The Rise of AI-Driven Predictive Analytics
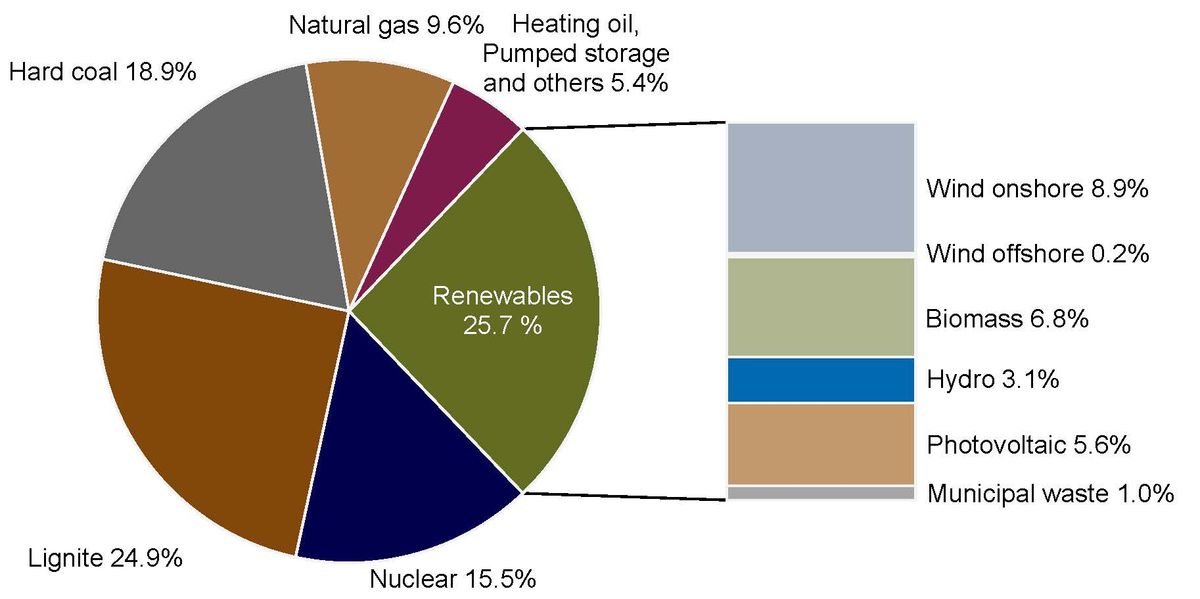
Integrating Machine Learning for Enhanced Forecasting
The integration of machine learning (ML) into predictive analytics marks a significant leap towards intelligent automation. Machine learning algorithms excel at processing vast amounts of data, identifying complex patterns and trends that might elude traditional analysis. This capability is pivotal for businesses aiming to enhance their forecasting accuracy and agility.
By automating analytical workflows, machine learning not only boosts efficiency but also uncovers insights that drive smarter decision-making. The symbiotic relationship between predictive analytics and machine learning is particularly evident in areas like supply chain management, where real-time data and predictive insights are crucial for maintaining agility.
The strategic incorporation of machine learning into business intelligence (BI) processes allows for the creation of sophisticated predictive models. These models are instrumental in anticipating market dynamics and enabling businesses to proactively adjust their strategies.
- Key Benefits of Machine Learning Integration:
- Automation of complex data analysis tasks
- Enhanced prediction accuracy
- Real-time analytics and streaming data capabilities
- Proactive identification of potential production challenges
The Impact of AI on Predictive Modeling
The integration of artificial intelligence (AI) into predictive modeling marks a transformative leap in how businesses forecast future trends and behaviors. AI algorithms enhance predictive analytics by automating data analysis, identifying complex patterns, and enabling real-time predictions, which are essential for dynamic decision-making.
However, the sophistication of AI models introduces challenges in interpretability and data privacy. Ensuring the ethical use of AI and mitigating biases are critical to maintaining trust in predictive insights.
The synergy between AI and predictive analytics is reshaping data-driven strategies, but it is imperative to navigate the complexities associated with advanced AI techniques to fully leverage their potential.
The benefits of AI in predictive modeling are clear, yet the path to integration is dotted with hurdles that require careful consideration:
- Ensuring the accuracy and interpretability of complex AI models
- Safeguarding data privacy and security
- Addressing ethical concerns and avoiding algorithmic biases
As AI continues to evolve, so too must the strategies for its implementation in predictive analytics, ensuring that the future of business decision-making is both intelligent and responsible.
Navigating the Ethical Implications of AI in Analytics
The integration of AI into analytics has brought about transformative changes in how businesses operate. AI consulting focuses on transforming business models with predictive analytics, RPA, and cognitive computing. Ethical AI practices are crucial for innovation and societal norms, and AI as a service adoption is increasing. However, the ethical landscape is complex, with concerns around the accuracy and interpretability of AI algorithms, data privacy, and the potential for biases within predictive models.
Ensuring ethical use of AI in analytics is not just about compliance with regulations; it's about fostering trust and fairness in the systems we build and the decisions they inform.
To address these challenges, a multi-faceted approach is necessary:
- Privacy and Consent: Transparent and legal data handling with explicit consent.
- Bias and Discrimination: Mitigating risks of bias that may lead to unfair practices.
- Accountability and Responsibility: Clear accountability for data processing and outcomes.
- Fairness and Equity: Equitable distribution of benefits and risks, avoiding social inequalities.
- Occupational Impact: Considering the job market impact and promoting equal employment opportunities.
Advancements in Data Processing Technologies
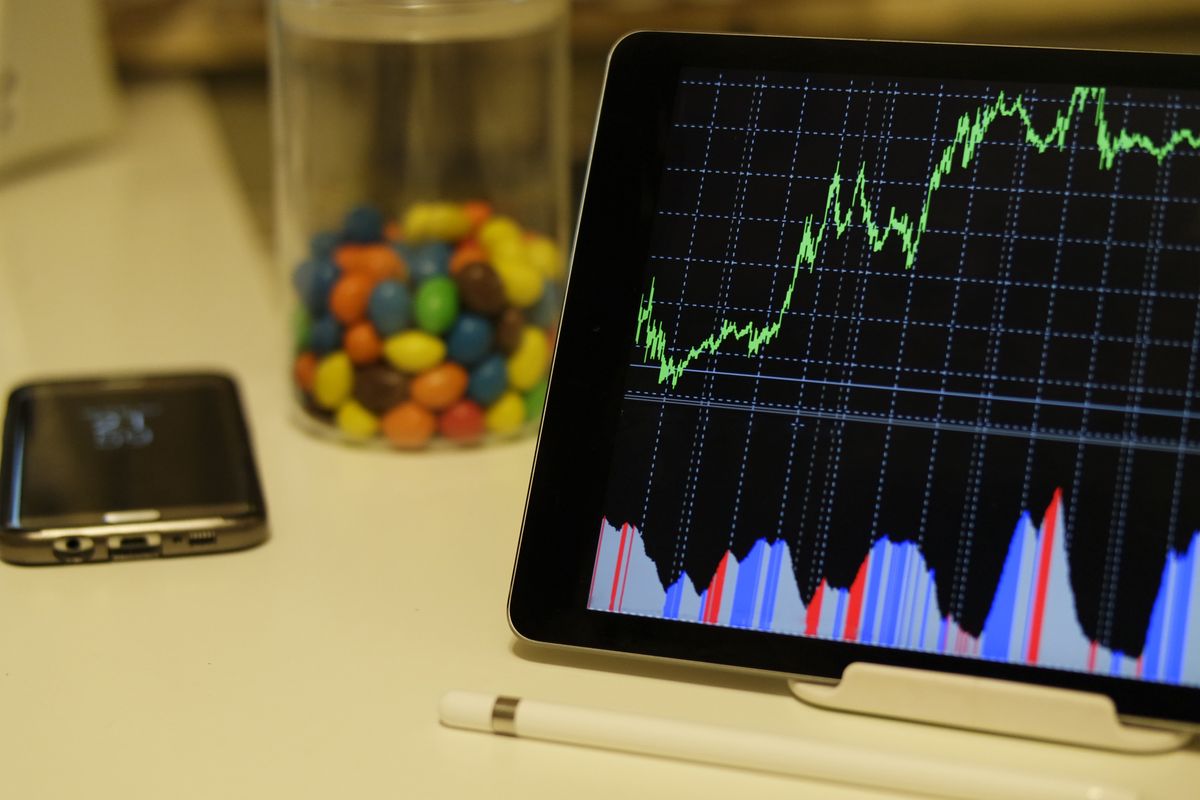
The Role of Big Data in Predictive Insights
The advent of Big Data has been a game-changer for predictive analytics, providing an unprecedented volume of information for analysis. Big Data is a complex of technologies that collect and manage these vast datasets, which are continuously growing in size and complexity. By leveraging Big Data, organizations can uncover patterns and trends that were previously indiscernible, leading to more accurate and insightful predictions.
The integration of Big Data with predictive analytics enables businesses to process and analyze large volumes of data in real-time. This capability is crucial for making timely decisions that can significantly impact business outcomes. For instance, in the retail sector, Big Data can help predict consumer buying patterns, allowing for better stock management and targeted marketing campaigns.
The synergy between Big Data and predictive analytics is transforming how businesses operate, driving efficiency and innovation across various industries.
While the potential of Big Data is immense, it also presents challenges such as data privacy, storage, and analysis. Addressing these challenges is essential for businesses to fully capitalize on the benefits of predictive insights.
Cloud Computing's Contribution to Analytics
The advent of cloud computing has been a game-changer for the field of analytics. Cloud computing and data analytics empower businesses to innovate and transform, leveraging big data for competitive advantage and informed decision-making. The scalability and flexibility offered by the cloud allow for the efficient handling of vast amounts of data, which is essential for predictive analytics.
Cloud-based predictive analytics platforms facilitate faster model development, deployment, and iteration, enabling organizations to respond swiftly to changing business conditions. This agility is crucial in today's fast-paced market environment, where the ability to quickly adapt and capitalize on emerging trends can be the difference between success and failure.
Predictive analytics in the cloud requires addressing issues of data quality, integration, and security to ensure accurate and reliable results. These challenges are not insurmountable, but they do require careful planning and strategic implementation.
The following list highlights some key benefits of cloud computing in analytics:
- Scalability to manage growing data volumes
- Cost-efficiency through pay-as-you-go models
- Accessibility for remote teams and collaboration
- Enhanced security features and compliance protocols
- Real-time data processing capabilities
Real-Time Data Processing and Its Effects on Business Strategy
The advent of real-time data processing has been a game-changer for businesses across the globe. By analyzing data as it is generated, companies can respond with unprecedented speed to market changes, ensuring that their strategies are always one step ahead. This agility is not just about keeping pace; it's about setting the pace in a competitive landscape.
Real-time analytics serves as a strategic tool, enabling organizations to seize emerging opportunities and mitigate risks promptly. The ability to make on-the-fly adjustments to business strategies, based on current market dynamics, is a powerful asset in today's fast-paced environment.
- Real-Time Analytics and Streaming Data
- Self-Service BI and Data Democratization
Predictive analytics drives revenue growth by forecasting trends, personalizing experiences, optimizing resources, and enhancing efficiency. Real-time data processing revolutionizes operations for swift decision-making and competitive advantage.
Predictive Analytics in Market Positioning
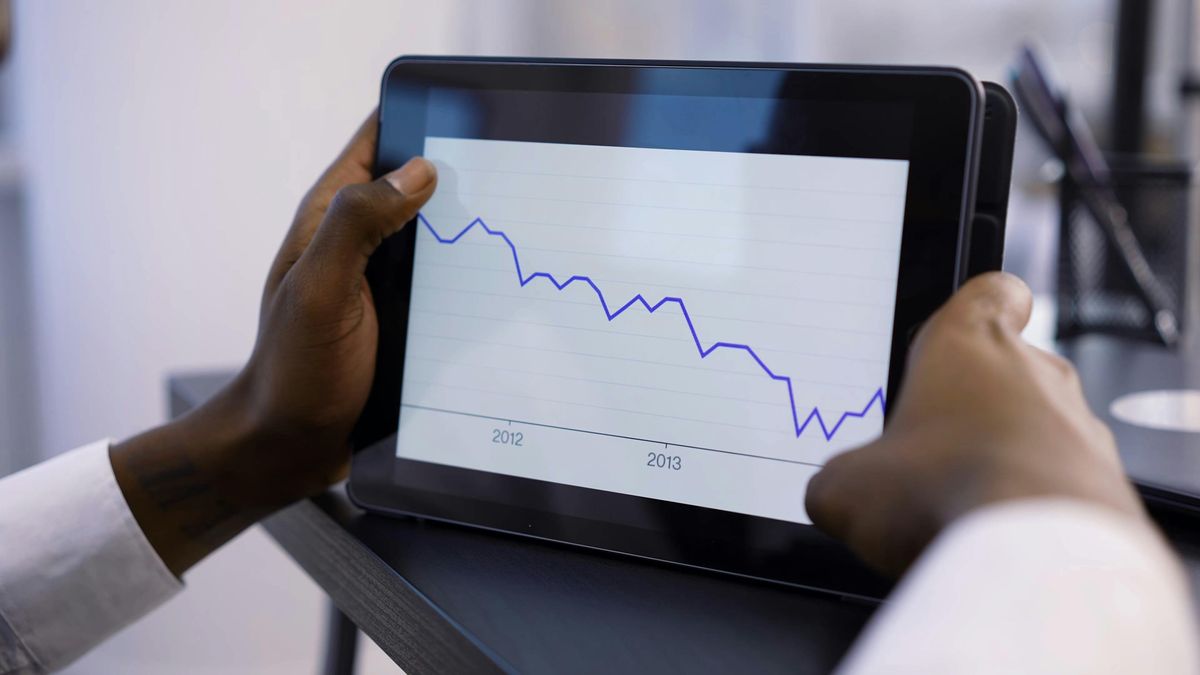
Anticipating Consumer Behavior Shifts
The ability to anticipate consumer behavior shifts is a cornerstone of modern business strategy. Utilizing predictive analytics, companies can now forecast market trends with greater accuracy, ensuring they stay ahead of the curve. This approach not only enhances decision-making but also allows for the customization of marketing campaigns to meet the evolving demands of consumers.
Predictive analytics promotes customer-centric decisions, empowering businesses to tailor their offerings. By analyzing customer browsing and purchase histories, companies can improve the shopping experience, leading to increased conversion rates and customer satisfaction. Moreover, this data-driven strategy aids in risk mitigation, preparing businesses for potential market fluctuations.
Embracing the power of predictive analytics means transforming data into actionable insights. It's about making informed, proactive decisions that align with consumer expectations and market dynamics.
Incorporating artificial intelligence in data science has further refined the predictive capabilities of businesses. The integration of AI allows for the processing of vast amounts of data from diverse sources, including IoT devices and social media. This leads to deeper customer insights and the ability to deliver targeted, personalized experiences that resonate with the market.
Strategic Decision-Making Based on Predictive Data
In the realm of business intelligence, the integration of predictive analytics has become a cornerstone for strategic decision-making. Data analytics integration drives strategic decision-making, operational efficiency, and predictive modeling for future trends, enhancing business productivity and profitability. By leveraging historical data, companies can forecast future outcomes, much like The Lloyd Company did in 1689 to estimate the risks of new sea voyages.
Incorporating artificial intelligence in data science has further empowered businesses to make informed decisions. These range from customer segmentation to optimizing manufacturing processes, all underpinned by the critical data analyzed by data scientists. As a result, organizations that embrace a data-driven culture can stay ahead of the competition, identify emerging opportunities, and make strategic decisions with greater accuracy.
Predictive analytics not only anticipates market dynamics and customer behavior but also flags potential challenges in production, enabling proactive problem-solving and strategic adjustments.
Considering the significant impact of predictive analytics, it is imperative for businesses to invest in data science capabilities. This investment will facilitate more accurate predictions and foster a culture that is both data-driven and forward-looking.
Case Studies: Successful Market Positioning Through Analytics
The strategic application of predictive analytics has proven to be a game-changer for businesses aiming to secure a competitive edge in their respective markets. By leveraging data-driven insights, companies can anticipate market trends and consumer needs with remarkable accuracy. This foresight enables them to tailor their products and services, ensuring they resonate with their target audience.
Case studies across various industries have highlighted the transformative power of predictive analytics in market positioning. For instance, a study by McKinsey & Company revealed that a comprehensive analytics approach could lead to substantial cost savings and efficiency gains. Here's a snapshot of the potential impact:
Sector | Potential Savings |
---|---|
Marketing Expenditure | Up to $200 billion globally |
The ability to customize offerings and align them with customer expectations not only enhances satisfaction but also fosters brand loyalty.
The insights gleaned from predictive analytics are not just about understanding current consumer behavior but also about predicting shifts and preparing for them proactively. This strategic foresight is invaluable for businesses looking to maintain and improve their market position in a rapidly evolving landscape.
Innovative Applications of Predictive Analytics
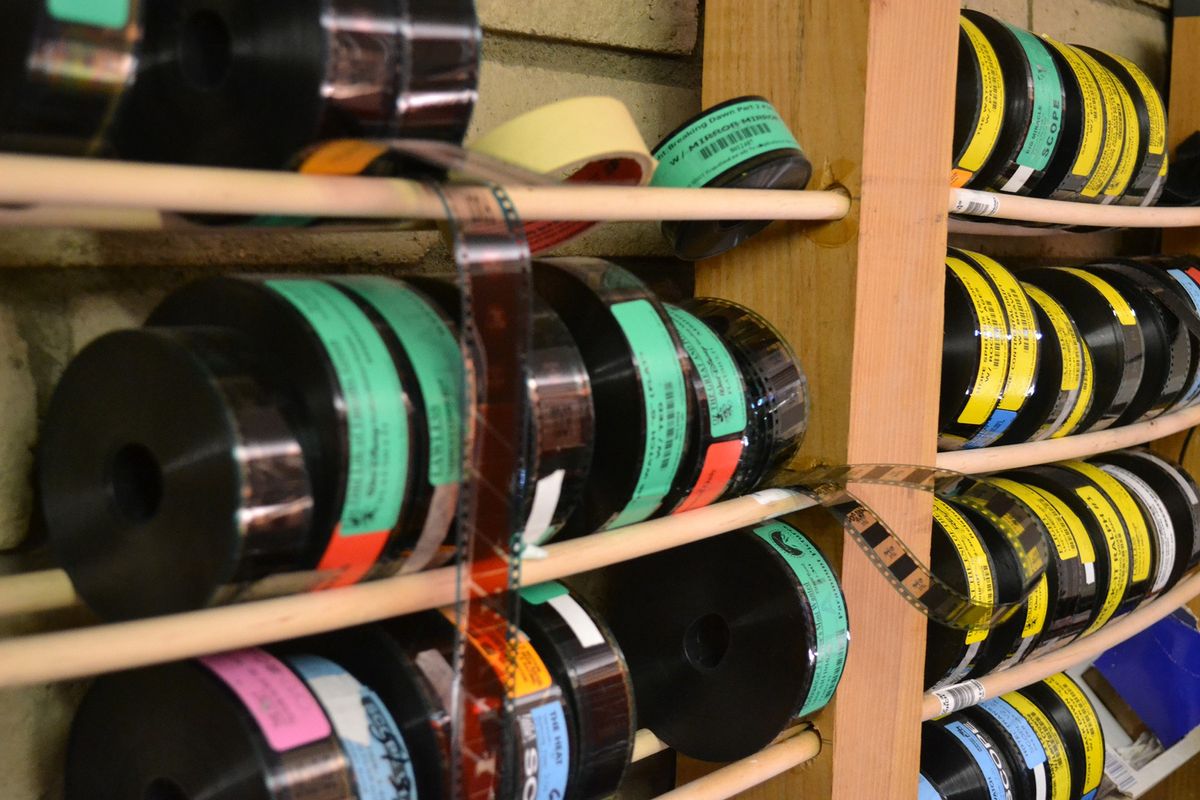
Revolutionizing Healthcare with Predictive Diagnostics
The integration of predictive analytics and AI is transforming the landscape of healthcare, offering unprecedented precision in diagnostics and patient care management. By harnessing vast amounts of patient data, including medical records and genetic information, healthcare providers can now anticipate diseases, tailor treatments to individual genetic profiles, and enhance patient outcomes.
- Predictive analytics enables early detection of diseases, often before symptoms appear, allowing for timely intervention.
- Personalized treatment plans can be developed based on a patient's unique genetic makeup, improving the efficacy of medical interventions.
- Continuous monitoring of patient data helps in managing chronic conditions more effectively, reducing hospital readmissions.
The potential of predictive diagnostics in healthcare is vast, with AI-driven tools not only improving the accuracy of diagnoses but also streamlining the entire healthcare delivery process.
The impact of these advancements is not limited to patient care alone; it also extends to accelerating medical research and augmenting surgical procedures. As we continue to develop and refine these technologies, the promise of a more efficient and personalized healthcare system becomes increasingly tangible.
Optimizing Supply Chain Management
In the realm of supply chain management, predictive analytics is a game-changer. By leveraging historical and real-time data, businesses can anticipate demand fluctuations and optimize inventory levels. This not only ensures timely deliveries but also minimizes stockouts and excess inventory, leading to a more efficient and cost-effective supply chain.
Inventory management is a critical component where predictive analytics can make a significant impact. Analyzing order frequencies and product demand patterns allows companies to make informed decisions about stock levels and reorder points. Here's how predictive analytics transforms inventory management:
- Demand Forecasting: Accurate predictions of product demand to avoid overstocking or understocking.
- Reorder Point Calculation: Data-driven determination of when to reorder products.
- Supplier Performance Analysis: Evaluation of supplier reliability and lead times to improve procurement strategies.
Predictive analytics empowers businesses with data-driven decision-making, leading to enhanced efficiency, reduced errors, and strategic foresight for competitive advantage. Success stories showcase transformative impact and operational efficiency.
Furthermore, predictive analytics facilitates sustainable scaling of services by identifying expansion opportunities and optimizing resource allocation. The end result is a streamlined supply chain that not only reduces costs but also bolsters the company's competitiveness in the market.
Personalization in Retail Through Predictive Trends
The retail industry is witnessing a transformative shift towards hyper-personalization, driven by predictive analytics solutions. By leveraging customer data, retailers are able to tailor experiences to individual preferences, leading to increased customer loyalty and sales. For instance, analyzing browsing and purchase history allows for more accurate product recommendations and dynamic pricing strategies, directly impacting the conversion rates.
Predictive analytics not only enhances the shopping experience but also promotes customer-centric decisions, ensuring that each interaction is relevant and engaging.
The table below highlights key applications of predictive analytics in retail:
Application | Description |
---|---|
Marketing Campaigns | Personalized promotions based on customer data |
Product Recommendations | Tailored suggestions using browsing and purchase history |
Inventory Management | Optimized stock levels through demand forecasting |
Pricing Strategies | Dynamic pricing based on demand and customer behavior |
Risk Mitigation | Identifying potential issues before they arise |
Embracing the power of predictive analytics allows retailers to stay ahead in a competitive market by anticipating consumer needs and delivering exceptional experiences.
Challenges and Solutions in Predictive Analytics
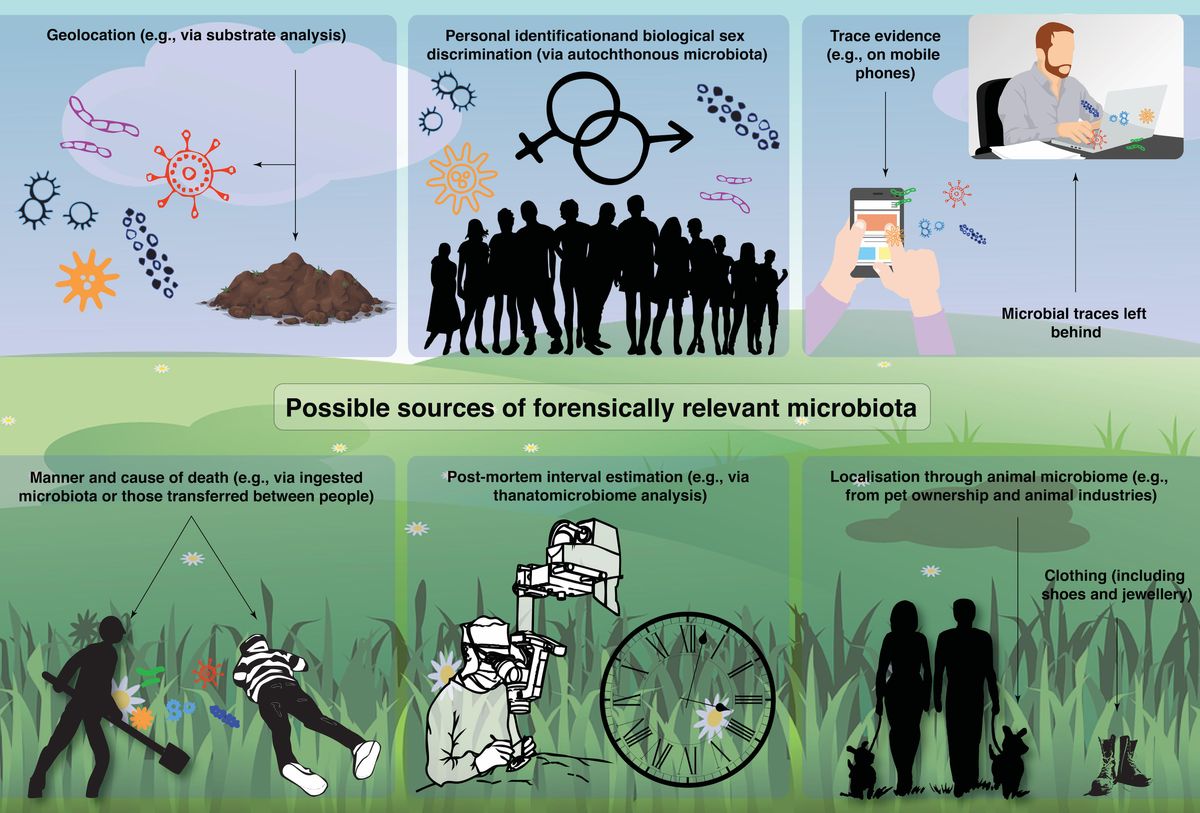
Addressing Data Privacy and Security Concerns
In the realm of business analytics, data privacy and security are of utmost importance. As companies increasingly rely on predictive analytics, the need to protect sensitive information from breaches and unauthorized access has never been greater. Encryption, advanced security features, and adherence to regulations like GDPR and CCPA are essential in maintaining trust and legal compliance.
Data breaches have underscored the urgency for robust security measures. Organizations must navigate a landscape filled with potential risks, including bias, discrimination, and misuse of data. To address these concerns, a multi-faceted approach is necessary:
- Privacy and Consent: Transparent and legal data handling with explicit consent.
- Bias and Discrimination: Mitigating risks in AI algorithms to prevent unfair practices.
- Accountability and Responsibility: Clear accountability to prevent data misuse.
- Security and Data Protection: Strong measures against unauthorized access.
- Algorithmic Transparency: Openness in AI decision-making processes.
Ensuring the ethical use of predictive analytics is not just about technology; it's about fostering a culture of responsibility and trust. Regulatory compliance and data governance play pivotal roles in shaping this environment.
Overcoming Data Quality and Integration Hurdles
Ensuring the accuracy and completeness of data is paramount in predictive analytics. Inaccurate or incomplete data can severely hinder the effectiveness of analytics efforts, necessitating robust data cleansing and validation processes. To address these challenges, organizations must focus on several key areas:
- Human errors: Minimizing manual data entry and implementing automated checks can reduce errors significantly.
- Lack of standardization: Establishing company-wide data standards ensures consistency across different datasets.
- Data integration complexities: Streamlining integration processes simplifies the consolidation of data from various sources.
- Legacy systems: Upgrading or integrating legacy systems can prevent them from becoming bottlenecks.
- Incomplete data: Employing strategies to fill data gaps ensures that datasets are comprehensive.
- Poor data governance: Developing a strong governance framework is essential for maintaining data quality over time.
The journey to high-quality data is continuous and requires a strategic approach to overcome the hurdles of data quality and integration. By prioritizing these areas, businesses can unlock the full potential of their analytics capabilities.
Data privacy and security are also critical considerations. As organizations transform data, they must comply with privacy regulations and protect sensitive information. This often involves a resource-intensive process, demanding significant computing power and skilled expertise to ensure that data remains secure throughout its lifecycle.
The Future of Regulatory Compliance in Analytics
As the landscape of analytics evolves, regulatory compliance becomes a critical pivot around which businesses must maneuver. The future of analytics is inextricably linked to the ability to adhere to evolving regulations without stifling innovation.
The harmonization of regulatory frameworks across jurisdictions is essential to facilitate seamless global operations and maintain the integrity of data analytics practices.
To stay ahead, organizations are investing in compliance analytics—a blend of technology and processes designed to ensure adherence to regulatory requirements. Here's a snapshot of key compliance analytics components:
- Risk Identification: Pinpointing areas of potential non-compliance.
- Data Management: Establishing robust governance to maintain data quality and lineage.
- Monitoring and Reporting: Implementing systems for continuous oversight and timely reporting.
- Remediation Processes: Developing protocols for addressing compliance breaches.
In the coming years, we can expect a surge in the use of advanced analytics to predict regulatory changes and assess compliance risks, enabling businesses to be proactive rather than reactive.
The Intersection of Predictive Analytics and IoT
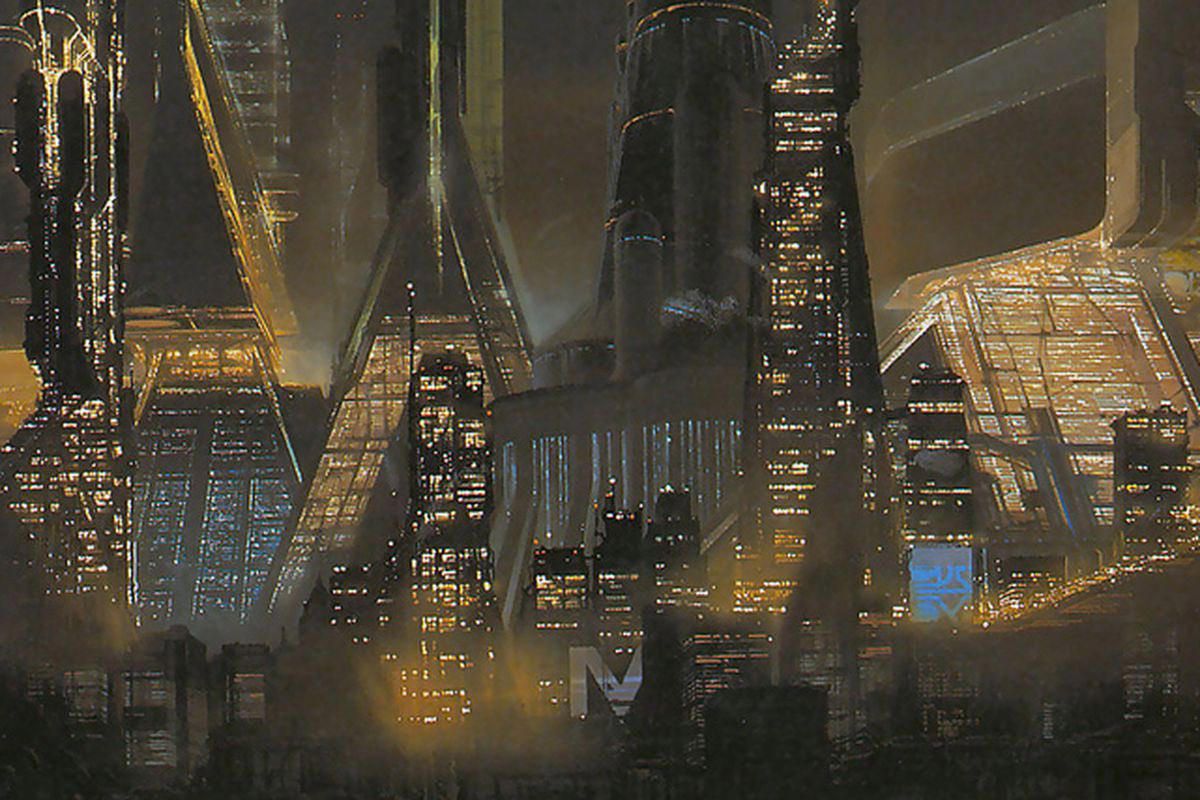
Enhancing Operational Efficiency with IoT Data
The integration of Internet of Things (IoT) data with predictive analytics is revolutionizing how businesses approach operational efficiency. Predictive analytics harnesses IoT data to forecast potential issues and optimize processes before problems arise. This proactive stance is critical in maintaining a competitive edge in a technology-driven marketplace.
- Improved Operational Efficiency: By analyzing historical data and identifying patterns, businesses can optimize inventory levels, anticipate demand fluctuations, and streamline supply chain processes.
- Promotes Customer-Centric Decisions: Leveraging IoT data helps in understanding customer behavior, leading to more informed decisions and enhanced product quality.
- Cost Reduction: Predictive analytics aids in identifying and preventing costly issues, thereby improving resource allocation and reducing waste.
Predictive analytics and AI are not just about data processing; they represent a strategic asset that can lead to significant improvements in operational efficiency and productivity.
The transformative opportunities provided by AI and IoT integration are pivotal for businesses aiming to enhance operations, customer management, and productivity. As digital adoption drives innovation, companies that leverage these technologies effectively can expect to see substantial gains in efficiency and market positioning.
Predictive Maintenance: Reducing Downtime and Costs
Predictive maintenance represents a transformative shift in how businesses approach equipment upkeep. By harnessing the power of IoT data, companies can anticipate potential failures before they occur. This proactive strategy is not only about preventing breakdowns but also about optimizing the maintenance schedule to enhance efficiency and extend the lifespan of machinery.
Predictive analytics is pivotal in minimizing operational disruptions and maintaining a competitive edge. Industries such as manufacturing and transportation are already reaping the benefits, as they can schedule maintenance interventions at the most opportune moments, thereby curtailing downtime and operational costs.
The integration of predictive maintenance within industrial operations signifies a move towards a more data-driven and strategic approach to asset management.
Here's how predictive maintenance impacts key areas:
- Reduces unexpected equipment failures: By analyzing historical and real-time sensor data, maintenance can be planned before issues escalate.
- Optimizes maintenance resources: Allocates personnel and resources more effectively, avoiding unnecessary checks.
- Enhances equipment longevity: Regular, data-informed maintenance can significantly prolong the life of machinery.
- Improves safety: Prevents accidents and ensures a safer working environment by maintaining equipment in optimal condition.
IoT-Driven Predictive Analytics in Smart Cities
The integration of IoT with predictive analytics is transforming urban landscapes into smart cities. Predictive insights derived from IoT data are crucial for urban planning and the management of city resources. These insights enable city officials to make informed decisions that enhance the quality of life for residents.
- Energy Management: Optimization of energy consumption in public spaces and residential areas.
- Traffic Flow Optimization: Reduction of congestion and improvement of commute times through predictive traffic management.
- Waste Management: Efficient waste collection routes and schedules based on predictive data analysis.
- Public Safety: Anticipation of potential safety threats and deployment of emergency services effectively.
By harnessing the power of IoT-driven analytics, cities can proactively address urban challenges, leading to more sustainable and efficient urban environments.
The potential of IoT in smart cities extends beyond these applications. It encompasses areas such as video analytics for security, health condition monitoring in public spaces, and predictive maintenance of urban infrastructure. The goal is to create a cohesive ecosystem where data not only informs but also anticipates the needs of the city.
The Role of Predictive Analytics in Financial Services
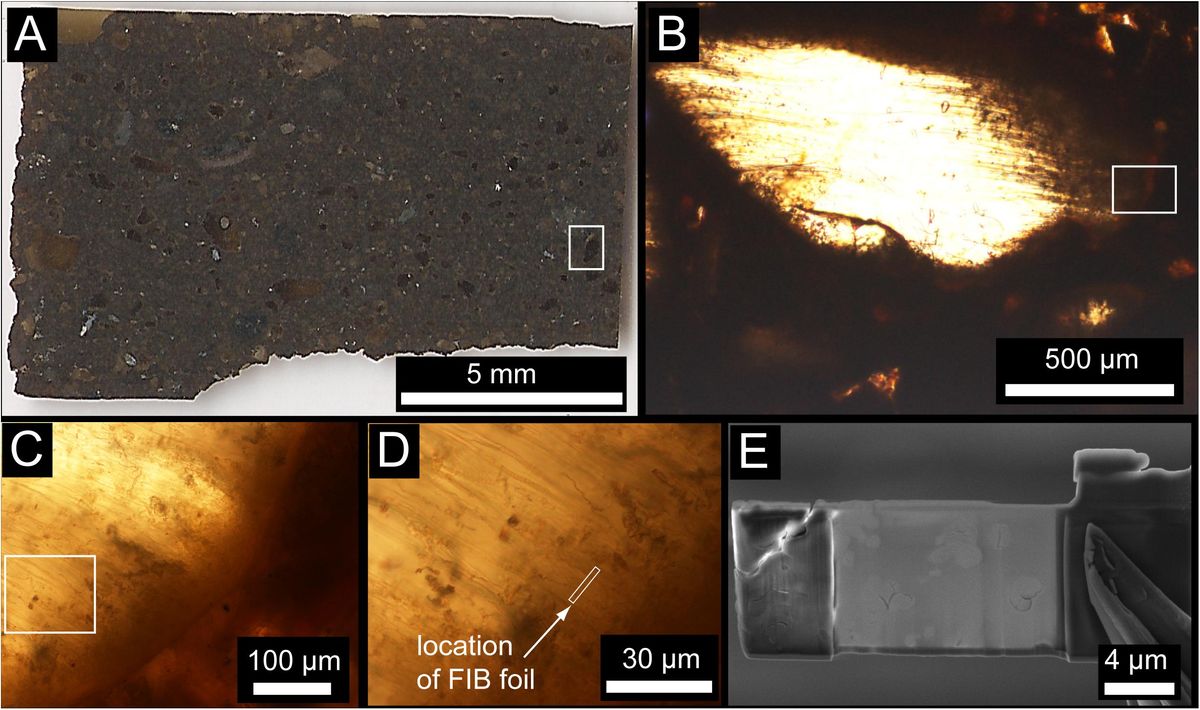
Risk Assessment and Credit Scoring Innovations
The integration of predictive analytics in the financial sector has revolutionized risk assessment and credit scoring. By harnessing historical data, financial institutions can now more accurately predict creditworthiness and manage risks associated with lending. This precision in credit scoring has led to more tailored financial products and services, benefiting both consumers and lenders.
Fraud detection has become a critical application of predictive analytics in finance. Anomalous patterns in transaction data are identified swiftly, enabling real-time responses to potential fraud. This proactive approach not only safeguards against financial losses but also enhances consumer trust.
The predictive analytics landscape in financial services is rapidly evolving, with continuous innovations leading to more robust risk management strategies and customer-centric solutions.
The following list highlights key benefits of predictive analytics in risk assessment and credit scoring:
- Improved accuracy in identifying credit risks
- Enhanced ability to forecast financial solvency
- Reduction in the incidence of fraudulent activities
- Customization of credit products to individual risk profiles
- Streamlined regulatory compliance through predictive models
Fraud Detection and Prevention Strategies
In the realm of financial services, predictive analytics plays a pivotal role in identifying and thwarting fraudulent activities. By sifting through vast datasets, these systems can pinpoint irregular patterns that may indicate fraudulent behavior, thereby safeguarding both consumers and institutions.
Fraud detection strategies have evolved significantly with the advent of AI, allowing for real-time analysis and immediate action. For instance, outlier models consider variables such as location, time, and transaction amount to assess the likelihood of fraud. A transaction in a user's hometown may be deemed less suspicious than one occurring in a foreign country, illustrating the nuanced capabilities of modern systems.
The integration of predictive analytics into fraud prevention mechanisms has not only enhanced security but also streamlined financial operations, ensuring a robust defense against illicit activities.
The following list outlines key components of a fraud detection system powered by predictive analytics:
- Anomaly detection to identify unusual patterns
- Real-time transaction monitoring for immediate alerts
- Advanced algorithms for assessing risk levels
- Integration with existing security protocols to bolster defenses
- Continuous learning models that adapt to new fraudulent tactics
Investment Forecasting and Wealth Management
The integration of predictive analytics into investment forecasting and wealth management has revolutionized the way financial advisors and investors make decisions. By leveraging historical data, forecast models predict future market trends and asset performance, enabling a more strategic allocation of resources. This data-driven approach not only enhances the accuracy of investment forecasts but also provides personalized financial recommendations.
The role of predictive analytics in wealth management extends beyond mere prediction. It encompasses risk assessment, portfolio optimization, and the anticipation of market shifts. Financial institutions that adopt these advanced analytics techniques gain a competitive edge through:
- Improved accuracy in predicting asset performance
- Enhanced customer experience with tailored investment strategies
- Increased regulatory compliance and risk mitigation
The predictive power of analytics is reshaping the financial landscape, offering a clearer vision for future investments and wealth preservation.
As the financial sector continues to evolve, the importance of integrating predictive analytics into investment strategies becomes increasingly clear. It is not just about forecasting; it's about creating a robust framework for financial growth and stability.
Emerging Trends in Predictive Analytics
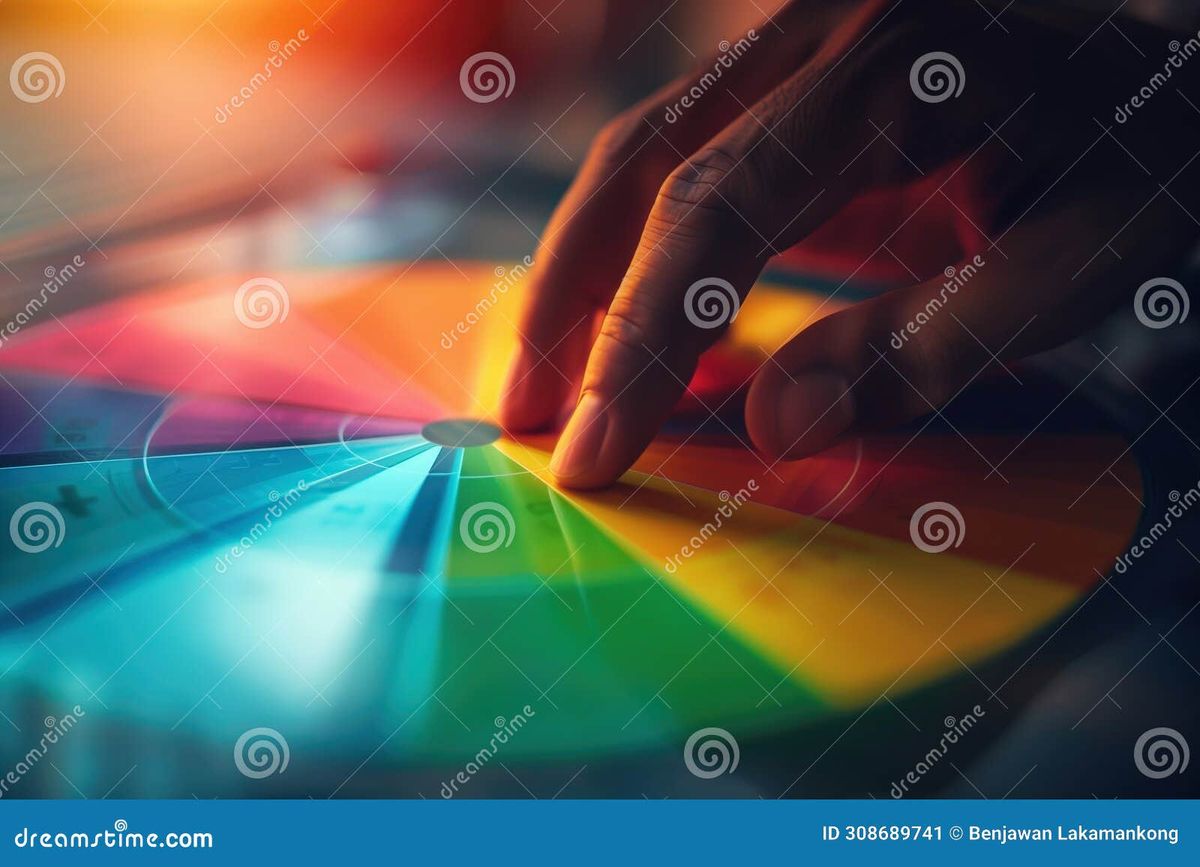
The Growing Importance of Prescriptive Analytics
As the landscape of data analytics evolves, the shift from predictive to prescriptive analytics is becoming more pronounced. Prescriptive analytics represents a significant leap forward, focusing not just on forecasting future events, but on shaping them. By analyzing potential actions and their outcomes, businesses can proactively steer results towards more advantageous scenarios.
Prescriptive analytics harnesses the power of machine learning, AI, and complex algorithms to simulate and recommend the best courses of action. This approach is transforming how organizations optimize processes and make strategic decisions. It's a step beyond traditional analytics, offering a dynamic feedback system that refines the relationship between actions and outcomes.
- Predictive Analytics: What will happen?
- Prescriptive Analytics: What should we do about it?
Prescriptive analytics empowers organizations to not only anticipate the future but to actively influence it, ensuring they remain agile and competitive in a rapidly changing environment.
Predictive Analytics in the Era of 5G Connectivity
The advent of 5G connectivity is set to revolutionize the landscape of predictive analytics. With its unprecedented speed and bandwidth, 5G will enable the real-time processing of vast amounts of data, facilitating more accurate and timely insights. This leap in connectivity is particularly significant for industries that rely on immediate data analysis, such as autonomous vehicles and smart city initiatives.
5G's impact extends beyond speed, as it also promises to enhance the reliability of predictive models. The ability to transmit and process data almost instantaneously means that businesses can make more informed decisions faster than ever before. This is crucial in environments where every second counts, such as in financial trading or emergency response scenarios.
The synergy between 5G and predictive analytics will empower organizations to not only predict outcomes but also to act upon them in real-time, thus optimizing operations and customer experiences.
The integration of 5G with predictive analytics will also drive innovation in various sectors. Here's a glimpse of potential advancements:
- Real-time health monitoring and personalized treatment plans in healthcare.
- Enhanced inventory management and demand forecasting in retail.
- Improved traffic flow and public safety measures in smart cities.
As we embrace the era of 5G, it is imperative for businesses to prepare for the influx of data and the subsequent need for advanced analytics capabilities. Investing in data democratization and the upskilling of the workforce will be key to harnessing the full potential of this transformative technology.
The Convergence of Analytics and Blockchain Technology
The fusion of predictive analytics and blockchain technology is paving the way for a new era of data integrity and transparency. Blockchain's immutable ledger ensures that analytical data is secure and trustworthy, providing a robust foundation for predictive models. This convergence is particularly significant in sectors where data authenticity is critical.
Blockchain enables the creation of decentralized databases that are resistant to tampering, enhancing the reliability of predictive insights. Here's how this integration can transform various industries:
- Finance: Streamlining transactions and improving fraud detection
- Supply Chain: Ensuring product authenticity and traceability
- Healthcare: Protecting patient data and managing medical records
The synergy between predictive analytics and blockchain not only strengthens data security but also opens up new possibilities for innovation and efficiency.
As we witness the integration of these technologies, it's essential to consider the broader implications for business intelligence. The convergence is a key trend that aligns with the future of Business Intelligence, focusing on advanced data processing and real-time analysis, ensuring that businesses stay ahead in a competitive landscape.
The Future Workforce and Predictive Analytics
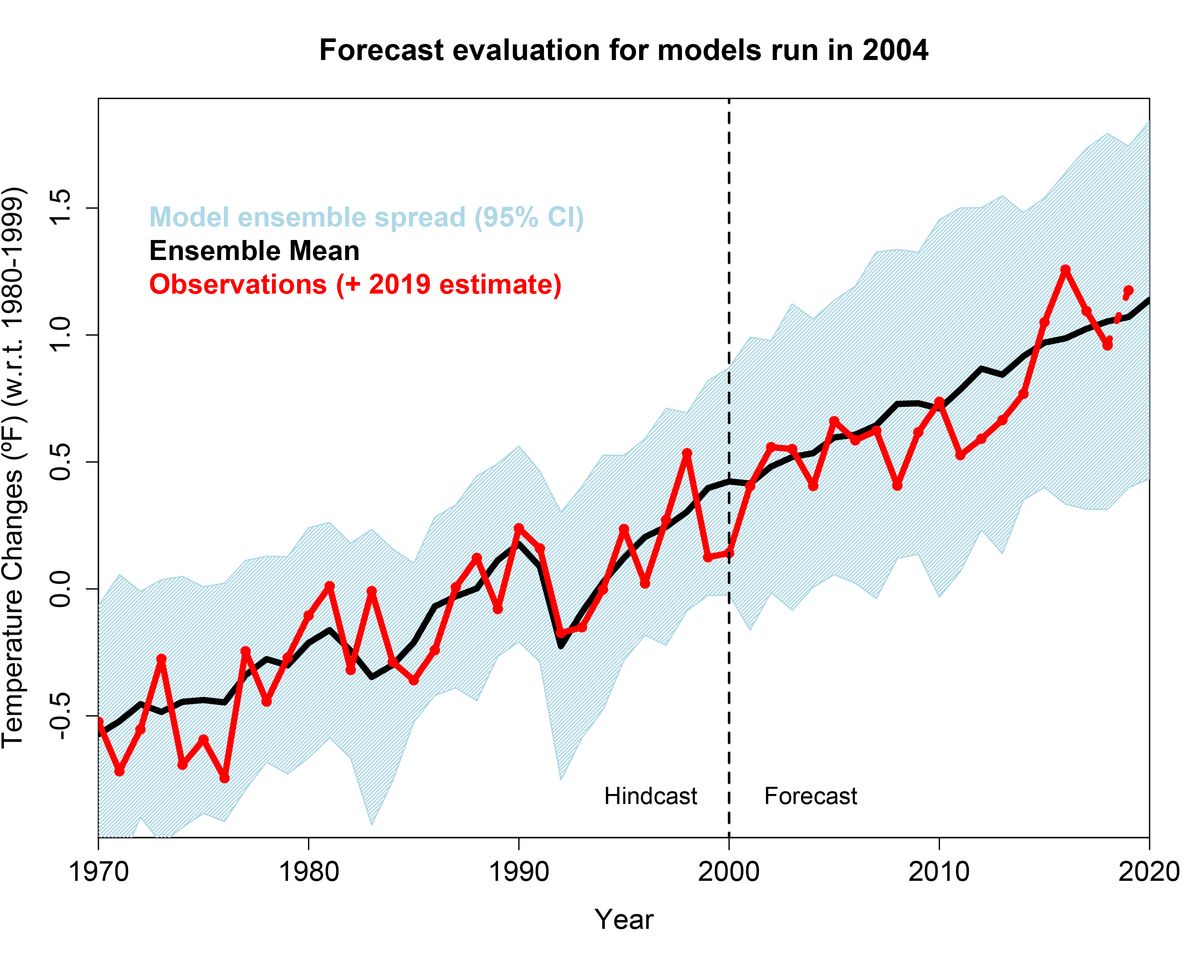
Upskilling for a Data-Driven Economy
In the data-driven era, continuous learning is not just an option; it's a necessity. As the landscape of business intelligence (BI) evolves, professionals are required to master a diverse set of skills, including data analysis, AI, and machine learning. To remain competitive, stakeholders must engage in regular, short-term training programs that keep them abreast of the latest developments in AI, data governance, and privacy.
The demand for data literacy and the ability to derive actionable insights from data is at an all-time high. Upskilling initiatives should focus on these competencies to empower careers in the rapidly changing BI landscape. Here's a list of essential skills for BI professionals in the current market:
- Proficiency in cloud technologies
- Advanced data analysis techniques
- Understanding of AI and machine learning concepts
- Data governance and monetization strategies
The future of BI is bright, but it hinges on the ability to adapt and grow with technological advancements. Organizations that invest in upskilling their workforce will not only enhance their operational capabilities but also foster a culture of innovation and resilience.
The Changing Landscape of Job Roles in Analytics
The advent of advanced analytics is not only transforming business strategies but also the job roles associated with them. The demand for data literacy is now ubiquitous across various job functions, not just within specialized data teams. As a result, roles such as data scientists and business analysts are evolving, with a growing emphasis on interdisciplinary skills and the ability to communicate insights effectively.
In response to the dynamic nature of analytics, organizations are increasingly seeking professionals who can bridge the gap between technical data analysis and strategic decision-making. This shift has led to the emergence of new roles like the analytics translator, who serves as a liaison between data teams and executive leadership, ensuring that data-driven insights are actionable and aligned with business objectives.
- Data Scientists: Deep technical expertise in machine learning and statistical analysis.
- Business Analysts: Proficiency in data interpretation and problem-solving.
- Analytics Translators: Ability to convert complex data into strategic business plans.
The future of work in analytics demands a blend of technical acumen and soft skills, preparing professionals to adapt to the ever-changing landscape of data-driven decision-making.
Predictive Analytics in Talent Acquisition and Management
The integration of predictive analytics into talent acquisition and management heralds a new era in human resources. By analyzing patterns within historical data, HR professionals can now forecast hiring needs and identify the traits of high-performing employees. This foresight enables organizations to proactively shape their workforce strategies.
Predictive analytics also plays a pivotal role in reducing turnover and enhancing employee engagement. By understanding the factors that contribute to job satisfaction and predicting potential issues, companies can implement targeted interventions to retain top talent.
- Streamlining recruitment processes
- Identifying skills gaps
- Optimizing training programs
- Predicting employee success
The strategic application of predictive analytics in HR not only refines the recruitment process but also fosters a dynamic and responsive work environment.
As the landscape of work continues to evolve, the ability to leverage data for talent management becomes increasingly critical. Companies that embrace these analytical tools are poised to build more cohesive, innovative, and successful teams.
As we navigate the evolving landscape of the future workforce, predictive analytics becomes an indispensable tool for businesses seeking to stay ahead of the curve. At OptimizDBA, we specialize in harnessing the power of data to optimize your database performance and empower your team with faster data solutions. Don't let your organization fall behind; visit our website to learn how our proprietary methodology and unparalleled experience can revolutionize your transaction speeds and elevate your operational efficiency. Take the first step towards a more agile and data-driven future by connecting with us today!
Conclusion: Harnessing Predictive Analytics for Future Success
As we have explored throughout this article, predictive analytics stands at the forefront of business intelligence, offering a crystal ball into the future of market trends, customer behavior, and potential risks. The integration of AI and machine learning into predictive models not only enhances accuracy but also revolutionizes decision-making processes, enabling businesses to operate with unprecedented foresight and agility. The future trends and opportunities in predictive analytics promise to further empower organizations, fostering innovation, competitive advantage, and growth. As the landscape of data science continues to evolve, those who embrace and invest in these advanced analytical tools will likely lead the charge in their respective industries, turning insights into action and challenges into opportunities. The era of data-driven decision-making is here, and predictive analytics is its cornerstone, shaping the business strategies of tomorrow.
Frequently Asked Questions
How is AI transforming predictive analytics?
AI is transforming predictive analytics by incorporating machine learning algorithms that enhance forecasting accuracy, enabling businesses to make more informed decisions by analyzing large data sets and identifying patterns that would be impossible to discern manually.
What are the ethical implications of AI in analytics?
The ethical implications include concerns about privacy, bias in decision-making due to potentially skewed data, and the transparency of AI algorithms. Ensuring ethical AI use involves addressing these issues through regulation, ethical guidelines, and responsible AI practices.
How does real-time data processing impact business strategy?
Real-time data processing allows businesses to react instantly to market changes, customer behavior, and operational issues, enabling a more agile and proactive approach to strategy and decision-making.
Can predictive analytics anticipate consumer behavior shifts?
Yes, predictive analytics can anticipate consumer behavior shifts by analyzing historical data, trends, and patterns, helping businesses to adapt their strategies and offerings to meet changing consumer demands.
What role does predictive analytics play in healthcare?
In healthcare, predictive analytics is used for predictive diagnostics, helping to forecast patient outcomes, personalize treatment plans, and improve overall healthcare delivery by anticipating future health trends and needs.
What are the main challenges in predictive analytics?
Challenges include ensuring data privacy and security, managing and integrating large volumes of data from various sources, and maintaining data quality. Overcoming these challenges is essential for reliable and accurate analytics.
How is IoT data enhancing operational efficiency?
IoT data enhances operational efficiency by providing real-time insights into the performance of machines and systems, enabling predictive maintenance and optimizing resource use, which reduces downtime and costs.
What emerging trends should we expect in predictive analytics?
Emerging trends include the integration of prescriptive analytics, the impact of 5G connectivity on data analysis speed and volume, and the convergence of analytics with blockchain technology for enhanced security and transparency in data handling.