Understanding the Foundations of Business Intelligence
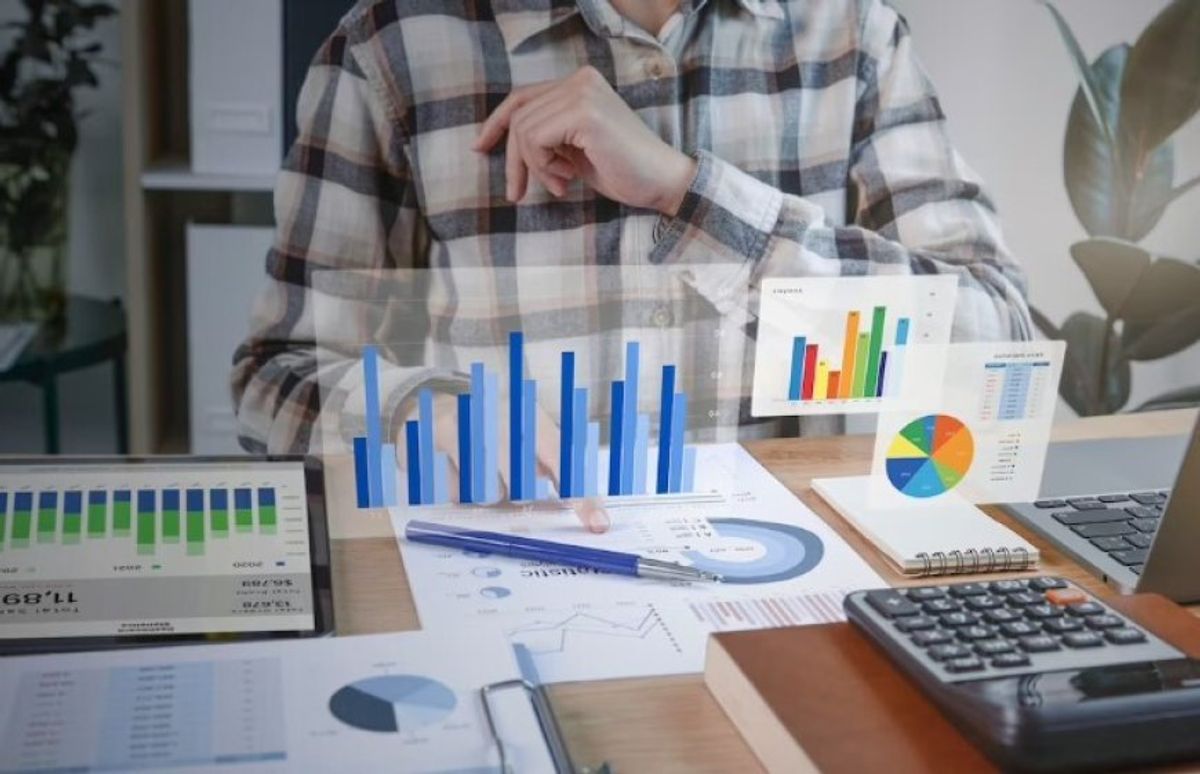
Defining Business Intelligence and Analytics
At the core of modern business strategy lies the concept of Business Intelligence (BI), a term that encompasses the tools, systems, and practices used to collect, integrate, analyze, and present an organization's raw data to create actionable insights. The goal of BI is to support better business decision-making, essentially turning data into a competitive advantage.
Analytics plays a crucial role in this process, providing the methodologies and technologies that transform data into meaningful patterns and metrics. These insights can then inform strategic decisions, ranging from operational improvements to new market opportunities.
The distinctions between being data-driven, data-enabled, and data-powered aren't rigid barriers but rather complementary facets of a holistic business strategy.
Understanding the different ways a company can utilize data is essential:
- Data-Driven: Decisions across all levels are informed by data.
- Data-Enabled: Operational processes are optimized through data insights and automation.
- Data-Powered: Products or services directly integrate data, analytics, or AI, enhancing the customer experience.
The decision to become data-powered depends on various factors such as industry demands, customer needs, and market positioning. Not every company will need to fully integrate data and AI into their products, but all should consider the benefits of being data-driven and data-enabled to remain competitive.
The Role of Data in Strategic Decision-Making
In the realm of strategic decision-making, data is the cornerstone that supports informed choices and drives business success. Business Intelligence (BI) tools enable organizations to transform data into actionable insights, leading to informed decision-making and competitive advantage. Data modeling plays a crucial role in uncovering opportunities through abstract representations of complex data structures.
Adopting a data-driven approach enhances strategic decision-making, embedding data-centric solutions into the core of everyday business activities. This transition emphasizes operational excellence through the application of data, analytics, machine learning (ML), and artificial intelligence (AI).
Transitioning to a data-driven approach is demanding, yet the subtle, indirect rewards it brings are substantial and transformative. By fostering a data-driven organizational culture, you unlock the potential to move more swiftly, efficiently, and strategically.
Understanding these nuances is vital for defining a precise vision and realizing the substantial benefits of informed decision-making. It guides the company's direction, avoiding the pitfalls of chasing trends and ensuring that decisions across all levels are informed by data.
Building a Data-Driven Organizational Culture
Cultivating a data-driven culture within an organization is pivotal for ensuring that every decision is anchored in solid, data-based evidence. Building trust in data is fundamental, and this trust is fostered through ensuring data accuracy, timeliness, and transparency. By enhancing data management and governance practices, organizations lay the groundwork for a culture that values and utilizes data effectively.
Transitioning to a data-driven approach is demanding, yet the rewards it brings are substantial and transformative. A data-driven culture enables organizations to move more swiftly, efficiently, and strategically.
To establish a data-driven culture, it is crucial to integrate data-driven solutions into everyday business operations. This requires not only the right mindset but also the necessary infrastructure that allows team members to align their efforts with strategic objectives. Intuitive dashboards and clear communication of data insights are essential tools that facilitate this integration.
Finally, it is important to recognize that the journey towards a data-driven culture begins with the empowerment of teams. Embedding a data-centric philosophy across all levels of the organization is a directive that leaders must champion. Amidst the escalating hype surrounding AI, the strategic acumen derived from dependable data and clearly articulated KPIs is invaluable.
Developing a Robust Data Strategy
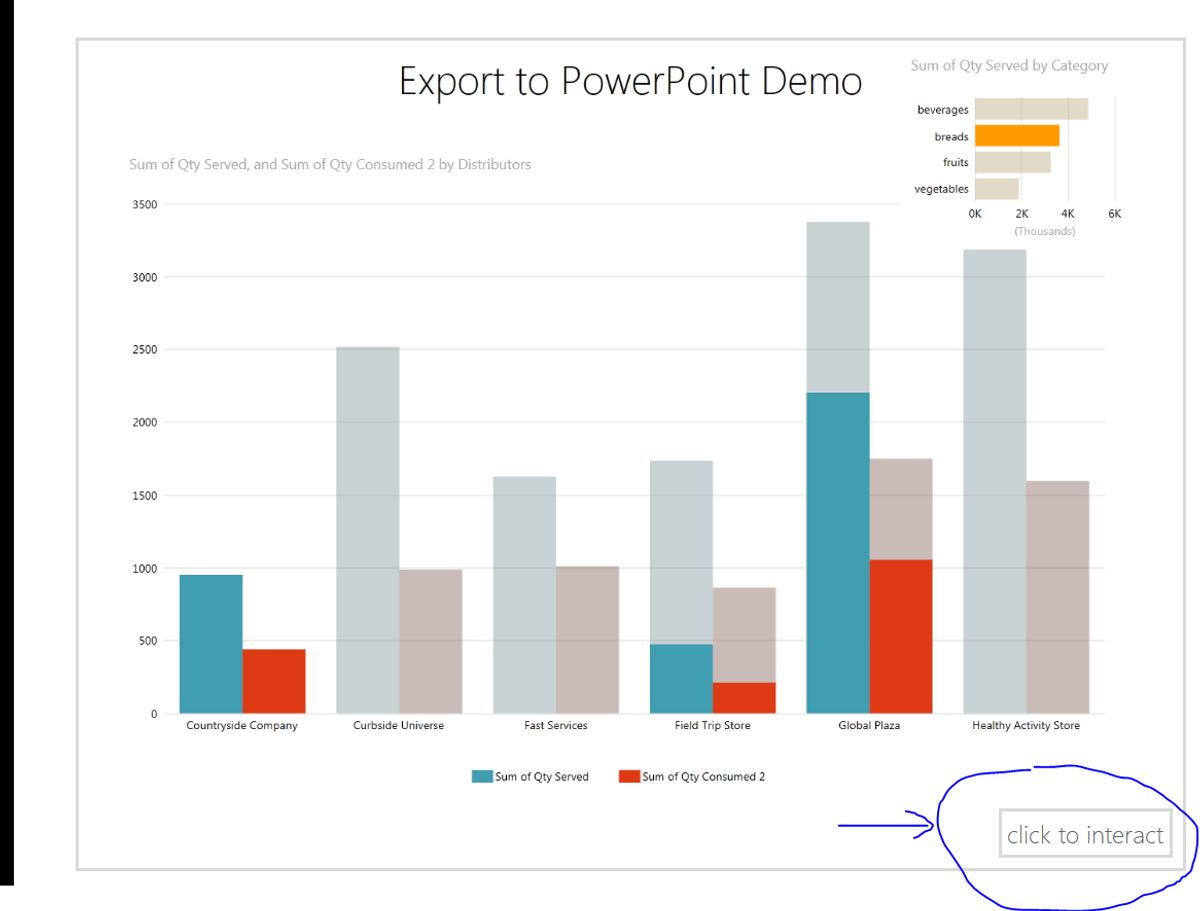
Creating a Data & AI Maturity Model
In the dynamic landscape of business intelligence, the creation of a Data & AI Maturity Model serves as a critical roadmap for organizations aiming to harness the full potential of their data assets. Developing a maturity model is essential for businesses to assess their current capabilities, identify gaps, and chart a strategic path towards advanced analytics and AI integration.
To construct a comprehensive maturity model, consider the following key dimensions:
- Data Management: How effectively is data collected, stored, and managed?
- Analytics Proficiency: What level of analytical complexity can your team handle?
- AI Integration: To what extent are AI capabilities embedded in business processes?
- Culture and Leadership: Is there a top-down commitment to data-driven decision making?
By evaluating these dimensions, organizations can pinpoint their position on the maturity scale and set clear objectives for progression. This self-assessment guides the allocation of resources and prioritization of initiatives that will drive the most value.
As businesses in 2024 focus on data quality, AI integration in BI tools, and fostering a data-driven culture, the maturity model becomes an indispensable tool. It not only benchmarks current performance but also illuminates the pathway to staying competitive and leveraging BI innovations.
Establishing a Comprehensive Data Policy
A comprehensive data policy is the cornerstone of a data-driven organization. It sets the stage for how data is managed, ensuring accuracy, integrity, and safety. By establishing clear rules and procedures, a data policy fosters a culture of trust in data, which is essential for informed decision-making.
A robust data policy not only protects the organization but also empowers employees by providing clear guidelines on data usage and handling.
To effectively implement a data policy, consider the following steps:
- Define the scope of data governance within your organization.
- Identify the roles and responsibilities for data management.
- Develop a framework for data access, quality, and privacy.
- Establish protocols for data storage, retention, and deletion.
- Create a process for ongoing policy review and updates.
Understanding the nuances of data management and governance will enable your organization to avoid the pitfalls of chasing trends and realize the substantial benefits of informed decision-making. Cultivating a culture of data-driven management is not just about technology; it's about embedding a data-centric philosophy across all levels of the organization.
Aligning Data Strategy with Business Goals
To ensure that a data strategy is not just a technical endeavor but a core part of the business strategy, it is crucial to identify relevant business drivers. These drivers, whether big or small, should be the foundation upon which the data strategy is built. By doing so, the data initiatives are more likely to produce outcomes that are in direct alignment with the company's objectives.
Alignment with business goals means that every aspect of the data strategy, from collection to analysis, is designed to support and enhance the strategic direction of the organization. This requires a clear understanding of the business's needs and a commitment to tailoring data solutions to meet those needs.
- Establish a clear link between data initiatives and business objectives
- Ensure that data governance aligns with the company's strategic goals
- Develop metrics and KPIs that reflect the success of data strategy in achieving business outcomes
By embedding a data-centric philosophy across all levels of the organization, companies can harness the transformative power of data to move more swiftly, efficiently, and strategically.
The journey towards a data-aligned business strategy is both challenging and rewarding. It demands a shift in mindset and the development of new capabilities, but the payoff is a more dynamic, informed, and competitive organization.
Implementing Data-Driven Solutions
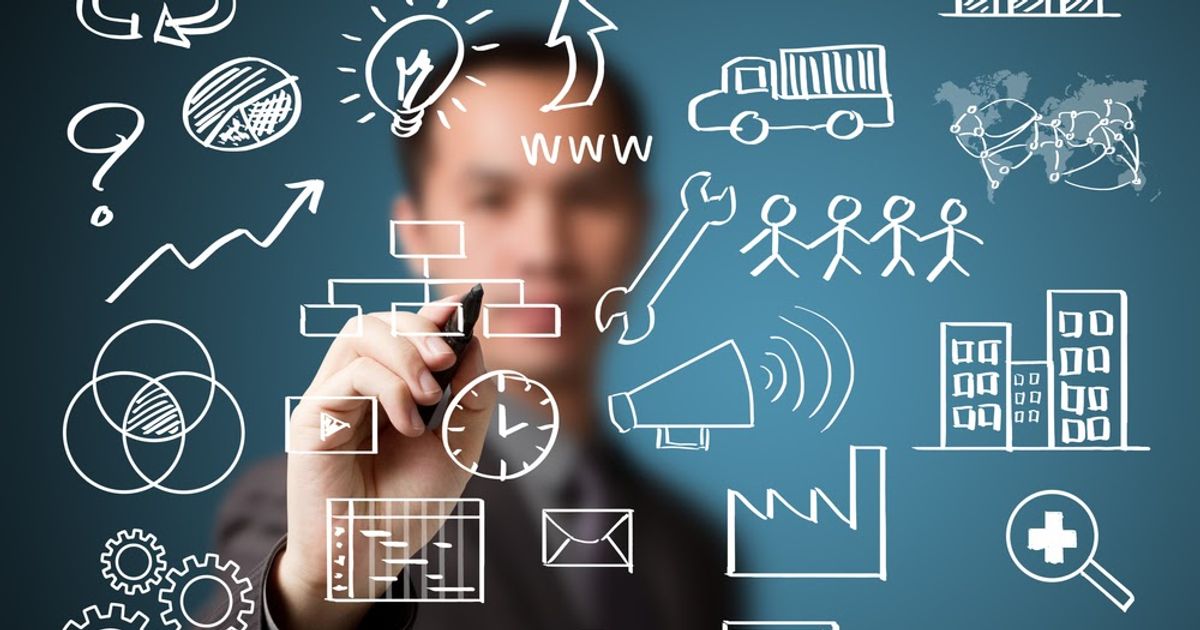
Integrating AI and Machine Learning in Operations
The integration of artificial intelligence (AI) and machine learning (ML) into business operations marks a transformative step towards operational excellence. Deploying AI and ML can lead to efficiency improvements in the range of 10-40%, offering a significant competitive edge. These technologies enable businesses to refine strategies, optimize processes, and innovate products.
The seamless integration of AI and ML into business operations is not just about adopting new technologies; it's about embedding data-centric solutions into the core of everyday activities, enhancing efficiency and productivity.
For instance, a consumer goods company might use advanced analytics to adjust its pricing strategy, while an energy firm could apply machine learning for end-to-end optimization. The impact of these integrations spans across various departments, from marketing, where generative AI models create innovative content, to legal, where AI assists in drafting standardized documents.
While the market offers a plethora of tools and ML/AI solutions, integrating them requires careful planning and sometimes the development of custom solutions. However, the strategic value derived from a data-driven approach far outweighs the initial effort. It's crucial to move beyond the AI hype and focus on leveraging data for a strategic edge.
Optimizing Processes with Data Analytics
The integration of data analytics into business operations is a transformative force, driving efficiency and innovation. Automation and data-driven strategies are essential for business success, enabling companies to refine their processes and gain a competitive edge. By implementing clear data governance, investing in technology, training teams, and leveraging analytics, organizations can tap into their maximum potential and foster innovation.
Skillfully implemented, data-enhanced solutions can give your offerings a distinct advantage over the competition, or even deliver groundbreaking value to your customers.
For instance, consider the impact of data analytics on supply chain management:
- Predictive analytics can forecast demand and optimize inventory levels.
- Real-time tracking ensures transparency and responsiveness in logistics.
- Machine learning algorithms can identify patterns and suggest improvements.
By adopting a data-driven approach, strategic decision-making is enhanced, embedding data-centric solutions into the core of everyday business activities. This transition emphasizes operational excellence and impacts areas from supply chain optimization to the strengthening of financial reports. As a result, the organization becomes more dynamic and well-equipped to navigate the complexities of the modern business landscape.
Ensuring Data Quality and Accuracy
In the realm of business intelligence, data quality is paramount. It is a measure of a data set's condition based on factors such as accuracy, completeness, consistency, reliability, and validity. High-quality data is the bedrock upon which reliable analytics and strategic decisions are made.
Establishing a culture that prioritizes data quality is not just about technology; it's about shaping the mindset and processes that drive strategic objectives. Trust in data is built on its accuracy, timeliness, and transparency.
To ensure data quality and accuracy, consider the following steps:
- Define clear data quality metrics and standards.
- Implement robust data governance and management practices.
- Regularly audit and cleanse data to maintain integrity.
- Provide training and resources to empower teams in maintaining data quality.
Understanding the nuances of data quality enables a precise vision, steering clear of trends and towards the substantial benefits of informed decision-making.
Enhancing Products with Data-Powered Features
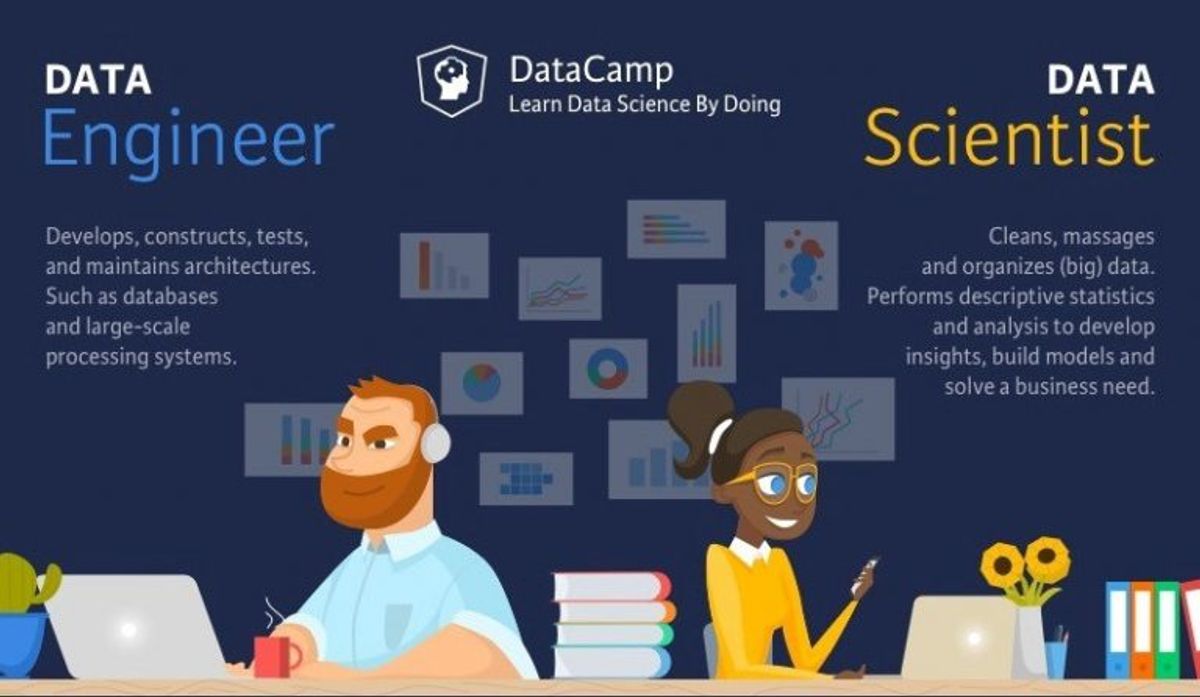
Innovating with Data-Enabled Offerings
Entering the realm of data-powered businesses means that your offerings incorporate data, analytics, or artificial intelligence in a way that is integral to the customer experience. Skillfully implemented, data-enhanced solutions can give your offerings a distinct advantage over the competition, or even deliver groundbreaking value to your customers.
The push towards adopting AI-powered products should be motivated by addressing unmet customer needs or enhancing product desirability, not just the allure of the technologies themselves. It's crucial to balance innovation with practicality to ensure that data-enabled features are not only impressive but also genuinely useful and reliable.
By focusing on the strategic integration of data and AI, companies can create offerings that not only meet but anticipate customer needs, fostering a strong competitive edge.
Here are some key considerations when innovating with data-enabled offerings:
- Understand the customer's pain points and how data can address them.
- Ensure the data-powered features are central to the product's value proposition.
- Regularly update and maintain the data infrastructure to support these offerings.
Meeting Customer Needs through Data Insights
In the realm of customer satisfaction, data insights serve as a compass guiding product development and service enhancement. By analyzing customer behavior and feedback, businesses can tailor their offerings to meet the evolving demands of the market. Personalization is key; it transforms generic interactions into meaningful experiences that resonate with individual preferences.
The strategic use of data insights not only satisfies existing customer needs but also anticipates future trends, setting the stage for innovation and sustained growth.
Understanding customer needs through data goes beyond mere analytics; it involves a deep dive into the customer journey, identifying pain points and opportunities for improvement. Here's a concise list of steps to ensure customer-centric data utilization:
- Collect diverse customer data points
- Analyze for patterns and preferences
- Implement changes based on insights
- Monitor feedback and iterate
This iterative process ensures that products and services evolve in a way that is both responsive to customer feedback and proactive in offering solutions that customers value. By leveraging data insights effectively, companies can create a competitive edge that is difficult to replicate.
Balancing Innovation with Practicality
In the quest to integrate cutting-edge technologies into business offerings, it's crucial to maintain a balance between the allure of innovation and the practicality of real-world applications. The drive for innovation should not overshadow the fundamental goal of becoming data-driven and data-enabled. Companies must ensure that their pursuit of AI-powered products is rooted in genuine customer needs and the enhancement of product desirability, rather than being swayed by the mere appeal of new technologies.
Skillfully implemented, data-enhanced solutions can provide a distinct competitive advantage or groundbreaking value to customers. However, this requires a deep understanding of the technology to deliver on the promised benefits reliably. The table below illustrates the balance between innovation and practicality in data-powered product features:
Feature Type | Innovation Level | Practicality | Customer Impact |
---|---|---|---|
Basic | Low | High | Essential |
Enhanced | Medium | Medium | Significant |
Cutting-edge | High | Low | Experimental |
Understanding these nuances will enable companies to avoid the pitfalls of chasing trends and realize the substantial benefits of informed decision-making.
Cultivating a culture of data-driven management and developing data-powered products opens new avenues for business innovation and customer engagement. It's essential to define a precise vision and align product development with strategic business goals to ensure that innovation remains practical and beneficial to the end-user.
Navigating the AI Hype in Business Operations
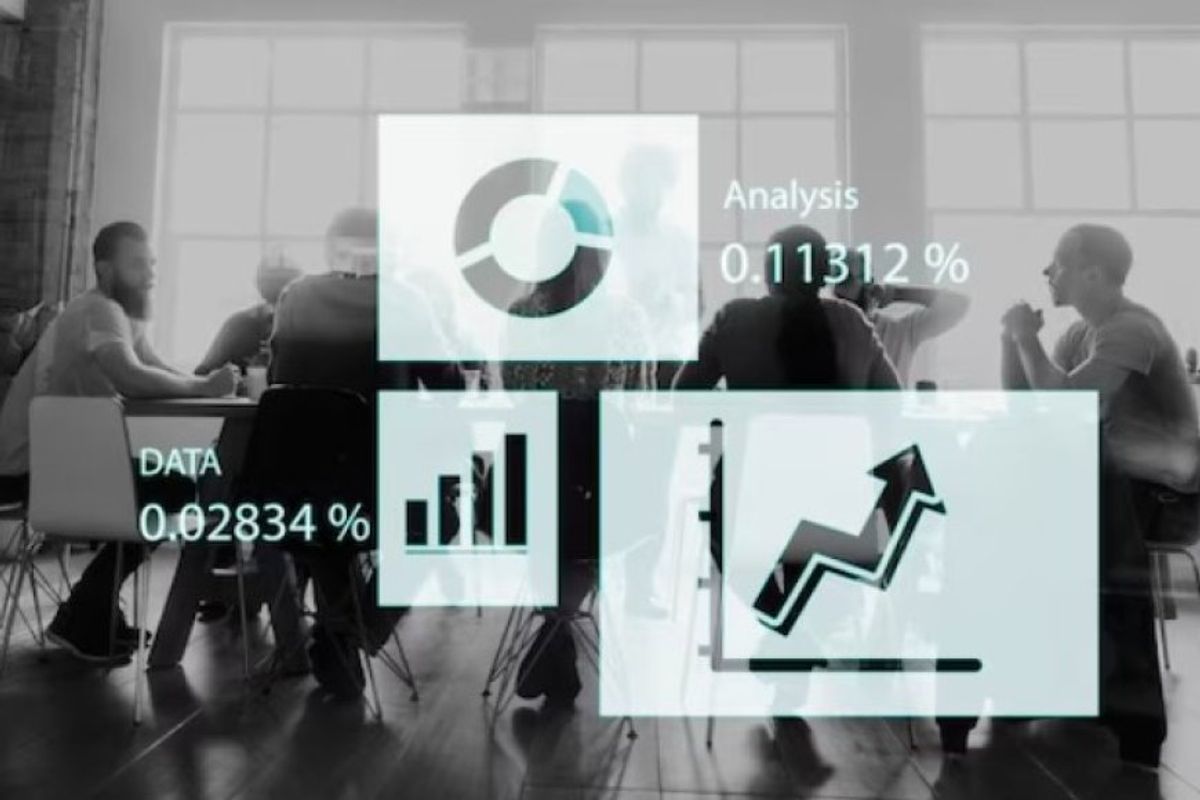
Separating AI Myths from Business Realities
In the landscape of modern business, AI myths can cloud judgment and lead to misaligned strategies. It's crucial to distinguish between the hype and the actual capabilities of AI. For instance, the myth that AI is universally understood and implemented is far from the truth. In reality, the journey to AI maturity varies widely among organizations.
Myth vs. Reality
- Myth: AI can solve all problems instantly.
- Reality: AI requires careful planning and alignment with business objectives.
Embracing a data-driven approach is more than just adopting new technologies; it's about integrating data into the core of business decision-making.
The push to integrate AI should be motivated by genuine business needs, not just the novelty of the technology. By focusing on practical applications and measurable outcomes, businesses can effectively leverage AI to gain a strategic edge.
Focusing on Value-Driven AI Implementations
In the landscape of modern business, the allure of Artificial Intelligence (AI) can often eclipse the core objective of enhancing value through data. Companies must prioritize AI implementations that drive tangible business outcomes, rather than succumbing to the hype of technology for technology's sake.
The drive for innovation should be firmly rooted in addressing unmet customer needs and improving operational efficiency. AI should not just be about the technology, but about the value it brings to the business.
To ensure that AI initiatives are value-driven, businesses can follow a structured approach:
- Identifying key areas of impact where AI can provide significant benefits
- Aligning AI projects with strategic business goals
- Measuring success through well-defined KPIs and ROI analysis
By focusing on these areas, organizations can move beyond the superficial appeal of AI and leverage it as a powerful tool for strategic advantage.
Avoiding Common Pitfalls in AI Adoption
In the pursuit of integrating AI into business operations, it's crucial to navigate the landscape with a clear understanding of the technology's practical applications. The excitement around AI often leads to its premature or inappropriate use, where the focus shifts from solving real problems to simply deploying the latest tech. To avoid these common pitfalls, consider the following points:
- Ensure that AI solutions align with specific business needs and objectives.
- Evaluate the readiness of your organization's data infrastructure.
- Prioritize the enhancement of your team's data literacy and AI skills.
- Maintain a balance between innovation and the proven value of AI applications.
By emphasizing a data-driven approach over the allure of AI, businesses can foster a culture that values meaningful innovation and strategic growth.
Adopting AI should not be an end in itself but a means to become more data-driven and effective in meeting customer demands. It's essential to remember that the core of a successful AI strategy lies in its ability to improve decision-making and operational efficiency, not just in the technology's sophistication.
Building a Data-Driven Workforce
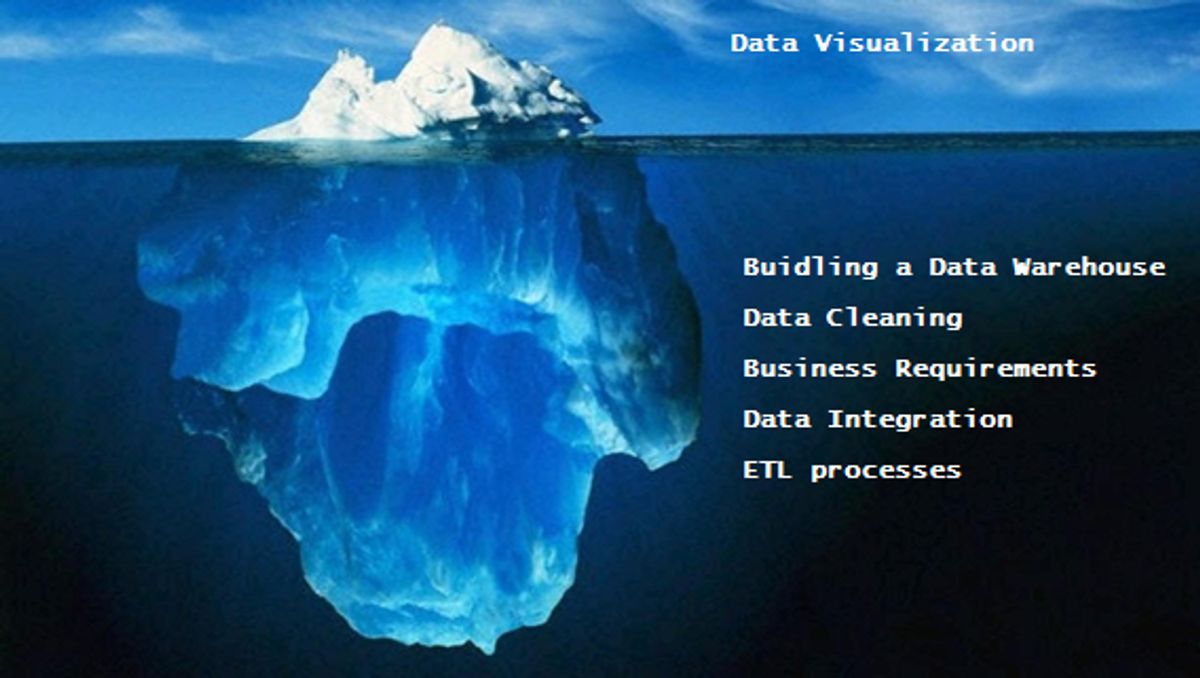
Investing in Data Training and Education
In the journey towards becoming a data-driven organization, investing in data training and education is a pivotal step. It's not just about having access to data or the latest tools; it's about ensuring that every member of the team understands the value of data and is equipped with the necessary skills to interpret and leverage it effectively.
- Educating employees on the importance of data literacy.
- Providing training on the latest data analytics tools and techniques.
- Fostering an environment where data-driven insights are valued and sought after.
By investing in the professional development of employees, businesses can cultivate a workforce that is not only proficient in data analysis but also adept at using data to drive strategic decisions and innovation.
The benefits of such an investment are manifold, leading to enhanced decision-making, improved operational efficiency, and a more agile response to market changes. Companies that prioritize data education are better positioned to harness the power of prescriptive analytics and AI-driven analytics, which are essential for optimizing outcomes and staying ahead in today's competitive landscape.
Recruiting and Retaining Data Science Talent
In the competitive landscape of data science, attracting and nurturing talent is paramount for maintaining a cutting-edge business intelligence team. Companies must not only focus on recruiting skilled individuals but also on creating an environment that encourages their growth and retention.
Compensation is a critical factor in this equation. As highlighted in the Rocket Recruiting Blog, it's important to offer competitive compensation and benefits packages to secure top talent. These packages often include perks such as remote work options and flexible schedules, which are increasingly valued by data professionals.
To ensure a steady pipeline of qualified candidates, businesses should consider the following strategies:
- Establish partnerships with educational institutions to identify emerging talent.
- Create internship and mentorship programs to foster early professional development.
- Implement continuous learning and development opportunities to keep skills sharp.
By investing in the professional development of data scientists, companies not only enhance their capabilities but also signal a commitment to their workforce, which can significantly improve employee loyalty and job satisfaction.
Fostering Collaboration Across Data Teams
In the realm of business intelligence, the synergy between multidisciplinary teams is pivotal. Domain experts, analysts, scientists, and engineers must work in unison to distill raw data into actionable insights. A robust data infrastructure and a cohesive operating model are the bedrock of this seamless collaboration.
Establishing a data-driven management infrastructure is not just about technology; it's about cultivating both the mindset and the capabilities for teams to efficiently align their efforts with strategic goals. Trust in data is cultivated through its accuracy, timeliness, and transparency, which are underpinned by sound data management and governance practices.
The empowerment of teams and the embedding of a data-centric philosophy throughout the organization are crucial. It is the first step towards a data-driven identity that can evolve to meet customer demands with data-powered solutions. To this end, intuitive dashboards and tools that provide a unified view of data are indispensable for fostering collaboration and ensuring that insights are drawn from diverse sources.
Leveraging Advanced Analytics for Competitive Advantage
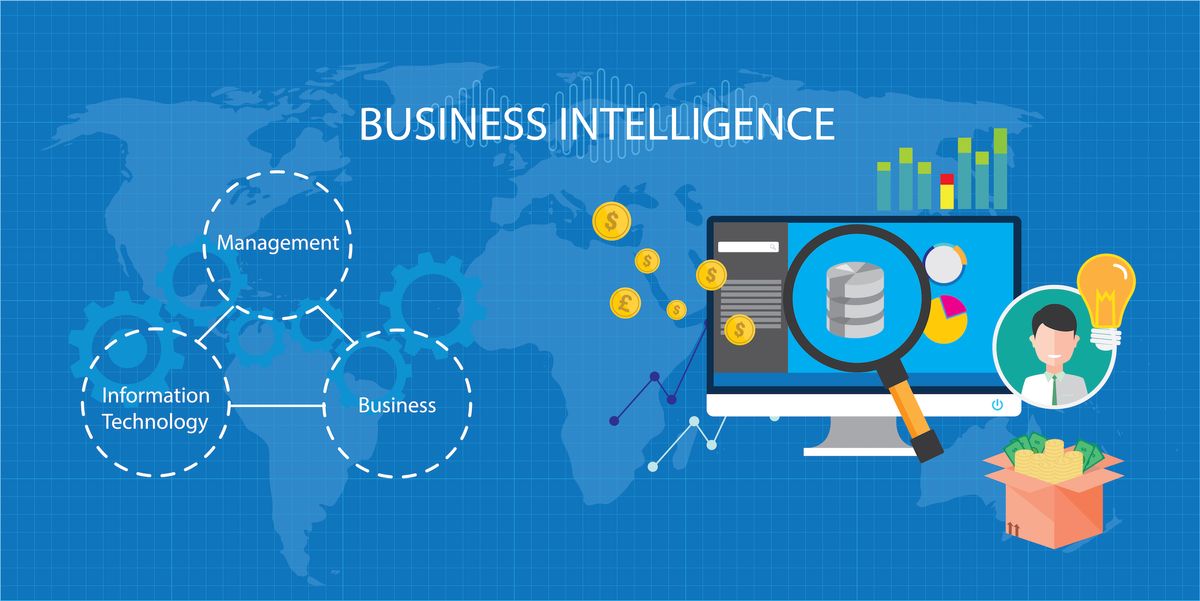
Utilizing Predictive and Prescriptive Analytics
The integration of predictive and prescriptive analytics is pivotal for businesses aiming to anticipate market trends and optimize decision-making. Predictive analytics harness historical data to forecast future events, while prescriptive analytics suggests possible actions to achieve desired outcomes.
Predictive analytics can be particularly transformative in areas such as demand forecasting, risk assessment, and customer behavior analysis. Prescriptive analytics, on the other hand, builds upon these predictions to recommend strategic actions that align with business objectives.
By effectively combining both predictive and prescriptive analytics, organizations can not only foresee potential challenges but also devise proactive strategies to address them.
Here is a simplified example of how these analytics can be applied:
- Predictive: A retail company analyzes past sales data to predict future demand for products.
- Prescriptive: The same company uses these predictions to adjust inventory levels, plan promotions, and set pricing strategies to maximize profits.
Understanding and implementing these analytics requires a clear data strategy and the right technological infrastructure. It is essential for businesses to invest in the necessary tools and talent to leverage these advanced analytics for a competitive edge.
Gaining Insights from Big Data
In the era of big data, businesses are inundated with volumes of information that hold the key to strategic insights. Transforming raw data into actionable intelligence is a pivotal step in leveraging data for a competitive edge. A multi-layered approach is essential, involving the integration of technology, processes, and people.
- Effective collaboration across multidisciplinary teams is crucial.
- A robust data infrastructure supports seamless teamwork.
- A cohesive operating model ensures the efficient transformation of data.
Transitioning to a data-driven approach is demanding, yet the rewards are transformative, enabling swifter, more strategic decision-making.
Understanding the nuances of big data analytics is vital for defining a precise vision for your company. It allows you to avoid the pitfalls of chasing trends and to harness the substantial benefits of informed decision-making.
Benchmarking Performance with Analytics
Benchmarking with analytics is a critical step in understanding your company's position in the market. By collecting and analyzing data, you can gain insights into how your business measures up against competitors. It's essential to determine what specific metrics you want to compare, such as sales growth, customer satisfaction, or operational efficiency, to ensure meaningful comparisons.
Benchmarking is not just about numbers; it's about translating data into actionable insights that can drive strategic decisions.
To effectively benchmark performance, consider the following steps:
- Identify key performance indicators (KPIs) relevant to your business goals.
- Gather data from internal systems and external sources.
- Analyze the data to identify trends and areas for improvement.
- Compare your performance against industry standards or direct competitors.
- Develop a plan to address any gaps and capitalize on strengths.
Creating a Scalable Data Architecture
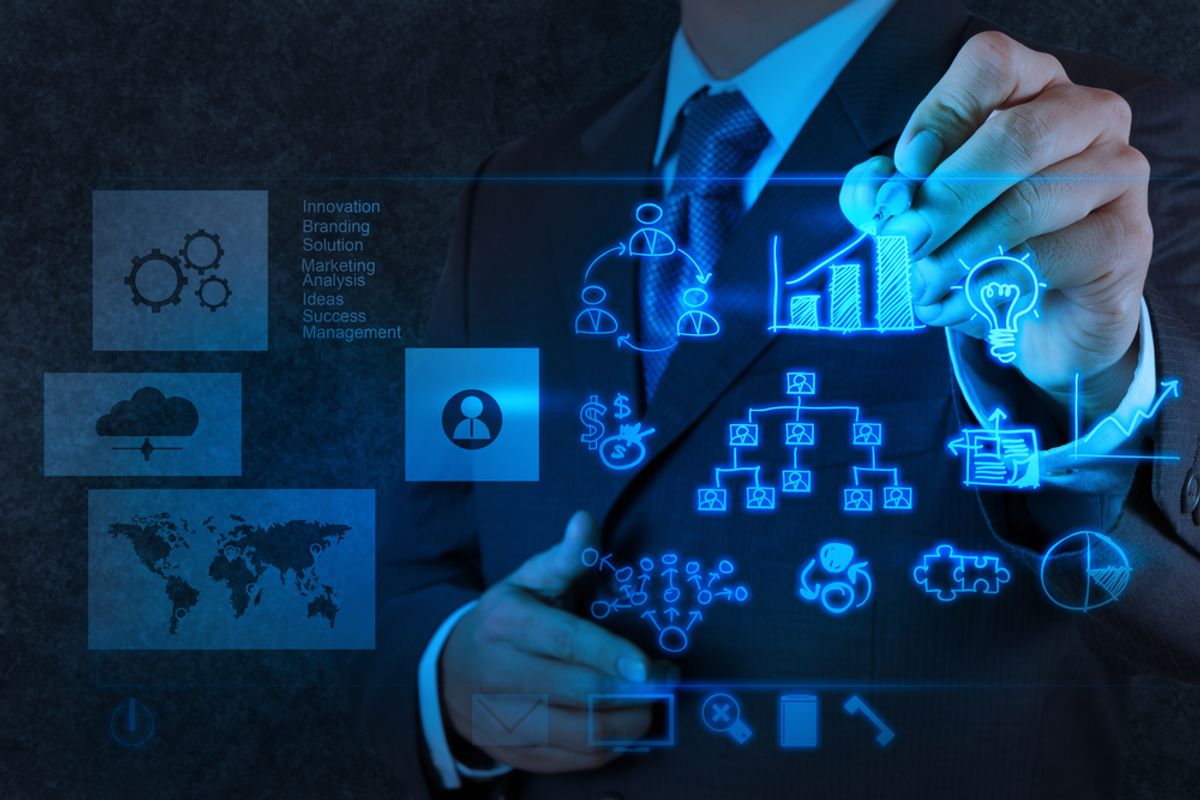
Designing a Future-Proof Data Infrastructure
In the rapidly evolving landscape of data technology, designing a future-proof data infrastructure is critical for maintaining a competitive edge. A robust infrastructure not only supports current analytical needs but also adapts to emerging technologies and scales with business growth.
Key components of a future-proof data infrastructure include:
- Scalability to handle increasing data volumes
- Flexibility to integrate new data sources and technologies
- Reliability to ensure continuous operation and data availability
- Security to protect against data breaches and ensure compliance
Scalability and flexibility are particularly important, as they allow an organization to pivot and adapt to market changes without the need for complete overhauls of the data system.
Establishing both the mindset and the infrastructure for data-driven management is essential. This includes fostering technical and process-oriented capabilities that allow team members to guide their efforts towards strategic objectives efficiently.
Transitioning to a data-driven approach is demanding, yet the subtle, indirect rewards it brings are substantial and transformative. By fostering a data-driven organizational culture, you unlock the potential to move more swiftly, efficiently, and strategically, gaining a competitive edge through smarter management and informed decisions.
Managing Data Across the Enterprise
In the quest to enhance business intelligence architecture, a comprehensive approach to managing data across the enterprise is crucial. It involves integrating data from various sources, ensuring that the analytics systems are aligned with business objectives, and leveraging AI to make smarter decisions.
- Establish a centralized data repository to ensure consistency and accessibility.
- Implement data governance standards to maintain data quality and compliance.
- Utilize advanced analytics tools to derive actionable insights from enterprise data.
By centralizing data management, organizations can streamline operations, reduce redundancies, and foster a culture of informed decision-making.
Adopting a holistic data management strategy not only supports operational excellence but also provides a competitive edge by enabling more strategic and informed decisions throughout the organization.
Ensuring Security and Compliance in Data Architecture
In the realm of data architecture, security and compliance are not mere checkboxes but the bedrock of trust and reliability. Establishing a robust framework for data protection involves a multi-layered approach, addressing both technical safeguards and regulatory adherence.
Governance practices are pivotal in this context, ensuring that data management aligns with both internal policies and external regulations. This is where the concept of data empowerment comes into play, integrating optimization and AI to foster digital innovation and growth.
- Conduct regular security audits
- Implement access controls and encryption
- Maintain compliance with evolving regulations
- Foster a culture of continuous improvement
By embedding a data-centric philosophy across all levels, organizations can navigate the complexities of data security with confidence.
Understanding these nuances is crucial for defining a precise vision that steers clear of trend-chasing and focuses on the substantial benefits of informed decision-making.
Driving Innovation with Generative AI
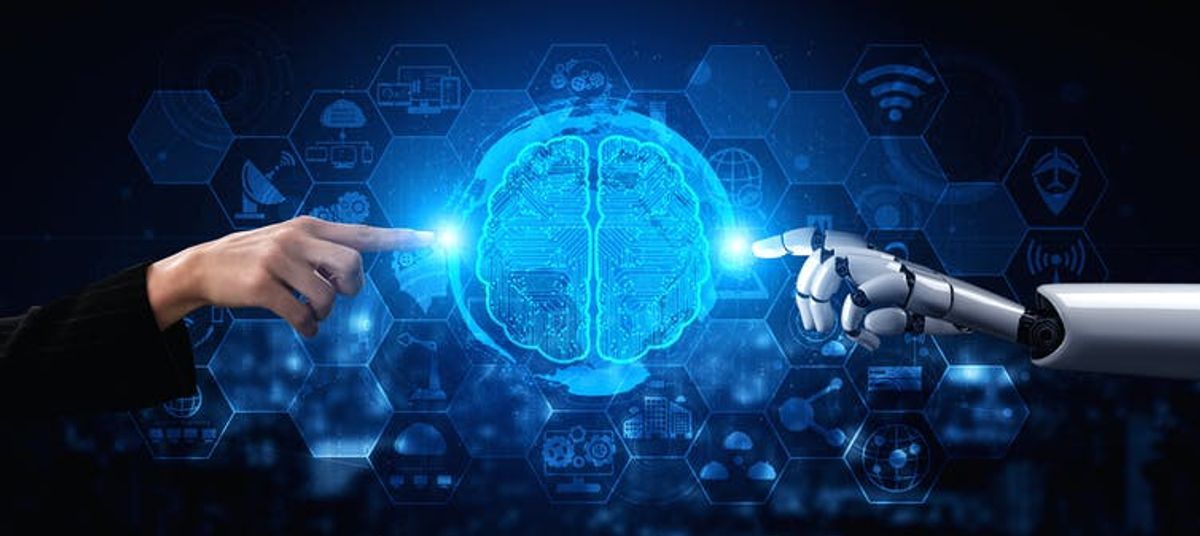
Exploring the Potential of Generative Models
Generative AI models are at the forefront of the emerging trends in Business Intelligence for 2024. These models have the capacity to transform a variety of business functions, from marketing to legal, by providing innovative solutions and automating routine tasks. For instance, a marketing team might use generative AI to create compelling advertising content, while a legal department could automate the drafting of standard documents.
Generative AI is not just about automation; it's about enhancing creativity and efficiency. By analyzing existing data, these models can generate new patterns, ideas, or even predict future trends, which can be particularly useful in dynamic sectors like consumer goods or energy.
- Consumer goods companies can refine pricing strategies.
- Energy firms optimize operations from supply to production.
The true value of generative AI lies in its ability to unlock new opportunities and drive innovation, making it a critical component for businesses looking to maintain a competitive edge.
As businesses integrate generative AI into their operations, it's crucial to focus on the quality of the data fed into these models. Accurate data and advanced analytics are the bedrock of effective generative AI applications, ensuring that the insights and content generated are both relevant and actionable.
Integrating Generative AI into Product Development
The integration of generative AI into product development marks a transformative step in leveraging technology for creative innovation. Generative AI steps in by offering a fresh perspective, generating designs that may have yet to be conceived through conventional methods. This technology can be particularly impactful in areas such as marketing, where it can produce innovative creative content, or in legal departments for drafting standardized documents.
The adoption of generative AI should be driven by the desire to meet unmet customer needs and enhance product desirability. It's crucial to ensure that the drive for innovation is aligned with the company's strategic vision and operational capabilities, rather than being solely motivated by the allure of new technologies.
The benefits of deploying generative AI are significant, often leading to efficiency improvements and fostering a competitive edge in the market. However, it's essential to integrate these solutions into the existing IT, data, and process frameworks thoughtfully.
Here are some considerations when integrating generative AI into product development:
- Assess the alignment with strategic business goals
- Ensure the technology addresses specific customer needs
- Evaluate the potential for efficiency improvements
- Plan for thoughtful integration with existing systems
By focusing on these aspects, businesses can harness the power of generative AI to innovate effectively and maintain relevance in a rapidly evolving market.
Ethical Considerations in Generative AI
As businesses explore the potential of generative AI, it is crucial to address the ethical implications that accompany its use. The creation and deployment of generative models must be guided by ethical standards to prevent misuse and ensure responsible innovation.
Transparency in how generative AI models are built and operate is essential for maintaining public trust. Users should be informed when they are interacting with content generated by AI, and the processes behind AI creations must be open to scrutiny.
- Consideration of data privacy and consent
- Mitigation of biases in AI-generated content
- Adherence to intellectual property rights
The ethical use of generative AI not only protects the rights of individuals and creators but also fosters a sustainable environment for innovation.
It is imperative for organizations to establish clear guidelines and governance structures to oversee the ethical aspects of generative AI. This includes regular audits, stakeholder engagement, and continuous education on the evolving landscape of AI ethics.
Measuring the Impact of Data Initiatives
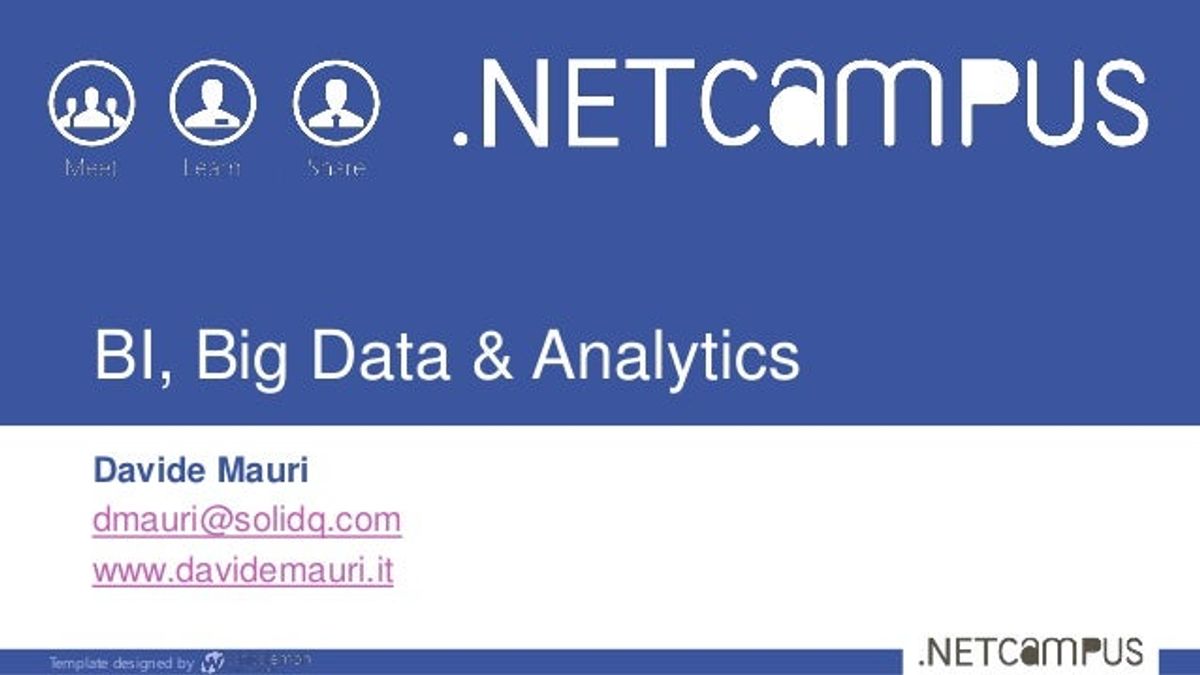
Setting KPIs for Data Science Projects
In the realm of data science, Key Performance Indicators (KPIs) serve as the compass that guides projects towards strategic goals. Establishing KPIs is a critical step in ensuring that data initiatives are aligned with business objectives and are capable of driving meaningful outcomes.
Leaders play a pivotal role in nurturing an environment that prizes data-informed decision-making. This entails setting up clear KPIs and developing frameworks to ensure that operational activities are in harmony with overarching strategic objectives.
A well-structured KPI framework might include:
- Quantitative metrics such as revenue impact or cost savings
- Qualitative measures like customer satisfaction or employee engagement
- Efficiency indicators, for example, time to insight or model accuracy
Understanding these nuances will enable you to define a precise vision and realize the substantial benefits of informed decision-making in guiding your company's direction.
Assessing ROI of Data-Driven Changes
The assessment of ROI (Return on Investment) for data-driven changes is a critical step in understanding the value and impact of these initiatives. Evaluating the financial benefits against the costs involved provides a clear picture of the profitability and effectiveness of the data strategies implemented.
To accurately measure ROI, businesses should consider both direct and indirect benefits. Direct benefits are quantifiable and often include increased revenue, reduced costs, and improved efficiency. Indirect benefits, while harder to quantify, can be equally significant, encompassing improved customer satisfaction, enhanced decision-making, and a stronger competitive position.
- Direct Benefits:
- Increased Revenue
- Reduced Costs
- Improved Efficiency
- Indirect Benefits:
- Enhanced Decision-Making
- Improved Customer Satisfaction
- Stronger Competitive Position
It is essential to adopt a comprehensive approach that accounts for both tangible and intangible outcomes when assessing the ROI of data-driven changes. This ensures a holistic view of the benefits that extend beyond mere financial metrics.
Continuous Improvement through Data Feedback Loops
The journey towards a data-driven enterprise is perpetual, with continuous improvement at its core. Data feedback loops are essential for refining processes, products, and strategies over time. By systematically analyzing outcomes and integrating insights back into the business cycle, organizations can adapt and evolve in an ever-changing market.
Feedback loops serve as a mechanism for ongoing learning and development. They enable businesses to test hypotheses, measure results, and iterate with precision. This cycle of learning is captured succinctly in the following steps:
- Collect data from various touchpoints
- Analyze the data to glean actionable insights
- Implement changes based on these insights
- Measure the impact of these changes
- Use the results to inform the next cycle of improvements
Embracing this iterative process ensures that data-driven initiatives remain aligned with organizational goals and market demands, fostering a culture of excellence and innovation.
It is crucial to establish clear metrics to gauge the effectiveness of data initiatives. These metrics should reflect both the performance of the data-driven solutions and their alignment with the strategic objectives of the organization. A table of Key Performance Indicators (KPIs) can serve as a guide for assessing progress and areas for enhancement:
KPI | Description | Target | Status |
---|---|---|---|
Customer Satisfaction | Measure of customer feedback post-implementation | Increase by 10% | In Progress |
Operational Efficiency | Reduction in process time or cost | Reduce by 15% | Achieved |
Revenue Growth | Increase in revenue attributed to data-driven changes | Grow by 20% | Pending |
By regularly reviewing and updating these KPIs, businesses can maintain a clear focus on what matters most, ensuring that their data-driven journey is both impactful and sustainable.
In today's data-driven landscape, the success of your business hinges on the efficiency and optimization of your databases. Measuring the impact of data initiatives is crucial, and that's where OptimizDBA comes into play. With unparalleled experience and a guarantee of performance increases, we stand ready to elevate your data operations to new heights. Don't let slow transaction speeds hold you back. Visit our website to learn how our proprietary methodologies can transform your data management and give you the competitive edge you need. Let's talk about your challenges and unlock the full potential of your data today!
Conclusion
In the journey to harness the full potential of data science for business intelligence, it is clear that a strategic, data-driven approach is paramount. Companies that successfully integrate data and analytics into their decision-making processes and operational frameworks not only enhance their efficiency but also pave the way for innovation and competitive advantage. The transition to being data-driven and data-enabled is not without its challenges, yet the rewards are transformative, offering a strategic edge that transcends the allure of AI hype. As organizations look to the future, the key to success lies in cultivating a culture that values data-driven insights, while carefully considering the integration of data-powered products to meet customer needs and industry demands. By doing so, businesses can move beyond trends, making informed decisions that drive growth and foster enduring success.
Frequently Asked Questions
What is Business Intelligence and how does it relate to Analytics?
Business Intelligence (BI) refers to the strategies and technologies used by enterprises for data analysis of business information. It is closely related to Analytics, which is the systematic computational analysis of data. BI leverages analytics to transform data into actionable insights for strategic decision-making.
How does a data-driven approach enhance strategic decision-making?
A data-driven approach improves strategic decision-making by providing evidence-based insights that inform management and operational decisions. This leads to more accurate predictions, better risk management, and a competitive edge through smarter, more efficient, and strategic actions.
What is a Data & AI Maturity Model?
A Data & AI Maturity Model is a framework that assesses the level of an organization's capabilities in managing and leveraging data and AI technologies. It helps companies understand their current position and guides them in developing a roadmap to achieve higher levels of data and AI maturity.
Why is it important to align data strategy with business goals?
Aligning data strategy with business goals ensures that the data initiatives support the overall objectives of the company. It helps in prioritizing data projects that will have the most significant impact on the business's success and ensures that resources are invested wisely.
What are the benefits of integrating AI and Machine Learning into business operations?
Integrating AI and Machine Learning into business operations can lead to improved efficiency, cost savings, and enhanced customer experiences. It allows for automation of routine tasks, more accurate forecasting, personalized customer interactions, and innovative product features.
How can companies ensure data quality and accuracy?
Companies can ensure data quality and accuracy by implementing robust data governance policies, regularly auditing and cleaning data, employing data validation techniques, and using advanced data management tools. Training employees to understand the importance of data integrity is also crucial.
What are the key considerations when adopting AI-powered products?
When adopting AI-powered products, key considerations include the alignment with customer needs, the potential for enhancing product desirability, the readiness of the organization's infrastructure, ethical implications, and ensuring that the technology adds real value rather than following trends.
How should companies measure the impact of their data initiatives?
Companies should measure the impact of their data initiatives by setting clear Key Performance Indicators (KPIs) that align with business objectives, assessing the Return on Investment (ROI), and continuously improving through feedback loops that inform about the effectiveness and efficiency of the data projects.