The Evolving Role of Database Administrators
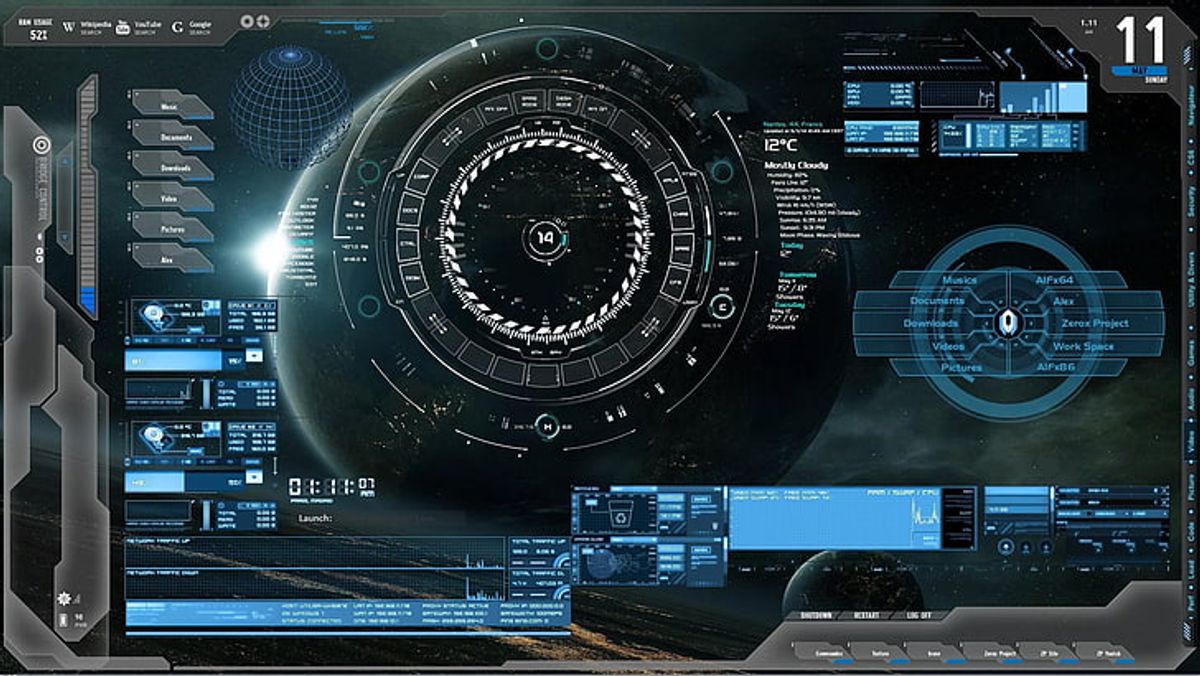
Adapting to Automation and AI
As we delve into the future of data management, the integration of machine learning and AI is becoming increasingly pivotal. Database administrators are now required to possess a nuanced understanding of AI's capabilities to maintain and enhance database systems effectively. This shift is not about replacing human expertise but augmenting it with intelligent automation, leading to more strategic roles in overseeing AI-driven processes.
- Understanding and implementing AI algorithms
- Structuring databases for machine learning workloads
- Collaborating with data scientists and machine learning engineers
The synergy between AI and human expertise is creating a new paradigm in database administration, where the focus is shifting from routine maintenance to strategic innovation.
The transformative impact of AI on database administration is evident in its ability to automate optimization processes, enhance data security, and streamline data integration. As we approach 2024, these advancements are not just optional; they are essential for businesses to remain competitive in a data-driven landscape.
Managing Diverse Database Environments
In the landscape of 2024 trends, the role of Database Administrators (DBAs) is being transformed by the rapid adoption of automation and AI technologies. As businesses increasingly migrate to the cloud, DBAs are required to develop new skills and strategies to manage a variety of databases across hybrid and multi-cloud environments.
- Embrace AI for a competitive edge in data management.
- Shift focus from routine tasks to strategic roles leveraging AI.
- Ensure data security during cloud migration.
The agility to adapt to diverse database environments is now a critical skill for DBAs, as it allows for the swift integration of new tools and the effective governance of data.
The traditional mix of database tools and management approaches is no longer sufficient. DBAs must now navigate a more complex landscape, where the ability to quickly leverage emerging opportunities can provide a significant competitive advantage.
Strategic Importance in Business Continuity
In the face of an ever-changing business landscape, database administrators (DBAs) are finding their roles increasingly intertwined with the strategic objectives of their organizations. The ability to maintain business continuity has become a paramount concern, with data platforms playing a critical role in ensuring operational resilience and relevance.
Data architectures are crucial for instant insights and are a cornerstone of the 2024 SQL trends, enabling businesses to adapt to market changes with agility. Future skills for DBAs will not only encompass advanced SQL techniques and data warehousing but also a broader spectrum of programming languages and essential soft skills.
The strategic integration of data platforms is not just about technology; it's about creating a cohesive and comprehensive solution that is seamlessly integrated with the entire organization.
As businesses strive to remain competitive, the need for robust infrastructure design becomes evident. DBAs are tasked with leveraging cloud technologies for enhanced scalability, disaster recovery, and global distribution, which are critical in supporting the ever-growing data needs. Waiting weeks or months to implement new tools can lead to missed opportunities and a loss of competitive advantage, making it necessary for businesses to leverage new tools and seize emerging opportunities swiftly and effectively.
Advancements in Real-Time Data Analytics
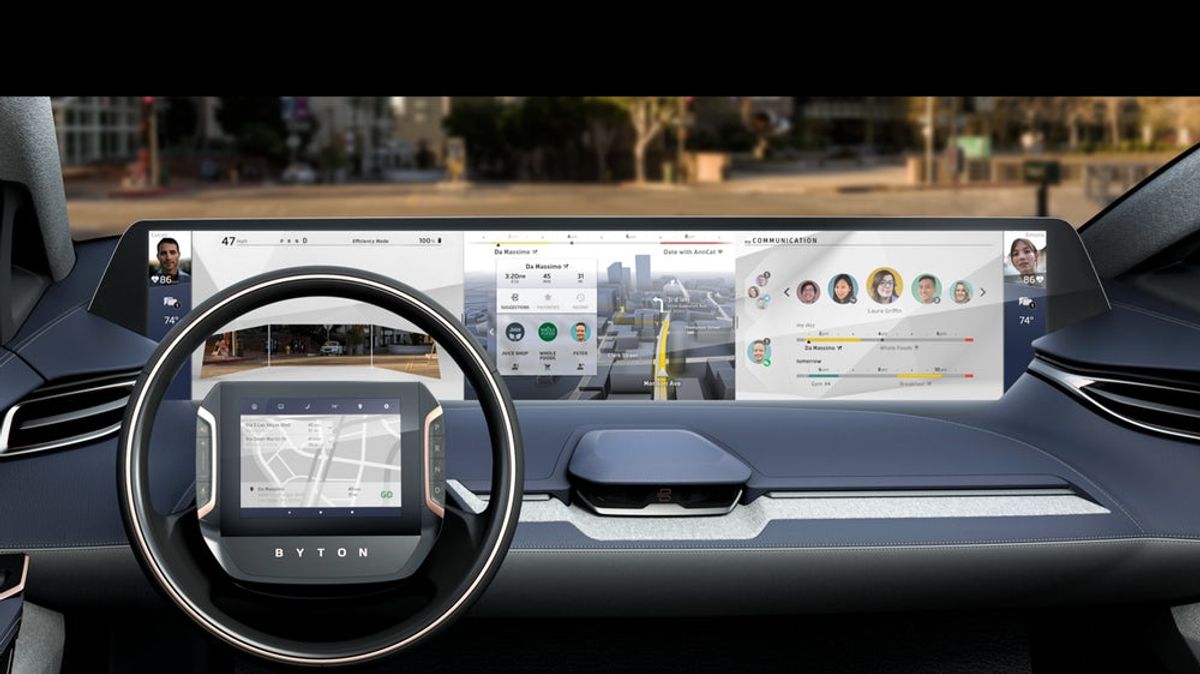
Cutting-Edge Data Architectures
In the dynamic landscape of 2024, cutting-edge data architectures are pivotal for businesses seeking to harness the power of their data. The integration of cloud computing and AI is not just a trend but a fundamental shift in how data ecosystems are structured and managed. Data Architects are now at the forefront, tasked with designing systems that are both scalable and agile to meet the ever-increasing demands of data processing and analytics.
- Master Advanced Data Modeling Techniques
- Deepen Knowledge in Cloud Services
- Integrate Machine Learning Models
These skills are essential for Data Architects to stay relevant in an environment where cloud storage and computing are ubiquitous. Proficiency in big data technologies, real-time analytics, and data streaming platforms is no longer optional. Moreover, understanding the ethical implications of data usage has become a critical component of the role, given the stringent regulations like GDPR and CCPA.
The rise of DataOps is closing the gap between data consumers and data producers, signaling a shift towards more collaborative and efficient data management practices.
Impact on Business Decision Making
The advent of real-time data analytics has revolutionized the way businesses make decisions. Speed is now the new currency in business, with the ability to react to market changes and consumer behavior in real-time being a significant competitive advantage.
Real-time analytics enables businesses to achieve faster time to insights, which is crucial in today's fast-paced market environment. By reducing the time from data collection to decision-making, companies can more swiftly seize emerging opportunities and maintain a competitive edge.
The strategic implementation of real-time data analytics is not just about speed; it's about the precision and relevance of the insights gained.
Here are some key benefits of real-time data analytics in business decision-making:
- Immediate identification of trends and patterns
- Quicker response to customer needs and market demands
- Enhanced operational efficiency and productivity
- Proactive risk management and mitigation
While the benefits are clear, the challenges in implementation should not be underestimated. Businesses must navigate the complexities of integrating these systems into their existing infrastructure and ensure that their teams are equipped to leverage the full potential of real-time data.
Challenges in Implementation
While the promise of real-time data analytics is alluring, the path to its successful implementation is fraught with challenges. Businesses must be agile, adapting quickly to new tools to capture emerging opportunities. Delays in leveraging these tools can result in missed chances and a weakened competitive stance.
Transitioning to advanced data platforms is a significant leap from traditional systems. It's a critical move for maintaining resilience and staying ahead in the business game. The complexity of this transition can be daunting, but with a well-defined educational roadmap, the learning curve can be managed effectively.
The role of Data Architects becomes crucial as they are tasked with translating business needs into technical solutions, ensuring a smooth transition and effective data strategy execution.
Challenges include:
- Navigating the complexity of new data architectures
- Bridging the gap between business and technical domains
- Ensuring timely adoption of innovative tools
- Developing a comprehensive educational plan for skill enhancement
Cloud Migration and Database Management
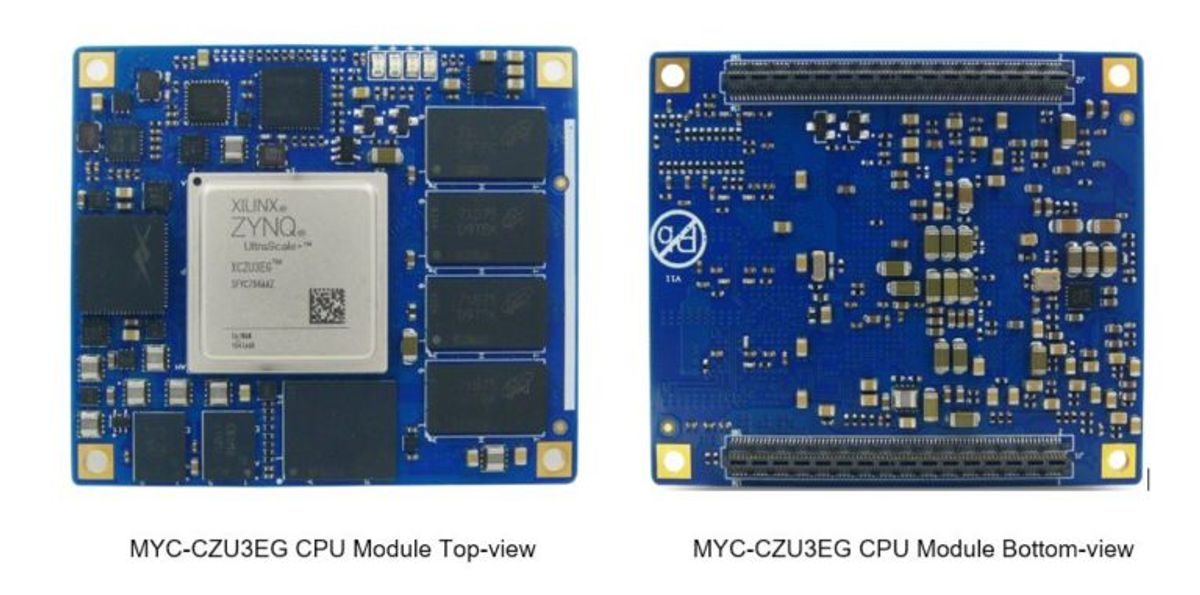
Trends in Database-as-a-Service (DBaaS)
The landscape of Database-as-a-Service (DBaaS) is rapidly evolving, with cloud migration becoming an imperative for modern database management. As businesses strive for agility and scalability, the strategic approach to DBaaS is crucial. The role of the modern DBA is transforming to accommodate these changes, with a significant growth in market dynamics for SQL Server and related technologies.
Cloud migration and automation are accelerating, offering companies an easy path to expand their database capabilities. This shift necessitates new database skills and strategies to effectively manage data across diverse environments. The following points highlight the key trends in DBaaS:
- Emphasis on security and compliance in cloud environments
- Integration of AI and automation to streamline database management
- Growth of hybrid and multi-cloud strategies to enhance flexibility
The number and variety of databases that need to be managed continue to grow, challenging DBAs to adapt to on-premise, cloud, and hybrid environments.
Understanding these trends is essential for organizations looking to leverage the full potential of DBaaS and maintain a competitive edge in the data-driven landscape of 2024.
Overcoming Storage and Scalability Challenges
As businesses migrate to the cloud, they encounter a myriad of storage and scalability challenges. Efficiently managing these challenges is crucial for maintaining system performance and ensuring business continuity. One key strategy involves leveraging elastic storage solutions that can dynamically adjust to fluctuating data demands.
- Implementing automated scaling policies
- Utilizing data compression and deduplication techniques
- Adopting multi-cloud strategies to enhance flexibility
By embracing these approaches, organizations can overcome the limitations of traditional storage systems and achieve a scalable cloud infrastructure.
It's also essential to consider the cost implications of storage solutions. Balancing performance with cost-effectiveness requires a nuanced understanding of the available options and their impact on the overall data management strategy.
Security Considerations in the Cloud
As organizations continue their cloud migration imperative, the role of Data Architects evolves to encompass a deep understanding of cloud services and security. In 2024, cloud computing expertise is not just desirable but essential, with a focus on architectures, storage solutions, and the nuances of cloud security.
- Understanding provider-specific features and services (AWS, Azure, Google Cloud Platform)
- Navigating hybrid cloud environments and cost-efficient infrastructure design
- Ensuring data privacy and compliance with evolving security standards
The ability to leverage cloud technologies for enhanced scalability, disaster recovery, and global distribution is critical in supporting the ever-growing data needs of modern businesses.
Data security and governance have become paramount as security threats evolve and data privacy regulations become more stringent. A solid foundation in data security practices is crucial for the design and maintenance of scalable and efficient databases. Data professionals must strategize for seamless cloud integration to stay ahead in the SQL 2024 landscape.
Emerging Job Roles in Business Analytics
Predicting Market Demand for Data Skills
The trajectory of the data science field is unmistakably upward, with LinkedIn's Emerging Jobs Report highlighting a surge from $37.9 billion in 2019 to an anticipated $230.80 billion by 2026. This growth signals a robust market for data skills, particularly in SQL and business analytics. Business analytics and SQL are experiencing significant growth, with industries keen on harnessing data for strategic decisions.
As the big data market is projected to double by 2027, the global demand for SQL professionals will likely mirror this expansion. Companies are increasingly seeking individuals who can translate complex data into actionable insights, making SQL proficiency a coveted skill.
- Understanding of data science fundamentals
- Proficiency in SQL and related technologies
- Ability to derive insights from big data
- Collaboration within data-driven environments
The importance of collaboration in the industry cannot be overstated, as it fosters innovation and enhances the value derived from data analytics.
Career Paths in Data Warehousing and Business Intelligence
The landscape of data warehousing and business intelligence (BI) is rapidly evolving, offering a plethora of career opportunities for those skilled in transforming raw data into actionable insights. Business Intelligence Managers and Data Warehouse Developers are at the forefront, devising strategic solutions that power informed business decisions.
- Business Intelligence Manager: Drives data-driven decisions, transforming raw data into actionable business insights.
- Data Warehouse Developer: Specializes in converting raw data into meaningful insights, fueling strategic business decisions.
- Database Analyst: Focuses on turning raw data into actionable insights, driving business decisions and growth.
- Analytics Engineer: Works on the technical side, ensuring that data is accurately transformed into valuable insights.
Embracing a career in data warehousing and BI requires a deep understanding of structured databases and the ability to craft complex SQL queries. Professionals must also be adept at using BI tools like Tableau, Power BI, or Looker to translate data into business insights. Continuous learning and networking are essential, as the field is dynamic with ever-changing technologies and methodologies.
The Rise of the Data Strategist
The emergence of the Data Strategist role is a testament to the evolving landscape of business analytics. Data Strategists are pivotal in transforming raw data into strategic assets, ensuring that organizations leverage their data for maximum competitive advantage. They are the architects of data-driven cultures, crafting policies and frameworks that empower teams to harness insights effectively.
- Understanding and interpreting complex data sets
- Developing strategic models for informed decision-making
- Integrating machine learning models into business strategies
- Ensuring data governance and compliance with regulations like GDPR and CCPA
Data Strategists not only focus on the technical aspects of data management but also on the strategic execution that aligns with long-term business objectives. Their role is crucial in anticipating future data needs and planning for sustainable growth.
In 2024, the demand for professionals who can navigate the intricate web of data analytics, cloud services, and real-time insights is surging. Data Strategists are at the forefront, equipped with the analytical thinking and project management skills necessary to lead organizations through the data revolution.
Innovations in Data Architecture
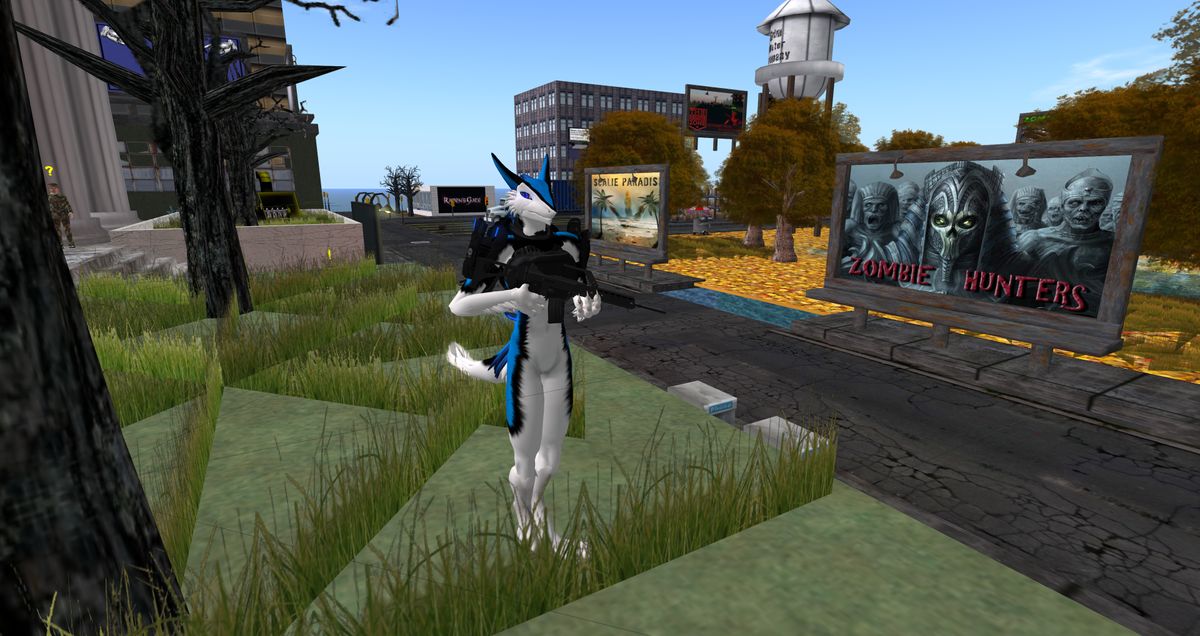
From Data Warehouses to Data Mesh
The transition from traditional data warehouses to a data mesh architecture marks a significant shift in how organizations manage and utilize their data. Data mesh promotes a decentralized approach, empowering domain-specific teams to own and operate their data as a product. This paradigm enhances agility and accelerates the delivery of data-driven insights.
- Decentralized data ownership
- Domain-oriented data control
- Self-serve data infrastructure as a product
The data mesh model is not just a technical architecture but a socio-technical approach that aligns with modern business needs for speed and adaptability.
As companies adopt data mesh, they face the challenge of integrating this model with existing systems. The key to success lies in a strategic implementation plan that includes:
- Identifying and empowering domain data owners
- Establishing a robust governance framework
- Building interoperable and scalable infrastructure
The shift to data mesh offers a promising avenue for businesses to enhance their data ecosystems, fostering innovation and competitive advantage.
Enabling Scalability and Flexibility
The unpredictable nature of data volumes and complexity underscores the importance of scalability in modern businesses. Traditional data analytics approaches often struggle to handle the massive influx of data, leading to performance bottlenecks, resource constraints, and diminished analytical capabilities.
Outsourcing emerges as a strategic solution, offering the flexibility to adapt and expand seamlessly in response to changing data requirements. Whether analyzing historical trends, processing real-time streams, or accommodating future growth, scalable data analytics platforms can effortlessly scale resources to meet evolving demands, ensuring the relevance and applicability of insights across diverse data scenarios.
The ability to scale teams up or down based on client needs is a significant competitive advantage of outsourcing. It's precious for businesses, such as startups, that require higher levels of adaptability in their operations.
The role of infrastructure design is pivotal in this context. Leveraging cloud technologies not only enhances scalability but also facilitates disaster recovery and global distribution, which are critical in supporting the ever-growing data needs of modern businesses.
Integrating AI and ML for Advanced Analytics
The integration of machine learning (ML) and artificial intelligence (AI) into SQL databases is not just a trend; it's a paradigm shift that is reshaping the landscape of data analytics. SQL 2024 trends include machine learning integration for advanced analytics in databases, emphasizing scalability and compliance with global regulations for responsible AI implementation. Data architects are now tasked with building data pipelines that cater to AI algorithms and structuring databases to efficiently handle ML workloads.
The ability to collaborate with data scientists and machine learning engineers is becoming crucial for creating systems that learn and adapt, offering predictive insights and a competitive edge.
To enable broader adoption of AI and ML analytics, companies are modernizing their data infrastructure. A modular data architecture is essential for accommodating new use cases swiftly. This modernization is not only a technical challenge but also a strategic initiative that involves mastering generative AI and leveraging large language models (LLMs) for a deeper understanding across various domains.
Data Engineering: The Backbone of Modern Analytics
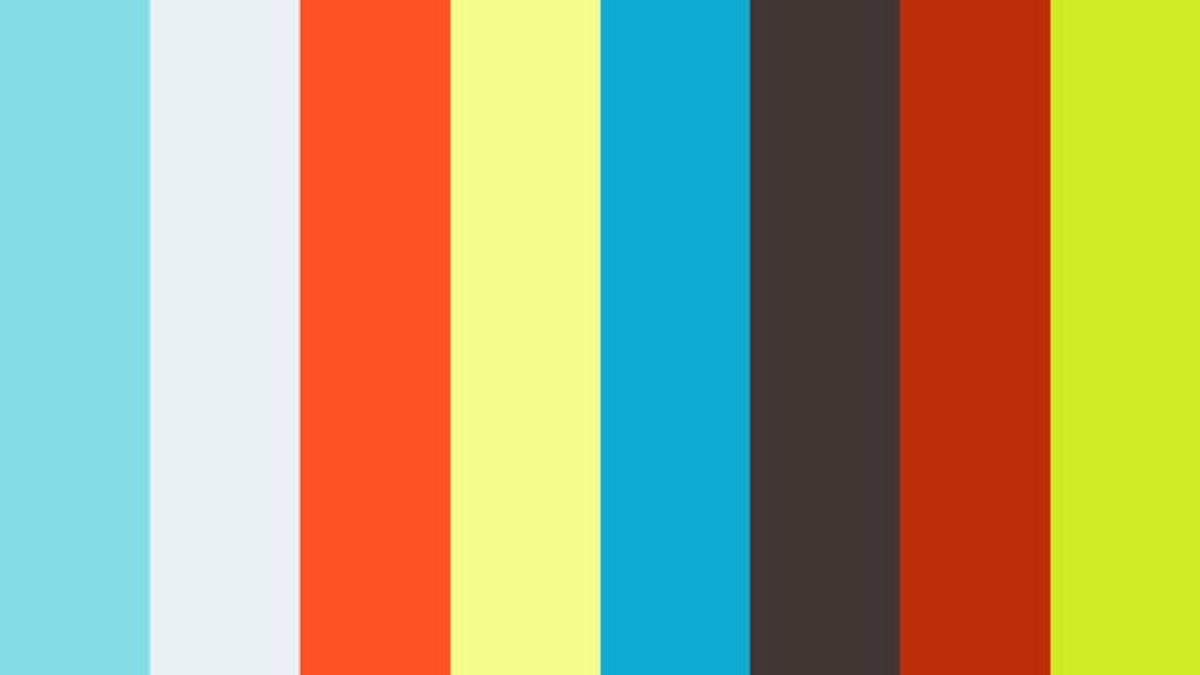
Trends Shaping Data Engineering in 2024
As we approach 2024, the landscape of data engineering is being transformed by several key trends. Augmented Analytics is leading the charge, enhancing traditional analytics with AI and machine learning to provide more sophisticated insights. The integration of Edge computing is another trend on the rise, bringing data processing closer to the source and reducing latency.
Real-time Analytics is becoming increasingly vital for businesses that require immediate insights for decision-making. Predictive Analytics 2.0, an evolution of existing models, is expected to offer even more accurate forecasts, leveraging advancements in AI and machine learning. These SQL trends in 2024 are not just technical upgrades; they represent SQL's critical role in strategic planning for businesses.
The importance of SQL in decision-making and strategic planning cannot be overstated. Its ability to handle complex queries and transactions makes it indispensable for businesses looking to leverage data for a competitive edge.
The following list highlights some of the emerging best practices in data engineering:
- Embracing cloud-native technologies for improved scalability and flexibility
- Implementing DataOps to streamline data workflows
- Prioritizing data quality and governance to ensure reliable analytics
- Adopting a multi-cloud strategy to avoid vendor lock-in and enhance disaster recovery plans
Best Practices for Data Pipeline Development
Developing robust data pipelines is essential for the efficient handling of data warehousing and big data solutions. Ensuring data quality at every stage is a cornerstone of this process. Here are some best practices:
- Have a clear understanding of the data to be collected.
- Utilize big data tools that align with the data type.
- Design pipelines with reusability in mind for various applications.
- Maintain thorough documentation for team clarity.
Embracing these practices not only optimizes the performance of the data pipeline but also facilitates the integration of emerging technologies such as cloud services and machine learning, which are vital for staying ahead in the field.
SQL developers are at the forefront, implementing advanced analytics that leverage these practices to deliver actionable insights. As the landscape evolves, the role of these professionals becomes increasingly strategic, navigating through the complexities of data management to drive business growth.
The Importance of Data Quality and Consistency
In the realm of data analytics, data quality and consistency are paramount. These factors directly influence the reliability of business insights and the effectiveness of data-driven decisions. To maintain high standards, organizations are increasingly turning to advanced technologies for data cleansing and validation.
Data governance plays a crucial role in this process, establishing the necessary policies and procedures to ensure uniformity across different data types. Without such governance, the risk of data discrepancies grows, potentially undermining strategic initiatives.
- Establishing clear data quality benchmarks
- Regularly auditing data for accuracy
- Implementing robust data validation processes
Ensuring data quality and consistency is not just a technical issue; it's a business imperative that affects every level of an organization.
As the analytics landscape evolves, the need for expert guidance becomes more apparent. Firms like OptimizDBA Team are stepping in to provide consultations on a variety of database systems, helping businesses navigate the complexities of data management and maintain the integrity of their data assets.
The Push Towards Unified Analytics Platforms
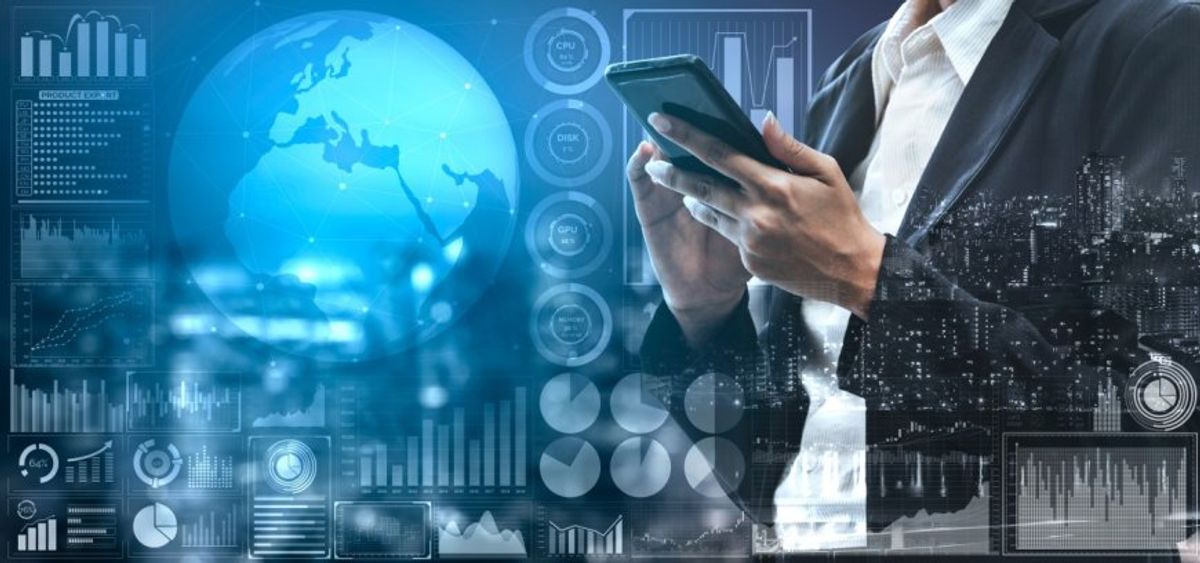
Overcoming Data Silos
The challenge of overcoming data silos is pivotal for businesses aiming to harness the full potential of their data assets. Silos, often a byproduct of legacy systems and departmental barriers, impede the free flow of information and stifle innovation. To dismantle these silos, organizations are turning to unified analytics platforms that offer a single source of truth for data across the enterprise.
By integrating disparate data sources into a cohesive analytics framework, companies can achieve a more holistic view of their operations and customer interactions.
A key strategy in this endeavor is the implementation of an agile data platform. Such platforms are designed to be scalable and secure, accommodating a variety of data sources and analytical tools. Below is a list of steps that can guide businesses in this transformation:
- Assess the current data landscape and identify existing silos.
- Define a clear data strategy that aligns with business objectives.
- Choose a flexible data platform that supports a wide range of data types and sources.
- Implement data governance policies to maintain data integrity and quality.
- Foster a culture of collaboration across departments to ensure data sharing and utilization.
The journey to a silo-free environment is not without its challenges, but the rewards of a unified data approach are substantial, leading to enhanced decision-making, improved efficiency, and a competitive edge in the market.
Next-Generation Data Platforms
The landscape of SQL in 2024 is rapidly evolving, with next-generation data platforms at the forefront of this transformation. These platforms are designed to be agile, accommodating a wide array of data types and models. From traditional SQL to advanced RDF and Geospatial models, they ensure that data is not only collected but also enriched and tailored to specific business needs.
- Integration: Seamlessly combining structured, semi-structured, and unstructured data.
- Enrichment: Applying metadata, ontologies, and taxonomies to enhance data utility.
- Delivery: Providing customized data streams for diverse business applications.
- Security: Maintaining robust security protocols to protect sensitive information.
The ideal data platform is a catalyst for innovation, enabling businesses to harness the full potential of their data assets. It is an essential tool for data-driven decision-making and business intelligence solutions.
As businesses strive to remain competitive, the adoption of these platforms is crucial. They offer a unified approach to data management, eliminating the constraints of disjointed systems and empowering organizations to swiftly adapt to market changes and consumer demands.
The Role of Unified Analytics in Business Strategy
In the landscape of modern business, Unified Analytics stands as a cornerstone for strategic decision-making. By integrating various data sources and analytics tools into a cohesive framework, businesses can gain a comprehensive view of their operations and market trends. This integration is not just about technology; it's about aligning with the right data strategy and ensuring that every decision is informed by accurate and timely insights.
- Alignment with Business Goals: Ensuring that analytics initiatives are directly tied to strategic objectives.
- Data Democratization: Making data accessible to non-technical users, fostering a culture of data-driven decision-making.
- Agility and Adaptability: Enabling quick responses to market changes and internal dynamics.
The Power of Unified Data: Transforming Business Insights, snippet: January 8, 2024.
The transition to unified analytics platforms is pivotal for organizations seeking to maintain a competitive edge. As the volume and variety of data continue to grow, the ability to streamline data processes and eliminate silos becomes increasingly important. Companies that embrace unified analytics are better positioned to harness the full potential of their data, driving innovation and growth.
Fostering AI and ML Analytics in Business
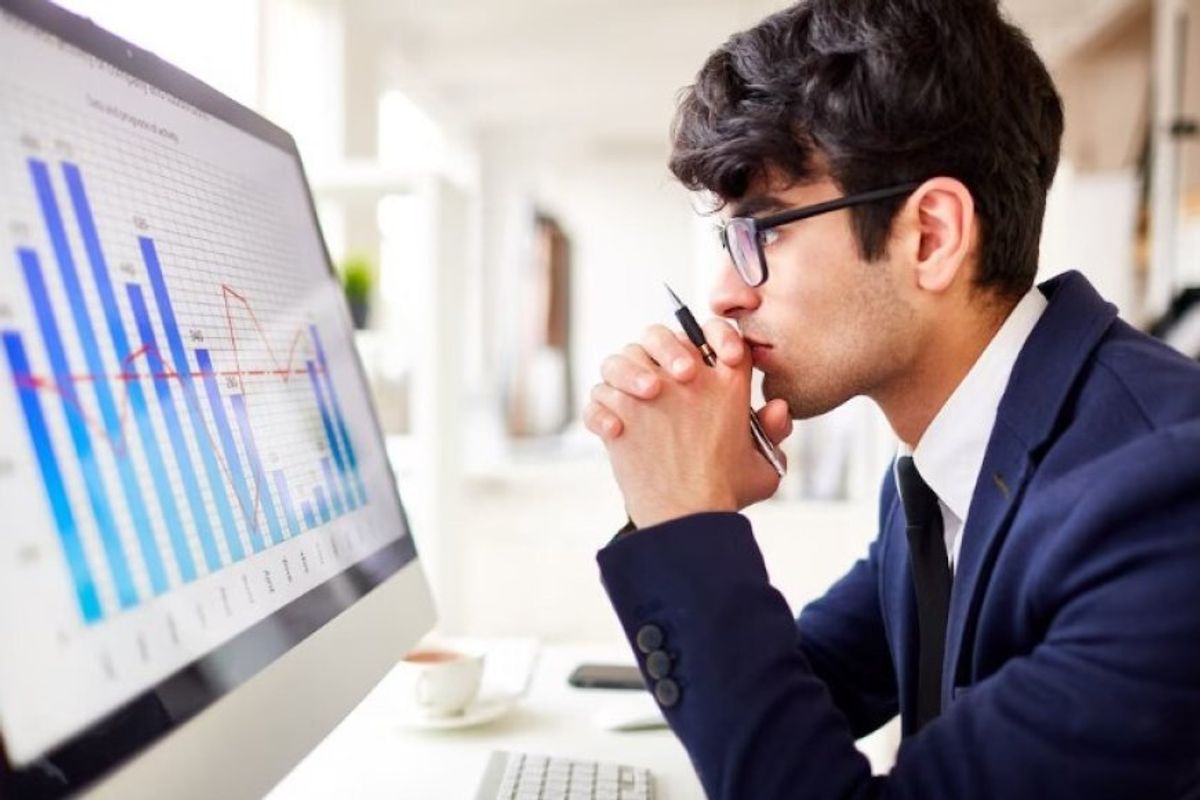
Data Platforms Enabling AI/ML
In the landscape of SQL in 2024, data platforms have become the cornerstone for enabling AI and ML analytics, transforming how businesses leverage data for competitive advantage. The ideal data platform is a versatile beast, capable of ingesting and processing various data forms to feed the ever-hungry AI algorithms. It's not just about volume; it's about the richness of data and the insights that can be extracted from it.
- Accommodates diverse data models
- Enriches data with metadata
- Ensures enterprise-grade security
An agile data platform is not a luxury but a necessity for organizations aiming to stay ahead in the AI era. It's the key enabler for businesses to quickly adapt to market changes and consumer demands. As the data estates grow in size and complexity, the role of these platforms in managing and analyzing data becomes increasingly critical. They are the unsung heroes, the backbone that supports the AI and ML analytics, driving business intelligence in sectors like healthcare, finance, and retail.
By adopting a singular, integrated data platform, businesses can unlock the true potential of their data, fueling growth and steering their course toward a prosperous future.
Cultivating a Data-Driven Culture
In the landscape of SQL 2024, the cultivation of a data-driven culture is paramount for businesses aiming to leverage data for growth. It's not just about having access to data, but about embedding the value of data into the very fabric of the organization. This requires a concerted effort across all levels, from executives to front-line employees, to prioritize data in decision-making processes.
To foster such a culture, businesses should consider the following steps:
- Encourage open access to data for all employees, fostering transparency and trust.
- Provide continuous training and development to enhance data literacy across the organization.
- Implement agile data platforms that can adapt to changing business needs and data types.
By embracing these practices, businesses can unlock the true potential of their data, fueling their growth and steering their course toward a prosperous and sustainable future.
The journey towards a data-centric mindset is not without its challenges, but the rewards are substantial. Companies that successfully cultivate a data-driven culture are better positioned to turn data into actionable insights, create value for customers, and navigate the complexities of the modern business environment.
Challenges in AI/ML Implementation
Implementing AI and ML in business systems presents a unique set of challenges that organizations must navigate to harness their full potential. Ensuring seamless integration with existing data architectures is critical, as AI and ML models require robust data pipelines for optimal performance. This integration is not just a technical endeavor but also a collaborative one, involving data architects, scientists, and machine learning engineers.
Collaboration is key in overcoming these challenges, as it allows for the pooling of expertise necessary to build systems that can adapt and learn over time. However, the complexity of these systems often leads to difficulties in management and scalability. Below is a list of common challenges faced in AI/ML implementation:
- Aligning AI/ML initiatives with business objectives
- Acquiring and retaining skilled personnel
- Ensuring data quality and consistency
- Managing computational resources effectively
- Adapting to continuous technological advancements
The strategic importance of AI/ML in providing competitive advantages through predictive analytics cannot be overstated. Yet, the journey to successful implementation is fraught with obstacles that require a clear and informed approach.
Modernizing Data Integration and Governance
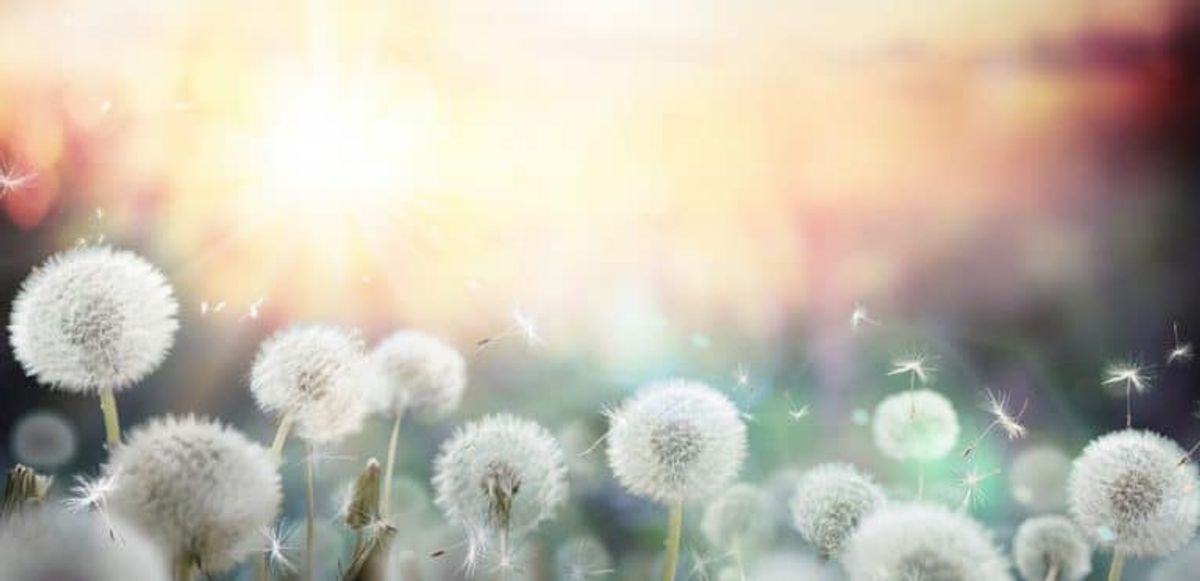
Adapting to Hybrid and Multi-Cloud Environments
As we move into 2024, the landscape of database management is rapidly evolving to accommodate the growing demand for hybrid and multi-cloud environments. Organizations are seeking to leverage the best of both worlds: the scalability and cost-efficiency of the cloud, alongside the control and security of on-premises solutions. This shift necessitates a new breed of Data Architects who are adept at navigating the complexities of cloud services from providers like AWS, Azure, and Google Cloud Platform.
The key to success in this new era is the ability to integrate and manage data across disparate systems, ensuring that it remains protected, reliable, and readily accessible.
To achieve this, companies are adopting modern data integration and governance strategies. Below is a list of emerging best practices:
- Embracing automation to streamline data workflows
- Implementing robust data governance frameworks
- Prioritizing cloud security and compliance measures
- Designing cost-efficient infrastructure for scalability
- Utilizing advanced tools for seamless data migration and synchronization
The Role of Data Governance in Compliance
In the landscape of 2024, data governance is not just a regulatory requirement but a strategic asset. As organizations navigate through a maze of regulations like GDPR and CCPA, the role of Data Architects becomes crucial in designing frameworks that ensure data quality, security, and privacy. These frameworks are the bedrock of trust with customers and stakeholders, highlighting responsible data stewardship.
Data governance and compliance expertise are essential for maintaining not only legal and ethical standards but also for adapting to the evolving landscape of data regulations. Ethical considerations around data usage have become critical, positioning Data Architects at the vanguard of a data-centric business environment.
The implementation of data governance is a multifaceted endeavor. It involves the establishment of policies and processes that are designed to deliver data downstream, tailored to the specific needs of the business, while upholding enterprise-grade security and governance.
To achieve consistency across various data types, organizations must design a set of standard policies and processes. This ensures that operations such as cleaning, securing, and transforming data are performed uniformly, regardless of the data's nature.
Best Practices for Data Integration
In the realm of data integration, best practices are essential for ensuring efficient and reliable data flow across systems. Documentation is key; it not only aids in maintaining the integrity of the data pipeline but also facilitates understanding among team members.
Simultaneous data flow should be preferred over sequential execution to optimize performance. Ensuring data quality at various stages is crucial, as is designing reusable pipelines that can serve multiple applications. Below is a list of best practices:
- Have a clear understanding of the data to be collected.
- Identify the most suitable big data tools for the data type.
- Design the data pipeline layout thoughtfully.
- Ensure all necessary tools are installed and configured.
- Prepare the infrastructure for robust data handling.
- Test and refine the pipeline iteratively for improvements.
Embracing these practices will not only streamline the data integration process but also bolster the overall data strategy, leading to more informed decision-making and enhanced business agility.
In the digital era, the ability to streamline data integration and enhance governance is crucial for any organization aiming to maintain a competitive edge. At OptimizDBA, we pride ourselves on delivering faster data solutions and unparalleled database optimization consulting. Don't let outdated systems slow you down. Visit our website to discover how our proprietary methodologies can revolutionize your data management and drive your business forward. Let's embark on this journey together and unlock the full potential of your data infrastructure.
Conclusion
As we navigate through the transformative landscape of SQL and data management in 2024, it's clear that the future is both promising and complex. The shift towards cloud-based database management, the proliferation of SQL Server across diverse platforms, and the heightened focus on data quality and governance underscore the dynamic nature of the industry. The evolving role of the DBA, the surge in real-time analytics, and the demand for advanced data architectures reflect a market that is rapidly adapting to the needs of a data-driven economy. With the anticipation of new job roles in business analytics and the integration of AI and ML into data platforms, professionals and organizations alike must stay agile and informed to harness the full potential of these trends. The insights and discussions from industry experts throughout 2023 and early 2024 provide a roadmap for navigating these changes, ensuring that we can meet the challenges and seize the growth opportunities that lie ahead in the SQL and data realm.
Frequently Asked Questions
How is the role of the Database Administrator (DBA) changing with the advent of AI and automation?
The role of DBAs is evolving to focus more on strategic tasks such as managing diverse database environments, ensuring data security, and contributing to business continuity plans, while routine maintenance is increasingly automated.
What are the key trends in real-time data analytics for 2024?
Real-time data analytics in 2024 is characterized by cutting-edge data architectures, a significant impact on business decision-making, and the challenges of implementing these systems effectively across organizations.
What does the shift towards Database-as-a-Service (DBaaS) mean for businesses?
DBaaS offers businesses scalability, flexibility, and cost-efficiency by outsourcing database management to cloud providers. It simplifies the management of database storage and helps overcome scalability challenges.
Which business analytics job roles are expected to be in high demand in 2024?
In 2024, roles such as data strategists, data warehousing specialists, and business intelligence analysts are in high demand, reflecting the growing strategic importance of data in decision-making.
How is data architecture innovating to support modern business needs?
Data architecture is moving towards models like data mesh, which provide greater scalability and flexibility, and integrate AI and ML for advanced analytics, catering to the dynamic needs of modern businesses.
What are the emerging best practices for data pipeline development in 2024?
Best practices include prioritizing data quality and consistency, leveraging modern data engineering trends, and employing methodologies that ensure efficient and reliable data pipelines.
How are unified analytics platforms transforming business strategy?
Unified analytics platforms are helping businesses overcome data silos, providing next-generation data management and analytics capabilities, and playing a crucial role in formulating data-driven business strategies.
What are the challenges companies face in implementing AI/ML analytics?
Challenges include integrating suitable data platforms, fostering a data-driven culture within the organization, and navigating the technical complexities of AI/ML implementation.