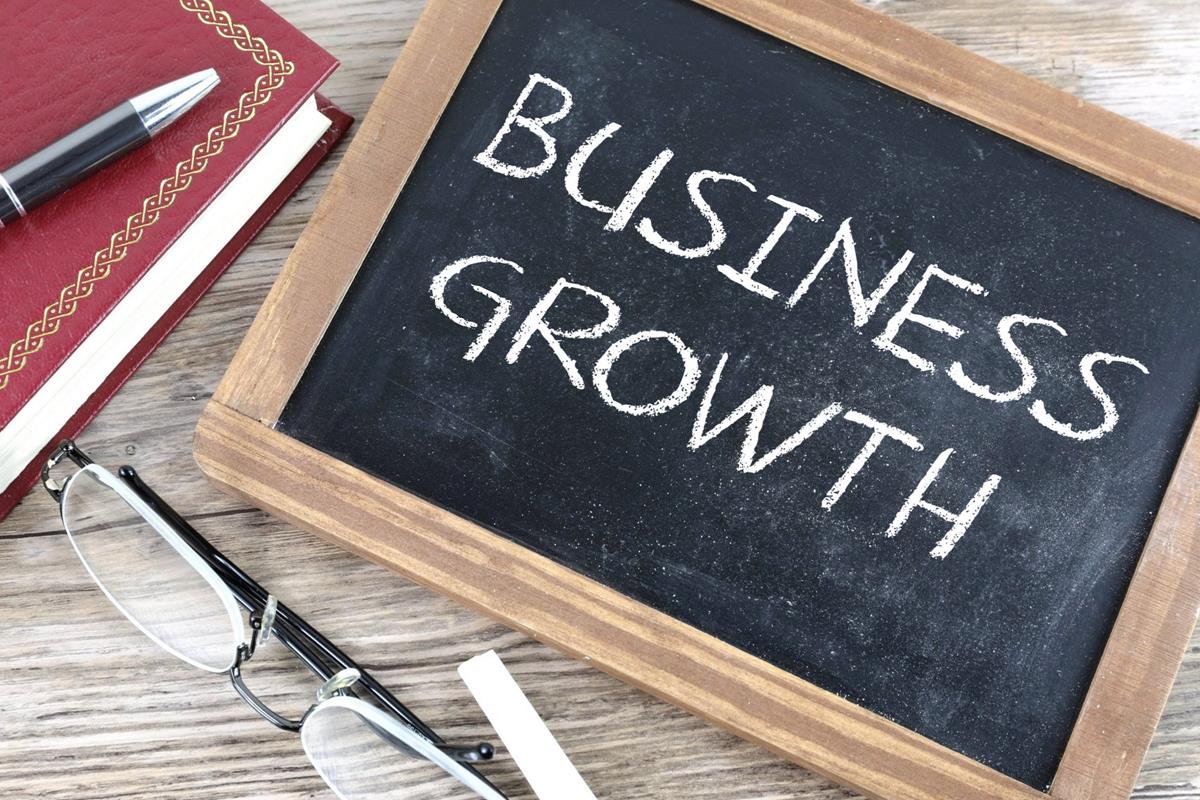
Summary of the benefits of advanced analytics
Advanced analytics provides businesses with valuable insights and enables data-driven decision making. By leveraging sophisticated techniques and algorithms, businesses can uncover hidden patterns, trends, and correlations in their data. This allows them to make more accurate forecasts, identify opportunities for growth, and mitigate risks. Additionally, advanced analytics empowers businesses to optimize their operations, improve customer satisfaction, and gain a competitive edge in the market. The use of business intelligence tools and technologies further enhances the capabilities of advanced analytics, enabling businesses to extract actionable insights from vast amounts of data.
Future trends in advanced analytics
As advanced analytics continues to evolve, there are several future trends that businesses should be aware of. One of these trends is the increasing importance of database maintenance. With the growing volume and complexity of data, maintaining a well-organized and efficient database is crucial for accurate analysis and decision making. Another trend is the emergence of real-time analytics, which enables businesses to gain insights and make decisions in the moment. Real-time analytics allows for faster and more proactive decision making, leading to improved business outcomes. Additionally, automated machine learning is expected to become more prevalent in the future. Automated machine learning tools can streamline the modeling process, making it easier for businesses to leverage advanced analytics without extensive technical expertise. These trends highlight the ongoing advancements in advanced analytics and the potential for businesses to unlock even greater growth and success.
Recommendations for implementing advanced analytics in business
To maximize the benefits of advanced analytics in business, it is crucial to implement SQL query tuning techniques. SQL query tuning involves optimizing the performance of SQL queries to improve the speed and efficiency of data retrieval. By fine-tuning the queries, businesses can reduce query execution time, enhance data processing capabilities, and improve overall system performance. This can lead to faster decision-making, better insights, and increased productivity. Implementing SQL query tuning as part of the advanced analytics strategy can help businesses unlock the true potential of their data and drive business growth.
In conclusion, OptimizDBA Database Optimization Consulting is the trusted industry leader in remote DBA services. With over 500 clients and a track record of delivering transaction speeds that are at least twice as fast as before, we guarantee a significant increase in performance. Our average speeds are often 100 times, 1000 times, or even higher! If you're looking to optimize your database and experience improved transaction speeds, contact OptimizDBA today. Visit our website to learn more about our services and how we can help you achieve optimal performance.