In our digital world, data is like gold for companies and groups everywhere. It helps them understand customers, improve operations, and make smart choices. But there's so much data, and managing it can be tough. That's where AI comes in. By using AI in databases, we can handle data better and make faster, smarter decisions.
Key Takeaways
- AI helps databases work smarter and faster by analyzing and predicting data patterns.
- Using AI in databases can improve data quality and reduce mistakes.
- AI-powered databases can automate many tasks, saving time and effort.
- Challenges like data privacy and bias need to be addressed when using AI in databases.
- The future of data management will see even more AI integration, making data handling easier and more efficient.
The Role of AI in Modern Databases
Understanding AI Integration
Artificial Intelligence (AI) is transforming how we manage and use databases. By integrating AI, databases can now handle tasks that were once too complex for humans. This includes analyzing large amounts of data quickly and accurately. AI integration allows databases to learn from data patterns, making them smarter over time.
Benefits of AI in Databases
The benefits of AI in databases are numerous. Firstly, AI can automate routine tasks, freeing up time for more important work. Secondly, AI can improve data accuracy by identifying and correcting errors. Lastly, AI can provide deeper insights into data, helping businesses make better decisions. With AI, databases become more efficient and reliable.
Challenges and Solutions
Despite the benefits, integrating AI into databases comes with challenges. One major challenge is ensuring data quality. If the data is not accurate, the AI will not work properly. Another challenge is the complexity of AI algorithms, which can be difficult to implement. However, these challenges can be overcome with proper planning and the right tools. For example, using AI-assisted micro-courses can help professionals learn how to integrate AI into databases effectively.
AI-Driven Data Management
Automated Data Integration
AI makes it easier to combine data from different sources. AI algorithms can automatically find and fix errors, making sure the data is clean and reliable. This saves time and reduces mistakes. For example, in e-commerce, AI can merge data from sales, customer interactions, and inventory, giving a clear picture of trends and preferences.
Real-Time Data Processing
With AI, data can be processed as soon as it is collected. This means businesses can get insights right away, helping them make quick decisions. AI can handle large amounts of data quickly, making it possible to analyze trends and patterns in real-time.
Enhanced Data Quality
AI helps keep data accurate and up-to-date. It can spot and correct errors, ensuring the information is always reliable. This is important for making informed decisions. AI also helps in maintaining data consistency, which is crucial for businesses to trust their data.
AI-driven data management tools efficiently scan metadata, analyze data relationships leveraging ML, and automate data lineage mapping. These functionalities help modern businesses keep up with the ever-evolving data deluge, guiding decision-making with actionable insights, reducing costs and errors, and streamlining workflows with automated data integration.
Machine Learning in Database Systems
Predictive Analytics
Machine learning (ML) is transforming databases by enabling predictive analytics. By analyzing historical data, ML algorithms can forecast future trends and behaviors. This is particularly useful in industries like retail, where predicting customer purchasing patterns can lead to better inventory management and targeted marketing strategies. Predictive analytics helps businesses make informed decisions, reducing risks and optimizing operations.
Adaptive Learning Algorithms
Adaptive learning algorithms are a key component of modern database systems. These algorithms continuously learn from new data, improving their accuracy over time. This means that the more data they process, the smarter they become. For example, in the finance sector, adaptive algorithms can detect fraudulent transactions by learning from past fraud patterns. This continuous improvement makes databases more reliable and efficient.
Use Cases Across Industries
Machine learning in databases has a wide range of applications across various industries:
- E-commerce: Personalizing shopping experiences by analyzing customer behavior.
- Healthcare: Predicting disease outbreaks and personalizing treatment plans.
- Finance: Assessing credit risk and detecting fraud in real-time.
The integration of machine learning into database systems is not just a trend; it's a revolution that is reshaping how we manage and utilize data. The ability to harness AI to revolutionize database management is a game-changer for many industries.
Machine learning is making databases smarter, more efficient, and more capable of handling complex tasks. As these technologies continue to evolve, their impact on data management will only grow, offering new opportunities and challenges for businesses worldwide.
Natural Language Processing for Databases
Text and Sentiment Analysis
Natural Language Processing (NLP) enables databases to understand and process human language. Text and sentiment analysis are key applications, allowing businesses to gauge customer opinions from reviews and social media. This helps in making data-driven decisions and improving customer satisfaction.
Voice-Activated Data Queries
Voice-activated data queries are transforming how users interact with databases. By using voice commands, users can retrieve information quickly and efficiently. This technology is especially useful in environments where typing is impractical, such as in healthcare or while driving.
NLP in Customer Feedback
NLP can analyze customer feedback to identify common issues and areas for improvement. By understanding the sentiments expressed in feedback, companies can better address customer needs and enhance their products or services.
NLP is revolutionizing how we interact with and understand data, making it more accessible and actionable for everyone.
Edge Computing and AI in Databases
Benefits of Edge Computing
Edge computing brings data processing closer to the source, reducing latency and improving real-time decision-making. This is especially important for applications that require immediate responses, such as autonomous vehicles and industrial automation. By processing data locally, edge computing minimizes the need for constant communication with centralized data centers, which can be both time-consuming and costly.
AI at the Edge
Integrating AI capabilities into edge computing environments allows for real-time data analysis and enhanced decision-making. This combination enables devices to operate more independently and efficiently. For instance, AI can help in predictive maintenance by analyzing data from sensors in real-time to predict equipment failures before they happen. Embracing cutting-edge technology is essential for competitiveness.
Case Studies
Several industries have successfully implemented edge computing and AI to improve their operations. In the healthcare sector, edge computing is used to process patient data locally, ensuring quick and accurate diagnostics. In manufacturing, AI-driven edge computing systems monitor machinery to optimize performance and prevent downtime. These examples highlight the transformative potential of combining edge computing with AI.
The integration of AI in business processes exemplifies how analysts can leverage technology for robust solutions.
By adopting these technologies, businesses can stay ahead of the curve and unlock new efficiencies, reshaping the landscape with unified platforms.
AI-Powered Automation in Data Management
Automating Routine Tasks
AI technology is transforming how businesses handle data by automating routine tasks. This includes data collection, storage, and cleaning. Automation improves accuracy, consistency, and scalability, allowing businesses to focus on strategic initiatives. AI tools can scan metadata, analyze data relationships, and automate data lineage mapping, making data management more efficient.
Optimizing Workflows
AI enhances workflows by streamlining data integration processes. Techniques like ETL (Extract, Transform, Load) and ELT (Extract, Load, Transform) are now more efficient with AI. These methods help break down data silos, enabling businesses to scale operations as needed. AI-driven databases continuously learn from data patterns, improving their ability to clean and integrate data over time.
Reducing Operational Costs
By automating data management tasks, AI significantly reduces operational costs. Businesses can save time and resources that would otherwise be spent on manual data handling. This cost reduction allows companies to allocate more resources to strategic analysis and decision-making. Additionally, AI revolutionizes data-driven decision-making by analyzing vast data sets to uncover patterns and insights, ensuring informed decisions based on accurate data.
Embracing AI in data management not only enhances efficiency but also fosters innovation by streamlining workflows and reducing costs.
Ethical Considerations in AI-Driven Databases
Transparency and Accountability
In the realm of AI-driven databases, transparency and accountability are crucial. AI systems often operate as "black boxes," making decisions without clear explanations. This lack of transparency can be problematic, especially in critical areas like healthcare and criminal justice. To address this, organizations must establish mechanisms for auditing AI models, monitoring their performance, and ensuring they provide understandable explanations for their decisions.
Bias and Fairness
AI algorithms learn from historical data, which may reflect societal biases. If not properly managed, these biases can perpetuate inequality and discrimination. Organizations must prioritize fairness by conducting bias assessments, diversifying training data, and implementing explainable AI algorithms. This ensures that AI-driven decisions are fair and just.
Regulatory Compliance
Navigating the regulatory landscape is another challenge. AI technologies are subject to evolving legal frameworks governing data privacy, intellectual property rights, and algorithmic accountability. Organizations must stay abreast of regulatory developments and engage in dialogue with policymakers to shape ethical and legal standards for AI deployment. This includes ensuring compliance with data protection regulations like GDPR and CCPA.
Fostering a culture of collaboration and knowledge-sharing across disciplines is crucial. Interdisciplinary teams can bring diverse perspectives to address challenges holistically, ensuring that AI deployments align with organizational values and societal expectations.
Future Trends in Database AI
Emerging Technologies
The future of database AI is bright with many new technologies on the horizon. One key trend is the integration of AI with edge computing. This allows data to be processed closer to where it is generated, reducing latency and improving real-time decision-making. Enhanced natural language processing (NLP) is also set to revolutionize how databases handle unstructured data like text and audio.
Market Dynamics
Market dynamics are shifting as more businesses recognize the value of AI in data management. Companies are investing heavily in AI-driven automation to optimize workflows and improve operational efficiency. This trend is pushing database administrators to adapt by acquiring new skills for managing diverse databases in hybrid and multi-cloud environments.
Predictions for the Next Decade
Looking ahead, we can expect AI to play an even bigger role in data management. AI-powered databases will become more autonomous, capable of not just storing and retrieving data but also analyzing and predicting trends. This will free up human resources for more strategic tasks, driving innovation and competitiveness.
As we move forward, the integration of AI in databases will not only enhance data management but also transform how businesses operate, making them more agile and responsive to market changes.
Building Scalable AI-Enhanced Database Infrastructures
Cloud Computing Solutions
Cloud computing offers a flexible and scalable solution for AI-enhanced databases. By leveraging cloud services, organizations can easily scale their database infrastructure to meet growing data and computational demands. Cloud platforms provide on-demand resources, allowing businesses to adjust their capacity as needed without significant upfront investments.
Hybrid IT Architectures
Hybrid IT architectures combine on-premises and cloud resources to create a more adaptable infrastructure. This approach allows organizations to maintain control over sensitive data while benefiting from the scalability and flexibility of the cloud. Hybrid solutions are particularly useful for businesses with fluctuating workloads or specific compliance requirements.
Scalability Challenges and Solutions
Scaling AI-enhanced databases comes with its own set of challenges. These include managing data consistency, ensuring high availability, and optimizing performance. To address these issues, organizations can implement strategies such as data partitioning, load balancing, and using distributed databases. These solutions help maintain performance and reliability as the database grows.
AI and Big Data: A Symbiotic Relationship
Understanding Big Data
Big data refers to the massive volumes of data generated every second. In 2020, the average person created 1.7 megabytes of data every second. This data comes from various sources like smartphones, tablets, and IoT devices. AI and big data are becoming inseparable because AI helps in parsing and understanding this enormous amount of data. To make the most out of big data, a good database foundation is essential to ensure the data is accurate and useful.
AI for Big Data Analytics
AI plays a crucial role in analyzing big data. It can sift through and parse data into a format that data scientists can work with. AI's learning capabilities allow it to recognize trends and adapt customer interactions accordingly. This makes AI an invaluable tool for businesses looking to gain insights from their data. For example, AI can help improve geolocation technology by analyzing data from various devices.
Transforming Data into Insights
AI transforms raw data into actionable insights. By integrating AI into databases, businesses can make big data useful. AI can automatically categorize and tag data, making it easier to analyze. This not only saves time but also makes the data more valuable. AI's predictive capabilities enable businesses to forecast trends and outcomes with heightened accuracy, thereby fostering agility and competitive advantage.
The integration of AI and big data is revolutionizing how businesses operate, making data an asset rather than a burden.
Collaborative Approaches to AI in Databases
Partnerships and Alliances
Collaborating with technology partners, research institutions, and startups can speed up the adoption of new AI technologies. This teamwork helps in sharing knowledge and resources, making it easier to tackle complex problems. Partnerships can also bring in diverse perspectives, which is crucial for innovative solutions.
Research and Development
Investing in research and development (R&D) is essential for staying ahead in the AI game. Companies should allocate resources to develop new AI algorithms and models. This not only fosters innovation but also helps in maintaining a competitive edge. R&D efforts can lead to breakthroughs that make AI more effective and efficient.
Knowledge Sharing
Sharing knowledge across different teams and departments is vital for the successful implementation of AI in databases. This includes sharing best practices, lessons learned, and new discoveries. A culture of knowledge-sharing ensures that everyone is on the same page and can contribute to the project's success.
Embracing a mindset of responsible innovation involves proactively engaging with stakeholders—including customers, employees, regulators, and communities—to solicit feedback, address concerns, and demonstrate the positive impacts of Database AI on society.
Practical Applications of AI in Databases
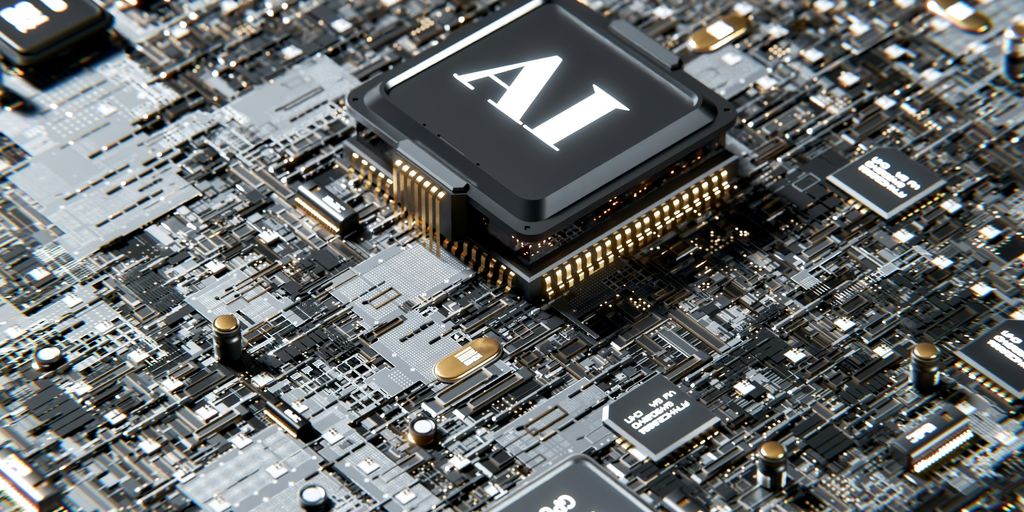
E-commerce and Retail
AI-driven databases are transforming the e-commerce and retail sectors by analyzing customer behavior and preferences in real-time. This allows businesses to offer personalized product recommendations, enhancing customer satisfaction and boosting sales. For instance, AI can analyze browsing history and purchase patterns to suggest products that a customer is likely to buy, both online and in-store.
Healthcare and Life Sciences
In healthcare, AI-powered databases can analyze vast amounts of patient data, including electronic health records (EHRs), medical imaging, and genetic information. By identifying patterns within these datasets, AI assists healthcare providers in diagnosing diseases earlier and personalizing treatment plans. This leads to better patient outcomes and more efficient medical research.
Finance and Banking
The finance and banking sectors benefit from AI-driven databases by enhancing fraud detection and risk management. AI algorithms can monitor transactions in real-time, identifying unusual patterns that may indicate fraudulent activity. Additionally, AI helps in credit scoring by analyzing a wide range of data points, making the process more accurate and inclusive.
AI in databases is not just a technological advancement; it's a game-changer across various industries, offering solutions that were previously unimaginable.
Enhanced Security Measures
AI-powered databases can proactively identify and mitigate potential security threats by continuously monitoring data access patterns and anomalies. This proactive approach strengthens data security and compliance with regulations such as GDPR and CCPA.
Conclusion
In summary, the integration of AI into databases is transforming how we manage and utilize data. By automating tasks, improving data quality, and providing real-time insights, AI-powered databases are making data management more efficient and effective. As we look to the future, embracing these technologies will be crucial for staying competitive and driving innovation. Organizations that invest in AI and adapt to emerging trends will be well-positioned to harness the full potential of their data, leading to smarter decision-making and greater success.
Frequently Asked Questions
What is Database AI?
Database AI means adding artificial intelligence to database systems. This helps them not only store data but also analyze and predict patterns in it.
How does AI improve data management?
AI helps by automating tasks like data integration and cleaning. This makes data more accurate and reduces the time needed for manual work.
What are the benefits of using AI in databases?
AI in databases can speed up data processing, improve data quality, and offer real-time insights, making it easier to make smart decisions.
What challenges come with AI in databases?
Challenges include the need for high-quality data, dealing with biases in AI models, and ensuring data security and privacy.
How does AI help in real-time data processing?
AI can quickly analyze data as it comes in, allowing for immediate insights and actions. This is especially useful in industries like finance and healthcare.
What is the role of machine learning in databases?
Machine learning helps databases learn from data over time, improving their predictions and the accuracy of their analyses.
How is natural language processing (NLP) used in databases?
NLP helps databases understand and analyze text data, making it possible to do things like sentiment analysis and voice-activated queries.
What ethical considerations are there for AI-driven databases?
It's important to ensure transparency, avoid biases, and comply with regulations to maintain trust and accountability in AI systems.