The Strategic Imperative of Advanced Analytics
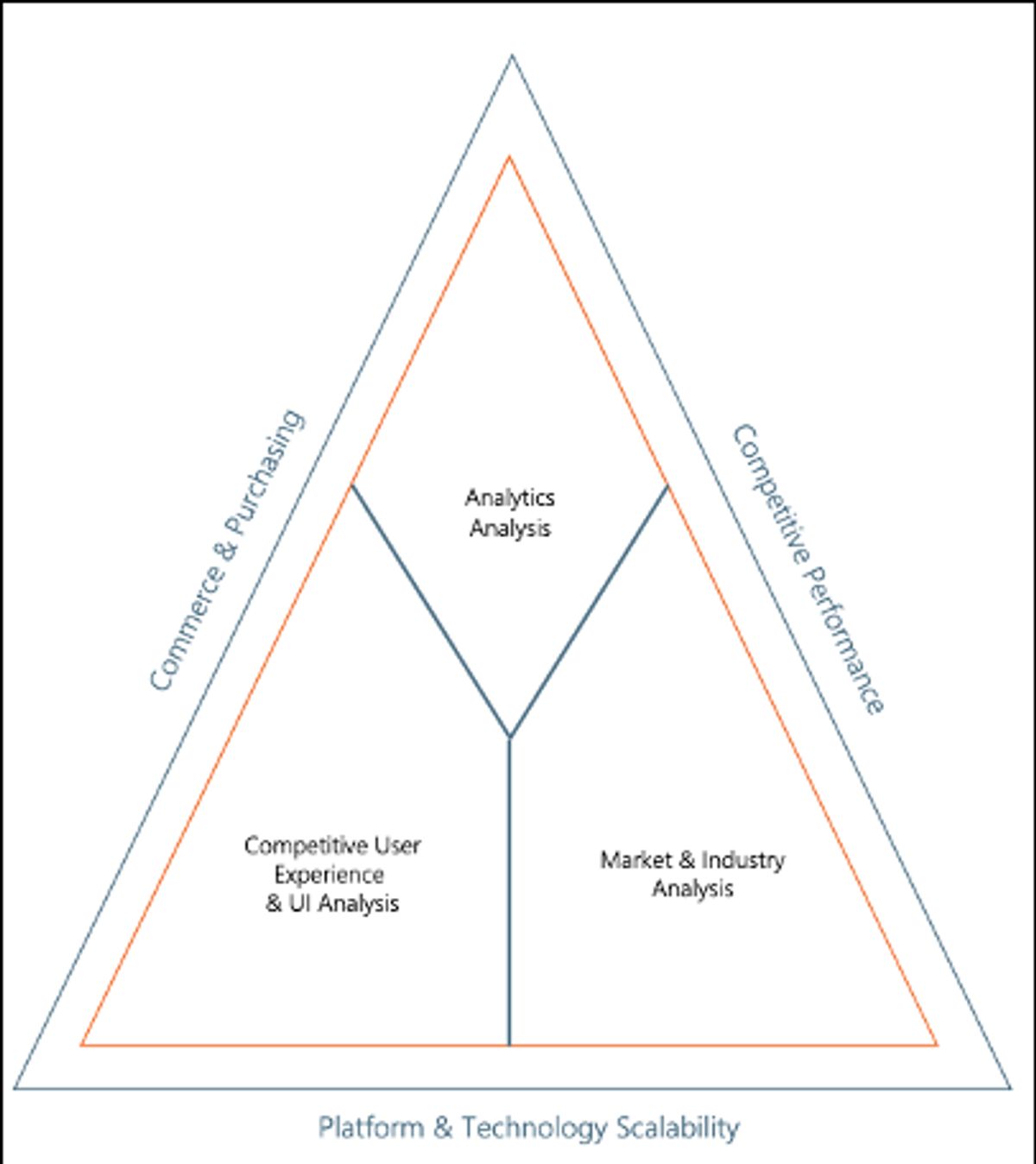
Fostering Data-Driven Culture
For organizations to innovate and remain agile, fostering a data-driven culture is essential. Employees across various teams and roles must be empowered to make informed decisions independently. This requires not only access to data but also the ability to interpret and utilize it effectively.
Data intelligence is crucial in promoting data literacy and democratizing access to insights. By treating data as a product and providing self-service tools and training, businesses can enable a self-sufficient decision-making environment.
A data-driven culture is not just about having data available; it's about embedding data into the core of business operations and decision-making processes.
Implementing a data-driven culture involves a systematic approach, including:
- Establishing clear messaging around the value of data-driven decisions
- Building a robust data ecosystem to support analytics
- Providing training to enhance data literacy across the organization
While the journey to a data-driven culture can be challenging, the rewards are substantial, leading to more innovative and agile business practices.
Integrating Analytics into Business Strategy
Integrating analytics into business strategy is not just about having access to data; it's about weaving insights into the very fabric of an organization's decision-making processes. Businesses that successfully embed analytics into their strategic planning are more likely to outperform their peers in operational efficiency and competitive positioning.
- Identify key performance indicators (KPIs) aligned with business objectives
- Develop a roadmap for analytics implementation
- Ensure cross-departmental collaboration and data sharing
- Continuously measure and refine analytics strategies
By making analytics a cornerstone of business strategy, companies can transform data into a strategic asset that drives growth, innovation, and a sustainable competitive edge.
Choosing the right analytics tools and fostering a collaborative environment are crucial steps in this integration. Organizations must also prioritize continuous learning and adaptation to keep pace with the evolving digital landscape and emerging business challenges.
Prioritizing Data Governance for Quality Insights
In the realm of advanced analytics, the integrity and reliability of data are paramount. Data governance is the cornerstone that ensures the quality and security of data used for business insights. It involves the establishment of policies, standards, and procedures that govern the collection, management, and usage of data across the organization.
Data Integration and governance are critical for synthesizing disparate data sources into a coherent and comprehensive view of the business landscape. This integration facilitates better understanding and decision-making, leading to strategic advantages in the marketplace.
- Data Quality: Implement measures to maintain accuracy and consistency.
- Data Security: Protect data assets from unauthorized access and breaches.
- Compliance: Adhere to regulatory requirements and ethical standards.
By prioritizing data governance, companies can ensure that their analytics efforts are built on a foundation of trustworthy data, enabling them to unlock strategic insights and drive enhanced decision-making.
Harnessing Real-Time Data for Agile Decision-Making
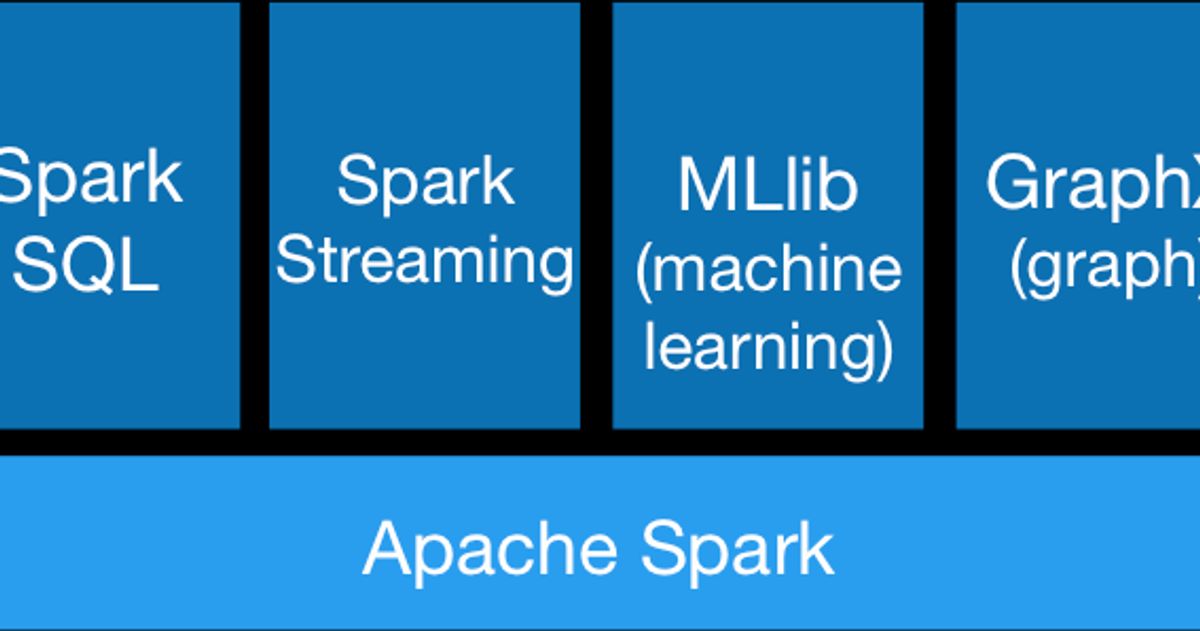
The Role of Real-Time Analytics in Business Agility
Real-time analytics is a transformative force in the business landscape, offering the ability to analyze data as it's generated. Businesses that leverage real-time analytics can make swift, informed decisions, adapting to market changes with unprecedented speed. This agility is not just about speed; it's about the capacity to harness insights that drive strategic action.
Instant insights from real-time analytics equip businesses with the power to monitor KPIs continuously, identify trends, and respond to customer behavior dynamically. The benefits are manifold:
- Improved Decision-Making: Quick access to data means decisions are no longer delayed by the lag of data processing.
- Increased Efficiency: Real-time data streamlines business processes, allowing for more efficient operations.
- Competitive Edge: The ability to act on immediate data insights can set a company apart from its competitors.
Embracing real-time analytics is essential for businesses aiming to thrive in a digital economy where agility and prompt action are key to maintaining a competitive advantage. The integration of technologies such as AI and Machine Learning, IoT Devices, and Data Visualization with real-time analytics is pivotal in fostering an environment of transparency and collaboration.
Overcoming the Challenges of Implementing Real-Time Data
Implementing real-time analytics is a strategic move for businesses aiming to enhance decision-making and maintain a competitive edge. However, the journey is fraught with challenges, such as minimizing latency in processing data streams. To overcome this, companies can employ solutions like in-memory computing and caching mechanisms, which are designed to deliver timely insights.
Latency is just one of the hurdles; others include integrating diverse data sources and ensuring data accuracy. Addressing these challenges is essential for leveraging the full potential of real-time analytics. Here are 8 solutions to common challenges:
- Utilizing in-memory computing
- Implementing caching mechanisms
- Integrating data from various sources
- Ensuring data accuracy and quality
- Scaling infrastructure to handle data volume
- Securing real-time data streams
- Developing a robust IT support system
- Fostering a culture that embraces real-time insights
Embracing AI and machine learning can significantly aid in analyzing data instantaneously, allowing organizations to respond to changing conditions with agility and informed precision.
Case Studies: Success Stories of Real-Time Decision-Making
Real-time analytics has revolutionized the way businesses operate, providing the agility needed to make decisions at the speed of the market. AI algorithms are at the forefront of this transformation, enabling rapid decision-making by processing incoming data streams in real time. This capability is crucial in dynamic environments where every second counts.
- Improved Decision-Making: Access to real-time insights allows for quick, data-driven decisions.
- Increased Efficiency: Organizations can streamline processes and optimize performance.
- Competitive Edge: Staying ahead by responding to market changes promptly.
For those seeking to enhance their decision-making and gain a competitive advantage, the implementation of real-time analytics is not just beneficial; it's imperative. > Don't wait for tomorrow to make decisions based on yesterday's data - leverage real-time analytics to steer your business towards success today!
Published findings highlight the success stories of businesses that have embraced real-time analytics. The CodeStringers report, titled "Data-driven companies: four compelling case studies," showcases how these organizations have not only survived but thrived by making swift, informed decisions.
Leveraging Machine Learning for Predictive Insights
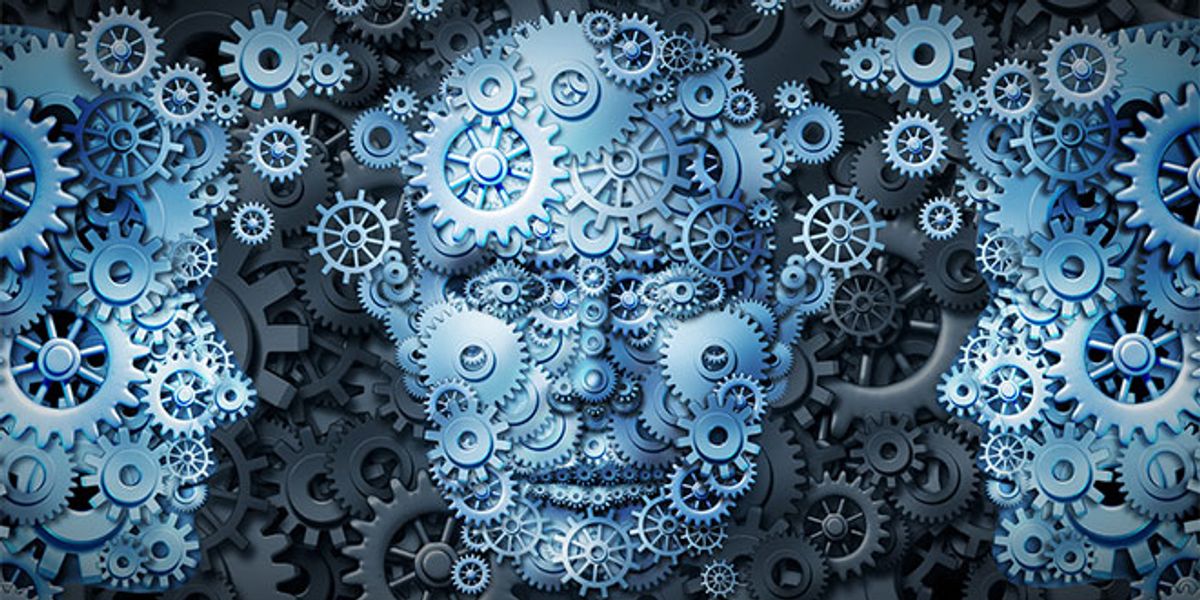
Improving Forecast Accuracy with Machine Learning
The integration of machine learning into forecasting models has revolutionized the way businesses predict future trends and outcomes. AI models are now at the forefront of forecasting accuracy, harnessing historical data patterns to provide predictive insights that are more precise than ever before.
Through continuous learning and adaptation, these models evolve, ensuring that each forecast is informed by the latest data. This iterative process is exemplified by solutions like the one described in the AWS Solutions' guide, which generates, tests, compares, and iterates on Amazon Forecast forecasts, enhancing the predictive capabilities of businesses.
- Benefits of Machine Learning in Forecasting:
- Anticipating market changes
- Identifying potential risks
- Seizing emerging opportunities
By leveraging sophisticated algorithms, businesses are empowered to make strategic decisions that are both data-driven and forward-looking, ultimately leading to optimized resource allocation and risk mitigation.
Machine Learning in Customer Behavior Prediction
Businesses are increasingly turning to machine learning (ML) to gain a competitive edge in understanding and predicting customer behavior. By analyzing comprehensive customer data, companies can create detailed customer profiles that inform targeted marketing campaigns and personalized experiences. This leads to enhanced customer engagement and loyalty, as well as improved conversion rates.
ML-driven recommendation engines are a prime example of this application. They utilize real-time data to deliver personalized content and product suggestions, revolutionizing customer interactions in sectors like marketing and e-commerce. The benefits of such systems are clear:
- Hyper-targeted marketing strategies
- Dynamic pricing models
- Personalized user experiences
- Increased brand loyalty
The integration of machine learning into customer behavior prediction equips businesses with the tools to create more impactful and profitable customer journeys. It's not just about the data collected, but how it's transformed into actionable insights that can drive a business forward.
Ethical Considerations in Predictive Analytics
As businesses increasingly rely on predictive analytics, the ethical implications of these practices come to the forefront. Leveraging accurate data for strategic choices enhances risk evaluation, opportunity identification, and alignment with business objectives. Machine learning not only improves predictive models but also empowers decision-making with advanced algorithms.
However, the use of predictive analytics must be balanced with ethical considerations. It is essential to ensure that data is not only accurate but also sourced and utilized responsibly. Ethical use of data involves transparency, consent, and the protection of individual privacy. Companies must navigate the fine line between personalization and intrusion, ensuring that predictive insights do not compromise customer trust.
The potential of predictive analytics is vast, yet it must be harnessed with a keen awareness of its ethical dimensions. This includes the avoidance of biases that can be inadvertently introduced by algorithms, which may lead to unfair or discriminatory outcomes.
To address these concerns, organizations can adopt a set of best practices:
- Establish clear ethical guidelines for data usage
- Implement regular audits to detect and correct biases
- Ensure transparency in data collection and analytics processes
- Foster an organizational culture that prioritizes ethical considerations
By adhering to these principles, businesses can responsibly exploit the power of predictive analytics while maintaining the trust of their customers and the public at large.
Optimizing Operations with Data-Driven Solutions
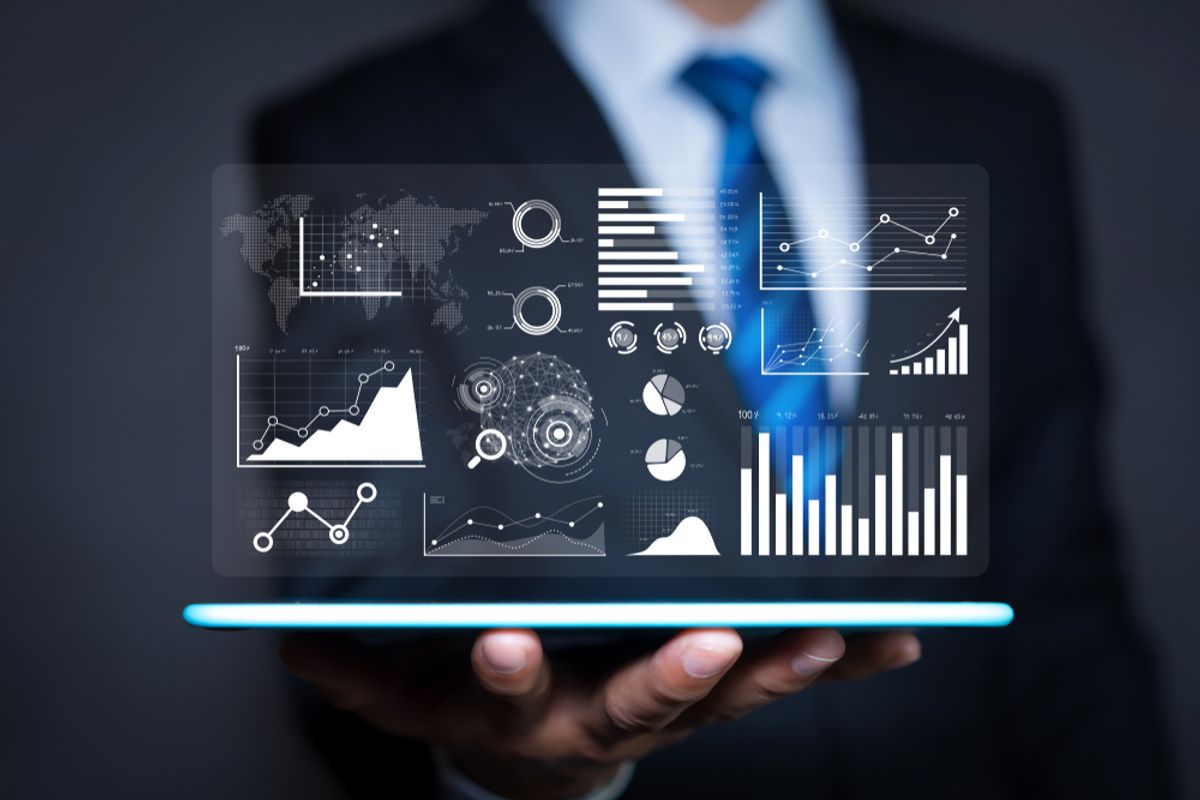
Streamlining Processes through Analytics
In the realm of business operations, the integration of analytics stands as a pivotal factor in streamlining processes. Integrating analytics into business processes optimizes operations, enhances decision-making, and improves efficiency through data insights and real-time analysis in cloud-based environments. This integration leads to a multitude of benefits:
- Improved decision-making: Leveraging data analytics equips companies with the insights necessary for informed decision-making, fostering business growth.
- Enhanced customer experience: Through the analysis of customer data, businesses can refine their offerings to better align with customer needs and preferences.
- Increased efficiency: Analytics serve as a tool to identify bottlenecks and optimize processes, ensuring maximum operational efficiency.
The operational efficiency gained through analytics not only reduces costs but also elevates productivity and overall performance. By pinpointing inefficiencies in real time, organizations can proactively tackle issues, enhancing workflow effectiveness.
Furthermore, AI-driven analytics tools are revolutionizing operational processes by automating routine tasks, optimizing resource allocation, and pinpointing inefficiencies swiftly. These tools, utilizing machine learning algorithms, empower businesses to make data-driven decisions that significantly boost operational efficiency.
Reducing Operational Costs with Data Insights
In the quest to trim operational expenses, businesses are turning to data analytics as a powerful ally. By analyzing patterns and pinpointing inefficiencies, companies can not only streamline processes but also uncover cost-saving opportunities that might otherwise go unnoticed.
Prescriptive analytics offer tailored strategies for customer engagement, improved efficiency, risk management, and profitability. These strategies are particularly effective in IT operations, where data insights can lead to increased efficiency and enhanced performance.
- Identify inefficiencies and address them promptly.
- Forecast trends to anticipate future needs.
- Make informed decisions based on actionable insights.
- Optimize resource allocation to reduce unnecessary expenses.
By leveraging AI-driven analytics tools, businesses can automate routine tasks and optimize resource allocation, leading to significant cost reductions and a more agile operational model.
Enhancing Supply Chain Efficiency with Predictive Analytics
The integration of predictive analytics into supply chain management marks a transformative step towards operational excellence. Predictive analytics helps businesses optimize supply chains by forecasting demand, preventing disruptions, and streamlining logistics. This proactive approach allows for decisions based on predictive analytics, thereby enhancing strategic planning, optimizing resource allocation, and mitigating potential risks.
By leveraging AI for real-time data processing, organizations can respond promptly to emerging trends, unforeseen challenges, or sudden opportunities, ensuring that they stay ahead of the curve and capitalize on timely insights.
The benefits of predictive analytics in supply chain management include:
- Identifying inefficiencies: Pinpointing areas that require improvement to enhance productivity.
- Forecasting trends: Anticipating future demands to ensure preparedness and resource availability.
- Improving decision-making: Utilizing actionable insights for informed strategic choices.
AI-driven analytics tools are not just about processing data; they are about transforming operational processes. These tools, powered by machine learning algorithms, enable businesses to make data-driven decisions that enhance operational efficiency and lead to better overall performance.
Empowering Innovation through Data Analytics
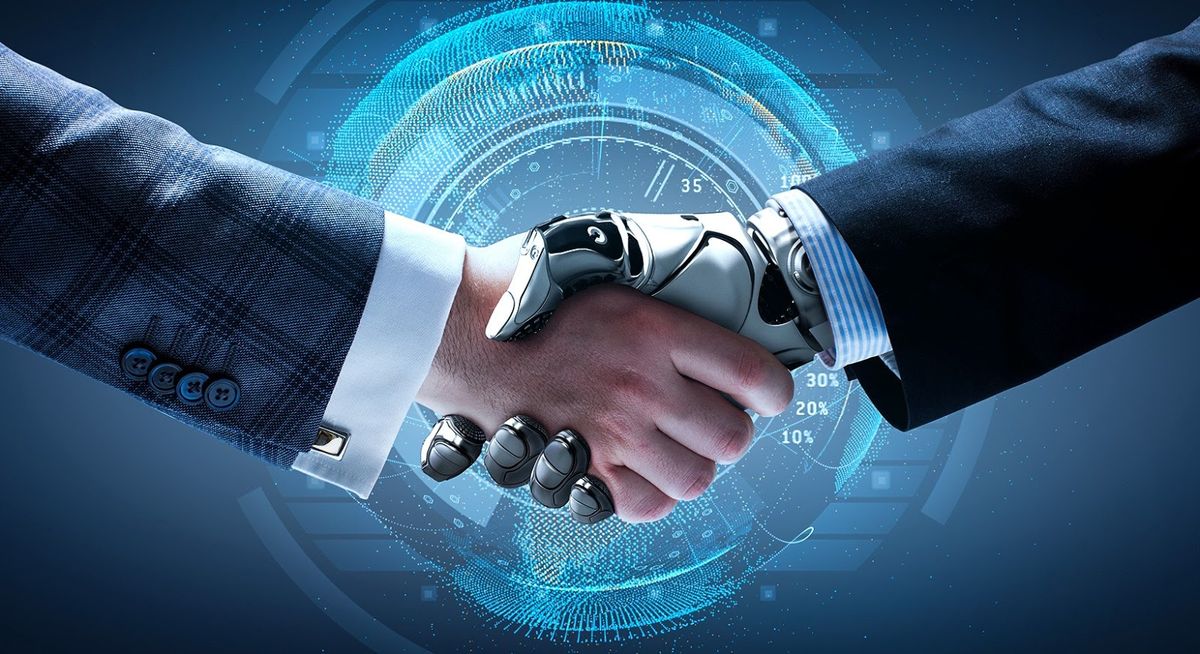
Cultivating a Culture of Innovation with Data
For organizations to excel in innovation, it's crucial to foster a data-driven culture where all employees, regardless of their roles, are empowered to make informed decisions. This culture is the bedrock of a self-sufficient, agile business environment.
Data intelligence is key to promoting data literacy and democratizing access to insights. By offering training and development opportunities, businesses can enhance their team's data skills, enabling them to harness the full potential of analytics for innovation.
Implementing data-driven strategies requires a systematic approach, including defining objectives, determining relevant data sources, and utilizing advanced analytics tools to extract actionable insights.
The power of data in driving IT innovation cannot be overstated. It acts as a catalyst for developing new products, optimizing operations, and identifying growth opportunities. Here's how businesses can start building this innovative culture:
- Define clear objectives for data usage
- Ensure access to relevant data sources
- Analyze data with advanced tools
- Encourage cross-departmental collaboration
- Promote ongoing data literacy and training
- Regularly review and adapt strategies
Analytics as a Catalyst for New Product Development
In the realm of product innovation, analytics serves as a powerful catalyst, transforming raw data into actionable insights that can shape the future of a business's offerings. By analyzing market trends, customer feedback, and competitive landscapes, companies can identify opportunities for new products that meet emerging needs.
Analytics enables businesses to iterate rapidly on product concepts, testing and refining ideas through data simulations before committing to full-scale development. This approach not only accelerates the product development cycle but also increases the chances of market success.
- Identify market gaps and opportunities
- Analyze customer data for preferences and pain points
- Prototype and test concepts using data models
- Refine product features based on feedback loops
By embedding analytics into the product development process, businesses can ensure that every new product is backed by a strong data-driven rationale, significantly reducing the risk of market failure.
The integration of analytics into product development is not just about creating new products; it's about reimagining the possibilities of what a product can be. With the right data insights, the next groundbreaking innovation could be just around the corner.
Benchmarking and Competitive Analysis through Data
In the realm of business, benchmarking and competitive analysis are critical for maintaining a competitive edge. Data analytics enables a deeper understanding of market positioning and performance relative to competitors. By systematically collecting and analyzing data on pricing, discounts, and stock availability, businesses can identify areas for improvement and strategize accordingly.
The synthesis of comprehensive data collection and interactive dashboard development facilitates a dynamic approach to competitive analysis, allowing for real-time adjustments and strategic pivots.
The following steps outline the process of leveraging data for competitive analysis:
- Data Collection: Gather relevant data from diverse sources to ensure a comprehensive dataset.
- Data Analysis: Employ advanced analytics to extract actionable insights from the data.
- Data Visualization: Create intuitive dashboards and visualizations to communicate findings effectively.
By integrating these steps, businesses can not only keep pace with but also outmaneuver their competition through informed decision-making and strategic initiatives.
Transforming Customer Experiences with Analytics
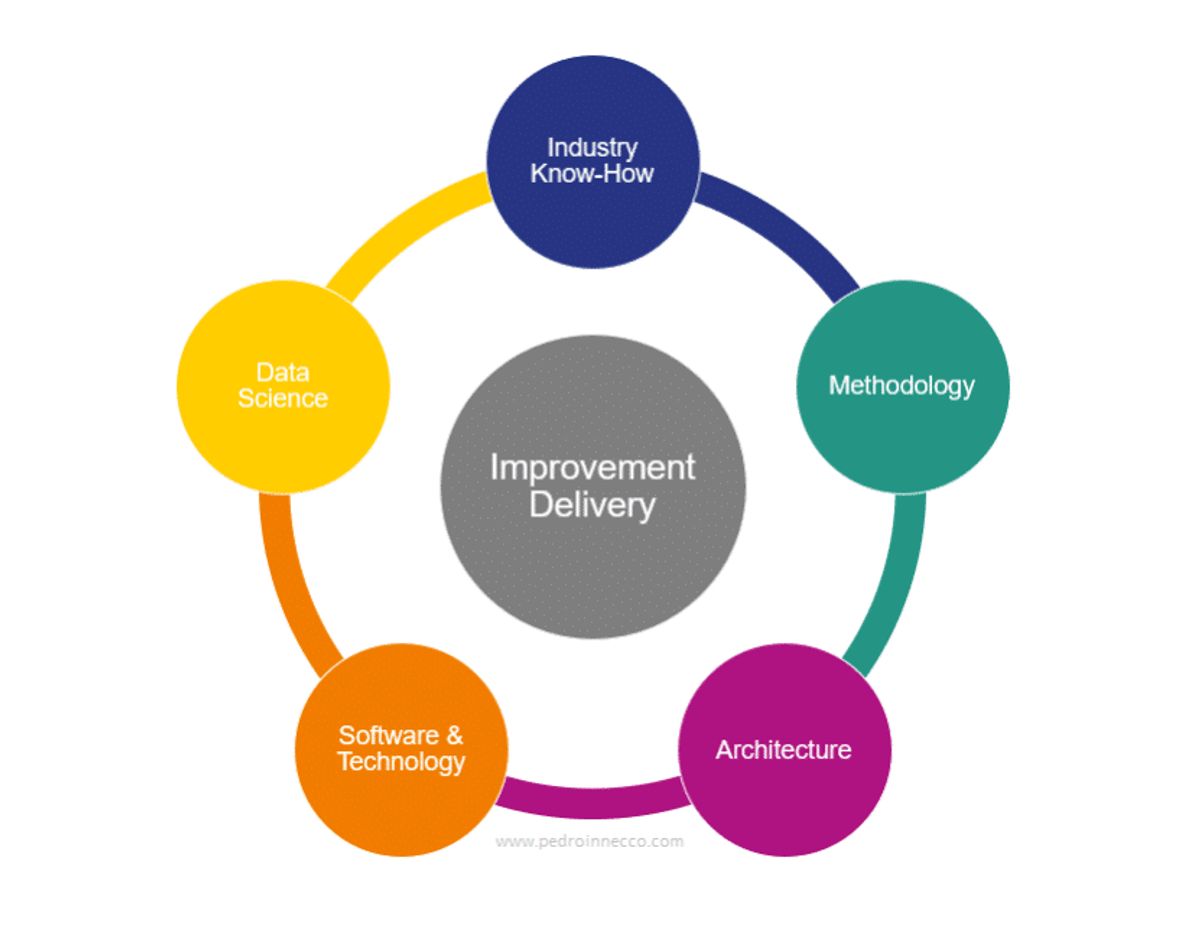
Personalization Strategies Powered by Data
In the realm of customer engagement, personalization is the cornerstone of modern business strategies. By leveraging artificial intelligence (AI), companies can dissect vast amounts of data to understand individual preferences and behaviors, enabling them to offer highly tailored experiences. This not only elevates customer satisfaction but also cultivates brand loyalty.
The integration of AI-powered recommendation engines in marketing and e-commerce has been transformative. Utilizing real-time data analysis, these engines curate personalized content, product suggestions, and targeted campaigns for each user, enriching their experience and driving business metrics.
Here are some key benefits of AI-driven personalization:
- Improved User Experience: Tailored interactions lead to greater customer satisfaction and engagement.
- Enhanced Decision-Making: Data-driven insights assist in making informed strategic choices.
- Increased Efficiency: Automation of routine tasks allows teams to focus on higher-value work.
Data intelligence is indispensable for businesses aiming to deliver hyper-personalized services at scale, ensuring that every touchpoint in the customer journey resonates with individual needs and preferences.
Understanding Customer Journeys through Analytics
The journey of a customer from initial awareness to final purchase and beyond is complex and multifaceted. By leveraging analytics, businesses can decode this journey, uncovering patterns and touchpoints that influence decision-making. Predictive analytics transforms the way companies interact with their customers, enabling personalized experiences at every stage.
Italics are essential in understanding the nuances of customer behavior. For instance, analyzing demographics, purchase histories, and real-time browsing activity can reveal preferences and tendencies that inform targeted marketing and dynamic pricing.
The integration of customer data from multiple sources allows for a comprehensive view that is critical for tailoring offerings and experiences to individual needs.
Here are some key benefits of understanding customer journeys through analytics:
- Enhanced personalization of marketing campaigns
- Improved customer engagement and loyalty
- Optimized product recommendations and pricing strategies
- Data-driven adjustments to maximize return on investment
By embracing a data-centric approach, companies can ensure that every customer interaction is informed and intentional, leading to increased satisfaction and sales.
Improving Customer Service with Actionable Insights
In the realm of customer service, data analytics plays a pivotal role in elevating the customer experience. By leveraging insights from customer data, businesses can anticipate needs and resolve issues proactively, leading to enhanced satisfaction. Actionable insights derived from analytics enable companies to tailor their interactions and services, ensuring that each customer feels heard and valued.
- Improved Decision-Making: Strategic data insights guide businesses to make informed choices, enhancing service quality.
- Increased Efficiency: Data-driven optimizations in customer service processes lead to quicker resolutions.
- Enhanced Customer Experience: Personalized service based on customer data insights fosters loyalty and trust.
By harnessing the power of CX data analytics, businesses can transform their customer service approach, moving from reactive to proactive engagement. This shift not only improves the customer's perception but also drives business growth through increased loyalty and positive word-of-mouth.
Building a Scalable Analytics Infrastructure
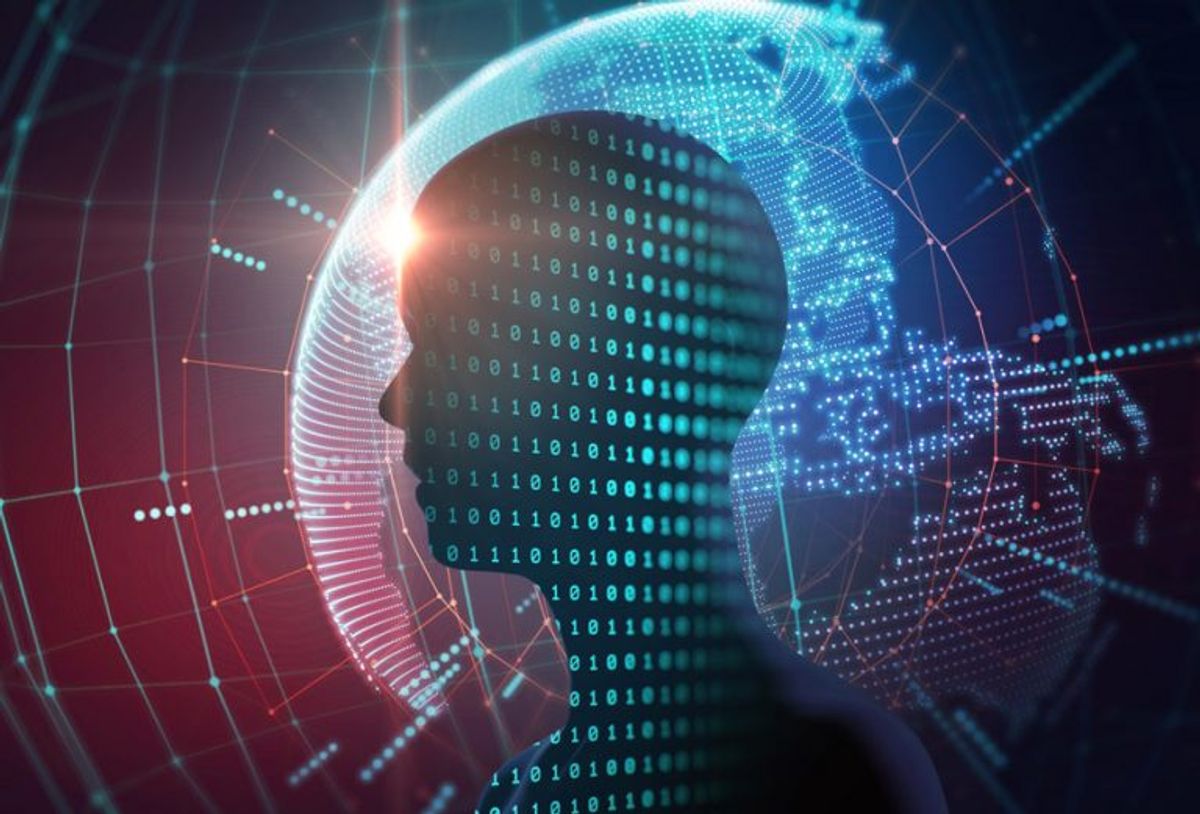
Choosing the Right Tools and Technologies
Selecting the appropriate tools and technologies is a cornerstone of building a robust analytics infrastructure. The right set of tools can significantly enhance the efficiency and accuracy of data analysis, enabling businesses to gain a competitive edge. It's essential to choose solutions that not only address current needs but are also scalable to accommodate future growth.
Scalability, ease of integration, and user-friendliness are critical factors to consider when evaluating analytics tools. Here's a list of considerations to keep in mind:
- Compatibility with existing systems
- Support for a wide range of data sources
- User access controls and security features
- Availability of technical support and community resources
- Total cost of ownership, including licensing and maintenance fees
By prioritizing these factors, organizations can ensure they invest in a technology stack that will serve them well into the future, without necessitating frequent overhauls or incurring unexpected costs.
Ensuring Scalability and Flexibility in Data Architecture
In the realm of data architecture, scalability and flexibility are paramount for businesses aiming to stay agile and responsive to the ever-growing data landscape. A data lakehouse architecture, for instance, provides a foundation that accommodates both structured and unstructured data, enabling companies to scale their data storage and processing capabilities efficiently.
To ensure that a data architecture can handle the increasing volume and complexity of data, several key factors must be considered:
- The ability to manage and analyze large datasets without compromising performance.
- Automated data discovery and organization to facilitate easy access to distributed data assets.
- Comprehensive data lineage and quality monitoring to maintain data trustworthiness.
By focusing on these aspects, businesses can build a data architecture that not only meets current needs but is also prepared for future demands. This proactive approach is essential for maintaining a competitive edge in today's data-driven market.
The Importance of Skilled Analytics Personnel
In the realm of data analytics, the human element cannot be overstated. Skilled analytics personnel are the linchpin in translating raw data into actionable insights. They possess the expertise to navigate complex data sets and the acumen to discern patterns that can inform strategic decisions. A proficient analytics team can leverage tools and technologies to their fullest potential, ensuring that investments in data infrastructure yield tangible benefits.
The decision to build or buy a People Analytics Platform is a critical one, as it involves considerations of cost, customization, and control over data. Such platforms are essential for the seamless storage, retrieval, and analysis of extensive historical workforce data, providing a comprehensive view that can drive human resource strategies.
The value of analytics personnel extends beyond technical skills; they must also excel in communication and collaboration, acting as a bridge between data and decision-makers.
To underscore the importance of these professionals, consider the following points:
- They ensure the quality and integrity of data, which is foundational for reliable analytics.
- Their insights can lead to improved decision-making, enhanced customer experiences, and increased operational efficiency.
- They are pivotal in fostering a data-driven culture within an organization, encouraging all employees to engage with data analytics.
Navigating the Ethical Landscape of Data Analytics
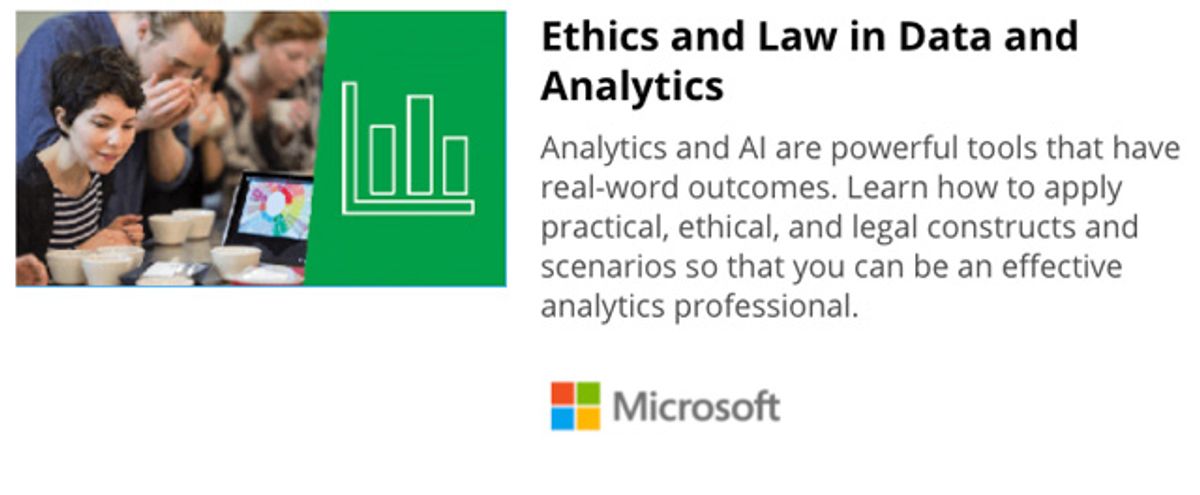
Addressing Privacy Concerns in Data Collection
In the era of big data, privacy concerns are paramount as businesses collect vast amounts of information. It is essential to strike a balance between leveraging data for competitive advantage and respecting individual privacy. To address these concerns, companies must adopt a proactive approach to data management.
- Transparency in data collection and use is the first step towards building trust with consumers.
- Ensuring compliance with evolving data protection regulations is not just a legal necessity but also a strategic advantage.
- Implementing robust security measures to safeguard data against breaches is critical.
By prioritizing privacy, businesses not only comply with regulations but also demonstrate their commitment to ethical practices, fostering consumer confidence.
Creating a privacy-centric culture involves educating employees about the importance of data protection and establishing clear guidelines for handling personal information. As privacy regulations continue to evolve, staying informed and agile is key to maintaining compliance and protecting your business's reputation.
Establishing Ethical Guidelines for Data Usage
In the era of big data, establishing ethical guidelines for data usage is not just a regulatory necessity but a cornerstone of consumer trust. Businesses must navigate the complex landscape of data ethics with a clear and actionable framework. This involves not only compliance with existing privacy laws but also a proactive approach to ethical data management.
- Understand your data: Recognize the types of data collected and their potential impact on privacy and user rights.
- Implement clear policies: Develop transparent policies that govern data access, sharing, and usage.
- Ensure accountability: Assign responsibility for data ethics to specific roles within the organization.
- Regularly review practices: Continuously assess and update data practices to align with evolving ethical standards.
By embedding ethical considerations into the data lifecycle, companies can mitigate risks and uphold their responsibility to protect individual rights. It is imperative to foster an environment where data ethics are not an afterthought but an integral part of the business strategy.
Balancing Innovation with Ethical Responsibility
In the quest for competitive advantage, businesses must tread carefully to balance the drive for innovation with ethical responsibility. The ethical use of analytics is not just a legal obligation, but a cornerstone of consumer trust and brand integrity. Ensuring this balance requires a clear ethical framework that guides data usage without stifling creativity.
Ethical considerations should be embedded into the innovation process, from the initial data collection to the final application of insights. This integration helps prevent ethical dilemmas from arising unexpectedly and ensures that all innovations are aligned with the company's values and societal norms.
- Establish clear ethical guidelines
- Conduct regular ethical audits
- Engage stakeholders in ethical discussions
- Promote transparency in data usage
By proactively addressing ethical concerns, companies can foster an environment where innovation flourishes within the boundaries of ethical practice. This approach not only mitigates risk but also enhances the company's reputation in the eyes of customers and partners.
In the rapidly evolving world of data analytics, ethical considerations are paramount. As you strive to harness the power of data while respecting privacy and integrity, it's crucial to partner with experts who can guide you through this complex terrain. Visit OptimizDBA for unparalleled database optimization consulting and experience transaction speeds that redefine efficiency. Our proprietary methodologies and deep industry experience ensure that your data analytics practices not only meet but exceed ethical standards. Don't navigate this landscape alone; let OptimizDBA be your trusted ally. Connect with us today to elevate your data solutions.
Conclusion
In the quest for a competitive edge, businesses must embrace the transformative power of advanced analytics. This article has underscored the pivotal role that data analytics plays in today's business landscape, from enhancing decision-making processes to optimizing operations and improving customer experiences. As we have seen, leveraging real-time data, strategic insights, and machine learning can propel companies to new heights of efficiency and innovation. The future belongs to those who can not only collect and store data but also unlock its full potential to drive actionable insights and make informed decisions swiftly. Therefore, it is imperative for businesses to invest in advanced analytics tools and foster a culture of data-driven decision-making to remain agile and ahead of the curve in an ever-evolving digital world.
Frequently Asked Questions
How does advanced analytics contribute to a competitive edge in business?
Advanced analytics provides businesses with deep insights, predictive capabilities, and data-driven decision-making processes that can lead to more efficient operations, better customer experiences, and innovative strategies, ultimately giving them a competitive advantage in the market.
What is the importance of fostering a data-driven culture in an organization?
Fostering a data-driven culture ensures that decisions across all levels of the organization are based on data and analytics, which leads to more informed decision-making, improved operational efficiency, and a better understanding of customer needs and market trends.
Why is real-time data crucial for agile decision-making?
Real-time data allows businesses to respond quickly to changing market conditions, customer behaviors, and operational challenges. This agility can improve the effectiveness of decisions and enable companies to capitalize on opportunities or mitigate risks promptly.
How can machine learning improve forecast accuracy?
Machine learning algorithms can analyze large datasets to identify patterns and trends that may not be apparent to human analysts. This can lead to more accurate predictions about future events, such as sales trends, inventory needs, or market movements.
What are the ethical considerations in predictive analytics?
Ethical considerations in predictive analytics include ensuring privacy and security of data, avoiding biased algorithms that could lead to unfair outcomes, and being transparent about how data is collected and used in making predictions.
In what ways can analytics optimize business operations?
Analytics can optimize business operations by identifying inefficiencies, predicting maintenance needs, streamlining supply chains, and reducing operational costs. This leads to smoother processes and can contribute to higher profitability.
What factors should be considered when building a scalable analytics infrastructure?
When building a scalable analytics infrastructure, businesses should consider the integration capabilities of tools and technologies, the flexibility and scalability of data architecture, and the availability of skilled personnel to manage and interpret data.
How can businesses navigate the ethical landscape of data analytics?
Businesses can navigate the ethical landscape by establishing clear privacy policies, adhering to regulations such as GDPR, implementing ethical guidelines for data usage, and maintaining a balance between innovation and ethical responsibility.