Harnessing the Power of Data Analytics Techniques
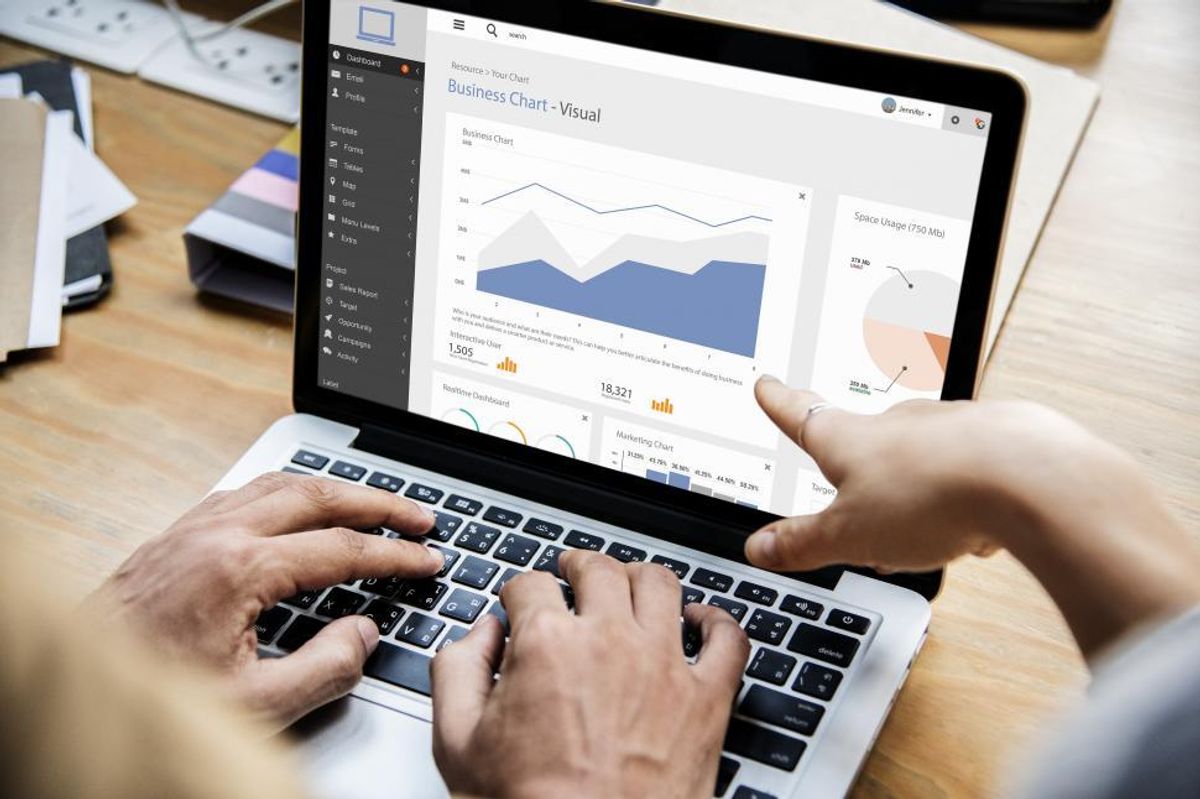
Data Extraction
The foundation of any advanced analytics strategy begins with data extraction. This process involves gathering data from a myriad of online and offline sources, ensuring that the raw material for insights is both comprehensive and relevant. Securing and integrating this data is crucial for maintaining its integrity and preparing it for the subsequent stages of analysis.
- Gather data from various sources
- Secure data transfer and integration
- Prepare a quality dataset for analysis
Once the data is collected, it undergoes a transformation process to refine it into a dataset that's primed for deeper examination. This step is essential for businesses to obtain a clean, organized, and actionable set of data. With the right tools and techniques, companies can turn their data into a valuable asset for data-driven decision-making.
The goal is to extract meaningful information that can guide strategic planning and optimize business operations.
Statistical Analysis
At the heart of transforming data into actionable insights lies the power of statistical analysis. This methodical approach involves the application of statistical techniques to interpret data, identify trends, and support hypothesis testing. Descriptive analysis is the starting point, providing a clear picture of the data through measures such as mean, median, and standard deviation.
Statistical analysis goes beyond mere number-crunching; it's about uncovering the story the data tells and the insights it holds.
Advanced techniques like regression analysis allow for the exploration of relationships between variables, offering predictions and deeper understanding. Factor analysis, another sophisticated tool, helps in identifying underlying influences that are not immediately apparent.
Here's a glimpse into how different statistical methods contribute to business insights:
- Descriptive Analysis: Summarizes data to understand its basic features.
- Regression Analysis: Explores relationships and predicts outcomes.
- Factor Analysis: Reveals hidden variables and patterns.
Leveraging data for business insights not only improves decision-making but also optimizes processes and identifies opportunities. Advanced analytics, through these statistical methods, unlocks valuable insights, providing a competitive edge and guiding strategic planning.
Predictive Modeling
At the forefront of advanced analytics is predictive modeling, a technique that leverages historical data and machine learning algorithms to forecast future events. Predictive analytics is not just about guessing; it's about making informed predictions based on data-driven insights.
Predictive modeling is the machine learning technique that would work best for any company that wants to predict the future outcomes for its business growth.
Regression Analysis is one such method under predictive modeling, crucial for understanding relationships between variables and predicting future trends. Here's a brief overview of how regression analysis can be applied:
- Identify the dependent variable you wish to predict.
- Select relevant independent variables that may influence the outcome.
- Collect and clean historical data for analysis.
- Develop a regression model to understand the relationships.
- Use the model to make predictions and inform decision-making.
The success of predictive modeling hinges on the quality of the data and the precision of the models. Inaccurate models or poor data quality can lead to misguided strategies and decisions. Therefore, it is imperative to ensure the integrity and accuracy of both the data and the models used.
Data Visualization
Data visualization stands as a cornerstone in the realm of data analytics, transforming complex datasets into clear and actionable insights. By leveraging visual analytics, decision-makers can quickly grasp intricate trends and patterns through dashboards, graphs, or charts. This not only streamlines the communication of insights but also democratizes data, making it accessible and central to strategic discussions.
Advanced data visualization incorporates innovative elements like heat maps and scatter plots, offering a multi-dimensional view of data. This enhances stakeholders' comprehension, ensuring insights are not just understood but also appreciated, leading to collaborative decision-making. However, the effectiveness hinges on choosing the right visual elements and presenting them with clarity. Here are some best practices:
- Entitle a specific audience and address their needs
- Choose the right visual to convey your message
- Apply text carefully to guide interpretation
Advanced data visualization is more than just aesthetics; it's about making data speak in a language that resonates with its audience.
While advanced visualization offers significant benefits, it's crucial to have a keen understanding of visualization principles to leverage it effectively. The selection of appropriate visuals and clarity of presentation are paramount to avoid misinterpretation and ensure the data's story is told compellingly.
The Crucial Role of Data Analytics in Decision-Making
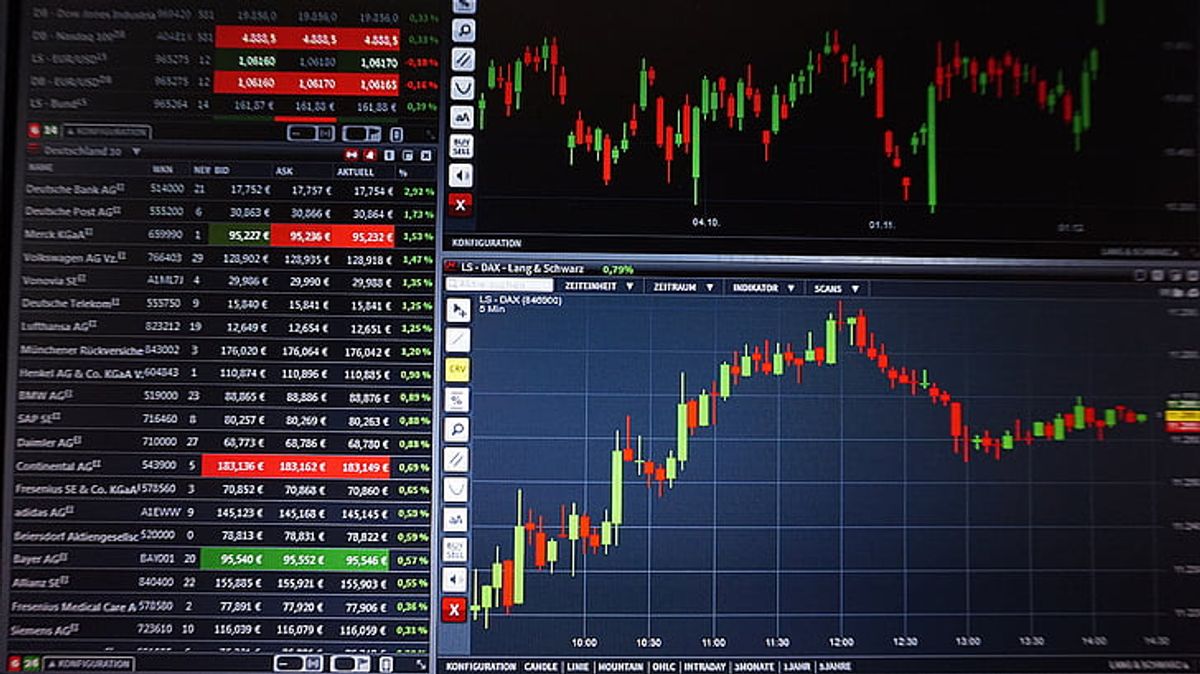
Leveraging Data for Decision-Making
In the fast-paced business environment, leveraging data for decision-making is essential for staying competitive. Data analytics transforms raw data into a strategic asset, enabling organizations to make informed decisions with confidence.
Data-driven decision-making is not just a buzzword; it's a cultural shift within organizations. By adopting this approach, companies can ensure that every decision is backed by evidence and insights derived from their data. This shift is facilitated by self-service analytics platforms, which empower decision-makers to access and interpret data without relying on data scientists.
The ability to make proactive decisions based on predictive analytics and real-time data is a game-changer for businesses. It allows for a more agile response to market changes and customer needs.
Here's how data analytics can guide decision-making:
- Identifying key performance indicators (KPIs) to align with business objectives.
- Sourcing data consistently for reliable statistical analysis.
- Providing decision support tools that deliver insights to all organizational levels.
- Fostering an organizational alignment where decisions are supported by solid evidence.
Guiding Businesses through Uncertainty
In the face of uncertainty, businesses must be agile, ready to adapt to the ever-changing market conditions. The ability to pivot and seize new opportunities is now more critical than ever. Reflecting on and improving how businesses respond to change is not just a one-time effort; it's an ongoing necessity.
The past few years have been a testament to the unpredictable nature of the global market, with businesses facing a relentless onslaught of challenges and crises.
Advanced analytics serve as a beacon, providing strategic guidance when the path ahead is unclear. By leveraging predictive modeling and analyzing complex market dynamics, businesses can chart a course through turbulent waters. Here are some ways analytics can guide decision-making:
- Identifying potential courses of action in the face of an uncertain future.
- Enhancing customer experiences to maintain loyalty during times of change.
- Securing data against cyber-attacks to protect the business's core assets.
Valuable Asset in Decision-Making
In the landscape of modern business, data analytics stands as a cornerstone, transforming raw data into a valuable asset for decision-making. It equips leaders with the clarity needed to steer their organizations towards success.
- Progress: Evidence-based organizational alignment in decision-making.
- Team: Collaboration between consultants and analysts to enhance decision quality.
Data analytics not only informs decisions but also shapes the very strategies that define competitive advantage.
By mapping out decisions and their potential outcomes, tools like Decision Tree Analysis aid in simplifying complex scenarios. This approach has proven its worth across various industries, from Gerber's strategic material choices to healthcare management decisions.
Enhancing Operational Efficiency with Advanced Analytics
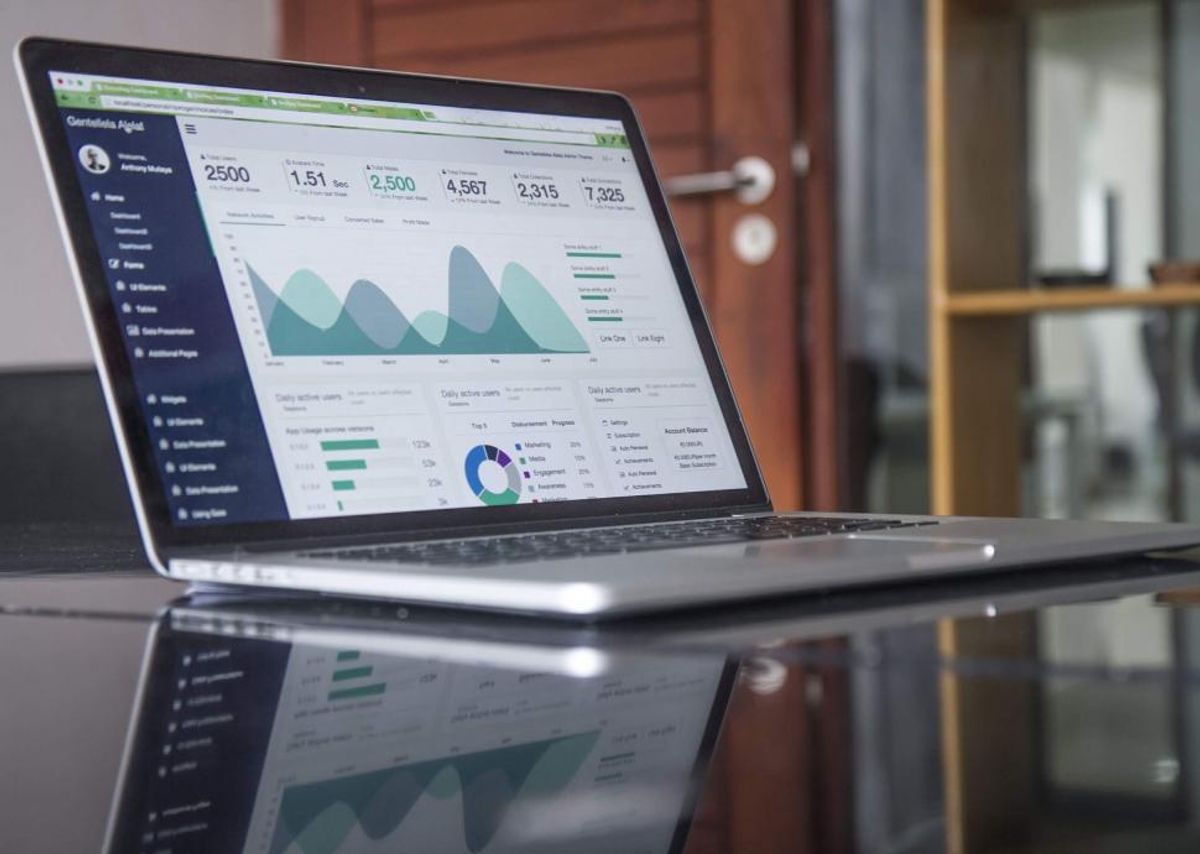
Optimizing Business Operations
In the quest for operational excellence, advanced analytics serve as a linchpin for identifying and rectifying inefficiencies within business processes. By dissecting data patterns and performance metrics, companies can pinpoint bottlenecks that hinder productivity and devise strategies to eliminate them, thereby enhancing the fluidity of operations.
Advanced analytics not only streamline workflows but also enable the effective allocation of resources, automating certain tasks to expedite processes and providing insights that guide human decision-makers.
For instance, consider the impact of analytics on resource allocation:
Resource Type | Before Analytics | After Analytics |
---|---|---|
Human Capital | Overutilized in some areas | Optimally distributed |
Financial | Wastage on non-critical tasks | Focused on growth areas |
Time | Consumed by manual processes | Saved by automation |
The implementation of business intelligence tools requires a strategic approach. Identifying key objectives and adopting best practices are essential steps to ensure that the integration of analytics translates into tangible operational improvements. As a result, businesses not only save on resources but also set the stage for sustained growth and a formidable competitive edge.
Improving Process Efficiency
In the realm of advanced analytics, improving process efficiency is a cornerstone for achieving a leaner, more agile business. By leveraging data analytics, companies can refine their allocation of resources, expediting and automating some processes while creating valuable insights to help human decision-makers navigate others.
Advanced analytics also automate some aspects of the decision-making process to make workflows quicker and nimbler. For example, automating credit scoring or fraud detection can significantly reduce manual interventions, translating to increased agility and operational efficiency.
However, it's crucial to avoid the trap of becoming too delivery-focused, where requests are made and fulfilled without strategic consideration. Optimization should be intentional, especially in areas like database design, to prevent blindly fulfilling requests that could be detrimental to overall results.
Driving Strategic Decision-Making
In the landscape of modern business, strategic decision-making is the cornerstone of success. Advanced analytics serve as a pivotal tool in this domain, enabling leaders to make informed decisions that are both timely and data-driven. By integrating advanced analytics and AI, particularly platforms like Keto AI+, organizations are not just reacting to market dynamics—they are anticipating them.
Data-driven decision making is more than a buzzword; it's a cultural shift within organizations. It involves a systematic approach to analyzing data, which includes the allocation of resources, automating processes, and generating insights that guide human decision-makers. This culture is essential for businesses aiming to maintain a competitive edge.
The ability to make proactive decisions based on historical evidence without significant additional analysis is a hallmark of progress in organizational alignment.
The use of tools such as Decision Tree Analysis exemplifies the practical application of analytics in strategic planning. It simplifies complex decision scenarios, mapping out options and their potential outcomes in a visual format that is easy to understand and act upon.
Decoding Data Analytics: A Game-Changer in Business
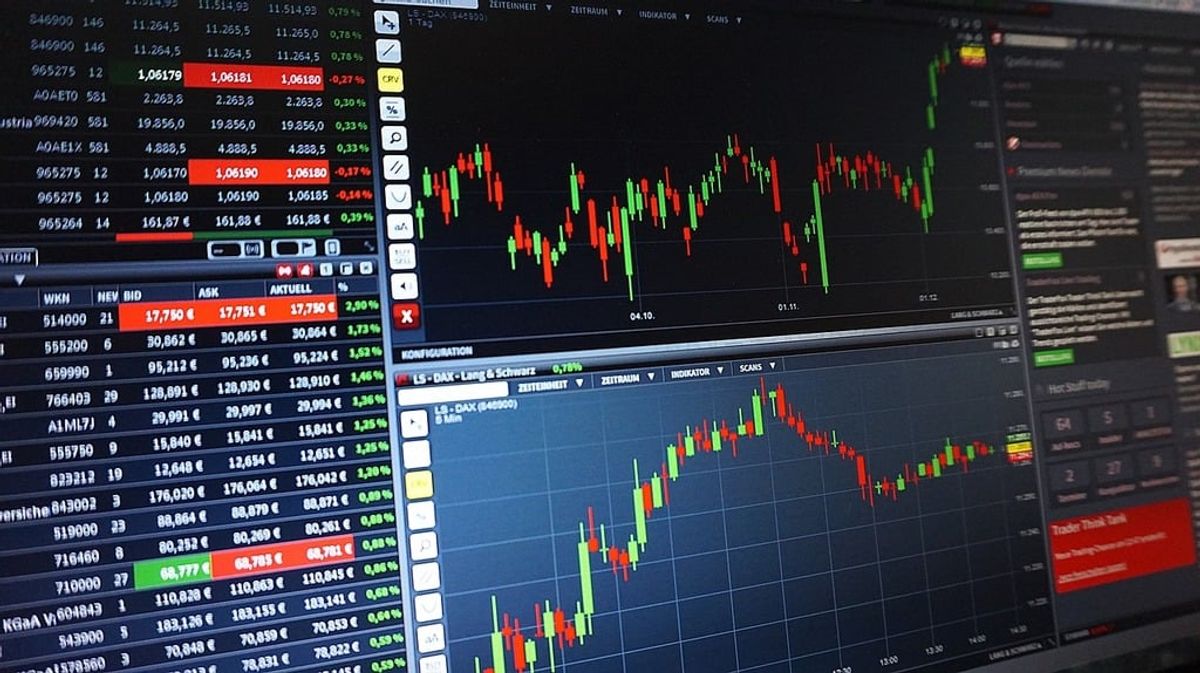
Unraveling Patterns
In the realm of data analytics, unraveling patterns is akin to deciphering a complex code. By meticulously analyzing the data, we can uncover the underlying structures that govern behaviors and trends. Hierarchical clustering, for instance, is a technique that groups similar data points into clusters, illuminating the proximity and relationships within the data.
Time Series Analysis is another critical method, especially when dealing with data collected over time. It helps in identifying cyclical patterns, trends, and even seasonality, which are invaluable for forecasting in various domains such as finance and economics.
The art of pattern recognition extends beyond mere analysis; it is about piecing together the disparate threads of data to weave a coherent narrative that can guide strategic decisions.
Understanding these patterns is not just an academic exercise but a practical tool for data-driven decision-making. As we delve deeper into the data, we begin to see the emergence of actionable insights that can transform businesses.
Predicting Trends
In the dynamic landscape of business, predicting trends is not just about looking at past patterns; it's about forecasting the future with precision and confidence. Predictive analytics harnesses historical data, statistical algorithms, and machine learning techniques to anticipate what's next. This foresight is invaluable for businesses aiming to stay ahead of the curve.
Regression analysis is a key player in this arena, modeling the relationship between variables to predict outcomes. It's a technique that has found its place across various industries, from finance to social sciences, helping to make informed predictions about future events.
The integration of AI has further enhanced predictive analytics tools, making them more accessible to both data scientists and business users. As we look towards 2024, here are six top tools identified by TechTarget:
- Tool A
- Tool B
- Tool C
- Tool D
- Tool E
- Tool F
Embracing these tools equips businesses with the ability to not only identify historic seasonal trends but also to forecast how these trends will evolve. This proactive approach can shape long-term business strategies, such as aligning content production with anticipated market demands.
Making Informed Choices
In the realm of business, data analytics is a game-changer, enabling companies to make choices that are not just reactive, but proactive and informed. By integrating Big Data into ERP systems, organizations achieve operational excellence, ensuring that every decision is backed by solid evidence and strategic insight.
- ADOPT A CULTURE OF DATA-DRIVEN DECISION MAKING
- Progress: Organizational alignment supported by evidence.
- Team: Consultant & Analyst
Decision Tree Analysis and Neural Networks are just two examples of the tools that can aid in mapping out decisions and their potential outcomes, providing a clear visual representation of complex scenarios. This approach is instrumental in simplifying decision-making processes, allowing for a more streamlined and effective strategy.
By leveraging advanced analytics tools and techniques, companies can uncover hidden patterns, trends, and correlations within their data, leading to more informed and strategic decision-making.
AI-Powered Analytics: Transforming Data into Insights
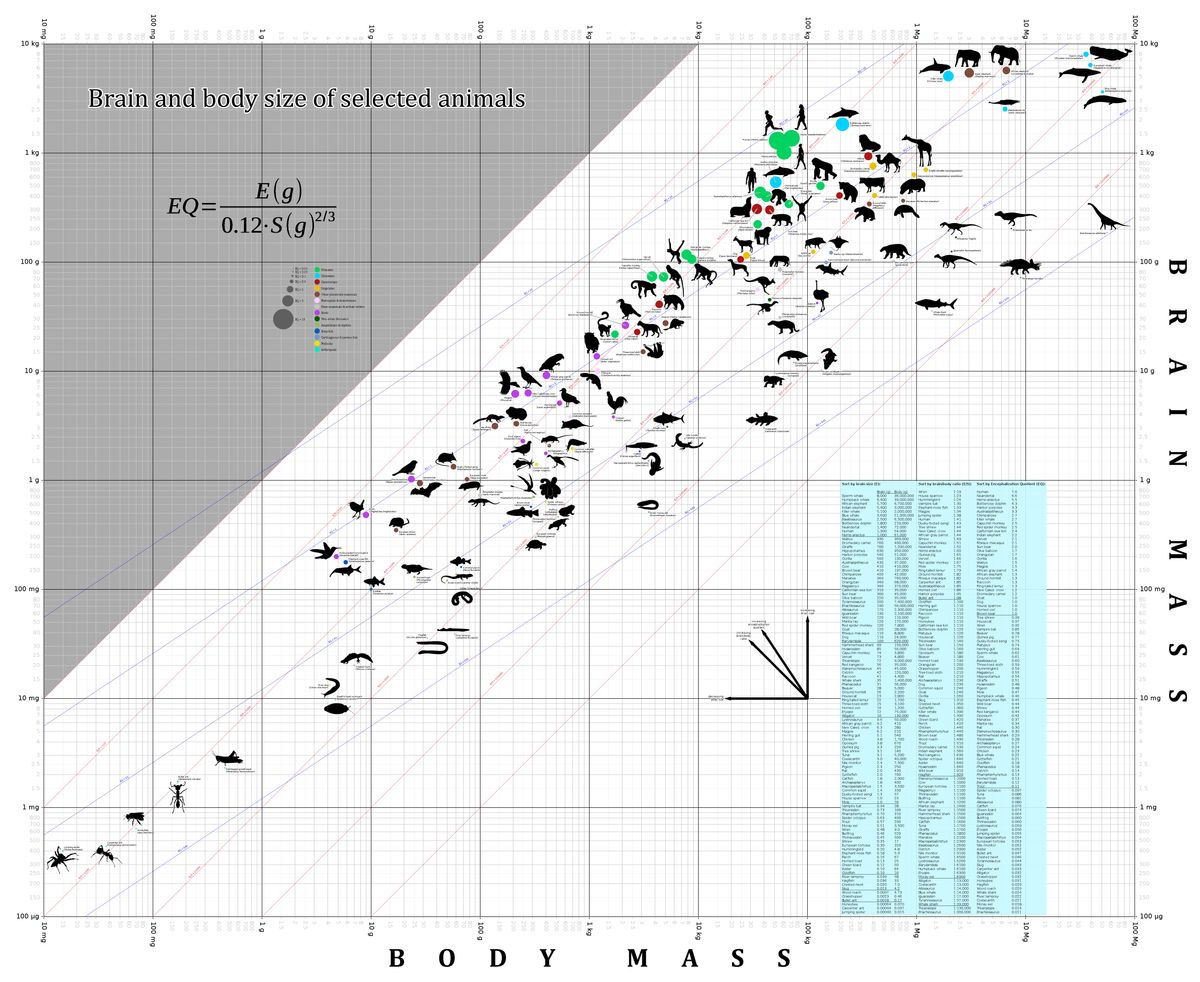
Empowering Business Agility
In an era where the pace of change is relentless, business agility has emerged as a fundamental element for success. AI-powered analytics serve as a catalyst, enabling organizations to respond swiftly to market shifts and emerging trends. By integrating analytics into their core strategy, businesses can foster a culture of agility that not only anticipates change but also embraces it as an opportunity for innovation and growth.
The agility of a business is often reflected in its capacity to harness data effectively. Those who can interpret and act on data insights quickly are better positioned to navigate the unpredictable waters of the global market.
The following points illustrate how AI-powered analytics empower business agility:
- Providing real-time data analysis for immediate action
- Identifying patterns that indicate potential market disruptions
- Enabling predictive decision-making to stay ahead of competitors
As the volume of data continues to expand, the role of AI in managing and interpreting this vast resource becomes increasingly crucial. Companies that leverage AI analytics effectively can maintain operational flexibility and informed decision-making, turning the burgeoning data mountain into a strategic advantage.
Driving Strategic Planning
In the realm of strategic planning, advanced analytics serve as a compass, guiding organizations through the complex landscape of business decisions. By leveraging data-driven insights, companies can forecast trends, optimize resource allocation, and ultimately, drive growth and innovation.
- Identify key objectives
- Assess current data governance maturity
- Develop an analytics roadmap
- Integrate analytics at all business levels
Advanced analytics and decision intelligence are not just about processing data; they are about transforming that data into a strategic asset that propels the business forward.
The implementation of business intelligence tools and best practices is a critical step in this journey. It ensures that the insights gleaned are not only accurate but also actionable, leading to data-driven revenue generation and enhanced decision-making.
Unlocking Insights
The journey from raw data to insights is pivotal for businesses aiming to thrive in a data-driven world. Transform data into actionable knowledge, and you unlock the hidden potential that can empower informed choices and innovation. Advanced analytics tools are the keys to this treasure trove, providing the clarity needed to cut through the noise of big data.
- Identify patterns and anomalies
- Understand customer behaviors
- Forecast market trends
- Optimize product offerings
By effectively unlocking insights, organizations can pivot from being data-rich to insight-driven, marking a significant leap towards achieving strategic goals and maintaining competitive advantage.
Self-Service Analytics for Empowered Decision-Making
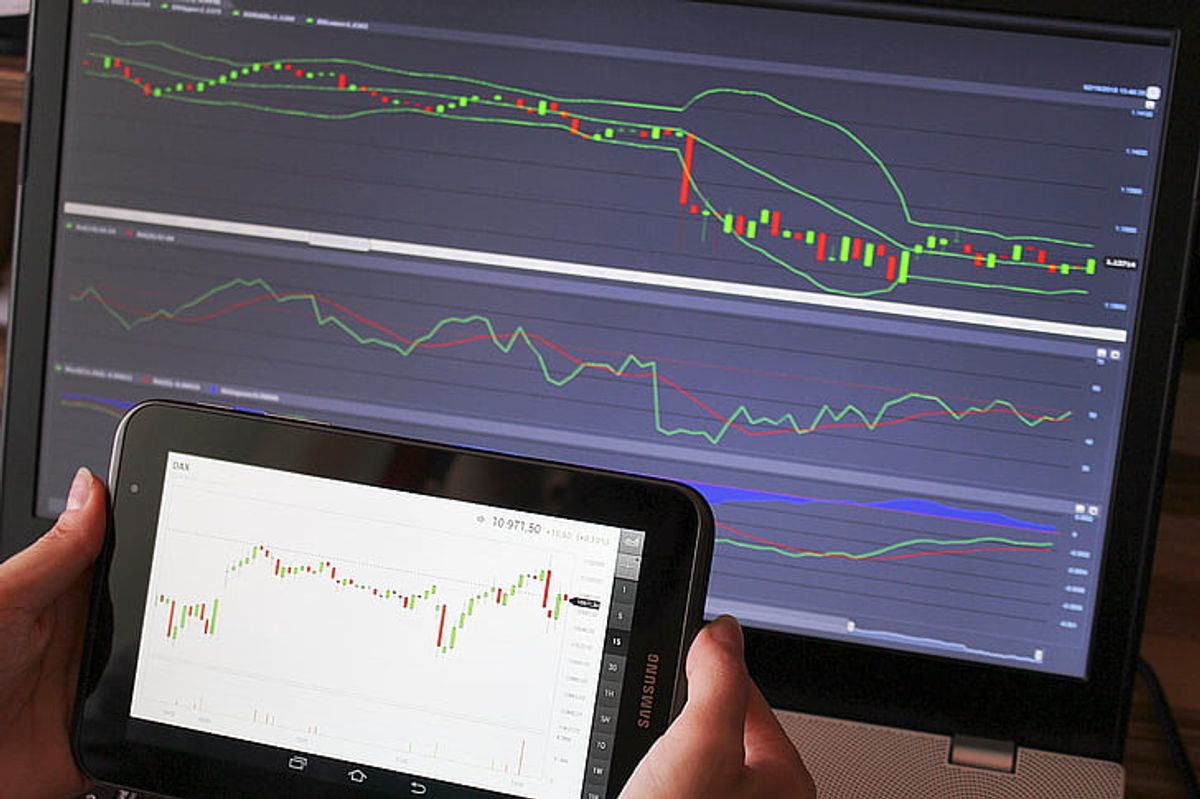
Algorithms and Machine Learning
In the realm of self-service analytics, algorithms and machine learning play a pivotal role in transforming raw data into insights. These technologies have evolved from merely descriptive to prescriptive analytics, marking a significant shift in business intelligence. The rise of self-service analytics platforms has democratized data analysis, allowing users to leverage sophisticated tools without deep technical expertise.
The accuracy of AI-powered insights hinges on the quality of data and the refinement of algorithms. High data quality and algorithmic precision are non-negotiable for reliable outcomes.
Here are some of the techniques that you can expect to use:
- Predictive modeling to forecast future outcomes using current and historical data.
- Data mining to identify trends, patterns, and anomalies within data sets.
- Continuous refinement of machine learning models to improve decision-making processes.
These techniques, when applied correctly, lead to a deeper understanding of business operations and customer behavior, ultimately enabling forecasting and optimization for data-driven decisions.
Data Mining and Visualization
Data mining and visualization are critical components in the journey from raw data to strategic insights. Data mining involves sifting through large datasets to uncover patterns and relationships that might otherwise remain hidden. It's a process that leverages algorithms, statistical analysis, and machine learning to transform data into valuable insights.
Data visualization complements data mining by translating these insights into a visual context. Complex information is presented clearly, fostering an environment where data-driven strategies can be easily understood and acted upon. Advanced visualization techniques ensure that insights are not only comprehended but also compelling to stakeholders, promoting informed and collaborative decision-making.
By integrating data mining with effective visualization, organizations can extract actionable insights and guide their strategic planning with confidence.
Here's a brief comparison of the outcomes from different analytical approaches:
Analysis Type | Focus | Outcome |
---|---|---|
Historical | Past data trends | Understanding operations |
Real-time | Current data streams | Immediate insights |
Predictive | Future trends | Forecasting decisions |
Predictive Modeling Techniques
At the core of predictive modeling is the ability to forecast future events by analyzing patterns in historical data. Predictive analytics harnesses statistical algorithms and machine learning techniques to project trends and outcomes. This process is not just about data crunching; it's about transforming data into a strategic asset that can guide decision-making and drive business success.
The effectiveness of predictive modeling is contingent upon the quality of the data and the precision of the models. Inaccurate models or subpar data can lead to erroneous forecasts, which can be costly for businesses. Therefore, it's crucial to ensure that data is clean, relevant, and robust.
Predictive modeling is a dynamic tool that empowers businesses to anticipate market demands, optimize marketing spend, and stay ahead of the competition. It's a form of advanced analytics that turns hindsight into foresight, enabling proactive rather than reactive strategies.
Here are some key techniques used in predictive modeling:
- Regression Analysis: Establishes relationships between variables to predict future outcomes.
- Data Mining: Uncovers trends and patterns from large datasets.
- Machine Learning: Employs algorithms to learn from data and make predictions.
By integrating these techniques, businesses can unlock the power of predictive modeling to not only foresee the future but also to shape it.
Conclusion
In conclusion, advanced analytics plays a crucial role in transforming data into actionable insights for businesses. By leveraging data analytics techniques, organizations can navigate the complexities of the digital age, make informed decisions, and drive strategic planning. The integration of AI-powered analytics is not just an option but a necessity for companies looking to thrive in a competitive market. Through data storytelling and self-service analytics, businesses can gain valuable insights, understand customer behavior, and optimize their operations. Overall, harnessing the power of data through advanced analytics solutions empowers organizations to problem-solve, predict trends, and enhance operational efficiency. The future of business success lies in the ability to harness the potential of data analytics to drive innovation and growth.
Frequently Asked Questions
What are the key data analytics techniques mentioned in the article?
The key data analytics techniques mentioned in the article include Data Extraction, Statistical Analysis, Predictive Modeling, and Data Visualization.
How does data analytics play a crucial role in decision-making?
Data analytics plays a crucial role in decision-making by leveraging data to guide businesses through uncertainty and providing valuable insights for strategic decision-making.
How can advanced analytics enhance operational efficiency?
Advanced analytics can enhance operational efficiency by optimizing business operations, improving process efficiency, and driving strategic decision-making.
What is the significance of decoding data analytics in business?
Decoding data analytics in business is significant as it empowers organizations to unravel patterns, predict trends, and make informed choices based on actionable insights.
What is the impact of AI-powered analytics on transforming data into insights?
AI-powered analytics have a transformative impact on turning data into actionable insights, empowering business agility, driving strategic planning, and unlocking valuable insights.
How does self-service analytics empower decision-making?
Self-service analytics empowers decision-making by utilizing algorithms, machine learning, data mining, visualization, and predictive modeling techniques to enable users to access and analyze data independently.
How can data storytelling contribute to a better understanding of market dynamics?
Data storytelling can contribute to a better understanding of market dynamics by illuminating complex market dynamics, customer behaviors, and campaign performance in an easily digestible format, facilitating the translation of insights into actionable strategies.
How can businesses benefit from business intelligence and analytics articles?
Businesses can benefit from business intelligence and analytics articles by gaining insights into advanced analytics solutions, problem-solving techniques, and the integration of AI-powered analytics into strategic frameworks for informed decision-making.