Unleashing the Potential of Data through Advanced Analytical Techniques
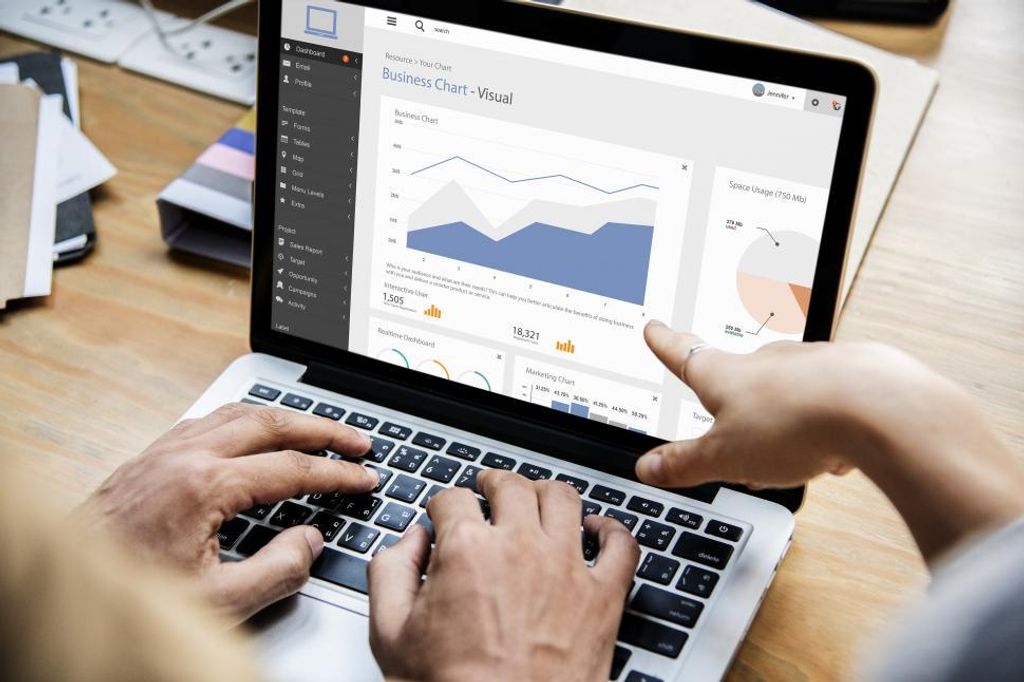
Predictive Analytics
Predictive analytics harnesses historical data patterns and machine learning algorithms to forecast future trends and events. This analytical power is pivotal in enhancing demand forecasting, optimizing supply chain management, and crafting targeted marketing strategies.
Predictive analytics is not just about foreseeing the future; it's about shaping it. By analyzing past and current data, businesses can anticipate customer needs, adjust strategies proactively, and maintain a competitive edge.
Predictive analytics transforms raw data into a strategic asset, enabling informed decision-making and foresight into market shifts.
The integration of AI and ML in predictive analytics automates and refines the analysis process, making it faster and more accurate. As businesses move towards a data-driven culture, predictive analytics becomes a cornerstone for strategic decisions, ensuring that they are not based on gut instinct but on solid data insights. Compliance with evolving data privacy regulations is also crucial in leveraging predictive analytics responsibly.
Data-driven Decision Making
In a fast-paced, data-driven world, businesses must harness the massive volumes of data to stay ahead. Advanced analytics transform raw data into actionable insights, optimizing strategies for a competitive edge.
Cloud platforms enable businesses to test new ideas with minimal risk, ensuring security and compliance, and driving growth through automation and scalability.
Embracing data-driven decision making is not just about collecting data; it's about cultivating a culture that values evidence-based strategies and continuous learning.
To foster a data-driven culture, consider these points:
- Ensure data quality and consistency for reliable insights.
- Balance data-driven insights with human intuition and creativity.
- Prioritize data security and privacy to protect sensitive information.
Strategic Business Intelligence
Strategic Business Intelligence (BI) transcends traditional data analysis by integrating technologies and practices that not only present business information but also drive long-term planning and risk management. The essence of BI lies in its ability to transform data into actionable insights, guiding organizations through the complexities of strategic planning.
- Collection and integration of business data
- Analysis and presentation of findings
- Future forecasting and strategic planning
Strategic BI is pivotal in cultivating a data-driven culture that emphasizes informed decision-making over intuition.
While BI traditionally focuses on descriptive analytics, it is increasingly incorporating predictive elements to enhance strategic decision-making. This evolution marks a shift from simply understanding current trends to anticipating future challenges and opportunities.
Harnessing the Power of AI/ML for Strategic Decision-Making
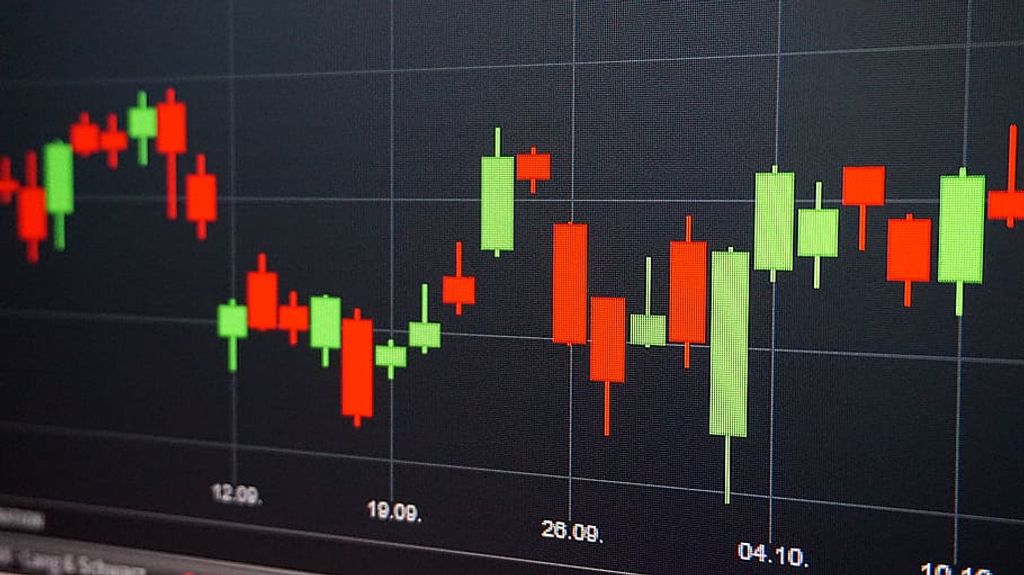
Integrating AI/ML into FP&A
The integration of Artificial Intelligence (AI) and Machine Learning (ML) into Financial Planning & Analysis (FP&A) is transforming the landscape of financial forecasting and strategic planning. AI/ML technologies enable a more dynamic and precise approach to financial modeling, allowing organizations to anticipate market trends and make informed decisions.
Cloud adoption strategies are crucial in this transformation, as they ensure the selection of reliable and secure cloud service providers. A seamless transition to the cloud is facilitated by a thorough workload assessment, with a focus on security and compliance.
By leveraging AI/ML, FP&A teams can shift from traditional, static financial models to adaptive, real-time predictive analytics that drive strategic business outcomes.
The table below outlines the key components of a successful AI/ML integration into FP&A:
Component | Description |
---|---|
Data Management | Ensuring data quality and accessibility for AI/ML algorithms. |
Model Development | Creating predictive models tailored to specific financial scenarios. |
Technology Infrastructure | Building a robust tech stack to support AI/ML initiatives. |
Skill Enhancement | Training FP&A professionals in AI/ML techniques and tools. |
Governance | Establishing policies for ethical AI use and model transparency. |
Decoding Possibilities with Data-driven Analytics
In the realm of strategic decision-making, data-driven analytics stands as a beacon of potential, guiding businesses through the complexities of the modern market. By leveraging the power of analytics, organizations can unlock a myriad of possibilities that were previously obscured by the limitations of traditional decision-making processes.
Data-driven analytics not only illuminates the path to innovation but also equips leaders with the foresight to anticipate market shifts and customer needs.
The following steps outline the journey to harnessing analytics for strategic advantage:
- Predictive Analytics: Utilize historical data and machine learning to forecast future trends.
- Prescriptive Analytics: Generate actionable recommendations to inform decision-making.
- Operational Efficiency: Enhance agility and drive performance through informed choices.
Embracing these analytical techniques can propel a business towards a future where decisions are not just reactive, but proactive and informed by a deep understanding of data's transformative power.
Overcoming Challenges in AI/ML Implementation
Implementing AI/ML solutions is a transformative step for organizations, but it comes with its own set of challenges. Ensuring data quality and consistency is crucial for the success of AI/ML projects. Without accurate and relevant data, the insights derived can be misleading and detrimental to decision-making processes.
Cloud computing offers a supportive infrastructure for AI/ML, providing cost savings, scalability, and flexibility. However, organizations must navigate through issues such as data migration, vendor lock-in, and compliance concerns to fully harness its benefits. Streamlined collaboration and improved data security are additional advantages that can enhance business operations.
Overcoming these challenges requires a strategic approach that includes thorough planning, continuous monitoring, and the willingness to adapt to new information and changing market conditions.
Here are some common challenges faced in AI/ML implementation:
- Data Quality and Consistency
- Data Migration Difficulties
- Vendor Lock-in Dilemmas
- Compliance and Regulatory Hurdles
- Adapting to Evolving Patterns and Trends
Driving Transformational Growth with Data-driven Decision Making
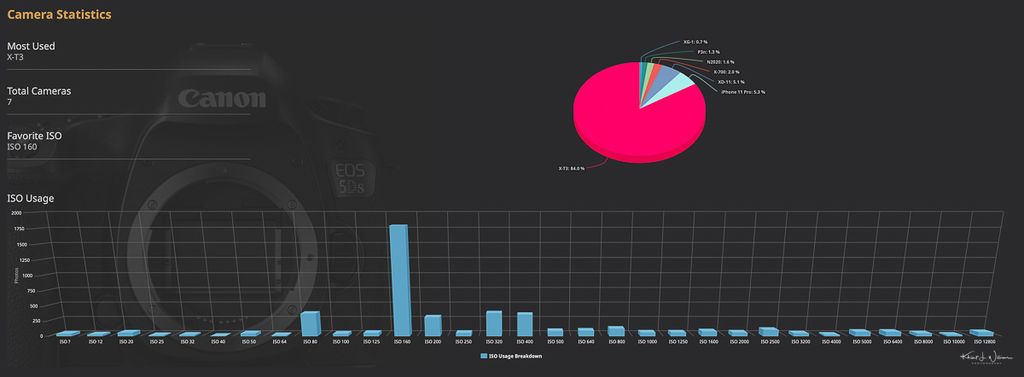
Cultivating a Data-driven Culture
To truly unleash the potential of analytics, organizations must develop robust strategies to harness their data's full potential and gain a competitive edge. Establishing a data-driven culture is not just about having access to data; it's about embedding data into the core decision-making processes. Here are key steps to success:
- Define Clear Objectives and Metrics: Start by setting specific, measurable goals that align with your business strategy.
- Ensure Data Quality and Consistency: High-quality data is the foundation for reliable insights.
- Balance Data and Intuition: Combine data-driven insights with human judgment for a holistic approach.
- Foster Continuous Learning: Stay updated with the latest analytics tools and techniques.
- Invest in Tools and Talent: Equip your team with the right tools and hire skilled professionals to interpret data.
Embracing a data-driven culture requires a shift from intuition-based decisions to strategies informed by data. This transition is pivotal for organizations aiming to thrive in today's competitive landscape.
While cultivating a data-driven culture, it's crucial to recognize the risks of intuition-based decisions and the benefits of data-driven strategies. By encouraging employees at all levels to rely on data over gut feelings, businesses can make more informed, strategic decisions that drive transformational growth.
Overcoming Challenges in Data-driven Transformation
The journey towards a data-driven transformation is fraught with obstacles, but the rewards are substantial. Ensuring data quality and consistency is paramount, as it forms the foundation for generating actionable insights. Establishing robust data management processes is essential to maintain high-quality datasets and prevent decisions based on faulty information.
While data is critical, it's important to balance it with human intuition and creativity. A holistic approach that values both data-driven insights and human judgment can lead to more nuanced and effective decision-making strategies.
As the landscape of business continues to evolve, the ability to adapt and innovate becomes crucial. Data-driven decision making is the key to unlocking informed strategies that optimize performance and secure a competitive edge.
The table below outlines the primary challenges and strategies for overcoming them in the context of data-driven transformation:
Challenge | Strategy |
---|---|
Data Quality and Consistency | Implement robust data management processes |
Balancing Data and Intuition | Foster a culture that values both analytics and experience |
Future of Intelligent Decision Making
As the digital age progresses, the landscape of intelligent decision making is poised to undergo a significant transformation. Predictive analytics will evolve beyond simple trend analysis, enabling companies to anticipate and shape future scenarios. The integration of artificial intelligence (AI) and machine learning (ML) will further automate and refine the decision-making process, leading to more accurate and timely insights.
The convergence of AI, ML, and advanced analytics will redefine how organizations approach strategic decisions, making them more proactive rather than reactive.
The following points highlight the anticipated changes in intelligent decision making:
- Enhanced predictive capabilities through advanced algorithms
- Increased automation in data analysis for efficiency
- A shift towards data-driven cultures across all organizational levels
- Stricter adherence to evolving data privacy regulations
These developments will not only streamline decision-making processes but also ensure that businesses remain agile and compliant in a rapidly changing environment.
Strategic Guidance through Advanced Analytics
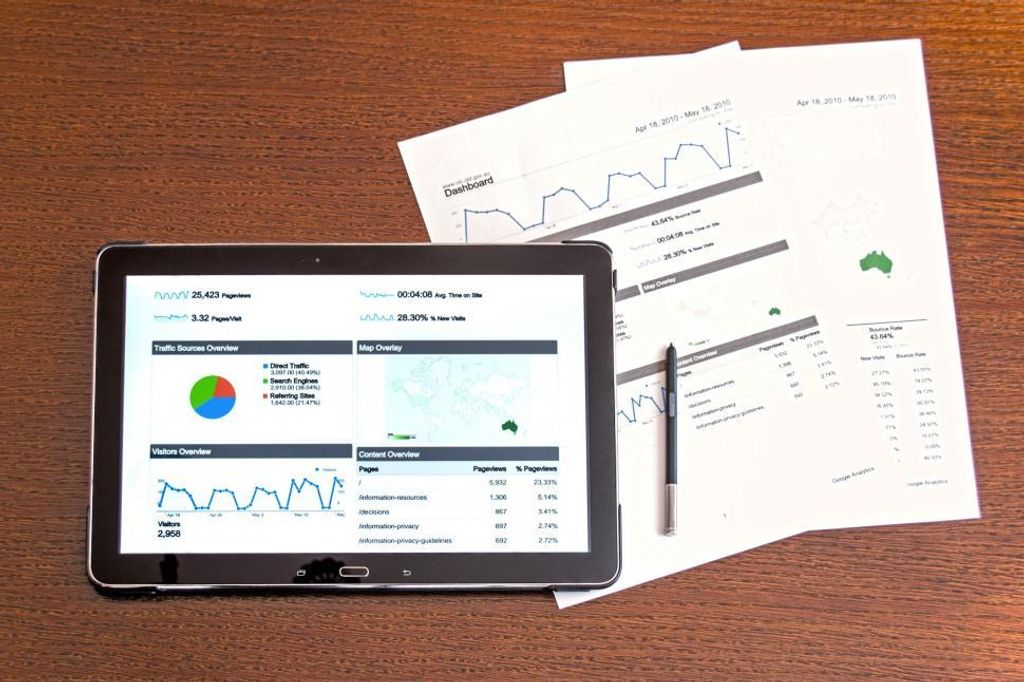
Predictive Modeling for Strategic Decisions
In the realm of strategic decision-making, predictive modeling stands as a cornerstone, enabling businesses to anticipate market trends and customer behaviors with remarkable accuracy. By integrating predictive analytics into their strategic arsenal, companies can outline potential future scenarios and make informed decisions that steer them towards success.
Predictive modeling transforms raw data into actionable insights, offering a foresight that is invaluable in navigating the complexities of today's business environment.
The process of predictive modeling often involves the following steps:
- Data Collection: Gathering relevant historical data.
- Data Processing: Cleaning and preparing data for analysis.
- Model Building: Selecting algorithms and constructing predictive models.
- Validation: Testing models to ensure accuracy and reliability.
- Deployment: Implementing models into decision-making processes.
Harnessing the power of predictive modeling not only provides strategic guidance but also helps in reducing bias inherent in human judgment. It's a proactive action for businesses faced with an uncertain future, allowing them to craft strategies that are both resilient and adaptable.
Reducing Bias in Decision-making
Cognitive biases can significantly skew strategic decision-making, leading to suboptimal outcomes. Advanced analytics serve as a counterbalance, providing an objective lens through which to view data and make informed decisions. By leveraging AI and machine learning, organizations can minimize the influence of inherent biases and enhance the fairness of their strategic choices.
Advanced analytics tools dissect complex data sets to reveal patterns and insights that might otherwise be obscured by human prejudice or oversight.
To effectively reduce bias, consider the following steps:
- Establish a baseline of historical data to identify trends and patterns.
- Implement predictive models to forecast outcomes without human bias.
- Regularly review and adjust algorithms to ensure they remain unbiased and accurate.
Embracing these practices not only mitigates risk but also promotes a more equitable decision-making process. It is crucial, however, to maintain a balance between data-driven insights and human intuition to foster a holistic approach to strategic decisions.
Market Dynamics Analysis
In the realm of strategic decision-making, understanding market dynamics is pivotal. By leveraging advanced analytics, businesses can dissect the complex interplay of factors that influence market behavior. This deep dive into market dynamics allows for the anticipation of trends, the adaptation of strategies, and the making of informed decisions that drive growth and profitability.
Marketing-mix modeling (MMM) is one such technique that exemplifies the power of advanced analytics in market dynamics analysis. It models the interactions between marketing investments, sales drivers, and external variables like seasonality. This enables marketers to design strategies that are both cost-effective and revenue-generating, thereby identifying growth opportunities and optimizing resource allocation.
Persistently evolving through data-driven performance analysis ensures your company remains competitive. It encourages you to foresee market changes and pivot accordingly, achieving sustainable growth and leadership.
Understanding the financial implications of operational changes is crucial. An ROI-driven business case is essential for ensuring that projects not only appear viable but also deliver a satisfactory return on investment. This approach to market dynamics analysis is integral to maintaining a competitive edge and fostering long-term success.
Investing in Scalable Analytics Solutions
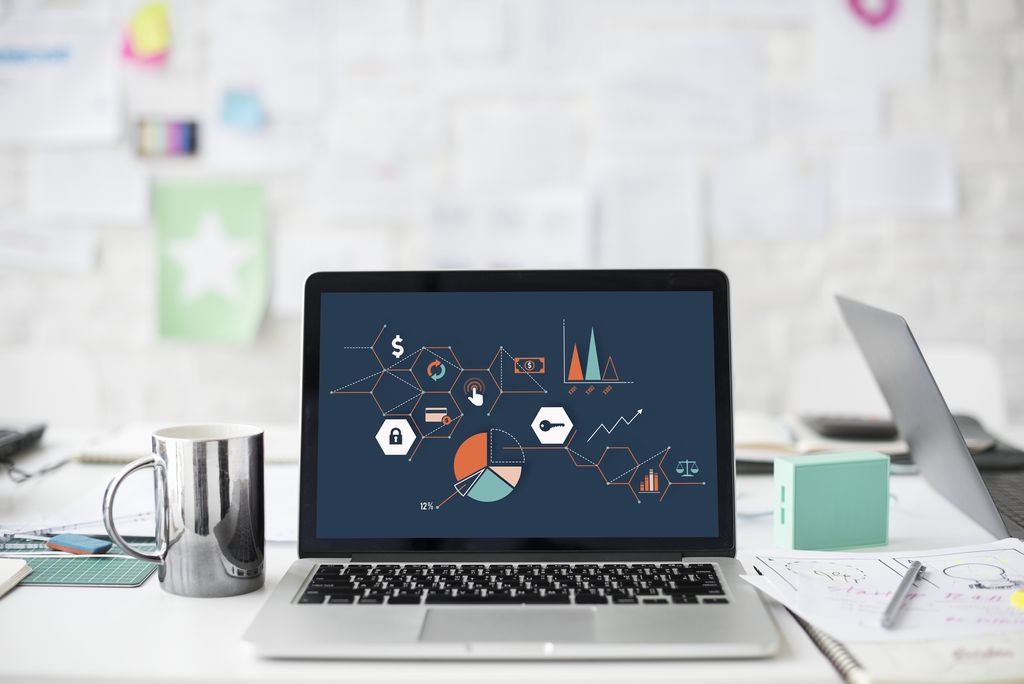
Scalable Growth Strategies
In the pursuit of scalable growth, it is essential to align strategies with long-term goals while also delivering immediate improvements. Quick-to-implement changes can create a ripple effect, enhancing productivity across all functions. By systematically reviewing processes and implementing targeted interventions, businesses can actualize swift value creation and set the stage for continuous improvement cycles.
Enterprise-wide integration is crucial for maximizing efficiency and productivity. Effective strategies should encompass the collective aspects of the business, ensuring that solutions are not siloed but influence various operational facets. This holistic approach allows for the impact of improvements to increase through interconnected departments.
Investing in scalable solutions allows a business to grow with its needs. As the business expands, robust systems endure, supporting scalable growth through expertise in managing and analyzing data effectively.
To truly capture the complexities of your industry, solutions must be agile and bespoke. Custom-built analytical tools and processes, akin to a "precision instrument," are required to harness unique insights that fuel process efficiency and elevate operational performance.
Cross-departmental Synergies for Innovation
Harnessing cross-functional collaborations unlocks innovation and drives enterprise-wide enhancements. Regular inter-departmental dialogue fosters an environment where collectively devised solutions have the potential to bring about transformative change. It's this cohesion that translates individual department strengths into comprehensive organizational advancement.
Embrace technologies that facilitate enterprise-wide transparency. Agile platforms and interoperable systems are fundamental in dismantling information silos, fostering a culture of collaboration.
Invest in scalable solutions to grow with your needs. Choose tools and protocols designed not only for immediate performance enhancements but also for setting the stage for continuous improvement cycles. Tailored analytics and decision-making frameworks must resonate with a firm's specific operational context and strategic objectives, ensuring strategies align with long-term goals while delivering immediate improvements.
Performance Benchmarking and Cost Optimization
Incorporating advanced analytics into performance benchmarking and cost optimization is a game-changer for businesses seeking to enhance decision-making precision. Advanced analytics transforms raw data into actionable insights, enabling organizations to dissect performance metrics and isolate cost drivers. This analytical approach is essential for strategic resource allocation and optimization initiatives.
Adopting a vigorous analysis not only underpins these initiatives but also facilitates detailed comparisons against industry benchmarks. Such benchmarking exposes areas ripe for innovation or cost reduction, leading to meaningful improvements in operational output and cost-efficiency.
By evaluating and improving process efficiency and workflow automation, companies can achieve substantial gains. Optimization through these levers encourages a proactive approach to operational challenges, ensuring that businesses remain competitive and agile in the face of market changes. It is crucial to understand the financial implications of operational changes, as projects must offer a satisfactory return on investment (ROI) to be considered successful.
Enhancing Decision-making Precision with Advanced Analytics
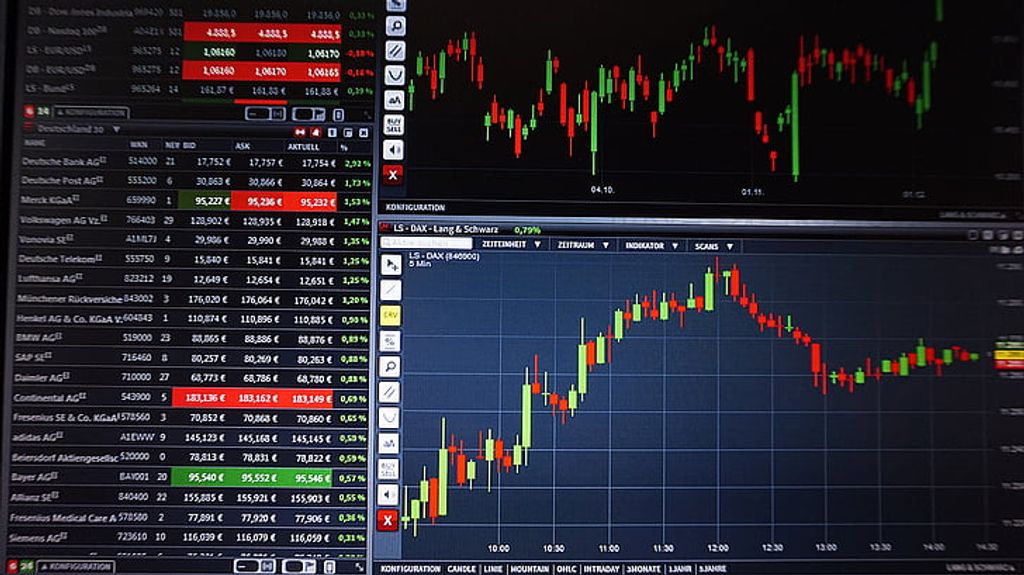
Interpreting Process Output Data
In the realm of advanced analytics, interpreting process output data is a cornerstone for enhancing decision-making precision. Ensuring data quality is the first critical step; without it, the foundation of analytics is compromised. Regular cleaning and validation are essential to maintain data integrity.
Applying appropriate statistical methods is next. These tools are pivotal in uncovering patterns, trends, and correlations that may not be immediately apparent. Here's a simple example of how data interpretation can manifest in a table format:
Metric | Q1 | Q2 | Q3 | Q4 |
---|---|---|---|---|
Sales | 20 | 35 | 45 | 50 |
Costs | 15 | 25 | 30 | 40 |
Profit | 5 | 10 | 15 | 10 |
By analyzing quarterly performance, stakeholders can identify areas of growth and concern, leading to more informed strategic decisions.
Understanding the interactions that influence efficiency provides invaluable perspectives. Qualitative diagnostics bring to light the human element, which is crucial for orchestrating impactful process improvements.
Isolating Cost Drivers
Incorporating advanced analytics into the process of isolating cost drivers is a transformative step towards strategic cost management. By dissecting performance metrics and leveraging data-driven analysis, businesses can pinpoint the cost drivers that significantly impact their bottom line. This analytical approach not only aids in resource allocation but also paves the way for comprehensive optimization initiatives.
Advanced analytics transforms raw data into actionable insights, which is essential for enhancing decision-making precision across an organization.
Understanding the financial implications of operational changes is paramount. An ROI-driven business case ensures that projects go beyond surface-level viability and contribute true value. Here's a simple breakdown of the value levers that can be pulled to drive process efficiency:
- Stabilize workflows to minimize fluctuations.
- Standardize processes for uniformity and ease of maintenance.
- Re-engineer complex systems for better performance.
- Automate repetitive tasks to increase efficiency.
Persistently evolving through data-driven performance analysis is not just about maintaining competitiveness; it's about foreseeing market changes and pivoting to achieve sustainable growth and leadership.
Quantifying Impact for Resource Allocation
In the realm of strategic decision-making, quantitative analysis underpins resource allocation and optimization initiatives. Adopting a vigorous analytical approach not only facilitates detailed comparisons against industry benchmarks but also exposes areas ripe for innovation or cost reduction. Through meticulous data analysis, organizations can identify granular inefficiencies that, when addressed, lead to meaningful improvements in operational output and cost-efficiency.
Measurement of quantifiable metrics is indispensable for assessing the effectiveness of process improvements. Precision in measurement is paramount, especially when analyzing the Return on Investment (ROI) of optimized processes. Accurate tracking reveals insights essential to strategic decision-making and sustained growth. The term quantitative underscores the objectivity of this empirical approach.
Incorporating advanced analytics is crucial for interpreting vast datasets related to process output. It transforms raw data into actionable insights, enhancing decision-making precision across your organization.
Business giants leverage data-driven analysis to systematically dissect performance metrics, isolating cost drivers, and quantifying their impact. This methodological approach is vital for robust financial management, ensuring resources are allocated effectively to drive organizational growth.
Utilizing Advanced Analytics for Forecasting and Decision Guidance
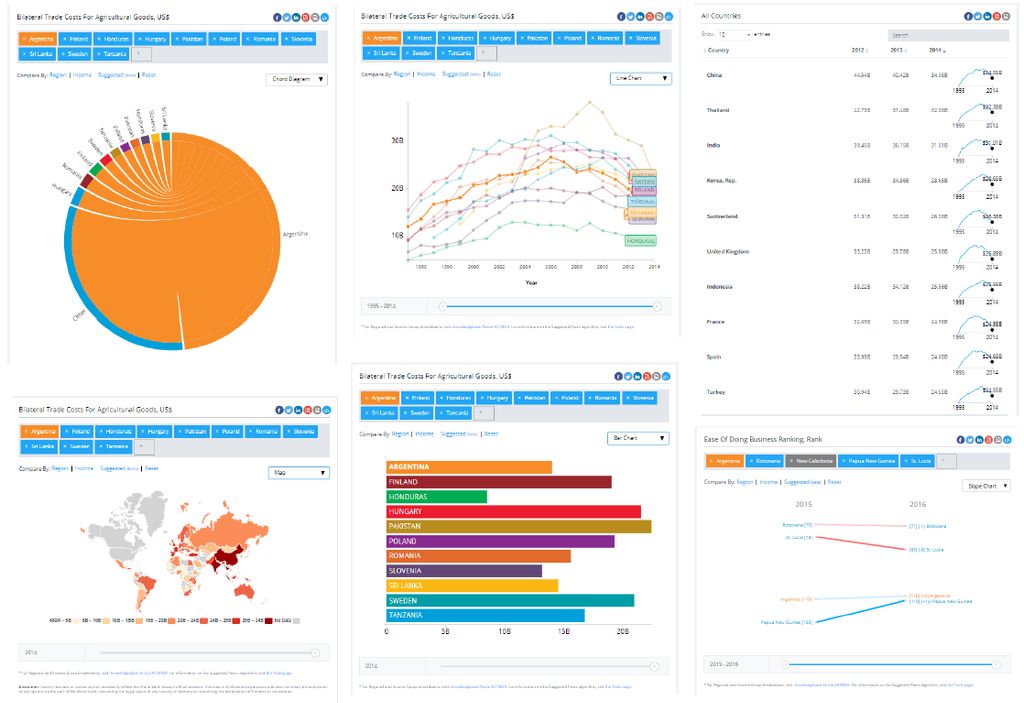
Forecasting Future Outcomes
The advent of advanced analytics has ushered in a new era of forecasting future outcomes with unprecedented precision. By leveraging predictive modeling, businesses can now anticipate potential scenarios based on a blend of current and historical data. This approach combines machine learning and data mining, two powerful tools that sift through vast datasets to identify trends, patterns, and anomalies.
Improved forecasting allows companies to make more informed decisions, tailoring strategies to meet the nuanced demands of their target markets. For instance, understanding customer behavior patterns leads to better customer acquisition and retention strategies.
Here are some key benefits of forecasting with advanced analytics:
- Enhanced understanding of customer behavior
- Proactive identification of market trends
- Strategic resource allocation based on predicted needs
As the cloud computing revolutionizes business strategy, it is essential to integrate these advanced capabilities to maintain a competitive edge. The journey involves careful planning, astute cost management, and addressing challenges such as data security and privacy.
Guiding Decision-making with Analytics
In the realm of strategic business decision-making, analytics act as a compass, guiding companies through the complex landscape of data to unearth actionable insights. By harnessing the power of analytics, organizations can transition from reactive to proactive stances, anticipating market shifts and customer needs with greater precision.
Analytics are not just about collecting data; they're about making sense of it to drive informed decisions. For instance, AI-powered tools like task assignment, chatbots, ticket routing, and predictive issue resolution enhance efficiency, customer support, and productivity by leveraging AI algorithms for better resource allocation and problem-solving.
Imagine the possibilities when decisions are not just based on hunches, but backed by solid data. The time to act is now—embrace data analytics and let it be the driving force behind your strategic business decisions.
To effectively guide decision-making with analytics, consider the following steps:
- Assess current data capabilities and identify high-impact areas.
- Invest in necessary tools and training for data analytics.
- Commit to a culture that embraces data-driven decision-making.
- Continuously analyze and refine strategies based on data insights.
Differentiating Business Insights from Predictive Analytics
While both business intelligence (BI) and advanced analytics provide valuable insights, they serve different purposes in the decision-making landscape. BI focuses on descriptive analytics, offering a snapshot of the past and present, whereas advanced analytics leans towards predictive and prescriptive models, shaping future strategies.
Advanced analytics harnesses historical data and machine learning to forecast future trends and prescribe actions, enabling businesses to anticipate market dynamics and optimize operations. In contrast, BI tools are often employed to understand current subscriber counts or popular content, reflecting the state of affairs rather than predicting or influencing future outcomes.
The distinction between BI and advanced analytics is pivotal for companies aiming to evolve from reactive to proactive stances in their strategic planning.
Understanding these differences is crucial for organizations to effectively allocate resources and tools for their specific needs. Here's a brief comparison:
- BI: Descriptive analytics, current/historic data analysis, reporting
- Advanced Analytics: Predictive modeling, prescriptive recommendations, future-oriented
Distinguishing Business Intelligence from Advanced Analytics
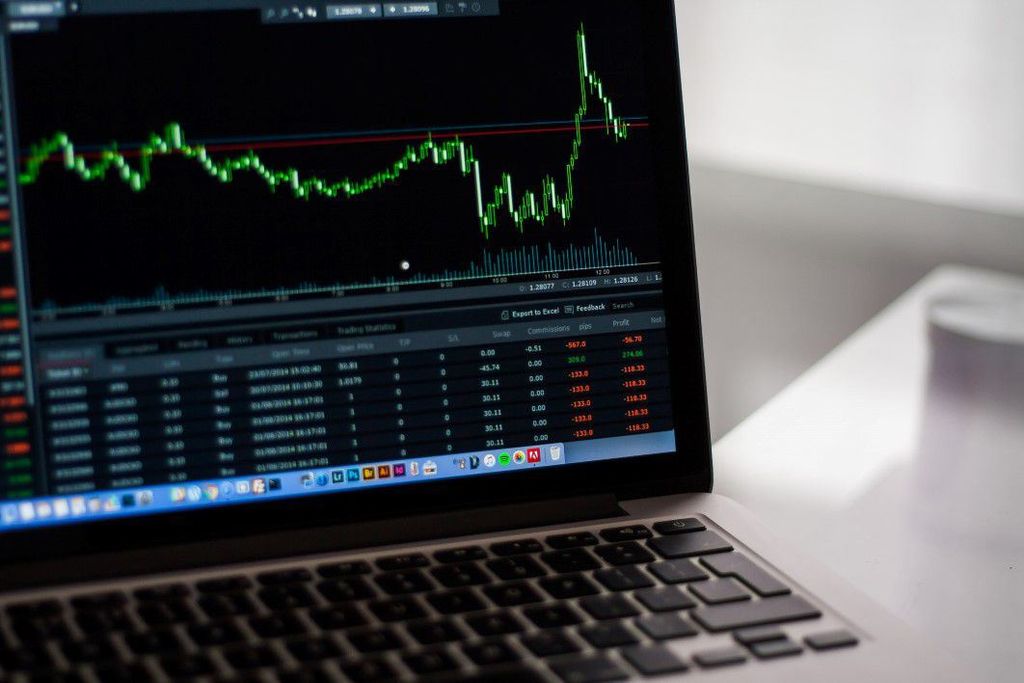
Key Differences and Use Cases
While both Business Intelligence (BI) and advanced analytics aim to enhance understanding and decision-making, they differ significantly in their approaches and applications. BI focuses on descriptive analytics, providing a snapshot of the past and present through techniques like data visualization and reporting. In contrast, advanced analytics goes beyond, employing predictive models and sophisticated algorithms to forecast future trends and optimize strategic decisions.
- Business Intelligence (BI): Descriptive analytics, historical data analysis, reporting, data mining.
- Advanced Analytics: Predictive analytics, forecasting, bias reduction, market dynamics anticipation.
Advanced analytics enables businesses to not only interpret past data but also to proactively shape their future strategies.
Understanding these differences is crucial for organizations to effectively allocate resources and choose the right tools for their specific needs. While BI might inform you about the current number of subscribers on a streaming platform, advanced analytics could predict subscriber behavior and content popularity, guiding the creation of future content to align with anticipated trends.
Traditional BI vs. Advanced Analytics
While both traditional Business Intelligence (BI) and advanced analytics serve to extract insights from data, they diverge significantly in their approaches and capabilities. Traditional BI focuses on descriptive analytics, providing a historical view of business performance through data visualization and reporting. In contrast, advanced analytics employs predictive models and machine learning to anticipate future trends and inform strategic decisions.
- Traditional BI: Descriptive, historical data analysis
- Advanced Analytics: Predictive, future-oriented insights
Advanced analytics goes beyond the rear-view mirror approach of BI, offering a forward-looking perspective that can drive transformative business strategies.
Choosing the right approach depends on the specific needs of the business. While BI might suffice for understanding past performance, advanced analytics is essential for those looking to gain a competitive edge through predictive insights and data-driven foresight.
Emerging Market Trends Identification
In the rapidly evolving business landscape, identifying emerging market trends is crucial for maintaining a competitive edge. Advanced analytics tools, particularly those leveraging AI, are instrumental in pinpointing trends that have the potential to disrupt industries. By scanning billions of web pages, AI-driven analytics can identify trends before they peak, providing businesses with the foresight to make strategic decisions.
To effectively harness these insights, companies must focus on the quality and objectivity of the data they analyze. Large sample sizes, such as those derived from Google Search data, ensure a more accurate reflection of market sentiments and consumer behaviors. This approach allows for the anticipation of market shifts and the strategic alignment of business models to capitalize on new opportunities.
The ability to adapt to market trends is not just about survival; it's about positioning your company to thrive in an uncertain future.
Understanding and acting upon these trends requires a structured approach. Below is a list of strategies that can enhance a company's trend identification process:
- Utilizing AI to scan and analyze extensive online data
- Focusing on data quality and objectivity
- Leveraging large datasets for comprehensive insights
- Anticipating shifts and aligning business strategies accordingly
- Continuously monitoring and adapting to the market
Conclusion
In conclusion, the integration of advanced analytics into strategic decision-making processes is paramount for modern businesses aiming to thrive in a data-driven landscape. By leveraging predictive analytics, uncovering hidden patterns, and embracing data-driven insights, organizations can enhance their agility, mitigate risks, and capitalize on emerging opportunities. The power of data-driven decision-making cannot be understated, as it enables businesses to adapt swiftly, innovate strategically, and maintain a competitive edge. Embracing advanced analytics is not just a choice but a necessity for organizations looking to unlock their true potential and drive transformational growth in the digital age.
Frequently Asked Questions
What are some examples of advanced analytical techniques used for data-driven decision making?
Some examples of advanced analytical techniques include predictive analytics, data-driven decision making, and strategic business intelligence.
How can AI and ML be integrated into Financial Planning & Analysis (FP&A) for strategic decision-making?
AI and ML can be integrated into FP&A for strategic decision-making by decoding possibilities with data-driven analytics and overcoming challenges in AI/ML implementation.
What are the key challenges organizations face in implementing data-driven transformation using advanced analytics?
Organizations face challenges in cultivating a data-driven culture, overcoming obstacles in data-driven transformation, and navigating the future of intelligent decision making.
How can businesses invest in scalable analytics solutions to support growth?
Businesses can invest in scalable analytics solutions by developing growth strategies, fostering cross-departmental synergies for innovation, and optimizing performance benchmarking and cost management.
What is the significance of isolating cost drivers and quantifying their impact for resource allocation using advanced analytics?
Isolating cost drivers and quantifying their impact with advanced analytics enhances decision-making precision, supports strategic resource allocation, and optimization initiatives within organizations.
How do businesses utilize advanced analytics for forecasting future outcomes and decision guidance?
Businesses leverage advanced analytics for forecasting future outcomes, guiding decision-making with analytics, and differentiating business insights from predictive analytics.
What are the key differences and use cases between business intelligence and advanced analytics?
Business intelligence and advanced analytics have key differences and use cases. While traditional BI focuses on common data analytics techniques, advanced analytics emphasize forecasting future outcomes, reducing bias in decision-making, and identifying emerging market trends.
How can businesses reduce bias in decision-making processes through advanced analytics?
Businesses can reduce bias in decision-making processes by leveraging advanced analytics techniques such as predictive modeling for strategic decisions, reducing cognitive biases, and analyzing market dynamics to make more informed choices.