In today's fast-paced and data-driven world, businesses are increasingly recognizing the critical role that data plays in driving decision-making and optimizing performance. Business intelligence (BI) and analytics have emerged as essential tools for organizations looking to harness the power of data, uncover valuable insights, and gain a competitive edge. This comprehensive guide delves into the various aspects of BI and analytics, from understanding data sources and key components to implementing advanced techniques and fostering a data-driven culture.
Key Takeaways
- Business Intelligence (BI) and analytics are essential tools for transforming raw data into actionable insights.
- Data-driven decision-making helps organizations stay competitive and optimize performance.
- Key components of BI systems include data warehousing, ETL processes, and data governance.
- Effective data visualization techniques are crucial for interpreting and communicating data insights.
- Future trends in BI include AI integration, real-time analytics, and leveraging big data.
Understanding Business Intelligence and Analytics
In today's fast-paced and data-driven world, businesses are increasingly recognizing the critical role that data plays in driving decision-making and optimizing performance. Business intelligence (BI) and analytics have emerged as essential tools for organizations looking to harness the power of data, uncover valuable insights, and gain a competitive edge. This comprehensive guide delves into the various aspects of BI and analytics, from understanding data sources and key components to implementing effective strategies.
Defining Business Intelligence
Business intelligence enhances decision-making processes, operational efficiency, and market trend identification. It involves the use of technologies, applications, and practices for the collection, integration, analysis, and presentation of business information. The goal of BI is to support better business decision-making.
The Role of Analytics in Modern Business
Analytics transforms raw data into actionable insights through descriptive, predictive, and prescriptive analytics. By leveraging advanced analytics tools and techniques, organizations can uncover hidden patterns, predict future trends, and make informed decisions that drive business success.
Key Differences Between BI and Analytics
While BI focuses on the collection and presentation of business information, analytics goes a step further by interpreting this data to provide deeper insights and foresight. Understanding these differences is crucial for businesses aiming to implement effective data strategies.
The Importance of Data-Driven Decision Making
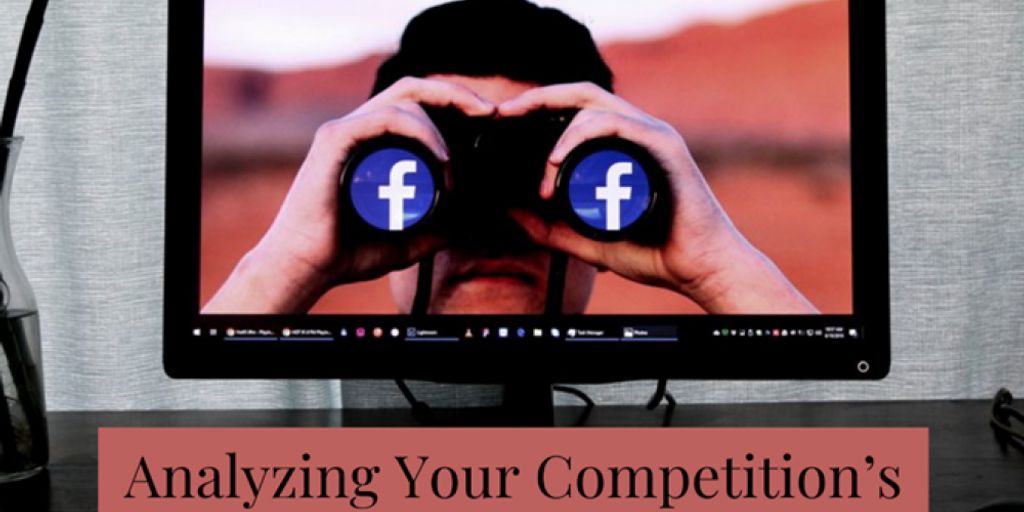
Benefits of Data-Driven Strategies
The capacity of data analytics to improve decision-making procedures is one of its main advantages. By analyzing historical data, organizations can identify trends and patterns that inform future strategies. This evidence-based approach reduces reliance on intuition and facilitates more accurate decisions.
- Informed Decision Making: Leveraging data-backed insights for unparalleled growth is essential for modern businesses. By implementing data-driven strategies, companies can unlock the power of data to make decisions and optimize performance.
- Operational Efficiency: Data-driven strategies help streamline operations, reduce costs, and improve overall efficiency.
- Competitive Advantage: Organizations that effectively use data can stay ahead of the competition by quickly adapting to market changes.
Challenges in Implementing Data-Driven Decision Making
While the benefits are clear, there are several challenges in implementing data-driven decision-making processes:
- Data Quality: Ensuring the accuracy and reliability of data is crucial for making sound decisions.
- Data Integration: Combining data from various sources can be complex and time-consuming.
- Resistance to Change: Employees may be hesitant to adopt new data-driven approaches, preferring traditional methods.
- Cost: Implementing data-driven systems can be expensive, requiring significant investment in technology and training.
Case Studies of Successful Data-Driven Companies
Several companies have successfully implemented data-driven strategies to achieve remarkable results:
- Amazon: By leveraging data analytics, Amazon has optimized its supply chain, personalized customer experiences, and improved inventory management.
- Netflix: Using data to understand viewer preferences, Netflix has been able to create highly engaging content and recommend shows that keep subscribers coming back.
- Walmart: Through data-driven decision-making, Walmart has enhanced its inventory management, reduced costs, and improved customer satisfaction.
The evolution of data utilization has seen a shift from mere data collection to advanced analytics and real-time processing, allowing businesses to be more agile and responsive to market changes.
Key Components of Business Intelligence Systems
Business Intelligence (BI) is a multifaceted field that encompasses various components essential for transforming raw data into actionable insights. These components work together to help organizations make informed decisions and optimize their operations.
Data Warehousing
A data warehouse is a centralized repository that stores integrated data from multiple sources. It enables organizations to perform complex queries and analysis on large volumes of data. Data warehousing supports business intelligence activities by providing a stable and consistent data source.
ETL Processes
ETL stands for Extract, Transform, Load. These processes are crucial for moving data from various sources into the data warehouse. The ETL process ensures that data is cleansed, transformed into a suitable format, and loaded into the data warehouse for analysis. This step is vital for maintaining data integrity and quality.
Data Governance
Data governance ensures that data is accurate, consistent, and secure. It establishes policies and procedures for data management, which is crucial for maintaining data quality and compliance with regulations. Effective data governance helps organizations trust their data and make reliable business decisions.
Implementing a successful BI strategy requires clear objectives, the right tools, and a skilled team.
Data Collection and Integration
Sources of Business Data
Gathering pertinent data is the initial step in the data analytics process. This can be achieved through various means such as surveys, transaction records, social media, sensors, and more. The quality and accuracy of the data collected are critical as they form the foundation of the entire analytics process.
Techniques for Data Integration
Organizations often struggle with integrating data from various sources, especially when dealing with large and complex datasets. Effective data integration requires sophisticated tools and techniques to ensure seamless data flow and consistency. Combining information from multiple sources directly into a single source of truth ensures compatibility and consistency. This is where data extraction, transformation, and loading (ETL) or real-time data integration happens. The good thing is that there are dozens of ETL tools to help.
Overcoming Data Silos
Develop a process to automatically gather and ingest data from multiple sources and formats into one consolidated and cohesive view. This can be achieved through ETL (extract, transform and load) processes. There are many ways to build data ingestion frameworks based on business model architecture. However, data ingestion itself can be done in two ways, batch or streaming.
Ensuring data quality involves continuous monitoring and management. Decisions made based on incomplete or inaccurate facts might be disastrous.
Tools and Technologies for Business Intelligence
In today's competitive business landscape, leveraging the right tools and technologies is crucial for unlocking the power of data. Choosing the right business intelligence software depends on the specific needs of your organization, whether it's interactive data visualizations or detailed financial data analysis.
Data Visualization Techniques
Importance of Data Visualization
Data visualization is the process of representing data in graphical or pictorial format. It helps in communicating complex data insights in a more understandable and visually appealing way. Effective data visualization is key to uncovering patterns and trends that might not be apparent in raw data. Visualization tools such as Tableau, Power BI, and matplotlib allow data analysts to create interactive charts, graphs, and dashboards that facilitate understanding and decision-making.
Common Data Visualization Tools
Data visualization tools help in converting complex data sets into visual formats like charts, graphs, and dashboards. These tools make it easier to understand and interpret data, enabling stakeholders to make informed decisions. Some popular data visualization tools include:
- Tableau
- Power BI
- D3.js
- matplotlib
Data visualization is not just about creating pretty charts; it's about telling a story with data to drive actionable insights.
Best Practices for Effective Data Visualization
To create effective data visualizations, consider the following best practices:
- Choose the right type of visualization: Different types of data require different types of visualizations. For example, use bar charts for comparisons, line charts for trends, and pie charts for proportions.
- Simplify your visuals: Avoid clutter and focus on the key message you want to convey. Use colors and labels wisely to highlight important information.
- Ensure data accuracy: Double-check your data sources and calculations to ensure the accuracy of your visualizations.
- Make it interactive: Interactive dashboards and charts can provide a more engaging experience for users, allowing them to explore the data in more detail.
- Tell a story: Use your visualizations to tell a compelling story that guides the audience through the data and highlights the key insights.
Advanced Analytics Techniques
Predictive Analytics
Predictive analytics involves using historical data to make informed predictions about future events. By analyzing patterns and trends, businesses can anticipate customer behavior, market trends, and operational outcomes. Predictive models are essential for proactive decision-making and strategic planning.
Prescriptive Analytics
Prescriptive analytics goes a step further by not only predicting future outcomes but also recommending actions to achieve desired results. This technique leverages advanced algorithms and optimization models to provide actionable insights. Businesses can use prescriptive analytics to streamline operations, enhance customer experiences, and maximize profits.
Machine Learning in Business Intelligence
Machine learning (ML) is a subset of artificial intelligence that enables systems to learn from data and improve over time without explicit programming. In business intelligence, ML algorithms can uncover hidden patterns, automate data analysis, and provide real-time insights. By integrating ML into BI systems, companies can unlock the power of data and drive innovation.
By leveraging these advanced analytics techniques, organizations can transform their data into insightful business intelligence, enabling them to make data-driven decisions and stay competitive in the market.
Implementing Business Intelligence Solutions
Implementing a business intelligence strategy is crucial for organizations that manage large amounts of data but are not yet leveraging it effectively. A well-executed strategy can transform data into actionable insights, driving better decision-making and operational efficiency.
Steps to Implement BI Solutions
- Setting Clear Objectives: Align your BI strategy with your organization's key strategic goals. Start by identifying these goals and then determine how BI can support them. This ensures that your analytics efforts are targeted at addressing important business challenges and opportunities.
- Choosing the Right Tools: Select BI tools that fit your organization's needs. Consider factors such as ease of use, scalability, and integration capabilities.
- Building a Skilled Team: Assemble a team with the necessary skills in data analysis, BI tools, and domain knowledge to effectively implement and manage BI solutions.
- Data Integration: Ensure seamless integration of data from various sources to provide a comprehensive view of the business.
- Continuous Improvement: Regularly review and update your BI strategy to adapt to changing business needs and technological advancements.
Common Pitfalls and How to Avoid Them
- Lack of Clear Objectives: Without clear objectives, BI initiatives can become unfocused and fail to deliver value. Ensure that your BI strategy is aligned with your business goals.
- Poor Data Quality: Inaccurate or incomplete data can lead to misleading insights. Implement robust data governance practices to maintain high data quality.
- Resistance to Change: Employees may resist adopting new BI tools and processes. Promote a data-driven culture and provide adequate training to ease the transition.
- Overcomplicating the Solution: Complex BI solutions can be difficult to manage and use. Aim for simplicity and user-friendliness in your BI implementation.
Measuring the Success of BI Initiatives
To measure the success of your BI initiatives, consider the following metrics:
- User Adoption Rate: The percentage of employees actively using BI tools.
- Decision-Making Speed: The time taken to make informed decisions based on BI insights.
- Return on Investment (ROI): The financial benefits gained from BI initiatives compared to the costs incurred.
- Data Accuracy: The reliability and accuracy of the data used in BI processes.
In the ever-evolving landscape of business, continuous improvement in BI practices ensures that companies remain agile and responsive to new challenges and opportunities.
Future Trends in Business Intelligence and Analytics
AI and BI Integration
The integration of Artificial Intelligence (AI) with Business Intelligence (BI) is transforming how businesses operate. AI-driven insights are enabling companies to make more informed decisions by automating data analysis and providing predictive insights. This trend is making BI more accessible and actionable for all levels of an organization.
Real-Time Analytics
Real-time analytics is becoming increasingly important as businesses seek to make timely decisions based on the most current data available. By leveraging real-time data processing, companies can respond to market changes and customer needs more swiftly. This capability is crucial for maintaining a competitive edge in fast-paced industries.
The Role of Big Data in Future BI
Big Data continues to play a significant role in the evolution of BI. The ability to analyze vast amounts of data from various sources allows businesses to uncover hidden patterns and trends. Scalability and data integration are key challenges that need to be addressed to fully harness the potential of Big Data in BI.
The future of business intelligence and analytics is bright, with advancements in technology making it easier for organizations to harness the power of their data. By staying ahead of these trends, businesses can ensure sustainable growth and competitiveness.
Building a Data-Driven Culture
Encouraging Data Literacy
Cultivating a data-centric culture for sustained growth requires a focus on data literacy across all levels of the organization. When everyone, from executives to frontline employees, embraces data-driven insights, businesses can unlock new levels of innovation and growth. This involves training programs, workshops, and continuous learning opportunities to ensure that all employees are comfortable working with data.
Promoting Data-Driven Mindsets
Building a data-driven culture is essential for any organization looking to thrive in today's competitive landscape. By leveraging data effectively, you can make informed decisions that drive growth and innovation. To foster this mindset, organizations should:
- Lead by example: Ensure leadership consistently uses data in decision-making.
- Celebrate data-driven successes: Highlight and reward instances where data-driven decisions lead to positive outcomes.
- Provide access to data: Make data easily accessible to all employees to encourage its use.
Overcoming Resistance to Change
Transitioning to a data-driven culture can face resistance. To overcome this, organizations should:
- Communicate the benefits: Clearly articulate how data-driven decision-making can positively impact the organization.
- Provide support: Offer resources and training to help employees adapt to new tools and processes.
- Foster collaboration: Encourage cross-departmental teamwork to integrate analytics into business strategy for innovation and agility.
Unlocking the potential of advanced analytics for competitive edge in business requires a committed effort to foster a data-driven culture. This commitment ensures that data becomes an integral part of decision-making processes, driving sustained growth and innovation.
Ethical Considerations in Data Analytics
As the use of data analytics and BI continues to grow, so do the ethical considerations surrounding data privacy and security. Organizations must navigate complex data privacy regulations and ensure that their data practices are transparent and ethical. This includes being mindful of biases in data and algorithms, which can impact decision-making processes.
Data Privacy Concerns
With the increasing amount of data being collected, data privacy concerns have become more prominent. Organizations must ensure that they comply with data protection regulations and implement robust security measures to safeguard sensitive information. Failure to address these concerns can lead to severe consequences, including legal penalties and loss of customer trust.
Ensuring Data Accuracy
Ensuring data accuracy is essential for data-driven decision-making and optimizing business performance through BI and analytics tools. Inaccurate data can lead to misguided decisions and strategies. Organizations should implement data validation processes and regularly audit their data to maintain its integrity.
Ethical Use of Data
The ethical use of data involves being transparent about how data is collected, stored, and used. Organizations should avoid using data in ways that could harm individuals or groups. This includes being aware of and mitigating biases in data and algorithms. Promoting a culture of ethical data use is crucial for maintaining trust and integrity in business practices.
The future of business intelligence and analytics is not just about technology; it's about creating a data-driven culture that values ethical considerations and empowers all users to make informed decisions.
Ethical considerations in data analytics are crucial for ensuring responsible and fair use of data. As data professionals, it is our duty to prioritize privacy, transparency, and accountability in all our projects. To deepen your understanding and enhance your skills in this vital area, explore our comprehensive courses designed by industry experts. Visit our website to learn more and take the first step towards becoming a more ethical data analyst.
Conclusion
In today's data-driven world, unlocking the power of data through business intelligence and analytics is no longer a luxury but a necessity for organizations aiming to thrive in today's competitive landscape. This comprehensive guide has walked you through the essential aspects of data analytics, from understanding its importance to implementing advanced techniques. By leveraging data effectively, businesses can uncover hidden patterns, predict future trends, and make informed decisions that drive growth and innovation. As we move forward, the ability to harness and interpret data will continue to be a critical factor in achieving sustained success and maintaining a competitive edge.
Frequently Asked Questions
What is Business Intelligence?
Business Intelligence (BI) refers to technologies, applications, and practices for the collection, integration, analysis, and presentation of business information. The goal of BI is to support better business decision-making.
How does analytics differ from Business Intelligence?
While Business Intelligence focuses on descriptive analytics to understand past and present data, analytics often includes predictive and prescriptive analytics to forecast future trends and suggest actions.
Why is data-driven decision making important?
Data-driven decision making is important because it allows organizations to make informed decisions based on actual data rather than intuition or observation alone. This leads to more accurate, effective, and efficient outcomes.
What are the key components of a Business Intelligence system?
Key components of a BI system include data warehousing, ETL (Extract, Transform, Load) processes, and data governance. These components work together to ensure that data is collected, processed, and presented in a useful manner.
What tools are commonly used in Business Intelligence?
Common BI tools include Tableau, Power BI, QlikView, and Looker. These tools help in data visualization, reporting, and dashboard creation, making it easier to analyze and interpret data.
What are some challenges in implementing a data-driven strategy?
Challenges include data quality issues, data silos, lack of data literacy among employees, and resistance to change. Overcoming these challenges requires a well-thought-out strategy and organizational commitment.
How can businesses ensure data privacy in analytics?
Businesses can ensure data privacy by implementing strong data governance policies, using encryption, anonymizing sensitive data, and complying with relevant data protection regulations such as GDPR or CCPA.
What is the future of Business Intelligence and Analytics?
The future of BI and Analytics is likely to be shaped by advancements in AI, real-time analytics, and the increasing role of big data. These trends will make BI more powerful and accessible, enabling even more precise and actionable insights.