The Evolution of Business Intelligence Tools
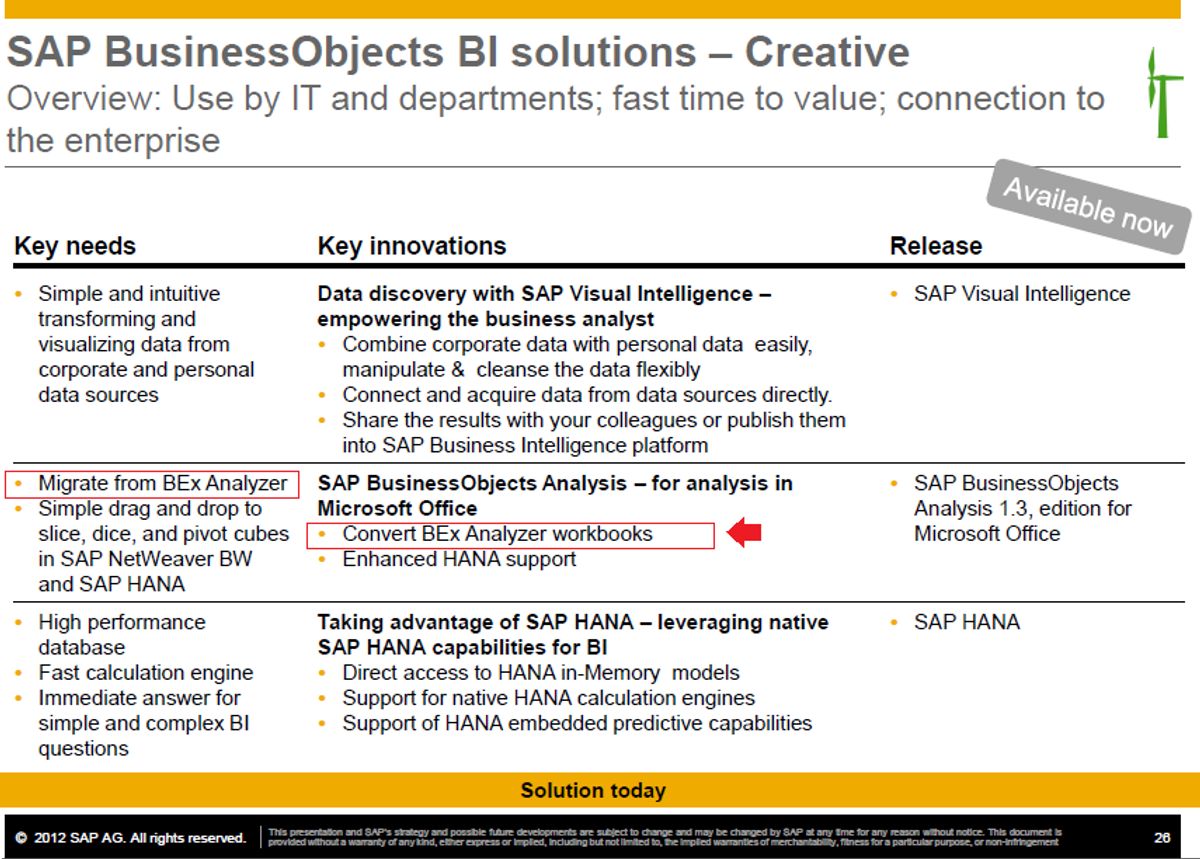
From Data Collection to Actionable Insights
The journey from raw data to actionable insights is a multi-stage process that transforms information into a powerful business tool. Data Collection and Preparation is the first critical step, where relevant data is gathered from diverse sources, including databases and social media. Ensuring the data's quality through meticulous cleaning and organization is essential for its subsequent usability.
Once the data is primed, Data Analysis takes center stage. Here, statistical and analytical techniques are applied to reveal patterns and relationships. Tools like data mining and machine learning are instrumental in this phase, leading to the generation of insights that drive informed decision-making within the organization.
The ultimate goal is to harness these insights to make better decisions that propel the business forward.
Finally, Data Visualization and Communication play a pivotal role in making the insights accessible. Interactive visualizations and advanced BI tools not only make the data easier to understand but also facilitate collaboration and enhance decision-making across the organization.
The Role of AI in Enhancing BI Capabilities
Artificial Intelligence (AI) has become a cornerstone in the evolution of Business Intelligence (BI), offering unprecedented capabilities in data analysis and insight generation. AI-driven BI tools are now essential for organizations seeking to harness the full potential of their data. By integrating AI, businesses can automate complex analytical processes, enabling quicker and more accurate decision-making.
Pattern detection and behavior recognition are two areas where AI significantly amplifies BI capabilities. Through machine learning, AI can analyze large and complex datasets to identify trends and anomalies that might go unnoticed by human analysts. This leads to more informed strategic decisions and a competitive edge in the market.
- Enhanced data processing speed
- Improved accuracy in predictions
- Real-time analytics and reporting
- Personalized insights for different user levels
AI is not just transforming BI tools; it's redefining the way organizations of all sizes interact with data. The democratization of data insights ensures that every level of an organization can make data-driven decisions.
As we look to the future, the synergy between AI and BI is poised to further revolutionize business decision-making. The integration of emerging technologies like IoT and edge computing with BI platforms will likely open new frontiers in business analytics, making it an exciting time for businesses ready to embrace these changes.
Integrating BI Across Organizational Departments
The integration of Business Intelligence (BI) tools across various organizational departments is a critical step in harnessing the full potential of data-driven strategies. Seamless data integration is essential for creating a unified view of the company's operations, which in turn empowers decision-making with strategic insights. By ensuring that all departments have access to the same high-quality data, businesses can enhance collaboration, streamline operations, and master analytical capabilities for organizational value and competitive advantage.
Data governance plays a pivotal role in this integration process. It ensures that useful data is made accessible and available to multiple business units simultaneously, fostering an environment where data is not just collected, but also effectively managed and monitored around the clock. This approach is crucial for maintaining data integrity and reliability across the organization.
The impact of BI in driving business success cannot be overstated. It transforms the way departments interact with data and with each other, breaking down silos and encouraging a culture of informed decision-making.
To achieve effective BI integration, consider the following steps:
- Create an in-house team for strategy, involving department heads and executives from all business units. Assign Key Performance Indicators (KPIs) to achieve clear objectives for each department through a comprehensive BI strategy.
- Team up with a competent BI service vendor, especially if internal resources and expertise are lacking. Working with agile BI teams can be a cost-effective method to ensure top-to-bottom understanding and implementation of BI tools.
Data-Driven Decision Making: The Heart of BI
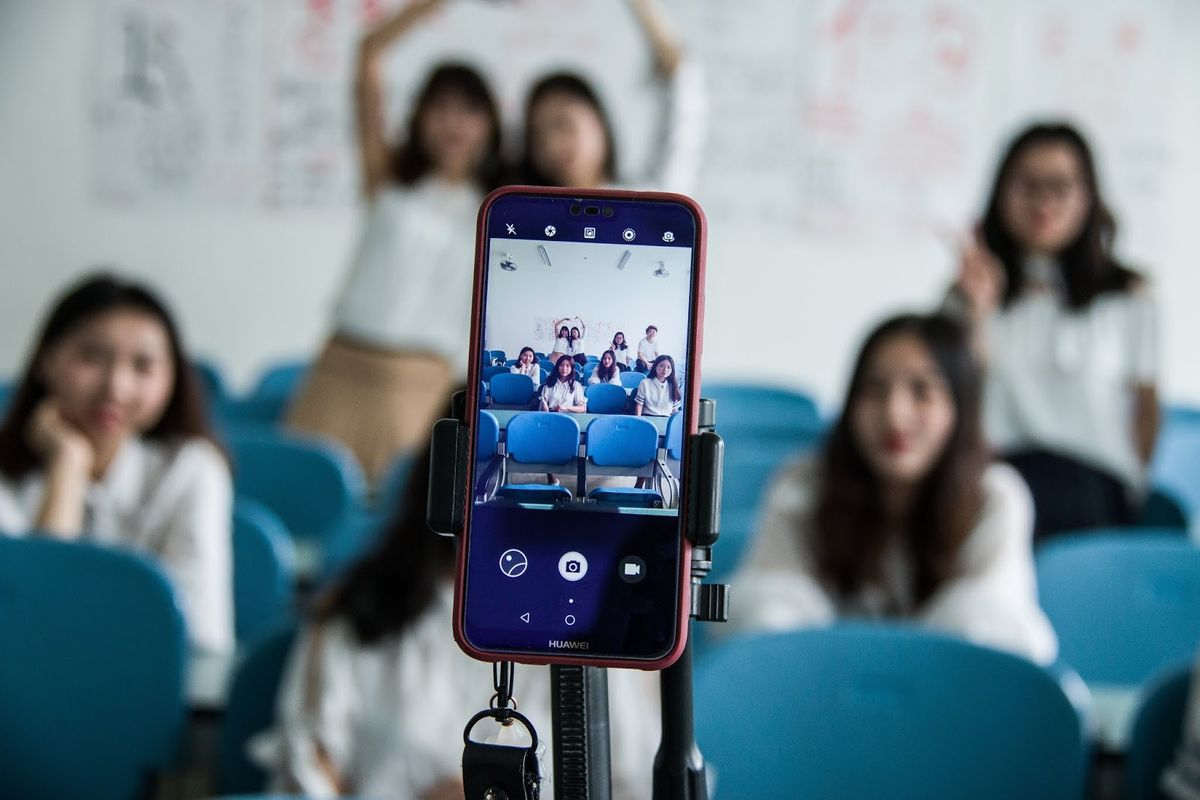
Defining Key Performance Indicators (KPIs)
Key Performance Indicators (KPIs) are the compass that guides businesses towards their strategic goals. Selecting the right KPIs is crucial as they reflect the organization's success factors and are essential for data-driven decision making. To define appropriate KPIs, businesses must align them with their specific objectives and utilize thorough data analyses to tailor solutions that meet their unique requirements.
Financial Performance: Monitoring financial health is a key aspect of BI. KPIs such as profitability, cash flow, and return on investment provide insights into financial stability and areas for improvement.
Risk Management: BI tools aid in identifying and mitigating risks by analyzing data from various sources, ensuring that KPIs related to risk thresholds are kept in check.
By setting clear KPIs, organizations can measure the impact and ROI of BI services, focusing on customer satisfaction, revenue growth, and operational efficiency.
To effectively track and analyze KPIs, businesses should:
- Identify business objectives.
- Conduct data analyses to determine relevant KPIs.
- Implement data visualization techniques for real-time monitoring.
- Perform pre/post implementation analysis to measure BI strategy success.
Ultimately, KPIs serve as a bridge between data and actionable insights, enabling businesses to steer their strategies with precision and clarity.
Leveraging Statistical Analysis and Machine Learning
In the realm of business intelligence, the fusion of statistical analysis and machine learning has become a cornerstone for predictive prowess. Statistical analysis provides the groundwork for understanding historical data, while machine learning offers the agility to adapt and learn from new data as it emerges. Together, they form a powerful duo that can not only interpret past trends but also anticipate future events with remarkable accuracy.
Statistical modeling, regression analysis, and time series analysis are just a few of the tools that businesses employ to forecast and prepare for what lies ahead. Machine learning models further refine these predictions by continuously learning from data, leading to more nuanced and precise insights.
By integrating both statistical and machine learning techniques, organizations can transform raw data into strategic foresight, driving informed decisions that propel business growth.
The following table illustrates the complementary roles of various analytical methods:
Analytical Method | Purpose |
---|---|
Descriptive Analytics | Understand past performance |
Predictive Analytics | Forecast future trends |
Prescriptive Analytics | Recommend actions |
Embracing these methods enables businesses to not only react to market dynamics but also to proactively shape their future. The Battle of the Brains between business intelligence and predictive analytics is not about one replacing the other, but about leveraging both to optimize resource allocation and drive growth.
The Importance of Data Quality in Analytics
The foundation of any analytics initiative is the quality of the data being analyzed. High-quality data is essential for accurate and reliable insights, which in turn drive informed decision-making. To ensure data integrity, businesses must implement robust data governance policies and regular data quality checks.
Data quality challenges can significantly impact the outcomes of business intelligence efforts. Inaccurate or inconsistent data can lead to skewed analytics and potentially erroneous decisions. A 2021 report by Gartner highlights the financial implications, with businesses losing an estimated $15 million annually due to poor data quality.
Maintaining the integrity and uniformity of data input is paramount. Companies need to establish a data governance framework that can monitor and maintain data standards.
To overcome these challenges, businesses can adopt best practices and continuously learn to integrate emerging BI trends, such as AI, into their data analysis processes. This requires a commitment to continuous improvement and education for BI professionals.
Data Visualization and Reporting Techniques
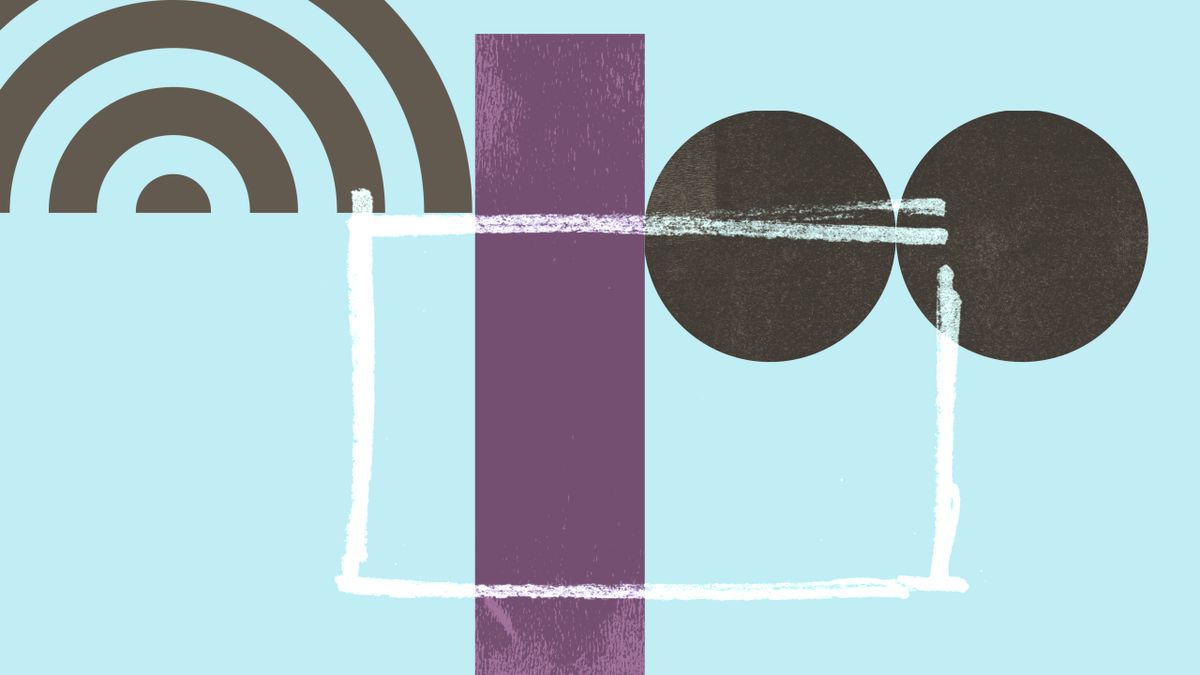
Creating Compelling Data Visualizations
In the realm of Business Intelligence, data visualization is a critical step that transforms complex datasets into clear and impactful visual stories. By leveraging the right visual tools, businesses can highlight key findings and convey intricate insights in a manner that is accessible to all stakeholders.
Charts, graphs, and interactive dashboards are not just tools for presentation; they serve as a bridge between data and decision-making. The effectiveness of a visualization is measured by how intuitively it communicates the data's story, prompting the necessary actions from its audience.
Effective data visualization goes beyond aesthetics; it is about making data comprehensible and actionable.
Here are some principles to consider when creating visualizations:
- Understand your audience and their information needs.
- Choose the right type of visualization for your data.
- Use color and design elements strategically to highlight important data points.
- Ensure that the visualization is accurate and free of misleading representations.
By adhering to these principles, organizations can create visualizations that not only capture attention but also drive informed business decisions.
Effective Reporting for Stakeholder Engagement
Effective reporting is not just about presenting data; it's about telling a story that resonates with stakeholders. By leveraging big data, businesses can craft compelling narratives that transform data into actionable knowledge. This process involves data visualization and storytelling techniques that highlight key findings and insights.
Data Visualization and Communication play a pivotal role in stakeholder engagement. Visual representations such as charts, graphs, and dashboards make complex information accessible and understandable at a glance. Here's a simple breakdown of the reporting process:
- Collect and analyze data relevant to business objectives.
- Employ data visualization techniques to summarize findings.
- Communicate insights through clear and intuitive reports.
- Implement and monitor decisions based on the reported insights.
By streamlining reporting processes with advanced analytics tools, businesses can stay ahead of changing regulations and demonstrate a commitment to ethical practices.
Ultimately, effective reporting enables stakeholders to make informed decisions, identify areas for improvement, and strategize for future growth. It is a continuous cycle of analysis, visualization, decision-making, and monitoring that drives business success.
Real-Time Insights for Informed Decision Making
The agility of an organization hinges on its ability to make swift, informed decisions. Real-time insights serve as the backbone for this agility, transforming raw data into a strategic asset. With the advent of self-service analytics tools, the time from data collection to decision-making has been drastically reduced.
Real-time monitoring of processes enables immediate identification of issues, allowing for quick corrective actions. This capability ensures that performance metrics such as cycle times and error rates are not just measured, but actively managed for continuous improvement.
The integration of real-time data analytics into business operations is a game-changer, empowering organizations to act on immediate insights and maintain a competitive edge.
Here is a simple representation of the benefits of real-time insights:
- Faster response to market changes
- Proactive issue resolution
- Enhanced collaboration across departments
- Continuous process optimization
By leveraging these insights, businesses can predict market conditions, gain a competitive advantage, and make data-driven decisions that are both timely and impactful.
Business Intelligence vs. Business Analytics
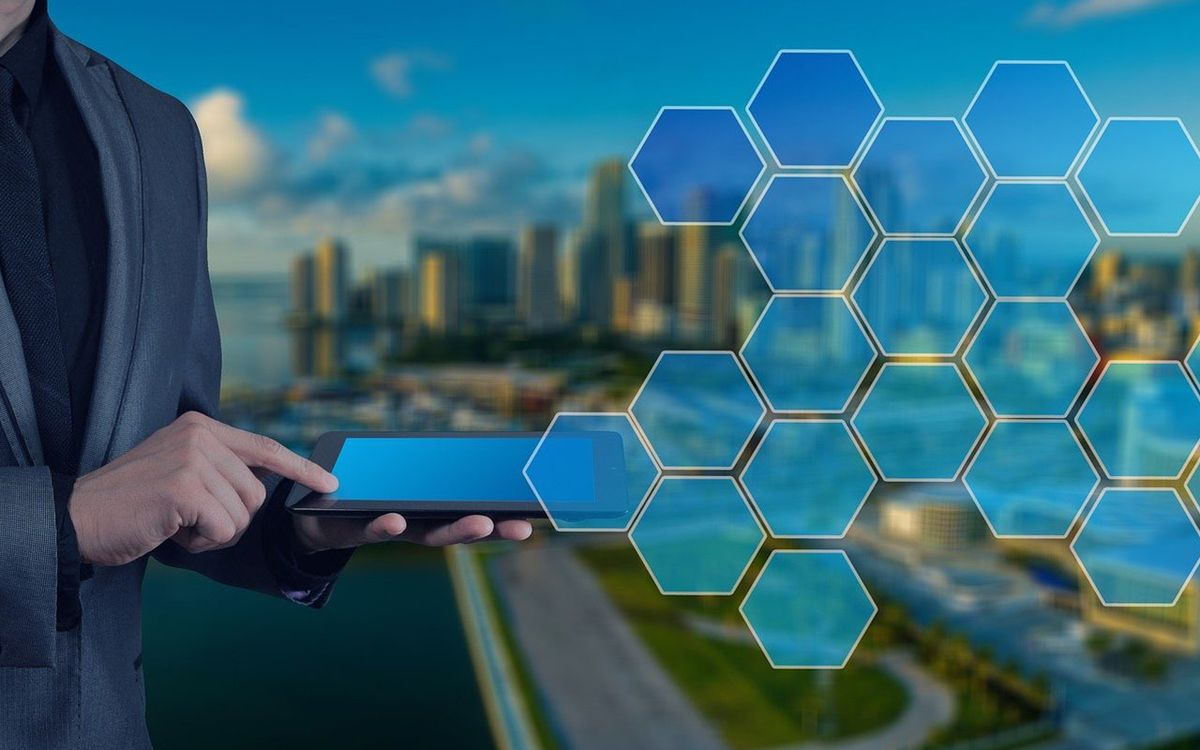
Understanding the Distinctive Features
The distinction between Business Intelligence (BI) and Business Analytics (BA) is fundamental to leveraging data effectively. BI focuses on the past and present, providing descriptive and diagnostic insights that inform current operations. In contrast, BA is forward-looking, utilizing predictive and prescriptive analytics to shape future strategies.
- Descriptive Analytics: Understand what happened.
- Diagnostic Analytics: Explore why it happened.
- Predictive Analytics: Forecast what might happen.
- Prescriptive Analytics: Advise on possible outcomes.
The synergy between BI and BA enables a comprehensive view of business performance, guiding both tactical and strategic decision-making.
While BI tools prioritize data accessibility and user-friendliness, BA tools delve deeper into data, uncovering patterns and correlations that drive sophisticated decision-making. Selecting the right approach hinges on the specific needs and maturity of the organization's data-driven culture.
How Each Drives Different Aspects of Business Growth
Understanding the unique contributions of Business Intelligence (BI) and Business Analytics (BA) is crucial for leveraging their full potential in driving business growth. BI is primarily about monitoring and understanding current performance, while BA focuses on applying statistical analysis and predictive modeling to drive change and predict future outcomes.
Speed is a key differentiator in how these tools impact business growth. BI tools enable rapid insight generation, which leads to faster decision-making and promotes organizational agility. On the other hand, BA often involves more complex analysis, but it can forecast trends and inform long-term strategic planning.
Fostering a data-driven organizational culture is essential. It empowers employees to utilize analytics in their roles, leading to significant improvements in customer acquisition and retention.
The integration of BI and BA can lead to a synergistic effect on business growth:
- BI: Enhances operational efficiency and customer experiences through real-time data monitoring.
- BA: Drives sustainable growth by predicting market trends and customer behavior.
By strategically combining the strengths of BI and BA, businesses can unlock a wealth of insights and achieve a competitive edge in the market.
Selecting the Right Approach for Your Business
Choosing between Business Intelligence (BI) and Business Analytics (BA) hinges on understanding their unique contributions to your business's growth. Business Intelligence is about looking back and interpreting what has happened, while Business Analytics focuses on looking forward and predicting what will happen. This distinction is crucial for aligning the right approach with your business objectives.
- BI is typically used for:
- Reporting historical data
- Descriptive analytics
- Dashboard creation
- BA is more about:
- Predictive modeling
- Prescriptive analytics
- Advanced data mining
The key is to integrate the insights from BI to inform the strategies developed through BA, creating a comprehensive data-driven decision-making framework.
Selecting the right approach also involves evaluating your current data infrastructure, the skill set of your team, and the specific industry challenges you face. It's not just about the tools, but how they are implemented and embraced across the organization. The table below summarizes the primary focus of each approach:
Approach | Primary Focus |
---|---|
BI | What happened |
BA | What will happen |
Ultimately, the choice between BI and BA should be made with a clear understanding of your business's needs and the potential of each approach to meet those needs.
Developing a Robust Data Strategy
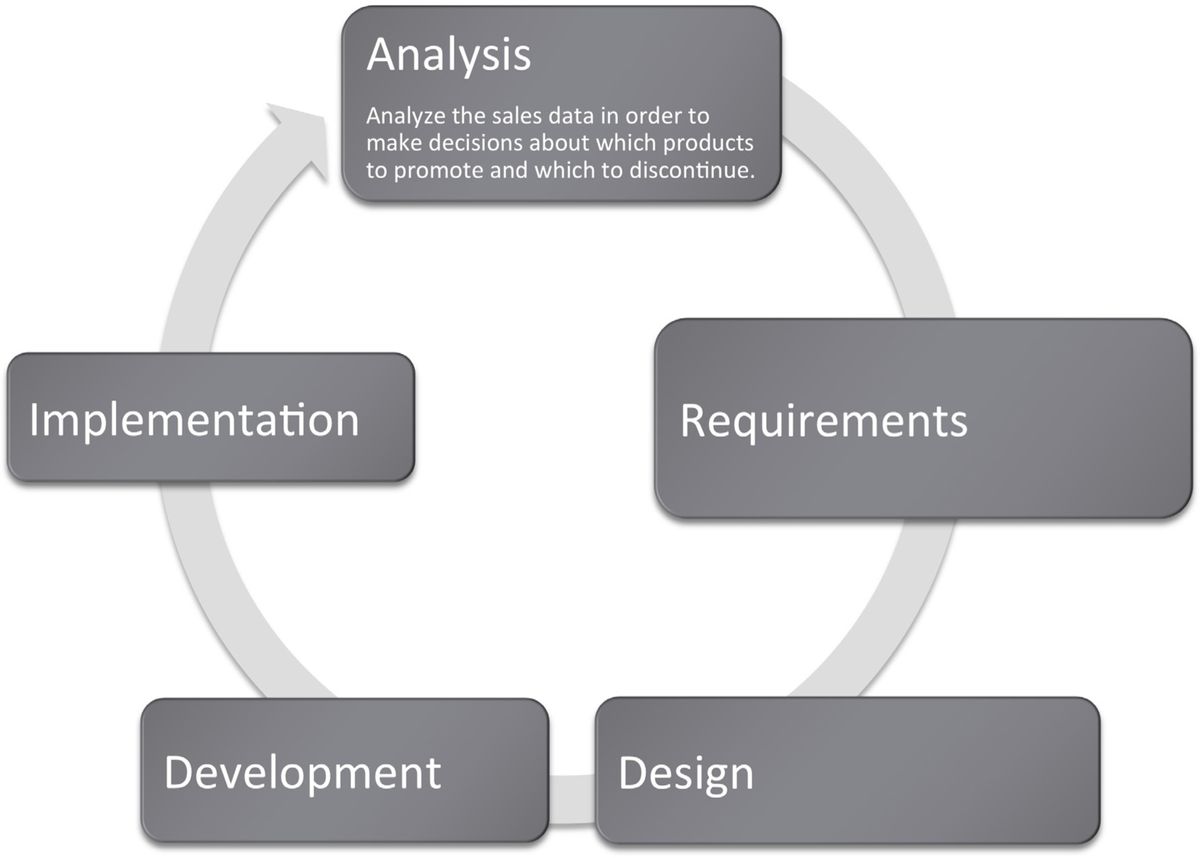
Aligning Data Goals with Organizational Objectives
In the pursuit of a robust data strategy, the alignment of data goals with organizational objectives is paramount. Organizations must ensure that their data initiatives support and drive the overarching business strategy. This alignment is not a one-time event but an ongoing process that involves continuous evaluation and adjustment.
- Define the concept of data-driven decision-making
- Formulate a data strategy aligned with organizational goals
- Aligning Data Strategy with Business Goals
To effectively measure the success of data strategies, it is essential to establish clear metrics that reflect the organization's priorities. Measurement is a critical step that provides feedback on the progress and effectiveness of the data initiatives. According to TriNet, it's of the utmost importance to measure your progress when aligning your HR goals with your business.
Fostering a data-driven organizational culture is a key factor in the successful implementation of data strategies. It empowers employees to utilize self-service analytics and make informed decisions that contribute to the achievement of business objectives.
Evaluating and Selecting Data Collection Methods
The foundation of any robust Business Intelligence (BI) system lies in the meticulous selection of data collection methods. Choosing the right data sources and collection techniques is crucial for ensuring the relevance and quality of the data that will feed into analytics and decision-making processes. It's essential to consider both structured data from databases and unstructured data from sources like social media.
The process of data collection should be aligned with the business's strategic goals, ensuring that the data gathered is not only accurate but also actionable.
When evaluating data collection methods, it's important to weigh factors such as the volume of data, the frequency of updates, and the ease of integration with existing systems. A structured approach to data collection and management can significantly enhance the efficiency of the BI process. Below is a list of steps that are typically involved in data collection and preparation:
- Identification of relevant data sources
- Ensuring data quality through cleaning and organization
- Data storage and management solutions
- Best practices for data management
Automation and data-driven strategies are essential for business success. Implement clear data governance, invest in technology, and leverage analytics for maximizing data potential and driving innovation.
Ensuring Data Accessibility and Governance
In the realm of Business Intelligence, ensuring data accessibility and governance is not just a regulatory requirement but a strategic asset. Data governance is crucial for data integrity, security, and compliance. It involves partnerships, best practices, privacy standards, and ethical frameworks. Encryption is essential for data security, safeguarding information from unauthorized access and breaches.
To maintain high data quality, systematic checks must be implemented to verify data accuracy. This is vital as decisions are only as good as the data they're based on. A 2021 Gartner report highlighted that businesses lose an estimated $15 million annually due to poor data quality.
Upholding robust data governance policies is imperative. These policies set up protective actions for sensitive data, ensuring consistency, accuracy, and safety.
Here are key steps to enhance data governance:
- Implement systematic checks to verify data accuracy.
- Guarantee that data is current and relevant to your needs.
- Set clear instructions to maintain uniformity across data sets.
- Apply strict data governance rules to comply with privacy regulations.
The Impact of BI on Competitive Advantage
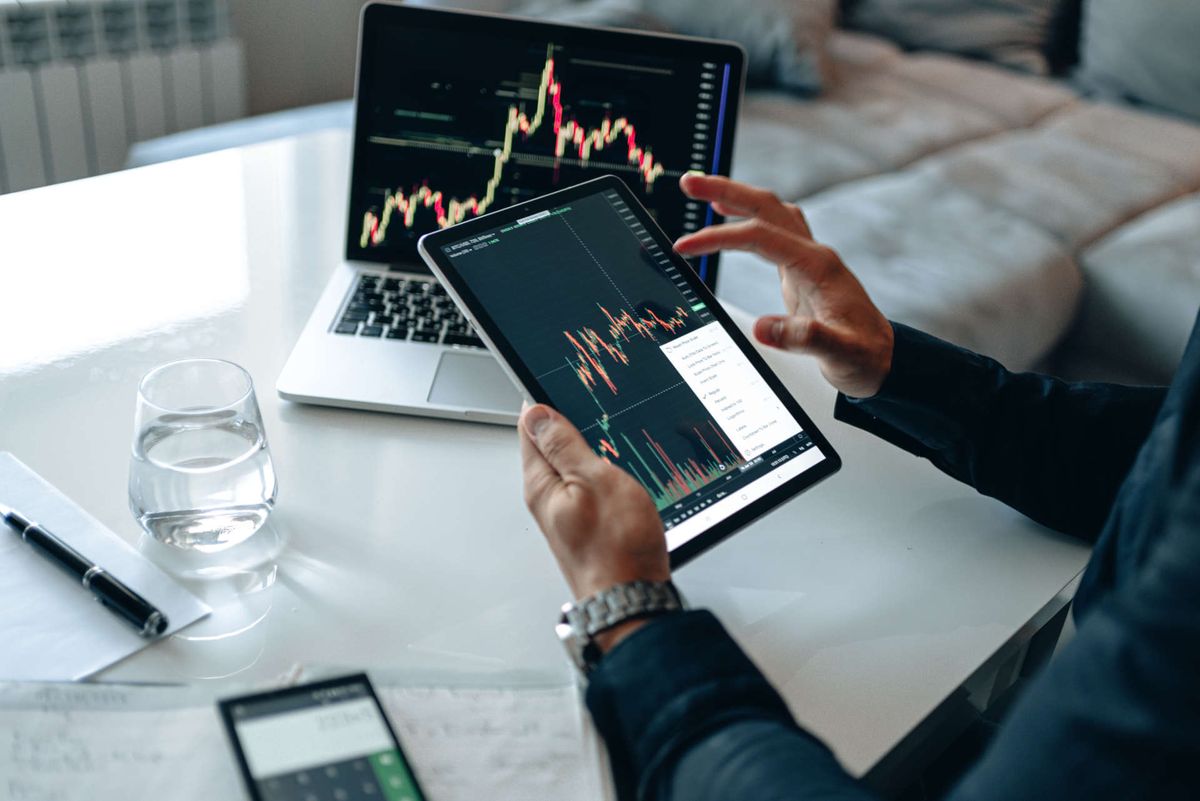
Gaining Insights for Operational Improvement
Operational efficiency is the cornerstone of a thriving business. By harnessing the power of business intelligence (BI), companies can identify inefficiencies and optimize processes to enhance productivity. BI tools enable organizations to analyze vast amounts of data, revealing trends and insights that lead to smarter operational decisions.
Real-time analytics play a crucial role in operational improvement. They provide immediate feedback on performance metrics, such as cycle times and error rates, allowing for swift identification and resolution of issues. This continuous monitoring facilitates ongoing process enhancements and can significantly improve quality control in manufacturing and other sectors.
The benefits of BI in operational improvement are clear:
- Streamlined data collection and analysis
- Reduced operational costs
- Increased productivity and quality
- Faster insights and decision-making
By prioritizing data-driven strategies, businesses can not only keep pace with market conditions but also gain a competitive advantage. The implementation of BI is not without its challenges, yet the potential for transformative impact on operations is immense.
Maintaining an Edge in a Data-Driven Market
In the relentless pursuit of competitive advantage, businesses must harness the power of data analytics to stay ahead. Dynamic pricing is a prime example of how real-time data can be leveraged to adjust prices based on demand and market conditions, ensuring optimal profitability and market responsiveness.
Prescriptive analytics plays a pivotal role in this landscape, guiding companies to not only interpret data but also to take decisive, proactive steps. This approach is essential for businesses aiming to outperform their rivals and secure their position in the market.
By understanding market trends and customer behavior through data analytics, companies can craft strategies that are both responsive and predictive, giving them the foresight to lead rather than follow.
Here are some key benefits of utilizing data analytics for maintaining a competitive edge:
- Informed predictions about future market conditions
- Enhanced customer insights for better targeting and retention
- Streamlined processes for improved operational efficiency
- Identification of trends and opportunities for future growth and innovation
Case Studies: Successful BI Implementations
The transformative effect of Business Intelligence (BI) is best illustrated through real-world applications. Early involvement of key stakeholders and a dedicated BI team are pivotal in navigating the complexities of BI projects. A robust data infrastructure underpins the agility and scalability of these initiatives.
Impact of BI on Business Success
- Data Visualization: Essential for tracking KPIs and enabling real-time monitoring on dashboards.
- Pre/Post Analysis: Conducting analyses before and after BI implementation reveals tangible performance improvements.
Modern enterprises that harness cutting-edge technologies and predictive analytics not only refine their decision-making processes but also secure a significant competitive edge. The synergy between advanced BI tools and organizational strategy leads to enhanced productivity and profitability.
The integration of BI services across departments ensures a unified approach to data management, fostering continuous improvement and strategic alignment.
AI and BI: A Synergistic Relationship
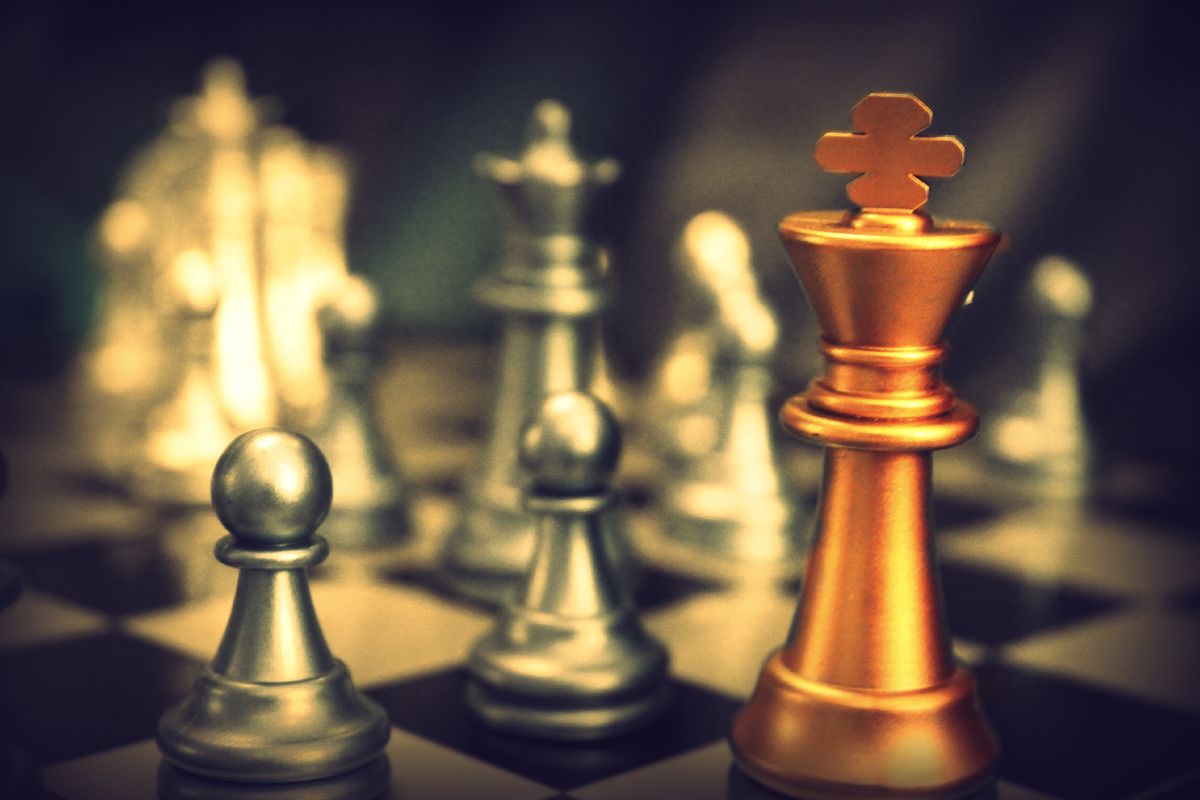
Exploring AI Opportunities in Business Intelligence
The integration of Artificial Intelligence (AI) into Business Intelligence (BI) tools is revolutionizing analytics, enabling a new era of decision-making capabilities. AI's role in BI extends beyond mere data analysis; it fosters a transformative environment where insights lead to smarter investments and enhanced customer experiences.
- Promote a culture of data-driven thinking and collaboration
- Demonstrate adaptability to emerging technologies
- Overcome challenges with resilience and a problem-solving attitude
AI is currently transforming how users interact with BI platforms, making it accessible for all organizational levels to harness valuable insights.
The synergy between AI and BI is evident in the way AI-powered analytics improve pattern detection and behavior recognition, making BI tools more intuitive and user-friendly. As we look to the future, the fusion of BI with technologies like IoT and edge computing promises to further amplify BI capabilities, offering unprecedented opportunities for businesses to grow and innovate.
Prioritizing AI Technologies for Maximum Impact
In the quest to harness the full potential of AI in Business Intelligence, it's crucial to prioritize AI technologies that align with specific business needs. A strategic approach involves evaluating AI opportunities and selecting technologies that offer the most significant benefits for data-driven decision-making.
- Explain why AI technology might be a good fit in various use cases
- Promote a culture of data-driven thinking within the organization
- Demonstrate adaptability to emerging technologies
- Overcome challenges with resilience
- Adopt AI technologies to support everyday activities
The trustworthiness of AI systems is paramount, as it influences user confidence and the reliability of insights derived from AI-enhanced BI tools. Factors such as accuracy, transparency, fairness, and robustness are critical in ensuring trust in AI applications. By prioritizing these aspects, businesses can foster a culture of ethical AI use and responsible development.
By carefully selecting specific AI technologies and integrating them into BI processes, companies can unlock new levels of efficiency and insight. This strategic focus on AI can lead to intelligent forecasting, customer evaluation, and cost optimization, ultimately driving business growth.
Integrating AI to Enhance BI Tools and Processes
The integration of Artificial Intelligence (AI) into Business Intelligence (BI) tools is revolutionizing the landscape of data analytics. AI's capability to process and learn from large, complex datasets has significantly improved pattern detection and behavior recognition within BI platforms. This transformation is not just about technology; it's about empowering every individual within an organization to access and leverage valuable insights and key metrics effortlessly.
Explain why AI technology might be a good fit in various use cases
- Promote a culture of data-driven thinking
- Encourage collaboration and knowledge sharing
- Demonstrate adaptability to emerging technologies
- Overcome challenges with resilience
- Appreciate the value of Power BI
- Apply AI technologies to support daily activities
The synergy between AI and BI is creating a self-service model where ad-hoc data analysis and actionable insights are readily available. This evolution is marked by the advent of augmented analytics and advanced data processing, which are embedded within the fabric of complex business processes. As we look to the future, the continued integration of AI will make BI tools more intuitive, user-friendly, and capable of extracting valuable insights with unprecedented efficiency.
Mastering Power BI for Business Solutions
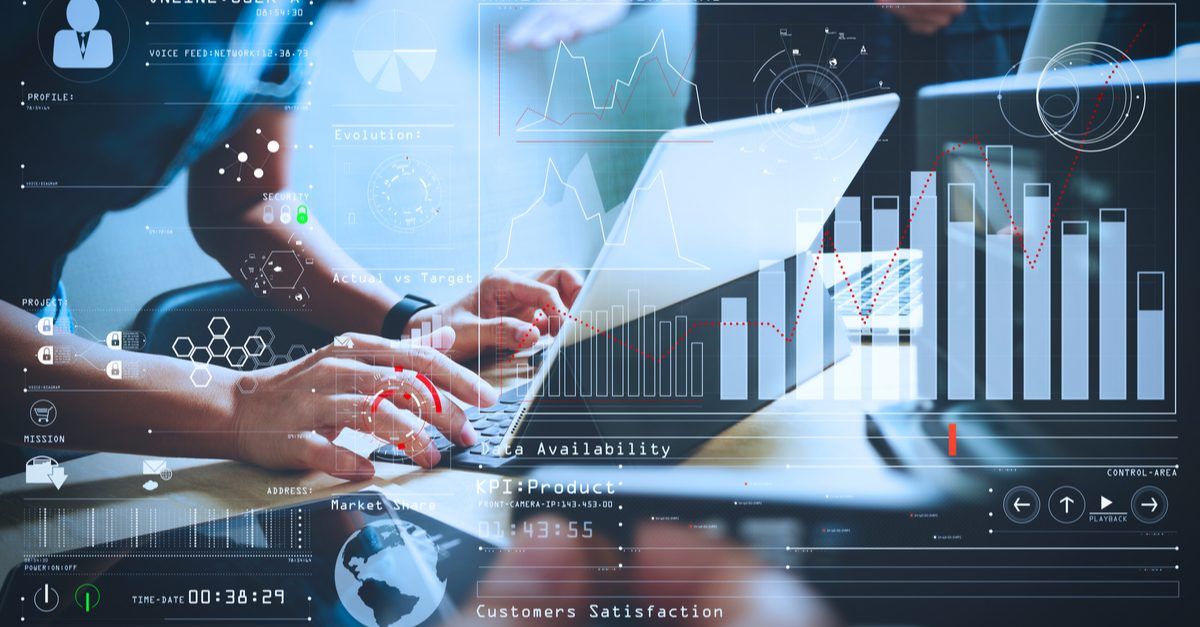
Importing and Cleaning Data in Power BI
The initial phase of leveraging Power BI for business solutions begins with importing and cleaning data, a critical step that sets the stage for all subsequent analytics. Power BI's Query Editor is an essential tool for transforming raw data into a format ready for analysis. This process includes removing duplicates, correcting errors, and standardizing data formats to ensure accuracy and consistency.
The integrity of business intelligence insights is directly tied to the quality of the data input. Hence, meticulous data preparation is non-negotiable.
Here are the key steps involved in data preparation within Power BI:
- Connect to various basic data sources, such as Excel files or databases.
- Load the data into Power BI Desktop.
- Utilize the Query Editor for data transformation and cleansing.
- Create necessary tables, including a Date table for time-based analysis.
By following these steps, users can build a solid foundation for creating a comprehensive business intelligence tool that effectively supports data-driven decision-making.
Performing Advanced DAX Calculations
Mastering DAX (Data Analysis Expressions) is crucial for extracting deeper insights from data within Power BI. Advanced DAX calculations allow for more complex and nuanced data analysis, going beyond basic sums and averages to include time intelligence, conditional logic, and more sophisticated aggregations.
To effectively perform advanced DAX calculations, one must understand the context of calculations, which includes filter context and row context. These contexts determine how calculations are performed on a dataset. For instance, a measure that calculates total sales will behave differently when placed in a table that has a filter applied for a specific year.
Here's a simple example of a DAX formula:
Measure | DAX Formula |
---|---|
Total Sales | SUM(Sales[Amount]) |
Yearly Growth | CALCULATE(SUM(Sales[Amount]), SAMEPERIODLASTYEAR(Sales[Date])) |
By mastering DAX, analysts can create powerful data models that provide actionable insights, driving informed decision-making across the business.
It's also important to recognize the role of DAX in creating calculated fields and measures that can dynamically adjust as data is filtered and sliced within reports. This dynamic nature of DAX is what makes it a powerful tool in the hands of data analysts and business intelligence professionals.
Visualizing Data for Business Problem Solving
Effective data visualization is a cornerstone of business intelligence, transforming complex datasets into clear and actionable insights. Visual representations such as charts, graphs, and dashboards are not just tools for analysis; they are essential for communication. By presenting data in an intuitive format, stakeholders can quickly grasp the underlying trends and patterns that are critical for informed decision-making.
Visualizations serve as a bridge between raw data and strategic action, enabling businesses to distill vast amounts of information into digestible formats. Here are some key steps in the data visualization process:
- Identify the key data points relevant to the business question.
- Choose the appropriate type of visualization for the data.
- Design the visualization for clarity and impact.
- Interpret the visualization to extract meaningful insights.
- Communicate the findings to stakeholders effectively.
The goal of data visualization is not merely to show numbers but to tell a story that drives the business forward. It is through this lens that data becomes a powerful tool for problem-solving and strategic planning.
The Future of Business Intelligence Services
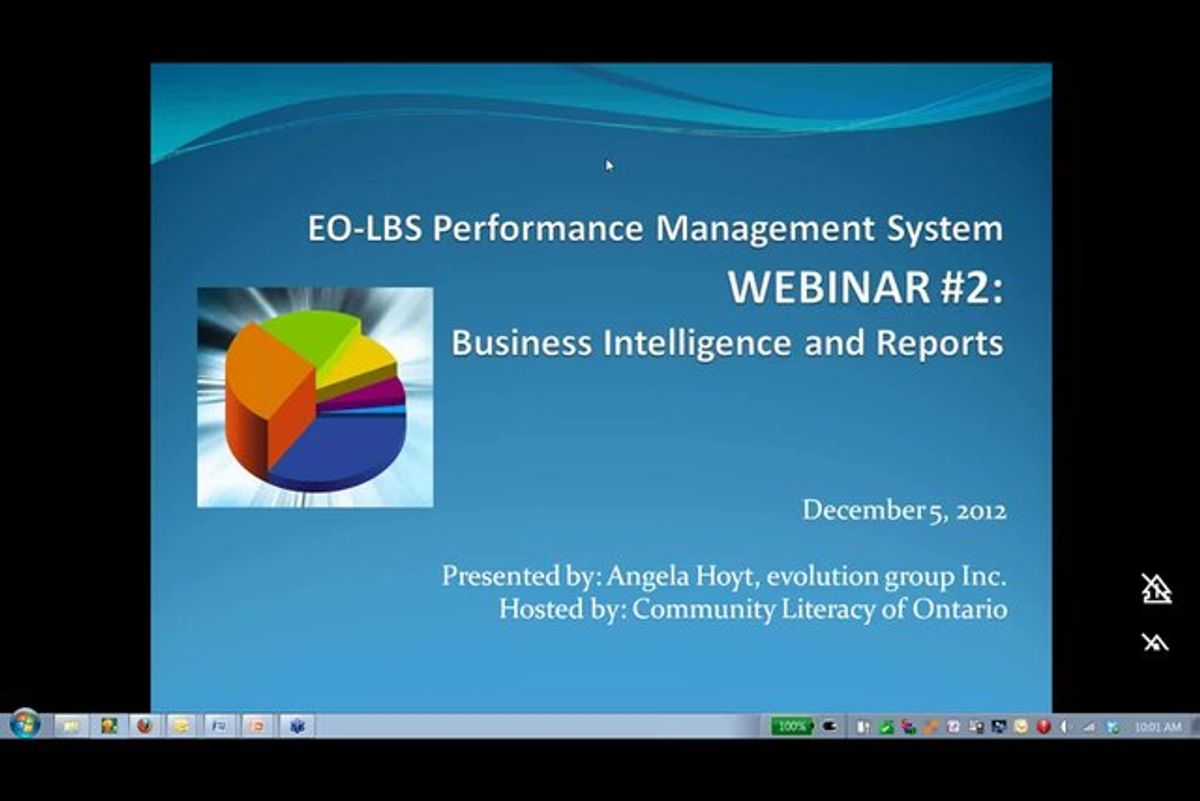
Trends Shaping the Evolution of BI
The landscape of Business Intelligence (BI) is continuously evolving, driven by technological advancements and changing business needs. Key trends in BI include the integration of AI, augmented analytics, and advancements in data visualization. These trends are not just reshaping the tools we use but also the way we approach data ethics and predictive modeling.
- AI integration is revolutionizing the predictive capabilities of BI tools, making them more proactive than reactive.
- Augmented analytics are simplifying the data analysis process, allowing users to gain insights with less technical expertise.
- Data visualization advancements are enabling clearer communication of complex data to stakeholders.
- Ethics in analytics is becoming a focal point, ensuring responsible use of data.
- Predictive modeling is shifting from a niche skill to a mainstream BI feature.
Staying updated with these emerging trends is crucial for businesses to maintain a competitive edge. The democratization of BI tools has allowed for a self-service model, where ad-hoc data analysis can be performed by business users, fostering a data-driven culture across organizations.
The integration of BI with other emerging technologies like IoT and edge computing is set to further enhance capabilities, offering unprecedented levels of insight and operational efficiency.
The Role of Continuous Monitoring and Data Management
In the realm of Business Intelligence (BI), the adage 'the only constant is change' holds particularly true. Continuous monitoring and data management are critical for maintaining the accuracy and relevance of BI systems. As new data streams in and business landscapes evolve, BI tools and processes must be agile enough to adapt.
- Implement internal controls and audits for data quality.
- Stay updated with regulatory changes and adapt accordingly.
- Plan architectural designs that accommodate data analytics integration.
- Prevent potential disruptions with thorough testing.
Upholding robust data governance policies is essential not only for data protection but also for ensuring the integrity and reliability of BI insights.
Providing continuous and consistent training is another cornerstone of effective BI practice. It empowers employees to leverage BI tools to their full potential, fostering a data-driven organizational culture that thrives on informed decision-making.
Data Governance and Interdepartmental Collaboration
In the realm of business intelligence, data governance is the cornerstone that ensures the integrity and security of data across the entire organization. Robust data governance policies are not just about compliance; they are about creating a culture of data consistency and accuracy that supports informed decision-making.
To truly unlock the power of BI, organizations must foster seamless interdepartmental collaboration. This means breaking down silos and encouraging a culture where data is shared and leveraged collectively. A federated data architecture can be instrumental in achieving this, as it allows for a unified view of data while it resides in different systems.
By prioritizing data governance and collaboration, businesses can ensure that their BI efforts are aligned with their strategic goals, leading to more effective and efficient operations.
Here are key steps to enhance interdepartmental collaboration through data governance:
- Establish clear data governance frameworks.
- Implement data virtualization tools for a unified data view.
- Promote continuous and consistent training for knowledge workers.
- Encourage a culture of shared analytics and insights.
As the digital landscape evolves, the future of Business Intelligence Services is set to revolutionize the way companies harness data for strategic advantage. At OptimizDBA, we offer unparalleled database optimization consulting to ensure your business stays ahead of the curve. Experience transaction speeds that are exponentially faster, and witness a significant increase in performance with our proprietary methodology. Don't let your business fall behind in the data-driven world. Visit our website to learn how we can transform your data management and propel your business forward.
Conclusion
In conclusion, the journey to unlocking the power of data through Business Intelligence (BI) and Analytics is a transformative one for any organization seeking to thrive in today's data-driven economy. By developing comprehensive BI tools, prioritizing data quality, and leveraging modern analytics techniques, businesses can enhance their decision-making processes, operational efficiency, and competitive advantage. The strategies discussed provide a roadmap for integrating data into the core of business operations, ensuring that insights are not only gathered but also effectively applied to drive growth and success. As we have seen, the impact of BI is profound, offering the ability to mobilize and monetize data to achieve tangible business outcomes. It is clear that those who invest in BI and Analytics are well-equipped to navigate the complexities of the modern market and emerge as leaders in their respective industries.
Frequently Asked Questions
What is the difference between Business Intelligence and Business Analytics?
Business Intelligence (BI) primarily focuses on the past and present data to help businesses operate effectively, while Business Analytics (BA) looks at historical data to predict future trends and to inform strategic decision-making.
How can AI enhance Business Intelligence capabilities?
AI can process large volumes of data much faster than traditional methods, provide predictive analytics, automate routine tasks, and offer deeper insights through advanced data modeling and machine learning algorithms.
What are Key Performance Indicators (KPIs) in the context of BI?
KPIs are measurable values that demonstrate how effectively a company is achieving key business objectives. In BI, KPIs are used to assess the success of an organization in particular areas relevant to its goals.
Why is data quality important for analytics?
High-quality data is critical for analytics because it ensures accuracy in the insights derived from the data. Poor data quality can lead to incorrect conclusions, which can negatively impact business decisions and performance.
What role does data visualization play in BI?
Data visualization is a crucial component of BI as it helps to communicate complex data in an easily understandable format, enabling stakeholders to grasp insights quickly and make informed decisions.
How does Business Intelligence provide a competitive advantage?
BI provides a competitive advantage by offering real-time insights, identifying efficiency improvements, and enabling data-driven decision-making, which can lead to better business strategies and outcomes.
What is Power BI and how is it used in business solutions?
Power BI is a business analytics service by Microsoft that provides interactive visualizations and business intelligence capabilities with an interface simple enough for end users to create their own reports and dashboards.
What are the emerging trends in Business Intelligence services?
Emerging trends in BI services include the integration of AI and machine learning, continuous monitoring and data management, increased emphasis on data governance, and the drive towards making data accessible across various departments.