In today's data-driven world, the ability to harness the power of data is crucial for any business looking to stay competitive. Business Intelligence (BI) and Analytics are two key components that enable organizations to make informed decisions, optimize operations, and gain a strategic advantage. This comprehensive guide will walk you through the essentials of BI and Analytics, from understanding their definitions to exploring the tools and techniques that can help you unlock valuable insights from your data.
Key Takeaways
- Understanding the fundamental differences between Business Intelligence and Analytics is essential for leveraging their full potential.
- Data-driven decision making can significantly enhance business performance and competitiveness.
- Choosing the right BI tools and integrating them with existing systems is crucial for effective data analysis.
- Ensuring data quality and employing best practices in data management are foundational to accurate insights.
- Advanced analytics techniques, including predictive and prescriptive analytics, can provide deeper insights and drive strategic decisions.
Understanding Business Intelligence and Analytics
In today's fast-paced digital landscape, businesses are inundated with vast amounts of data. The ability to harness this data effectively can be the difference between leading the market and falling behind. This is where Business Intelligence (BI) and Analytics come into play. Together, they provide the tools and insights necessary to transform raw data into actionable information, driving informed decision-making and strategic planning.
The Importance of Data-Driven Decision Making
Benefits of Data-Driven Decisions
Unlocking the power of data transforms decision-making processes within organizations. By harnessing data, companies can gain a competitive advantage, access real-time insights, and empower employees to make informed decisions. The benefits include:
- Improved accuracy in forecasting and planning
- Enhanced customer experiences through personalized services
- Increased operational efficiency and cost savings
- Better risk management and compliance
Challenges in Implementing Data-Driven Strategies
Despite the clear benefits, implementing data-driven strategies is not without its challenges. Organizations often face obstacles such as:
- Data silos and integration issues
- Lack of data literacy among employees
- High costs of data infrastructure and tools
- Resistance to change within the company culture
Overcoming these challenges requires a strategic approach, including investing in the right technology and fostering a culture that values data-driven decision-making.
Case Studies of Successful Data-Driven Companies
Several companies have successfully leveraged data to drive their business strategies. For instance, a leading e-commerce platform uses data analytics to optimize its supply chain, resulting in significant cost reductions and improved delivery times. Another example is a financial services firm that employs predictive analytics to enhance fraud detection, thereby safeguarding customer assets and building trust.
These case studies highlight the transformative potential of data when effectively integrated into business operations.
Essential Tools for Business Intelligence
In today's competitive business landscape, leveraging the right tools and technologies is crucial for unlocking the power of data. Choosing the right business intelligence software depends on the specific needs of your organization, whether it's interactive data visualizations or detailed financial data analysis.
Data Collection and Management
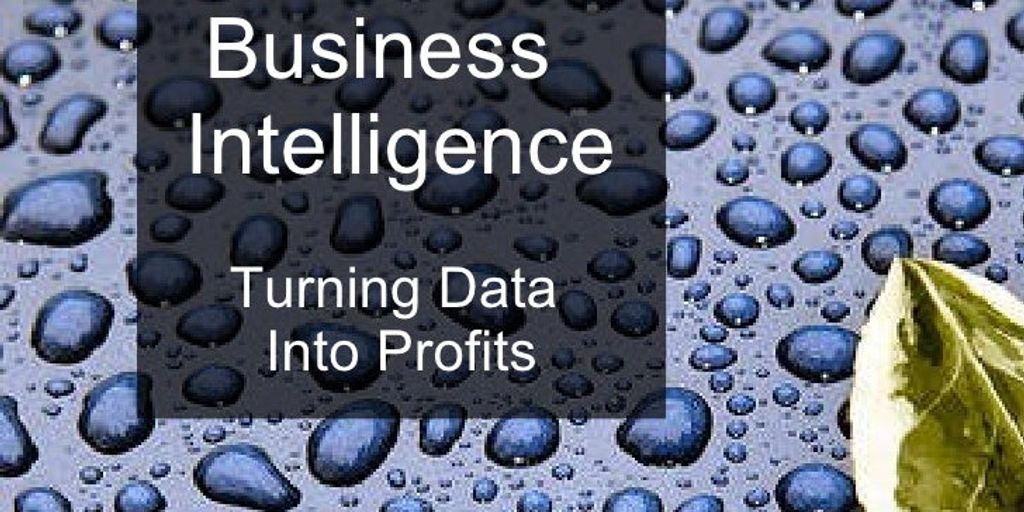
Methods of Data Collection
Gathering data from various sources is crucial for any business intelligence strategy. Data can be collected from customer databases, CRM systems, and social media platforms. This diverse range of sources ensures a comprehensive dataset for analysis.
Data Management Best Practices
Effective data management involves several key steps:
- Data Profiling: Assess the quality of the data and identify any issues such as missing values or inconsistencies.
- Data Cleansing: Remove or correct any errors, duplicates, and inconsistencies in the data.
- Data Transformation: Standardize formats and convert data into a suitable format for analysis.
Ensuring Data Quality
Once the data is collected, it needs to be cleaned and prepared for analysis. This process involves removing duplicate records, correcting errors, standardizing formats, and addressing missing or inconsistent data. Ensuring data quality is essential for accurate and reliable analysis.
A well-maintained data dictionary can serve as a foundation to build the data model and lay out the logic for data relationships.
Data Visualization Techniques
Principles of Effective Data Visualization
Data visualization is the process of representing data in graphical or pictorial format. It helps in communicating complex data insights in a more understandable and visually appealing way. Some common techniques include:
- Bar Charts
- Line Graphs
- Pie Charts
- Scatter Plots
- Heat Maps
Effective data visualization enables stakeholders to quickly grasp complex information and make data-driven decisions.
Tools for Data Visualization
Data visualization tools are used to present data in a graphical format, making it easier to understand and interpret. These tools help in creating dashboards, charts, and graphs that provide a visual representation of the data. Some popular data visualization tools include:
- Tableau
- Power BI
- Google Data Studio
- D3.js
- QlikView
Common Pitfalls in Data Visualization
While data visualization can be incredibly powerful, there are common pitfalls to avoid:
- Overloading with Information: Too much data can overwhelm the viewer.
- Misleading Visuals: Ensure that the visual representation accurately reflects the data.
- Lack of Context: Provide enough context for the viewer to understand the data.
- Poor Design: Aesthetics matter; a poorly designed chart can be confusing.
Implementing BI in organizations is crucial for unlocking the power of data: an introduction to business intelligence and analytics. Data visualization transforms insights for informed decision-making.
Advanced Analytics Techniques
Advanced analytics techniques are crucial for businesses aiming to remain competitive in today's data-driven landscape. By mastering these techniques, organizations can make more informed decisions and drive success.
Predictive Analytics
Predictive analytics leverages historical data to forecast future outcomes. This technique uses statistical models and machine learning algorithms to predict future events, enabling businesses to anticipate trends and make proactive decisions. For instance, a company might use predictive analytics to forecast sales for the next quarter, helping them adjust their strategies accordingly.
Prescriptive Analytics
Prescriptive analytics goes a step further by not only predicting future outcomes but also recommending actions to achieve desired results. This technique combines data, algorithms, and business rules to suggest the best course of action. For example, a logistics company might use prescriptive analytics to determine the most efficient delivery routes, saving time and reducing costs.
Descriptive Analytics
Descriptive analytics involves summarizing historical data to identify patterns and trends. This technique helps organizations understand what has happened in the past and provides a foundation for future analysis. By applying various methods, analysts can uncover patterns, trends, and correlations that drive informed decision-making.
Mastering advanced analytics techniques is essential for any organization looking to harness the full potential of their data. By integrating these methods, businesses can transform raw data into actionable insights, leading to better decision-making and improved outcomes.
Building a Data-Driven Culture
Encouraging Data Literacy
To foster a data-driven culture, it's essential to promote data literacy across all levels of the organization. This involves training employees to understand, interpret, and utilize data effectively. Workshops, online courses, and hands-on training sessions can be instrumental in achieving this goal. Additionally, creating a data dictionary that records all data sources and their functions can serve as a valuable resource for employees.
Overcoming Resistance to Change
Implementing a data-driven culture often faces resistance from employees accustomed to traditional methods. To overcome this, start with smaller, impactful data projects that demonstrate the benefits of data-driven decision-making. This approach helps in securing internal buy-in and gradually managing resistance. It's also crucial to communicate the long-term benefits and possibilities that data-driven strategies can offer.
Creating a Data-Driven Mindset
A data-driven mindset can be cultivated by integrating data into everyday business processes. Encourage teams to rely on data for decision-making and problem-solving. Regularly sharing success stories and case studies of data-driven projects can also inspire and motivate employees. Moreover, aligning data initiatives with business goals ensures that data-driven strategies are not just a trend but a core part of the organizational culture.
Building a data-driven culture is not a one-time effort but a continuous process that requires commitment and collaboration from all levels of the organization.
The Role of Machine Learning in BI and Analytics
Introduction to Machine Learning
Machine learning, a branch of artificial intelligence, empowers systems to learn from data and enhance their performance over time without explicit programming. This technology is increasingly pivotal in business analytics, enabling more accurate predictions and deeper insights. AI-driven insights are becoming a cornerstone of strategic planning and operational efficiency.
Applications of Machine Learning in BI
Machine learning automates the process of identifying patterns and making predictions from data. This allows businesses to analyze large and complex datasets more efficiently, deriving insights that would be challenging to obtain through traditional methods. Key applications include:
- Predictive modeling
- Customer segmentation
- Fraud detection
- Supply chain optimization
Challenges of Implementing Machine Learning
Despite its potential, implementing machine learning in BI comes with challenges. These include data integration, data quality issues, and a lack of skilled personnel. Overcoming these challenges requires careful planning, adequate resources, and a strong commitment to data governance.
As the landscape of BI and Analytics continues to evolve, businesses must prepare for future challenges. This includes unlocking the power of data: the future of business intelligence and analytics.
Future Trends in Business Intelligence and Analytics
The future of Business Intelligence and Analytics is set to be even more transformative as technology continues to advance. Key trends that will shape the future of BI and analytics include:
AI and BI Integration
AI and machine learning are becoming integral components of BI and analytics tools. These technologies will enable more advanced data analysis, automate routine tasks, and provide more accurate predictive insights. Staying ahead of these trends will be crucial for maintaining a competitive edge in the market.
The Rise of Self-Service Analytics
Self-service analytics tools are empowering users to generate their own insights without relying heavily on IT departments. This democratization of data access allows for quicker decision-making and fosters a more data-driven culture within organizations.
The Impact of Big Data on BI
The explosion of big data is revolutionizing how businesses approach BI. With the ability to process and analyze vast amounts of data in real-time, companies can gain deeper insights and make more informed decisions. This shift is driving the need for more robust data management and governance practices.
Unlocking the power of data is central to the future of BI and Analytics. Embracing these trends will be key to achieving business success.
Real-World Applications of BI and Analytics
Business Intelligence (BI) and Analytics are revolutionizing industries by providing actionable insights and data-driven decision-making capabilities. By leveraging these tools, businesses can transform raw data into actionable insights, unlocking new opportunities and optimizing operations.
Business Intelligence (BI) and Analytics are transforming industries by providing actionable insights and data-driven decision-making capabilities. From enhancing customer experiences to optimizing operations, the real-world applications are vast and impactful. Discover how you can leverage these powerful tools to drive success in your own projects. Visit our website to explore our comprehensive courses and start your journey towards becoming a BI and Analytics expert today!
Conclusion
Armed with the insights from this comprehensive guide, you are now well-equipped to unlock the full potential of your business data. Business Intelligence (BI) and Analytics are not just for experts; they are accessible tools that every business professional can use to gain valuable insights, make informed decisions, and stay ahead in today's data-driven world. By leveraging advanced analytics tools and techniques, you can uncover hidden patterns, predict future trends, and drive growth and optimization within your organization. Start your data analytics journey today and watch your business thrive with newfound intelligence.
Frequently Asked Questions
What is Business Intelligence (BI)?
Business Intelligence (BI) refers to technologies, applications, and practices for the collection, integration, analysis, and presentation of business information. The purpose of BI is to support better business decision-making.
What is the difference between Business Intelligence and Analytics?
Business Intelligence focuses on descriptive analytics, providing historical data insights to inform decision-making. Analytics, on the other hand, includes predictive and prescriptive analytics, which forecast future trends and suggest actions to achieve desired outcomes.
Why is data-driven decision making important?
Data-driven decision making is important because it allows organizations to make informed decisions based on actual data, leading to more accurate, effective, and efficient outcomes. It helps businesses stay competitive and innovative.
What are some popular BI tools?
Some popular BI tools include Microsoft Power BI, Tableau, QlikView, Looker, and SAP BusinessObjects. These tools help organizations visualize and analyze their data to gain actionable insights.
What are the challenges in implementing data-driven strategies?
Challenges include data quality issues, lack of skilled personnel, data integration difficulties, high costs of BI tools, and resistance to change within the organization.
How can businesses ensure data quality?
Businesses can ensure data quality by implementing data governance practices, conducting regular data audits, using data cleaning tools, and establishing data quality metrics and standards.
What role does machine learning play in BI and Analytics?
Machine learning plays a significant role in BI and Analytics by automating data analysis, identifying patterns, making predictions, and providing prescriptive insights. It enhances the ability to process large volumes of data and uncover hidden insights.
What future trends are expected in Business Intelligence and Analytics?
Future trends in BI and Analytics include the integration of artificial intelligence (AI), the rise of self-service analytics, and the increasing impact of big data. These trends will make BI tools more accessible, powerful, and integral to business operations.