The Evolution of Predictive Analytics in Market Analysis
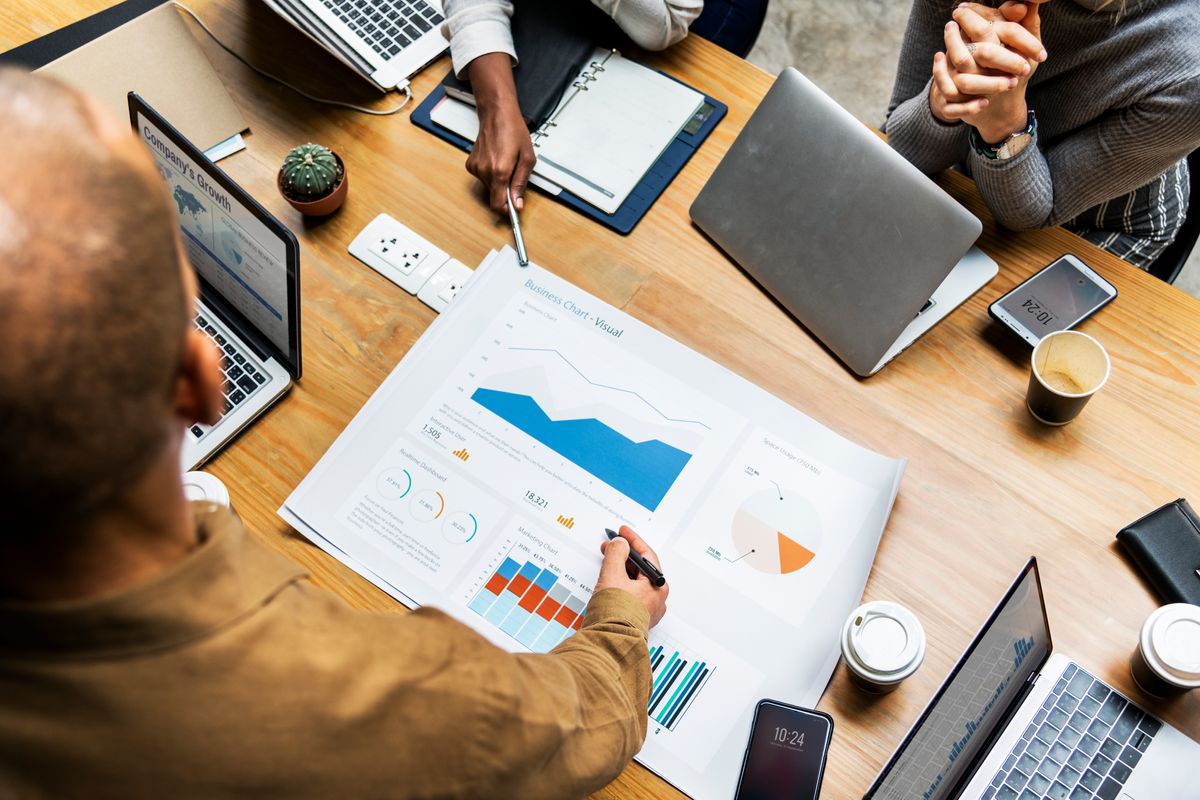
From Reactive to Proactive: The Shift in Business Strategy
The transition from a reactive to a proactive approach in business strategy marks a significant evolution in market analysis. By strategically utilizing predictive analytics, companies are no longer merely responding to market changes; they are anticipating them. This shift enables businesses to maintain leaner inventories, reduce excess costs, and negotiate better terms with suppliers.
- Proactive Problem-Solving: Predictive analytics empowers businesses to stay ahead, meeting demand with real-time efficiency.
- Optimizing Production: Leveraging predictive data for production planning transforms operations to meet market challenges confidently.
- Profit Maximization: Strategic decision-making based on predictive insights ensures efficiency and innovation in all business aspects.
The proactive use of predictive analytics is not just a competitive edge; it is a transformative force that reshapes how businesses approach market dynamics and demand forecasting.
Historical Data: The Bedrock of Predictive Modeling
At the heart of predictive analytics is the meticulous examination of historical data. This data, encompassing everything from sales figures to customer transactions, is not merely a record of what has happened; it is the foundation upon which future forecasts are built. By analyzing past trends and performance, companies can gain valuable insights that guide strategic decision-making.
- The Predictive Analytics Framework
- Data Foundation: Your journey begins with the robust collection and secure storage of quality data, which forms the bedrock for all subsequent analysis.
- Modeling and Machine Learning: Statistical models and machine learning algorithms come into play, transforming raw data into a clear forecast. The incorporation of external data, like weather or social media trends, can further sharpen this forecast.
Predictive models find intricate patterns that often elude the human eye, turning vast amounts of historical information into actionable intelligence.
Understanding the role of historical data in predictive analytics is crucial. It involves collecting data from various sources, including customer transactions and digital footprints, and mining it for patterns and trends. These insights are then applied to create predictive models using statistical and machine learning algorithms, capable of forecasting future events with a reasonable degree of accuracy.
Technological Advancements Driving Predictive Capabilities
The landscape of market analysis is undergoing a seismic shift, thanks to the rapid progression of technological innovations. Algorithmic intelligence, particularly through machine learning algorithms, has become the cornerstone of predictive analytics, enabling systems to adapt and refine forecasts with unprecedented precision.
- Enhanced Predictive Techniques
- AI-Driven Predictive Maintenance
- Streamlined Production
By integrating advanced tools and techniques, predictive analytics is not just forecasting the future but actively shaping it, ensuring businesses stay at the forefront of market dynamics.
The transformative potential of these technologies is not limited to mere predictions; it extends to operational excellence and revenue uplift. Streamlined production processes, informed by accurate demand forecasting, exemplify the tangible benefits of predictive analytics. As businesses embrace these advancements, they position themselves to not only navigate but also lead in the competitive landscape of tomorrow.
Harnessing Algorithmic Intelligence for Market Insights
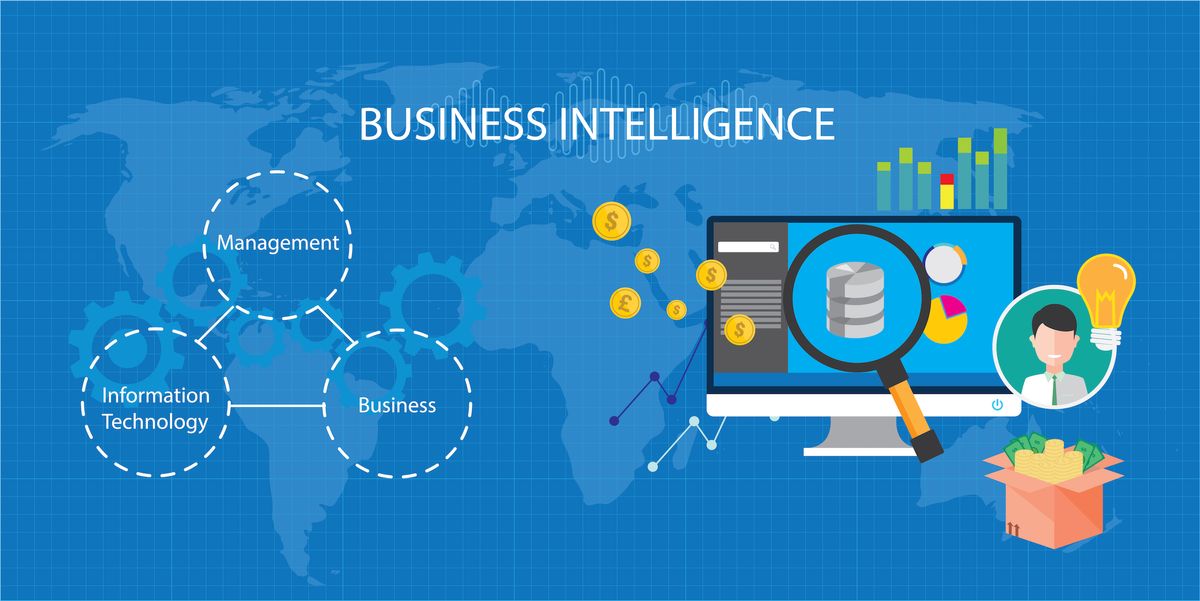
Machine Learning Algorithms at the Core
At the heart of modern market analysis lies the power of machine learning (ML) algorithms, which have revolutionized the way businesses forecast and adapt to market changes. These algorithms are capable of identifying complex patterns and trends that are imperceptible to traditional analysis methods, providing a competitive edge in predictive accuracy.
The continuous improvement of ML algorithms through active learning ensures that each new piece of data refines the predictive model, making it more accurate over time.
The application of ML spans various domains, each contributing uniquely to the predictive analytics framework:
- Digital Analytics: Leveraging ML for digital marketing and web analytics.
- Risk Analytics: Enhancing credit, fraud, and insurance assessments.
- Advanced Analytics: Driving marketing insights and operational excellence.
- Life Sciences: Innovating in bioinformatics and healthcare analytics.
- Computer Vision: Advancing robotics, VR, AR, and autonomy.
However, the reliance on algorithmic intelligence comes with a caveat. The potential for algorithmic bias must be acknowledged and addressed to ensure that the insights generated are both accurate and fair. Regular evaluation and auditing of these algorithms are essential to maintain their integrity and the trust of those who rely on them.
Beyond Traditional Analysis: Embracing Complexity
The modern market landscape is characterized by its dynamic and constantly changing nature, requiring businesses to adopt a more agile approach to decision-making. Quick, yet flexible decisions are essential, allowing for course correction as new data emerges. This agility is underpinned by advanced forecasting modeling techniques, which provide valuable insights into market dynamics, consumer behavior, and future trends.
In the realm of predictive analytics, simplicity should not be underestimated. Overly complex models can lead to overfitting, while a lack of domain expertise can result in inaccuracies. The most effective strategy often involves a straightforward approach, coupled with a deep domain understanding to effectively solve problems with data.
Predictive analytics redefines the boundaries of marketing, embedding strategies in a nuanced understanding of consumer needs.
The evolution of predictive analytics tools is also noteworthy:
- Linear and Multiple Regression Analysis will continue to evolve, offering more nuanced insights.
- Time Series Analysis will become more sophisticated, allowing for better utilization of seasonal trends.
- The complexity of Machine Learning algorithms will increase, unlocking deeper pattern recognition capabilities.
Ethical Considerations in Data Utilization
The integration of predictive analytics into market analysis brings with it a host of ethical considerations. Protecting customer privacy is paramount, as the use of personal data can lead to concerns over surveillance and misuse. It is imperative for businesses to operate with integrity, ensuring that data is used responsibly and with the explicit consent of individuals.
In the realm of predictive analytics, avoiding the perpetuation of biases is a critical challenge. Algorithms must be designed to be fair and impartial, preventing discriminatory outcomes. Transparency is a key factor in maintaining consumer trust; customers should be informed about how their data is being used and have control over their personal information.
The ethical use of predictive analytics is not just a legal obligation but a cornerstone of consumer trust and brand reputation.
Here are some best practices to consider:
- Challenge: Ensuring ethical use of data without infringing on privacy.
Solution: Develop clear privacy policies and obtain explicit consent. - Challenge: Preventing algorithmic bias and discrimination.
Solution: Regularly audit and update models to ensure fairness. - Challenge: Maintaining transparency and building trust.
Solution: Communicate openly with customers about data usage and provide options for data control.
Strategic Decision-Making with Predictive Analytics
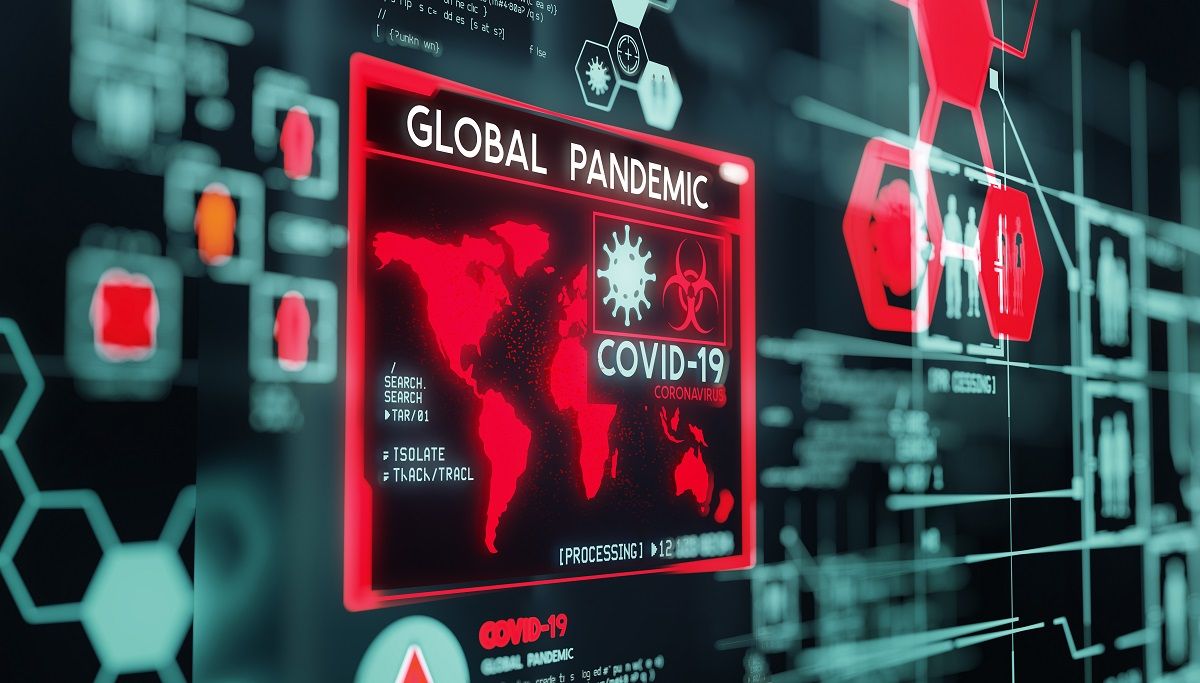
Anticipating Market Trends and Consumer Behavior
The transformative power of predictive analytics lies in its ability to enable businesses to move from a reactive to a proactive stance, forecasting trends and behaviors well in advance. By leveraging historical data and algorithmic intelligence, companies can now anticipate market shifts and consumer preferences with remarkable precision.
Historical Data Analysis is at the core of this predictive prowess. Meticulous examination of past sales and customer interactions reveals patterns that inform future strategies. This analysis is complemented by insights into market dynamics, ensuring a holistic approach to forecasting.
The strategic leverage of predictive analytics fine-tunes marketing efforts to align with anticipated customer needs, offering a competitive edge in a rapidly evolving marketplace.
The following points highlight the key aspects of anticipating market trends and consumer behavior:
- Utilizing machine learning algorithms to transcend traditional analysis.
- Integrating market dynamics with consumer behavior for comprehensive forecasts.
- Identifying potential challenges such as data silos and system compatibility issues.
Data-Driven Decisions for Competitive Advantage
In the modern business landscape, data-driven decisions are the cornerstone of gaining a competitive advantage. The shift from intuition to informed choices has been monumental, with C-Level executives now harnessing the power of analytics to steer their organizations towards success.
- Informed Decision-Making: Leveraging data for strategic choices
- Proactive Responses: Anticipating market changes and consumer needs
- Innovation and Agility: Staying ahead through continuous improvement
By placing data at the heart of decision-making, organizations not only enhance their operational efficiency but also proactively navigate future challenges and opportunities.
The integration of Business Intelligence (BI) tools has transformed the strategic landscape, fostering innovation and growth. As the data analytics market evolves, staying updated with industry trends and regional variations is crucial for maintaining a dynamic and pivotal role in shaping the future of strategic planning.
The Role of Predictive Analytics in Demand Forecasting
In the realm of demand forecasting, predictive analytics is transforming the landscape. By leveraging historical data and machine learning, businesses can anticipate future demand with remarkable accuracy. This foresight enables companies to optimize inventory levels, manage supply chain logistics, and prepare for market fluctuations.
Predictive analytics uses historical data and machine learning to forecast trends, while advanced analytics tools aid in data-driven decision-making for business growth and competitiveness.
The benefits of predictive analytics in demand forecasting are manifold. Here's a concise list:
- Enhanced accuracy in predicting consumer demand
- Reduced inventory costs through better stock management
- Improved customer satisfaction by meeting demand without overstocking
- Strategic pricing based on anticipated market changes
By integrating predictive analytics into their operations, businesses not only streamline processes but also gain a competitive edge in the market.
Predictive Analytics in Crafting Marketing Strategies
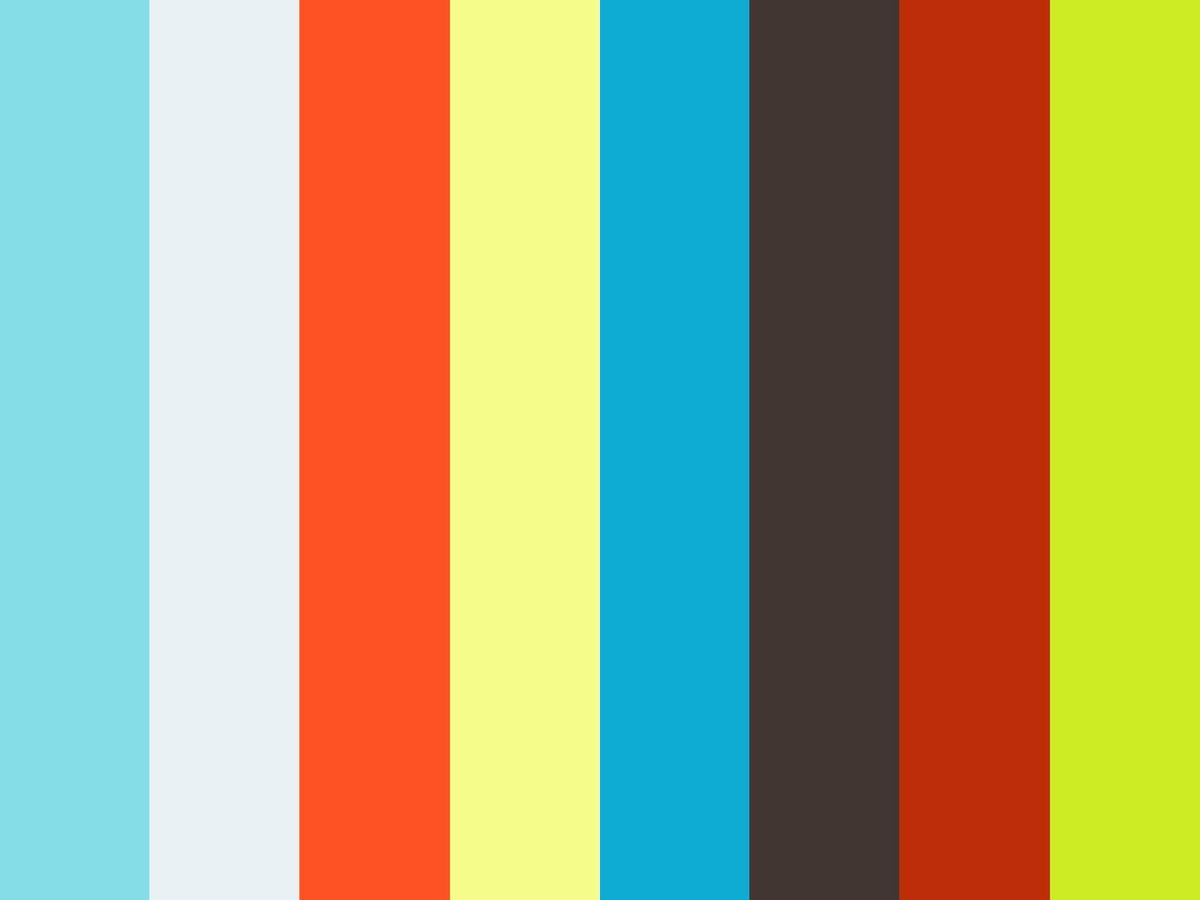
Tailoring Campaigns to Future Customer Needs
In the realm of market analysis, predictive analytics is revolutionizing the way businesses approach marketing campaigns. By leveraging customer data, companies can now anticipate and cater to the evolving preferences of their audience with unprecedented precision.
Personalized promotions and optimized messaging are just the tip of the iceberg. Predictive models enable businesses to dissect their audience into distinct segments, each with unique needs and potential responses to marketing stimuli. For instance, a retailer might use predictive insights to distinguish between customers who favor casual wear and those who prefer formal attire, subsequently crafting campaigns that resonate with each group.
By harnessing the power of predictive analytics, marketers can not only predict future trends but also shape them, creating a proactive rather than reactive marketing strategy.
Here's how predictive analytics can enhance marketing efforts:
- Audience Segmentation: Identify and target specific customer groups based on predicted preferences.
- Personalized Promotions: Craft promotions that align with the anticipated needs of each segment.
- Optimized Channels: Determine the most effective channels for reaching different segments.
- Messaging Tailoring: Fine-tune messaging to speak directly to the customer's desires and expectations.
The integration of predictive analytics into marketing strategies ensures that campaigns are not just tailored, but also timed perfectly to meet future customer needs, thereby maximizing engagement and conversion rates.
Enhancing Customer Engagement through Predictive Insights
Harnessing predictive analytics transforms mountains of data into valuable insights, offering marketers a key to unlock the complexities of consumer behavior with unmatched precision. It sifts through historical data to identify emerging patterns and trends, shedding light on potential avenues for engagement, retention, and growth. Such insights enhance the relevance and effectiveness of marketing efforts, fostering a stronger bond between brands and their audiences, and setting the foundation for sustained customer relationships.
Among the most transformative tools reshaping marketing strategies today is predictive analytics. This advanced approach goes beyond traditional analysis, leveraging vast amounts of data and sophisticated algorithms to anticipate future customer behaviors, preferences, and trends. Predictive analytics empowers marketers to preemptively tailor their campaigns, refine targeting strategies, and enhance customer engagement with unprecedented precision. By embracing this predictive prowess, businesses are not only improving their customer acquisition strategies but also revolutionizing product development and innovation.
Tailored Business Strategies with Predictive Insights
- Personalized Promotions: By predicting which products customers are more likely to purchase, you can craft targeted promotional campaigns that resonate with your audience, driving both revenue and customer loyalty.
- Optimized Channels and Messaging: Predictive analytics informs you about the most effective channels and messaging for reaching your target market, ensuring that your marketing efforts yield the highest return on investment.
Optimizing Marketing Efforts for Maximum Impact
In the pursuit of maximizing the impact of marketing efforts, predictive analytics emerges as a pivotal tool. By leveraging data-driven insights, businesses can customize their marketing campaigns to align with future market trends and consumer preferences. This strategic approach not only enhances the effectiveness of marketing efforts but also fosters a stronger bond between brands and their audiences, setting the foundation for enduring loyalty and success.
Utilizing predictive analytics for market trends allows businesses to stay ahead in the competitive landscape. Advanced analytics tools are instrumental in optimizing decision-making processes, ensuring that each marketing campaign is not only well-targeted but also cost-effective.
Here are some key benefits of using predictive analytics in marketing:
- Personalized Promotions: Craft targeted campaigns that resonate with your audience.
- Optimized Channels and Messaging: Determine the most effective channels and messaging for your target market.
- Enhanced Customer Engagement: Foster a stronger bond with your audience through relevant and timely interactions.
Advanced Tools and Techniques for Precision Forecasting
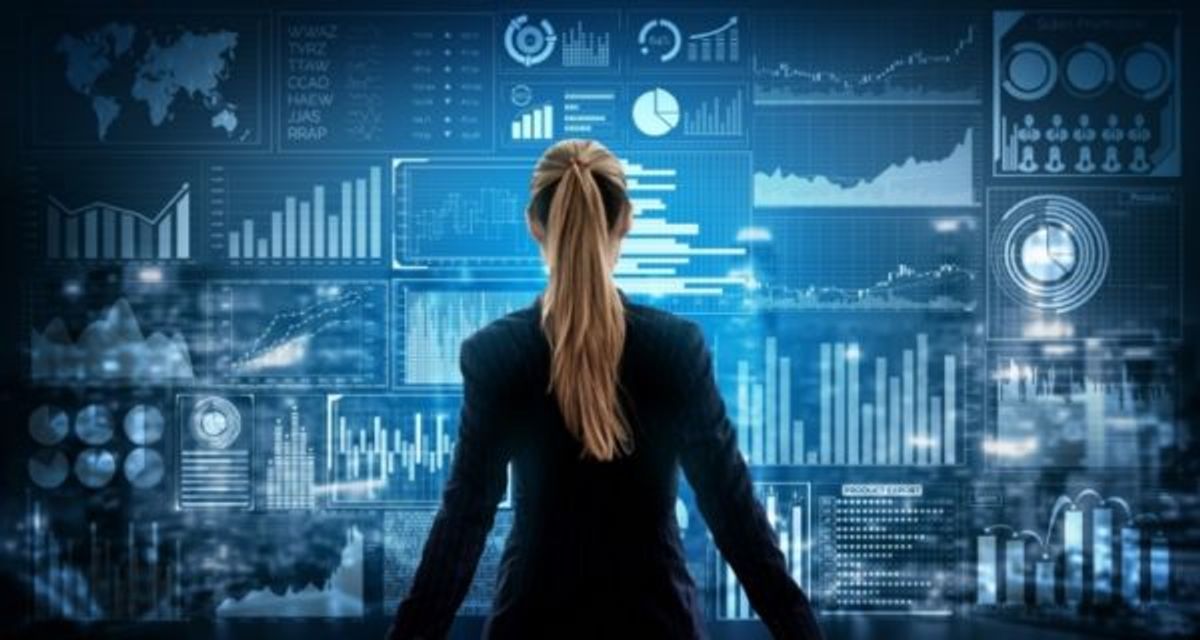
Deciphering Patterns for Accurate Forecasts
At the core of predictive analytics is the ability to identify and interpret complex data patterns. Pattern recognition is crucial for transforming raw data into meaningful insights. Historical data analysis serves as the foundation for this process, where sales figures and customer transactions are scrutinized to uncover trends that might otherwise go unnoticed.
Predictive models not only analyze past data but also integrate market trends and consumer behavior to deliver forecasts that are strategic assets. The continuous improvement loop inherent in predictive analytics means that the system learns from each decision, constantly refining its forecasting accuracy.
The final step is to convert these data-driven predictions into actionable strategies. This requires setting clear objectives, allocating resources wisely, and monitoring outcomes to achieve desired goals.
The predictive power gained from these methods allows for the anticipation of future events with a reasonable degree of accuracy. Whether it's predicting customer purchasing habits or the potential success of marketing campaigns, this foresight is invaluable for businesses aiming to optimize efforts and enhance efficiency.
Integrating Market Dynamics with Consumer Insights
The synergy between market dynamics and consumer insights is pivotal in sculpting the landscape of predictive analytics. By weaving together the intricate patterns of market trends with the rich tapestry of customer behavior, businesses can forecast demand with unparalleled accuracy. This integration ensures decisions are well-informed and attuned to the external market pulse.
Predictive analytics, powered by algorithmic intelligence, transcends traditional analysis by not only interpreting historical data but also by anticipating future needs and preferences. Harnessing this capability allows for the creation of dynamic pricing models that adapt in real-time to market fluctuations, optimizing sales and profit margins.
The true power of predictive analytics lies in its ability to transform data into actionable insights, offering a competitive edge in today's fast-paced market.
Understanding and managing predictions is crucial in this context. For instance, Dynamics 365 Customer Insights provides tools to edit predictions, refresh them with the latest data, and generate input data usability reports, ensuring that your predictive models remain relevant and effective.
The Importance of Continuous Data Analysis
In the realm of market analysis, continuous data analysis is not just a practice but a necessity. The dynamic nature of the market demands that businesses stay vigilant, constantly updating and refining their predictive models. This iterative process is crucial for maintaining the relevance and accuracy of insights derived from predictive analytics.
The agility afforded by continuous data analysis enables organizations to respond swiftly to market changes, ensuring that strategic decisions are informed by the most current data.
To achieve operational excellence and revenue uplift, consider the following points:
- Establish robust data collection and quality assurance protocols.
- Utilize machine learning algorithms for a continuous learning curve.
- Streamline production to align with predictive demand forecasts.
- Adapt to latest trends and challenges through training and co-development.
The integration of augmented data management and the convergence of analytics with business intelligence are recent industry developments that underscore the importance of continuous analysis. These advancements facilitate the automation of data quality and integration processes, reinforcing the role of BI in strategic decision-making.
The Ethical Implications of Predictive Analytics
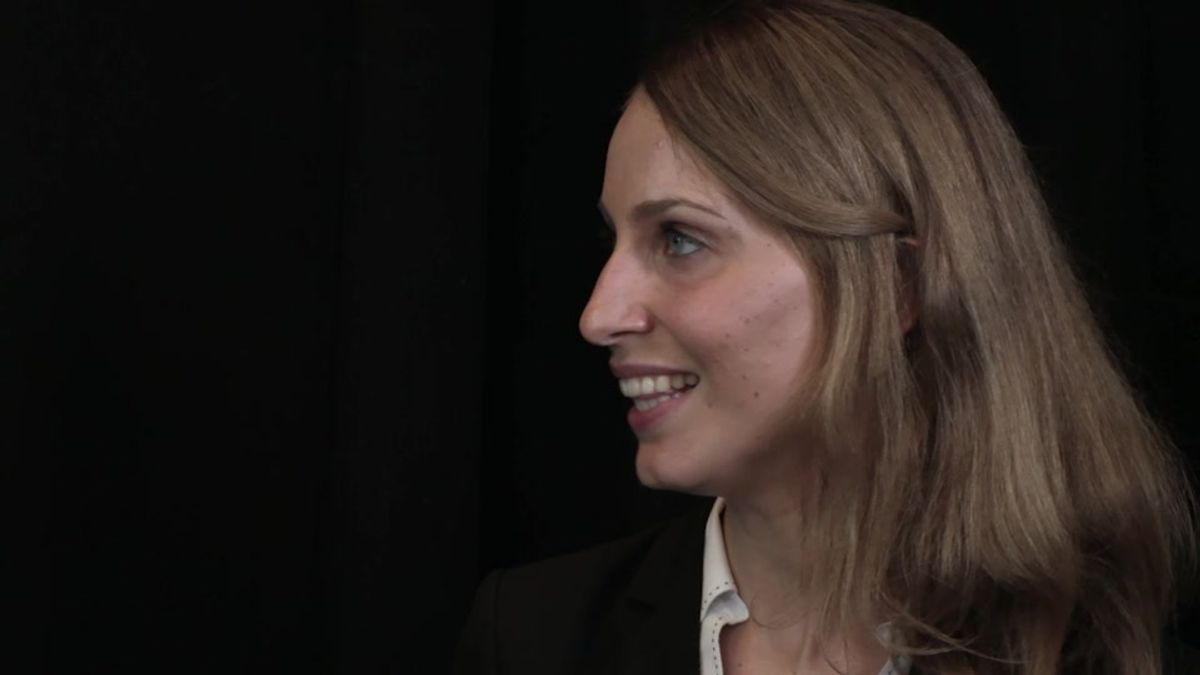
Balancing Innovation with Privacy Concerns
In the quest to harness the power of predictive analytics, businesses must navigate the delicate balance between innovation and privacy. Businesses should prioritize data transparency, compliance, ethics, and security to maintain consumer trust while exploring new frontiers in market analysis.
- Challenge: With great data comes great responsibility—protecting sensitive information is paramount.
- Solution: Implement stringent data encryption and access controls. Ensure compliance with all relevant data protection regulations, instilling confidence in your predictive analytics framework.
While the potential of predictive analytics in marketing is immense, it is crucial to consider ethical implications and adhere to best practices. As marketers delve into personal data and employ predictive algorithms, privacy concerns arise.
Ensuring ongoing accuracy and addressing potential biases in predictive models are critical for maintaining trust. Continuous monitoring and refinement of models improve accuracy and fairness. Transparency is fostered by providing clear explanations of how predictions are generated, and by offering users control over their data and privacy settings.
Best Practices in Predictive Analytics
The potential of predictive analytics is transformative, offering a proactive stance in market analysis. To harness this potential effectively, certain best practices are paramount.
- Define Clear Objectives: Establish what you aim to achieve with predictive analytics. Without clear goals, it's challenging to measure success or derive actionable insights.
- Quality Data Matters: The accuracy of predictions hinges on the quality of the underlying data. Ensuring data integrity is crucial for reliable outcomes.
- Appropriate Model Selection: Choose the right models that align with your objectives and data structure. This choice is critical in predicting future trends accurately.
- Continuous Model Improvement: Predictive models are not set-and-forget tools. They require ongoing refinement to adapt to new data and evolving market conditions.
By adhering to these best practices, businesses can navigate the complexities of predictive analytics, ensuring ethical use and sustainable success. It's essential to prioritize customer data protection and maintain transparency to build trust and adhere to privacy concerns.
Building Trust through Responsible Data Management
In the realm of predictive analytics, trust is the cornerstone of any successful strategy. Businesses must not only focus on harnessing data for insights but also on the ethical management of that data. Transparency in model management is a key challenge, requiring continuous monitoring and refinement to ensure accuracy and fairness. By providing clear explanations of predictions and offering control over data and privacy settings, companies can foster a deeper sense of trust with their users.
Security is another critical aspect, with stringent data encryption and access controls being non-negotiable. Compliance with data protection regulations reassures stakeholders of the integrity of the predictive analytics framework. Moreover, the foundation of predictive analytics lies in the quality of the data itself. Establishing robust data collection and quality assurance protocols is essential, as is aligning predictive tools with strategic goals through pilot studies.
Predictive analytics empowers businesses to anticipate customer behaviors, leverage industry trends, and mitigate risks for sustainable success through data-driven insights.
Finally, integration and change management are vital for ensuring that predictive analytics tools enhance rather than disrupt strategic objectives. Enhanced data management not only secures data but also ensures it is backed up and retrievable, maintaining operational integrity and decision-making capabilities.
Future-Proofing Businesses with Predictive Analytics
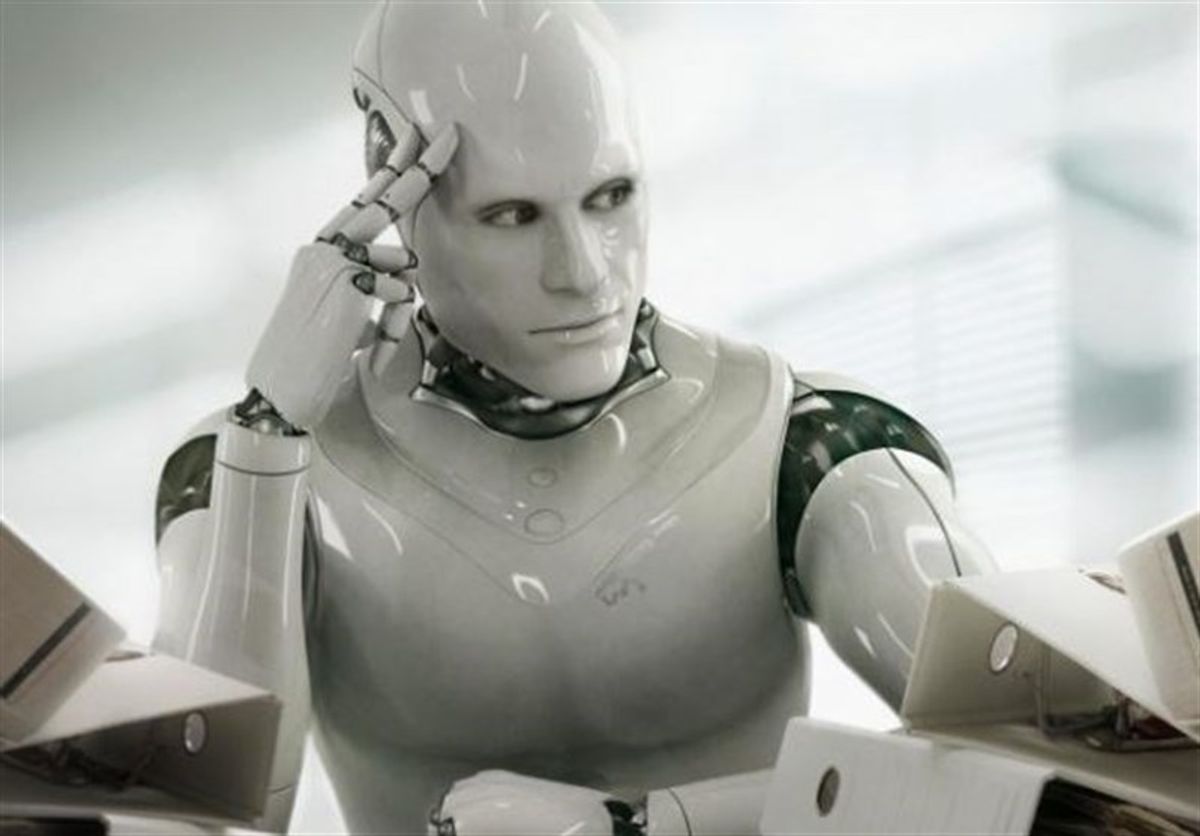
Staying Ahead of the Curve in a Dynamic Market
In the race to harness the full potential of predictive analytics, businesses are discovering innovative ways to not just keep pace, but to truly lead in a dynamic market. By integrating predictive analytics into their strategic planning, companies can anticipate changes and adapt swiftly, ensuring they are always ahead of the competition.
- Dynamic Pricing Models: Utilize predictive data analytics to develop dynamic pricing strategies that respond in real-time to market demands.
- Competitive Advantage: Gain insights into market trends to position products competitively and tailor prices based on predictive models.
By strategically utilizing predictive analytics, you're not just responding to the market—you're staying several strides ahead, poised to meet demand in real-time and with maximum efficiency. This strategic foresight is not just a competitive edge; it's a transformative force in how you approach demand forecasting and production planning.
Predictive analytics is not only about staying competitive; it's about being a transformative force in the marketplace, ensuring that marketing strategies are both current and forward-thinking.
The Role of Predictive Analytics in Sustainable Growth
In the pursuit of sustainable growth, businesses are increasingly turning to predictive analytics to ensure longevity and relevance in a competitive market. By leveraging predictive supply chain data analytics, companies can not only optimize their operations but also engage in ethical sourcing and environmentally conscious practices.
Predictive analytics enables a proactive approach to market demands, fostering a business ecosystem that is both resilient and adaptable to change.
The strategic use of predictive analytics in business operations leads to enhanced operational efficiency, which is crucial for maintaining a sustainable growth trajectory. Here are some key benefits:
- Anticipating consumer needs and market trends
- Optimizing production to reduce waste
- Ensuring ethical sourcing and sustainable practices
By integrating predictive analytics into their strategy, businesses can set the stage for sustained growth and success, navigating the complexities of the market with foresight and precision.
Preparing for the Unpredictable: Flexibility and Adaptation
In the realm of market analysis, preparing for the unpredictable is a crucial aspect of maintaining a competitive edge. The use of predictive analytics has become a cornerstone in crafting strategies that are both flexible and adaptive to market changes. By strategically utilizing predictive analytics, businesses are not just responding to the market—they are staying several strides ahead, ready to meet demand with maximum efficiency.
Adapt and innovate: Stay agile by continuously exploring and experimenting with the latest AI tools and technologies. This proactive approach ensures the ability to leverage the most effective solutions that align with evolving project needs and industry trends.
By fine-tuning predictive models and embracing a continuous improvement loop, organizations can deliver forecasts that are not just predictions, but strategic assets. These models learn from each decision, creating a self-optimizing system that perpetually enhances forecasting accuracy.
The integration of continuous learning and adaptation into business intelligence (BI) practices ensures that organizations remain agile, adapting to the latest trends, challenges, and opportunities as they arise. Whether choosing data science or AI, staying adaptable and continuously learning will be key to success in these dynamic fields.
The Integration of Predictive Analytics in Various Industries
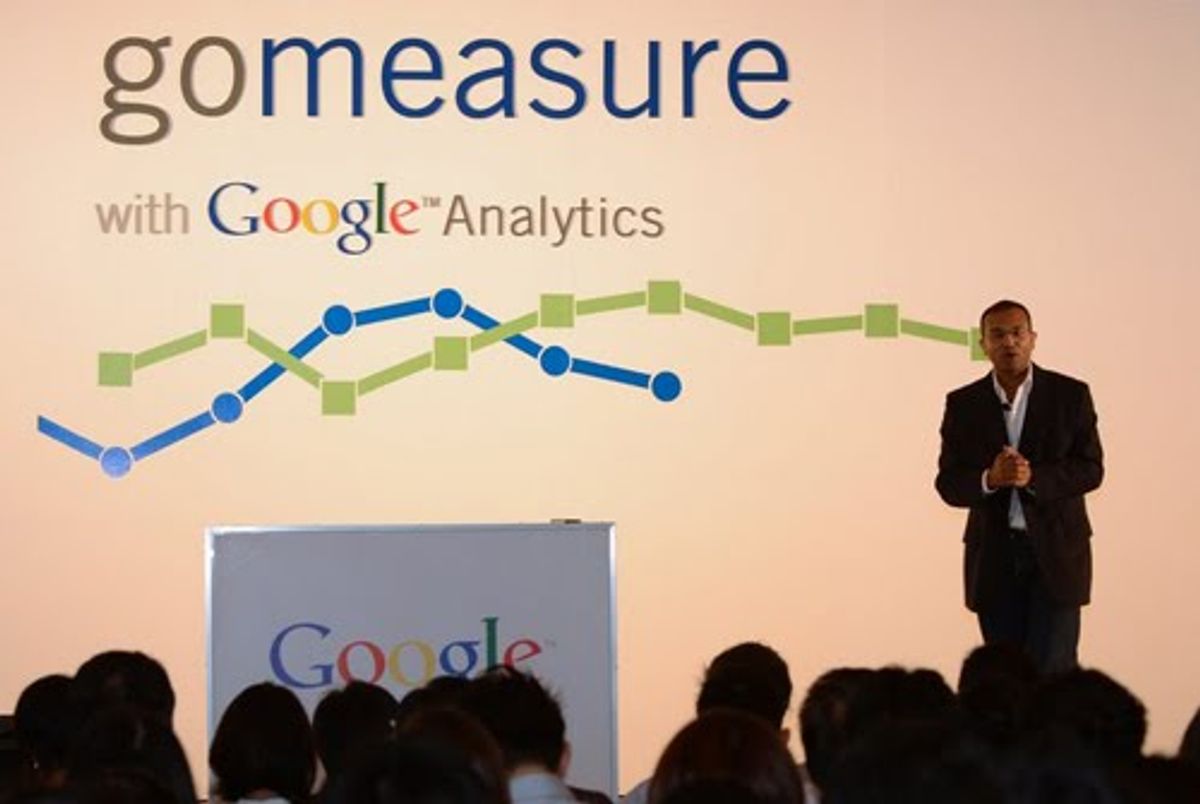
Case Studies: Success Stories Across Sectors
The application of predictive analytics is not confined to a single industry; it has been a game-changer across various sectors. Predictive analytics revolutionizes market positioning, healthcare, and strategic decision-making. By leveraging data-driven insights and AI integration, businesses have been able to gain a significant competitive advantage through proactive problem-solving.
In the healthcare sector, predictive models have improved patient outcomes by anticipating disease trends and optimizing treatment plans. The financial services industry has seen a reduction in risk and fraud through predictive risk assessment tools. Retailers are using predictive analytics to personalize shopping experiences and manage inventory more efficiently.
The integration of predictive analytics into business operations has become a cornerstone for success in today's data-centric world.
Here are a few examples of industries that have successfully implemented predictive analytics:
- Education: Enhancing student learning outcomes and optimizing administrative processes.
- Automotive: Predicting maintenance needs and driving innovation in vehicle design.
- Manufacturing: Streamlining production lines and predicting equipment failures.
Each case study reflects the transformative power of predictive analytics, demonstrating its vital role in shaping the future of business strategies.
Industry-Specific Challenges and Solutions
Each industry faces unique challenges when integrating predictive analytics into their operations. Data governance is paramount, requiring robust practices to maintain data quality and security. Skill development is another hurdle, necessitating investment in training or hiring experts in data analytics.
While industries like education, automotive, and healthcare are leveraging predictive analytics, they must overcome issues such as data silos and system compatibility to unlock deeper market insights.
A strategic approach to these challenges includes identifying potential roadblocks and fostering an environment that embraces change. For instance:
- Data Governance: Ensuring high data quality and security.
- Skill Development: Equipping teams with necessary predictive modeling expertise.
- System Compatibility: Streamlining integration with existing infrastructure.
By addressing these industry-specific challenges, businesses can harness the full potential of predictive analytics for sustainable growth.
Cross-Industry Learnings and Best Practices
The integration of predictive analytics across various industries has highlighted the universal value of data-driven insights. Best practices have emerged that transcend sector-specific challenges, offering a blueprint for successful implementation regardless of the industry.
- Data Governance: Ensuring high data quality and security.
- Skill Development: Building expertise in data analytics.
- Algorithmic Intelligence: Leveraging machine learning for continuous improvement.
- Operational Excellence: Fine-tuning production to meet forecasted demand.
Embracing these cross-industry learnings not only streamlines the adoption of predictive analytics but also fosters an environment of continuous innovation and improvement.
The synergy between different sectors has led to a collaborative approach in overcoming common obstacles. By sharing knowledge and strategies, industries are able to accelerate their growth and adapt more swiftly to market changes.
Predictive Analytics and the Customer Experience
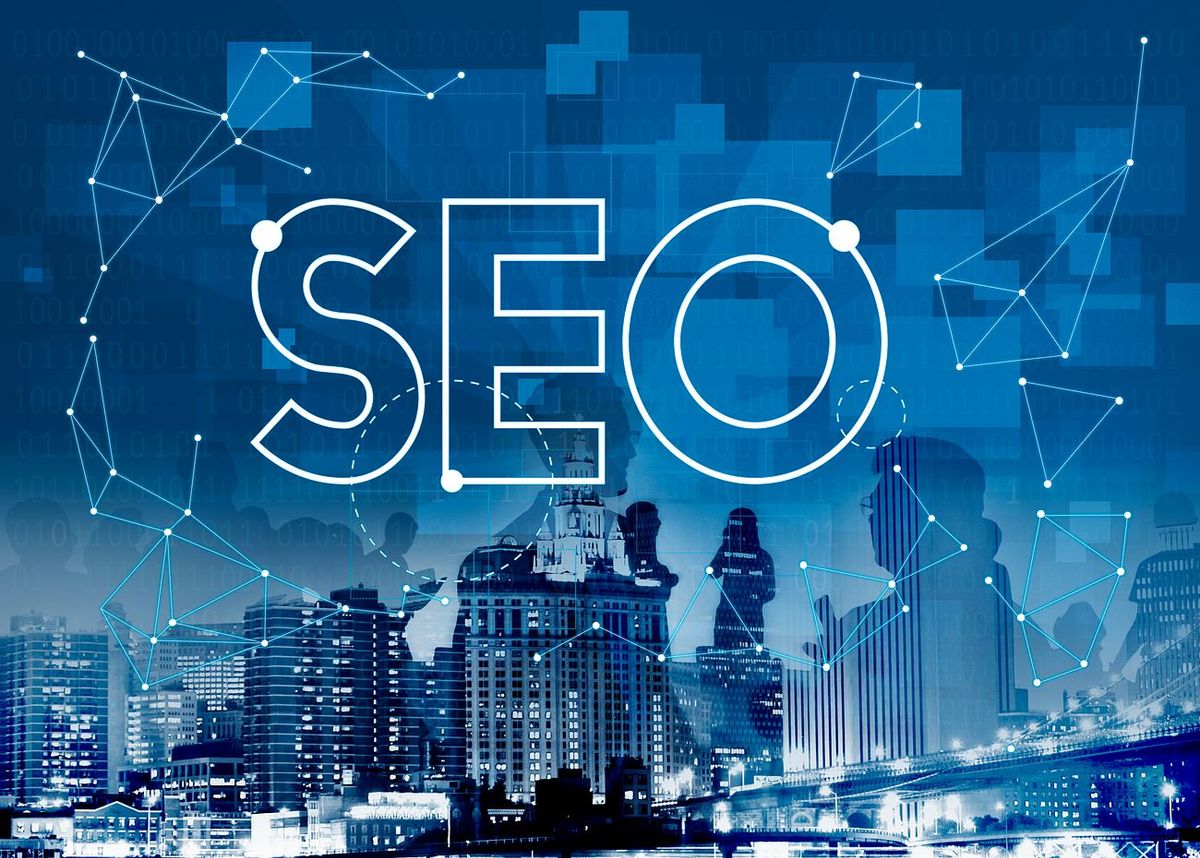
Personalization at Scale: The New Frontier
The advent of cloud-based analytics has revolutionized the way businesses interact with their customers. By harnessing the power of machine learning tools, companies can now engage in predictive personalization, crafting marketing strategies that resonate deeply with consumers. This marks a new era where marketing transcends conventional outreach and becomes a predictive dialogue with the audience.
As predictive analytics continues to evolve, it redefines the boundaries of marketing, promising a future where strategies are not just reactive but deeply embedded in a nuanced understanding of consumer needs.
One illustrative example is a brand that implemented personalized marketing strategies recommended by their IT support. The result was a 40% increase in customer engagement and a significant uptick in sales, demonstrating how technology can directly contribute to competitive advantage and business success. AI-driven business models offer agility and competitive advantage in various industries, enabling businesses to stay ahead of the curve.
POPULAR INSIGHTS:
- SHOP WITH PURPOSE AT IMPACT MART!
- CUSTOMER ONBOARDING: PROVEN TECHNIQUES FOR SEAMLESS CLIENT INTEGRATION
- CUSTOMER JOURNEY: OPTIMIZING INTERACTIONS FOR LASTING CUSTOMER LOYALTY
- EMAIL OPEN RATES: ENHANCING THE READERSHIP OF YOUR MARKETING EMAILS
- REVENUE: THE FUNDAMENTAL PILLAR OF BUSINESS VIABILITY AND GROWTH
Predicting and Shaping the Customer Journey
The art of predicting and shaping the customer journey is a transformative force in modern marketing. By leveraging predictive analytics, businesses can anticipate future customer behaviors and preferences with a remarkable degree of accuracy. This foresight enables the creation of more personalized and effective marketing strategies.
Predictive analytics empowers marketers to preemptively tailor their campaigns, refine targeting strategies, and enhance customer engagement with unprecedented precision.
Among the tools that make this possible, predictive analytics stands out for its ability to process vast amounts of data and sophisticated algorithms. It's not just about understanding current trends but also about forecasting future events, from customer purchasing habits to the potential success of marketing campaigns. The predictive power is invaluable for businesses looking to optimize marketing efforts, enhance customer experiences, and increase overall efficiency.
- Tailored Business Strategies with Predictive Insights
- Personalized Promotions: Craft targeted promotional campaigns that resonate with your audience.
- Optimized Channels and Messaging: Ensure your marketing efforts yield the highest return on investment.
As predictive analytics continues to evolve, it redefines the boundaries of marketing, promising a future where strategies are deeply embedded in a nuanced understanding of consumer needs. For those who navigate these waters successfully, predictive analytics offers a competitive edge in a digital marketplace that is constantly in flux.
Measuring the Impact of Predictive Analytics on Customer Satisfaction
The integration of predictive analytics into marketing strategies has marked a significant shift towards a more data-driven approach. By analyzing patterns in customer data, businesses can tailor their interactions to meet the evolving needs of their clientele. Predictive analytics enhance customer satisfaction and loyalty by enabling companies to foresee and act upon customer desires with greater precision.
Personalization is at the heart of this transformation. With advanced algorithms, businesses can segment customers more effectively, predict potential churn, and optimize sales and inventory to ensure that customer demands are met promptly and accurately. This AI-driven personalization leads to more meaningful and engaging customer experiences.
The true measure of predictive analytics lies in its ability to revolutionize the customer experience, turning data into actionable insights that drive satisfaction and retention.
To quantify the impact of predictive analytics on customer satisfaction, consider the following metrics:
- Customer Satisfaction Score (CSAT)
- Net Promoter Score (NPS)
- Customer Effort Score (CES)
- Retention and Churn Rates
- Sales Conversion Rates
These indicators provide a structured way to assess the effectiveness of predictive analytics in creating a positive customer experience and fostering loyalty.
Navigating the Complexities of Market Analytics
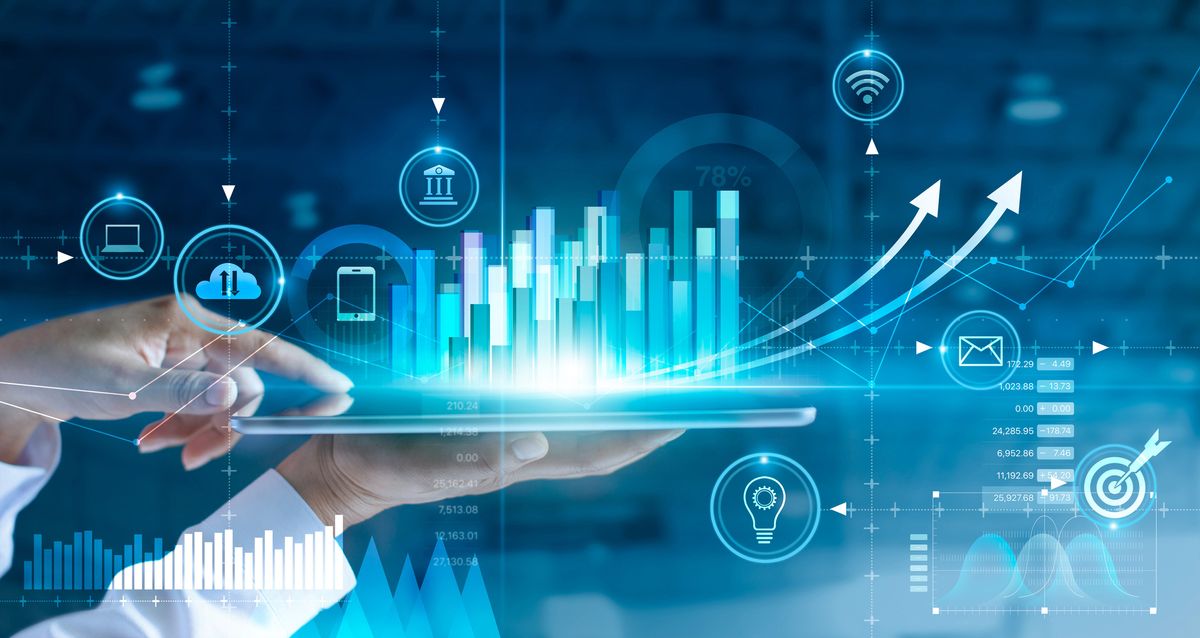
The Convergence of Big Data and Predictive Analytics
The fusion of Big Data and predictive analytics marks a transformative era in market analysis. With vast amounts of data at their disposal, businesses are now equipped to delve deeper into market complexities and uncover patterns that were previously indiscernible. The synergy between extensive datasets and sophisticated analytics paves the way for a more nuanced understanding of consumer behavior and market trends.
Predictive analytics is not just about forecasting; it's about shaping the future of business strategies. By integrating historical data with real-time insights, companies can anticipate changes and adapt swiftly, ensuring they remain competitive in a rapidly evolving marketplace. This proactive approach is encapsulated in the ability of AI-driven predictive analytics to enable businesses to adapt to market dynamics, forecast trends, optimize operations, and personalize customer experiences for sustainable growth.
The predictive models that emerge from this convergence are instrumental in driving strategic decisions. They serve as a compass, guiding businesses through the uncertainty of market fluctuations and consumer preferences.
Here are some key functions of predictive analytics in market analysis:
- Forecasting market trends and consumer behavior
- Optimizing marketing campaigns and operations
- Personalizing customer experiences
- Identifying new market opportunities
- Mitigating risks by anticipating potential challenges
Overcoming Analytical Challenges with Advanced Technologies
The implementation of predictive analytics is fraught with challenges that can impede its integration into market analysis. Expertise in data analytics is essential, as the complexity of predictive models requires a deep understanding of data science and machine learning. To address this, businesses are increasingly turning to user-friendly platforms that simplify the use of predictive analytics, offering intuitive interfaces and guided analytics.
By investing in these advanced technologies, companies can streamline their predictive analytics processes, ensuring that their market analysis is both accurate and actionable.
Another key challenge is data governance. High data quality and security are paramount for reliable predictions. Here's a strategic approach to overcoming these hurdles:
- Data Governance: Implement robust practices to ensure data integrity.
- Skill Development: Train or hire experts to navigate predictive models.
- Algorithmic Intelligence: Leverage machine learning for continuous improvement.
By tackling these challenges with strategic solutions, businesses can secure a place at the forefront of digital innovation, transforming their approach to market analysis and decision-making.
Future Trends in Predictive Analytics and Market Analysis
The predictive analytics market is experiencing substantial growth, driven by increased awareness and the need for data-driven decision-making. Future trends suggest a continued expansion of capabilities, with businesses leveraging predictive insights to stay ahead of the curve. The integration of market dynamics with consumer behavior is key to crafting strategies that resonate with customers on a deeper level.
- Market Trends and Customer Insights: Predictive analytics now goes beyond internal data, incorporating external market pulses for more precise demand forecasting.
- Algorithmic Intelligence: Machine learning algorithms are pushing the boundaries of traditional analysis, offering nuanced insights into consumer needs.
The strategic leverage of predictive analytics ensures that marketing efforts are fine-tuned to customer needs, offering a competitive edge in a digital marketplace that is constantly in flux.
As we look to the future, the role of predictive analytics in market analysis will only grow more integral. Companies that harness this power will find themselves at the forefront of innovation, ready to meet the evolving demands of the marketplace.
In today's fast-paced business environment, understanding and leveraging market analytics is crucial for staying ahead of the competition. At OptimizDBA, we specialize in providing faster data solutions and database optimization consulting to transform your data management and enhance your decision-making process. Our proprietary methodologies and extensive experience ensure that your transaction speeds will soar, often by 100 to 1000 times. Don't let data complexity slow you down. Visit our website to learn how we can accelerate your business growth and schedule a consultation to discuss your unique challenges.
Conclusion
As we stand on the cusp of a new era in market analysis, predictive analytics emerges as a pivotal force, redefining the landscape of business strategy and customer engagement. The insights provided in this article underscore the transformative potential of predictive analytics, from deciphering complex patterns in historical data to anticipating market trends and consumer behaviors with remarkable accuracy. Businesses equipped with these advanced tools can navigate the future with confidence, making informed decisions that are not merely reactive, but strategically proactive. However, the journey ahead is not without its challenges. Ethical considerations, the need for technological expertise, and the imperative for continuous innovation are all critical factors that businesses must address to harness the full power of predictive analytics. For those who can adeptly balance these elements, the rewards are substantial—a competitive edge in a rapidly evolving digital marketplace and the ability to shape the future of market analysis.
Frequently Asked Questions
What is predictive analytics in market analysis?
Predictive analytics in market analysis is a sophisticated approach that uses historical data, statistical algorithms, and machine learning techniques to forecast likely future market trends, customer behaviors, and outcomes, enabling businesses to make proactive, informed decisions.
How does predictive analytics shift business strategy?
Predictive analytics shifts business strategy from a reactive to a proactive stance, allowing companies to anticipate market changes and customer needs, and to strategize accordingly for competitive advantage.
What role does historical data play in predictive analytics?
Historical data is the foundation of predictive analytics, providing the raw material for models to identify patterns and trends that inform future forecasts and strategic decisions.
What technological advancements enhance predictive analytics?
Advancements such as machine learning algorithms, big data processing, and artificial intelligence significantly enhance the capabilities of predictive analytics by enabling more complex and accurate analysis.
What are the ethical considerations in predictive analytics?
Ethical considerations include data privacy, consent, avoiding bias in algorithms, and ensuring transparency in how data is collected, used, and managed to maintain consumer trust and adhere to regulations.
How does predictive analytics impact marketing strategies?
Predictive analytics allows marketers to tailor campaigns to future customer needs, enhance engagement by predicting behaviors, and optimize marketing efforts for maximum impact and ROI.
What advanced tools are used in predictive analytics for precision forecasting?
Advanced tools in predictive analytics include data mining, statistical modeling, machine learning algorithms, and real-time analytics platforms that help in deciphering complex patterns for accurate forecasting.
How can businesses navigate the complexities of predictive analytics?
Businesses can navigate the complexities by investing in the right technology and expertise, continuously analyzing data, and staying abreast of the latest trends and best practices in predictive analytics.