Understanding the Fundamentals of Analytics
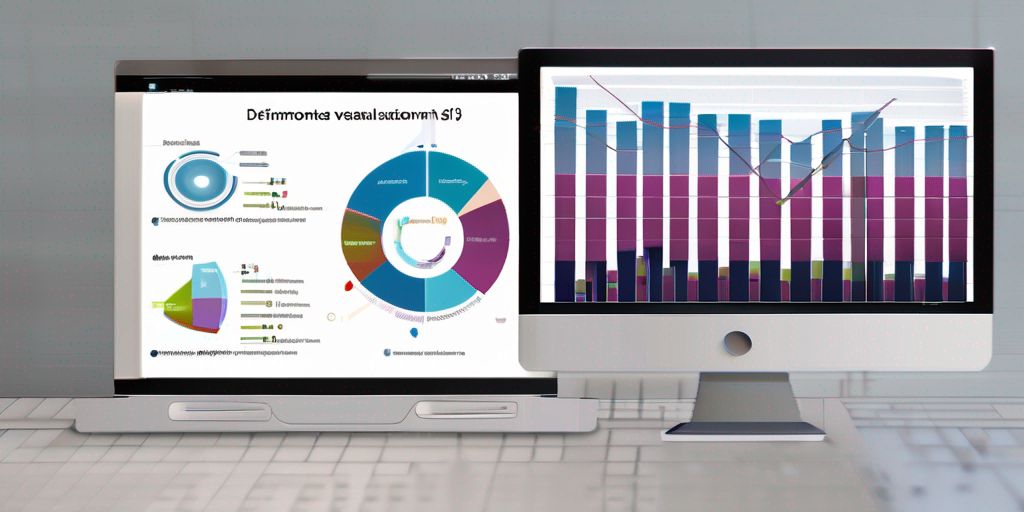
Defining Analytics and Its Importance
Data analytics is fundamental for enabling data-driven decision making. It plays a pivotal role in providing the necessary tools and insights to extract meaningful information from the vast amounts of data that organizations generate and collect. By leveraging data analytics, businesses can transform raw data into actionable insights, enabling them to make informed decisions that drive growth and success.
The Importance of Data Analytics for Business Decision Making
Data analytics has become an essential tool for businesses across various industries. It offers a multitude of benefits that can significantly impact the decision-making process. Let's explore some key reasons why business owners should embrace data analytics for effective decision making:
- Identifying Customer Needs and Preferences
- Gaining a deeper understanding of operations, customers, and market trends
Data analytics empowers business owners to stay ahead of the competition and make more effective, data-driven decisions.
Types of Analytics: Descriptive, Predictive, Prescriptive
Data analytics is the process of leveraging raw data to answer questions, find insights into operational workflows, or observe trends. The four major types of data analytics are descriptive, diagnostic, predictive, and prescriptive. These types of analytics serve different purposes and provide valuable insights for decision-making. Here's a brief comparison of the four types:
Type of Analytics | Purpose |
---|---|
Descriptive | Summarizes historical data to provide context and understanding. |
Diagnostic | Focuses on identifying the reasons behind past outcomes or events. |
Predictive | Utilizes historical data to make informed predictions about future outcomes. |
Prescriptive | Recommends actions or strategies based on predictive analysis. |
Understanding the distinctions between these types of analytics is crucial for extracting actionable insights and making informed decisions. It's important to choose the right type of analytics based on the specific goals and requirements of the analysis.
The Analytics Process: From Data Collection to Interpretation
Understanding the four types of data analytics is crucial for making data-driven decisions. Data analytics encompasses descriptive, diagnostic, predictive, and prescriptive analytics. Each type serves a unique purpose in extracting insights from raw data. Here's a brief overview:
Type of Analytics | Purpose |
---|---|
Descriptive | Summarizes historical data |
Diagnostic | Identifies reasons behind past outcomes |
Predictive | Forecasts future trends |
Prescriptive | Recommends actions for desired outcomes |
By understanding and applying these analytics, businesses can uncover valuable insights and make informed decisions. Here are some key considerations:
- Develop actionable insight statements based on clean, contextualized data
- Implement operational changes based on data outputs
- Stay updated with the latest tools, techniques, and trends in data analytics
Transitioning to a data-driven approach can lead to improved efficiency, better customer experiences, and a competitive edge in the market.
In today’s data-driven world, leveraging data analytics for decision making is no longer a luxury but a necessity for businesses striving for success. Data analytics provides valuable insights into customer needs and preferences, helps optimize operations, and shapes strategic decision-making.
The Role of Big Data in Modern Analytics
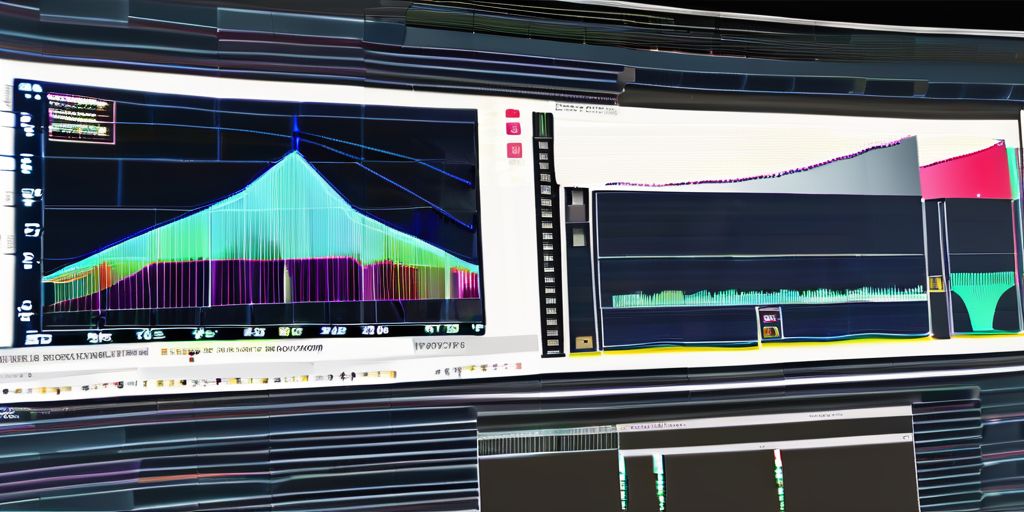
Characteristics of Big Data
Big data is characterized by its complexity and volume of data. It presents a significant challenge in terms of data management and analysis. To illustrate, consider the following table:
Characteristic | Description |
---|---|
Complexity | High |
Volume | Massive |
Additionally, big data requires advanced analytics techniques and tools to facilitate insightful and rapid decision-making. It is essential to treat big data as a key business asset and leverage it to outperform competitors. Here are some key points to consider:
- Implement advanced analytics techniques and tools
- Treat big data as a key business asset
- Leverage big data to outperform competitors
In dealing with big data, it's important to remember the following advice:
Embrace advanced analytics techniques and tools to unlock the potential of big data.
How Big Data Fuels Analytical Models
Big data plays a crucial role in fueling analytical models, providing the vast and diverse datasets necessary for robust analysis and prediction. By harnessing the power of big data, organizations can gain valuable insights into customer behaviors, market trends, and future sales prospects. This enables data scientists and analysts to develop sophisticated models that drive informed decision-making and business growth. The influence of big data on analytical models is undeniable, shaping the way organizations interpret and utilize data for strategic foresight and actionable insights.
In the realm of big data analytics, structured and quantitative data can be effectively presented in a Markdown table, allowing for clear and concise representation of complex datasets. Additionally, a bulleted list can be utilized to outline the steps involved in leveraging big data for analytical modeling, providing a structured guide for organizations to follow in their data-driven endeavors.
Harnessing the power of big data is essential for informed decision-making and business growth. Organizations must embrace the potential of big data to unlock new opportunities and drive strategic foresight.
Challenges and Solutions in Big Data Analytics
The challenges in big data analytics are multifaceted, requiring innovative solutions to address the burgeoning complexity and volume of data. Implementing robust data governance practices is crucial for ensuring data quality and integrity. Additionally, organizations can benefit from leveraging advanced analytics techniques and tools to facilitate rapid decision-making. It's important to establish a data-driven culture that prioritizes continuous improvement and learning. Furthermore, organizations should consider the ethical implications of data analysis and interpretation, ensuring that data privacy and security are upheld. Finally, fostering collaboration between data scientists and business stakeholders is essential for overcoming these challenges effectively.
Technological Enablers of Advanced Analytics
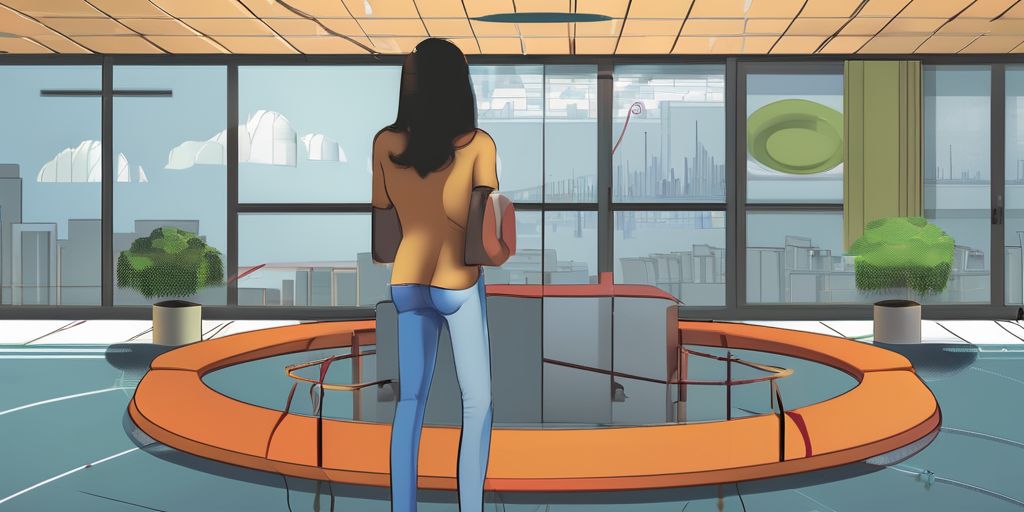
The Rise of Machine Learning and AI in Analytics
The rise of Machine Learning and AI in analytics has revolutionized the way data is processed and interpreted. With the ability to handle complex patterns and large datasets, machine learning algorithms have become essential in uncovering valuable insights from data. AI, on the other hand, enables intelligent decision-making and automation in analytics processes. These technological advancements have significantly enhanced the capabilities of analytics, leading to more accurate predictions and actionable recommendations.
- Implementing Machine Learning and AI in analytics requires a strategic approach to data processing and model development. It involves:
- Data preprocessing and feature engineering
- Model training and validation
- Deployment and monitoring of AI models
Tip: When integrating machine learning and AI into analytics, it's crucial to prioritize data quality and model interpretability to ensure reliable and ethical decision-making.
Cloud Computing and Its Impact on Data Analysis
The advent of cloud computing has revolutionized the way organizations approach data analysis. By leveraging the scalable resources of the cloud, businesses can handle larger datasets and more complex analytical tasks without the need for significant capital investment in physical infrastructure. This shift has democratized data analytics, making it accessible to companies of all sizes.
Key services like AWS, Microsoft Azure, and Databricks have emerged as pivotal players in this transformation. They offer a range of solutions that integrate AI and data, viewing data as a strategic asset for rapid, data-driven decision-making. Here's a brief overview of the services provided:
- Cloud Data Analytics
- Data Management & Enablement
- Customer-centric analytics solutions
- Procurement strategy implementation
These services are not just about storage and computing power; they enable organizations to derive better business value from data. The cloud's ability to support advanced analytics techniques and tools facilitates insightful and rapid decision-making across various domains, including customer connectivity, supply chain optimization, and employee engagement.
Tip: When transitioning to cloud-based analytics, it's crucial to ensure that your data governance policies are adapted to the new environment to maintain data integrity and security.
The Importance of Data Warehousing and ETL Processes
Data warehousing and ETL processes play a crucial role in the analytics ecosystem, providing the foundation for efficient data management and analysis. The integration of data from disparate sources into a centralized data warehouse enables organizations to perform complex analytical queries and derive actionable insights. Additionally, ETL processes ensure data quality and consistency, laying the groundwork for reliable decision-making.
To illustrate the significance of data warehousing and ETL processes, consider the following table:
Data Warehousing and ETL Processes | Benefits |
---|---|
Centralized data storage | Improved data accessibility and security |
Data integration and transformation | Enhanced data quality and consistency |
Efficient data processing | Accelerated analytical insights |
Furthermore, businesses can benefit from the following key points:
- Implement a data-driven decision-making process
- Cultivate a data culture within the organization
- Ensure data privacy and security
In conclusion, embracing robust data warehousing and ETL processes is essential for organizations seeking to harness the full potential of analytics and make informed, data-driven decisions.
Analytics in Action: Industry Case Studies
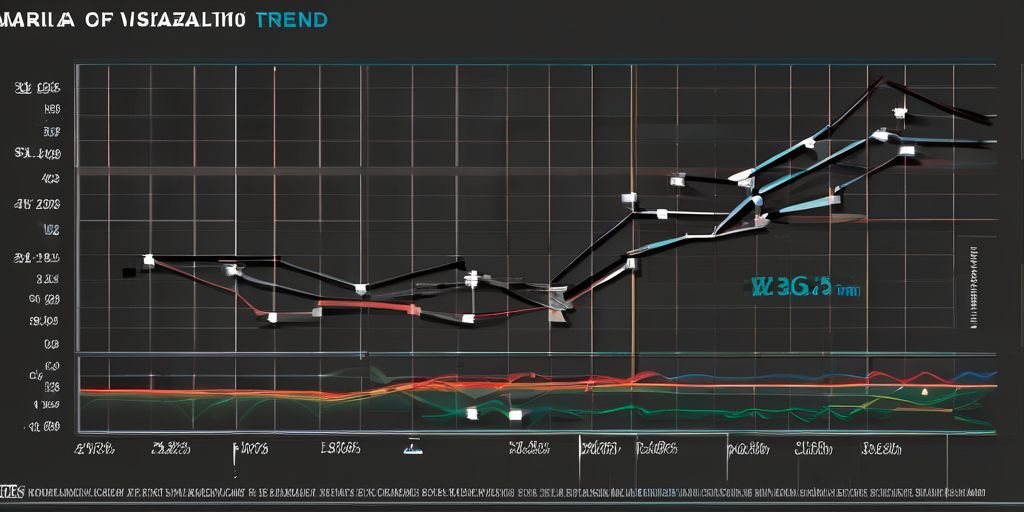
Retail Sector: Personalization and Customer Insights
Utilizing data analytics in the retail sector is crucial for customer retention, operational efficiency, and personalized marketing. Segmentation, behavior analysis, and personalized experiences are key drivers of business growth. For instance, discovering that a significant portion of website traffic originates from mobile users prompts optimization of the mobile platform, ensuring a seamless shopping experience for the majority. Responding to customer feedback expressing a growing interest in eco-friendly packaging not only meets expectations but positions the brand as environmentally responsible — a winning strategy. Additionally, data analytics facilitates refining pricing strategies, enhancing the shopping experience, and anticipating market trends. Are you prepared to transform your data into an invaluable ally for boosting sales? Let’s embark on this transformative journey!
Healthcare: Improving Patient Outcomes with Predictive Analytics
In the healthcare industry, predictive analytics plays a crucial role in improving patient outcomes and delivering better services. By leveraging data-driven insights, healthcare providers can anticipate patient needs, identify high-risk individuals, and personalize treatment plans. This proactive approach leads to more effective care, reduced hospital readmissions, and overall improvement in patient satisfaction. Implementing predictive analytics in healthcare involves analyzing historical patient data, identifying patterns, and using machine learning algorithms to predict future health outcomes. This enables healthcare professionals to intervene early, optimize resource allocation, and ultimately improve the quality of care provided to patients.
Key Benefits of Predictive Analytics in Healthcare:
- Early identification of high-risk patients
- Personalized treatment plans
- Reduced hospital readmissions
- Improved patient satisfaction
- Optimized resource allocation
By harnessing the power of predictive analytics, healthcare organizations can revolutionize patient care and drive positive outcomes for both patients and providers.
Finance: Risk Management and Fraud Detection
In the financial sector, the application of analytics for risk management and fraud detection has become a cornerstone of maintaining integrity and stability. Financial institutions leverage a variety of analytical tools to identify patterns that may indicate fraudulent activity and assess risks associated with lending, investments, and other financial transactions.
- Risk Advisory Services* and Forensics & Dispute Advisory Services play a critical role in this domain, offering expertise in navigating the complex regulatory environment and providing insights into financial data. These services encompass a range of solutions from Cybersecurity assessments to Transaction Advisory, ensuring comprehensive coverage against potential threats.
Tip: Always integrate risk management practices with regulatory compliance to ensure a robust defense against financial fraud.
The table below illustrates some of the key services that support risk management and fraud detection in finance:
Service Category | Description |
---|---|
Regulatory Compliance | Ensures adherence to financial laws and regulations |
Internal Audit | Evaluates the effectiveness of internal controls |
IT Audit & Consulting | Assesses technology risks and security measures |
Forensics & Dispute Services | Investigates financial discrepancies and litigations |
By harnessing the power of analytics, financial institutions can not only detect and prevent fraudulent activities but also gain a competitive edge through informed decision-making and strategic risk assessment.
The Human Element: Bridging the Gap Between Data and Decisions
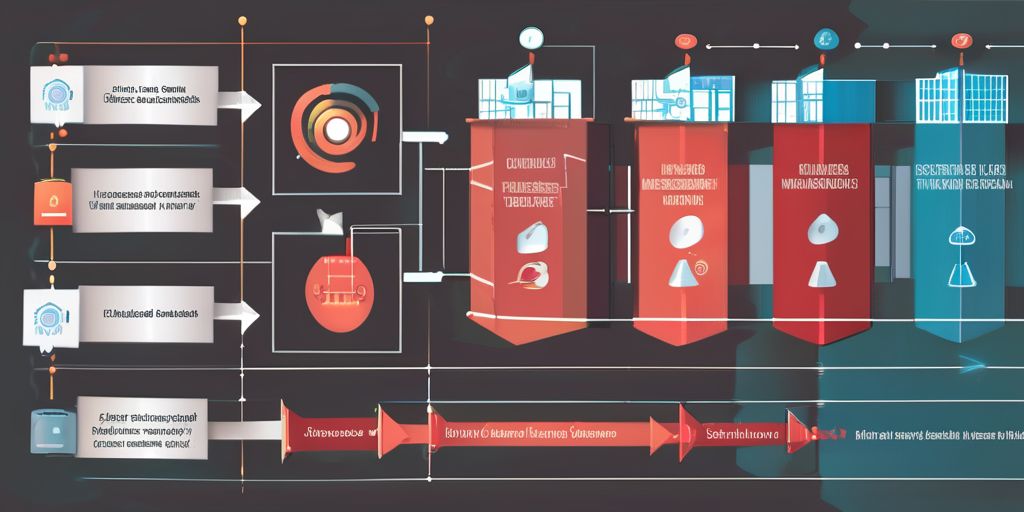
The Role of Data Scientists and Analysts
In the realm of analytics, data scientists and data analysts are the linchpins that convert complex data into actionable insights. While data analysts typically focus on interpreting structured data to address specific business issues, data scientists delve into larger datasets, often unstructured, to uncover patterns and predict future trends. Both roles are critical in guiding data-driven decision-making within organizations.
Data scientists and analysts must possess a unique combination of skills:
- Statistical expertise and knowledge of algorithms
- Proficiency in data manipulation and analysis tools
- Business acumen to understand industry-specific challenges
- Communication skills to convey complex findings in an understandable manner
Tip: The most effective data professionals are those who can not only analyze data but also communicate their findings to non-technical stakeholders, bridging the gap between data and strategic decisions.
Choosing the right data analytics consultant or firm is pivotal for businesses. The ideal partner should have a proven track record in delivering results and experience in handling data complexities akin to your own. It's not just about technical expertise; understanding the unique challenges and objectives of your industry is equally important.
Cultivating a Data-Driven Culture in Organizations
Becoming a data-driven business requires a fundamental shift in how organizations operate and make decisions. Here are some key steps to becoming a data-driven business: Start by identifying clear objectives that align with your business strategy. Ensure that the objectives you set are measurable and relevant to your overall goals. Foster a culture where data is valued and utilized throughout the organization. Encourage employees to seek data-driven solutions and provide training to enhance data literacy. Establish robust data collection mechanisms and integration. Develop data analytics capabilities and implement a data-driven decision-making process. With the right approach and tools, businesses can harness the power of data to drive growth and achieve their goals.
Ethical Considerations in Data Analysis and Interpretation
Ethical considerations in data analysis and interpretation are crucial for maintaining integrity and responsibility. It involves emphasizing values and ensuring the potential impact of data analysis is carefully considered. When dealing with ethical considerations, it's important to implement operational processes and procedural guidance to ensure the responsible use of data outputs. Developing actionable insight statements is essential, as they summarize the discoveries made during data analysis and provide understanding into the reasons behind the data. This ensures that data is not underutilized and that meaningful potential is realized. Collaboration across different teams within the organization is also vital for data-driven decision making, fostering a cross-functional effort involving various departments. Staying updated with the latest tools, techniques, and trends in data analytics is crucial for ensuring the effectiveness of data initiatives.
Visualizing Data for Better Understanding
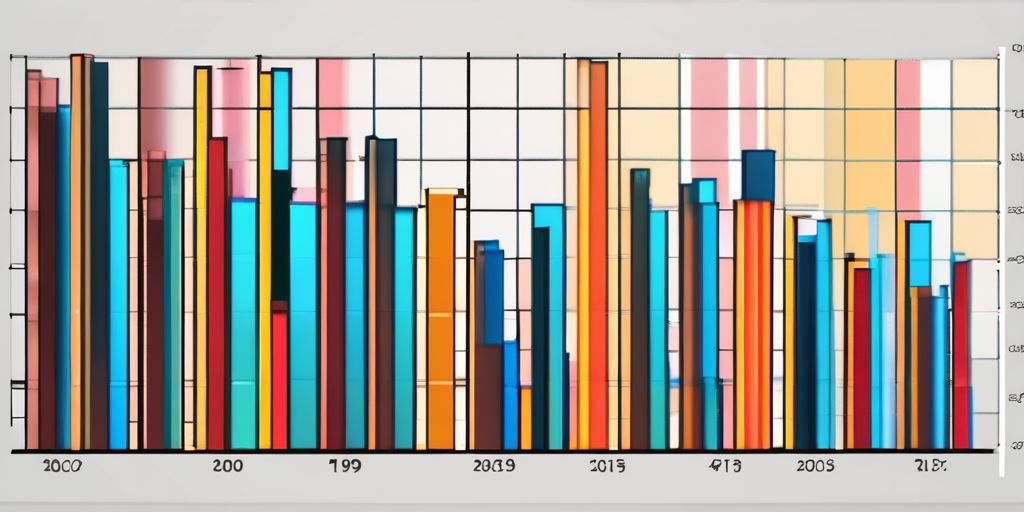
The Art and Science of Data Visualization
Visualizing data effectively is crucial for conveying insights to stakeholders and fostering a data-driven culture within the organization. Users can customize visual elements such as charts, graphs, and pivot tables to create interactive and engaging dashboards. To ensure accurate and impactful visualizations, it's important to clean raw data and place it in the right context. This involves scrubbing data for accuracy, eliminating manual entry errors, and ensuring completeness. Additionally, collaboration and sharing of reports and dashboards facilitate real-time decision-making and access to up-to-date information. Data visualization is an essential tool for uncovering patterns, trends, and correlations within data, providing a deeper understanding of business performance. DataArt and Zoho Analytics are two companies that offer advanced analytics features and visualization options to meet specific business needs.
Tools and Techniques for Effective Data Presentation
When presenting data, it's important to utilize data visualization tools to make complex data more accessible and understandable. Dashboards and reports can help stakeholders track KPIs and understand trends at a glance. Additionally, implementing a table for presenting structured, quantitative data can provide a clear and concise way to convey information. On the other hand, using a bulleted or numbered list can be effective for less structured content, such as steps, qualitative points, or a series of related items. Remember to keep the presentation clear and concise to ensure that the audience can easily grasp the insights being conveyed. Finally, continuous improvement is key, so continuously monitor and analyze results, and use feedback to refine strategies and adapt to changing conditions.
Case Studies: Impactful Data Visualizations
The use of web-based dynamic reporting capabilities, available through dashboarding, provided consistent reporting and facilitated analysis opportunities across a wide range of institutional stakeholders. Analytics are only as good as the data they come from. The system was able to identify gaps in their own data (e.g., a professor listed in course scheduling data, but not payroll data) and clean up their reporting. They learned what and how to measure, and what kind of custom reporting provided the most valuable insights—and could then make better, more data-driven decisions. Users can customize these visual elements to suit their needs, creating interactive and engaging dashboards. Visualizing data effectively is crucial for conveying insights to stakeholders and fostering a data-driven culture within the organization. Collaboration and Sharing: Zoho Analytics promotes collaboration by allowing users to share reports and dashboards with team members. This facilitates real-time collaboration and ensures that decision-makers have access to the most up-to-date information. Additionally, users can schedule automated report
Overcoming Common Challenges in Analytics
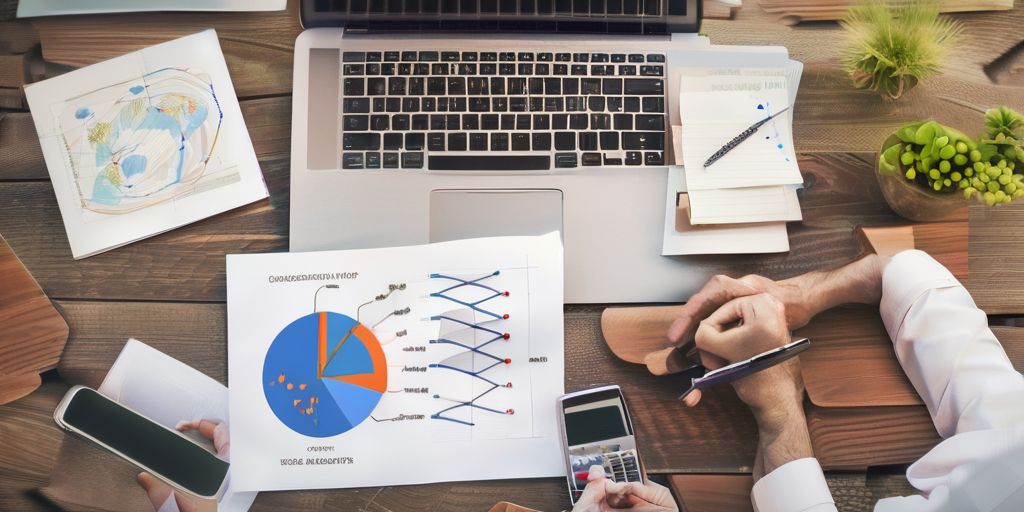
Dealing with Incomplete or Dirty Data
Raw data could be referred to as source data. It’s a direct, unprocessed export. This means it will need to be cleaned before it can resemble something actionable. Many organizations utilize data visualizations, such as dashboards, with descriptive charts or graphs. It’s important to remember that the sleek colors and flashy graphics are populated by raw data. Cleaning raw data and placing it in the right context are crucial steps to ensure that all visualizations are unaffected by junk or misplaced data entries. Clean data should not include inaccuracy, manual entry errors, be outdated, or contain incomplete documentation. Unfortunately, in the laboratory, we are often faced with process variability that leads to junk data creation. Context is what places data in the right position to generate actionable insights. Leaders should consider environmental factors, operational workflows, or process deviations that may impact the data. Recognizing where convenience has replaced or impaired standardization is critical. For example, staff may choose to type comments instead of utilizing standard, pre-built ones. In some cases, it may be necessary to fix an operational process or procedural guidance prior to utilizing any data outputs. Parameters should be set carefully to ensure accurate representation of metrics. Access to raw data is important because it allows leaders to trace back decisions and ascertain the context of data outputs.
Ensuring Data Privacy and Security
Data privacy and security are critical aspects of any analytics initiative. It is essential to comply with relevant regulations and implement robust security measures to protect sensitive data. One important aspect to consider is the ability to handle data securely and comply with data protection regulations. Additionally, verifying the use of advanced and appropriate tools and technologies is crucial for ensuring data security. Client testimonials and case studies can provide valuable insights into the expertise of analytics service providers. Clear communication channels and a collaborative approach are essential for seamless integration with your team. Post-implementation support is also a key factor to consider. To ensure long-term data retention and accessibility, archiving best practices should be implemented. Finally, staying updated with the latest tools, techniques, and trends in data analytics is crucial for maintaining the effectiveness of data initiatives.
Bridging the Skills Gap in Analytics Teams
To bridge the skills gap in analytics teams, it's essential to ensure clear communication channels and a collaborative approach for seamless integration with your team. Post-Implementation Support should be considered, evaluating the level of support offered after the implementation of data analytics solutions. Look for consultants with expertise in relevant fields such as data analytics, machine learning, and artificial intelligence. Here's a table summarizing the key steps:
Steps | Description |
---|---|
Clear Communication | Establish clear communication channels within the team |
Collaborative Approach | Foster a collaborative approach for seamless integration |
Post-Implementation Support | Evaluate the level of support offered after implementation |
Additionally, it's important to implement a data-driven decision-making process and identify relevant Key Performance Indicators (KPIs) to measure progress and success. Encouraging decision-makers to base their choices on data insights is crucial for bridging the skills gap in analytics teams. Remember, the expertise of data analytics consultants and consulting firms is invaluable for deciphering vast amounts of data and extracting actionable insights. Their blend of statistical expertise, business acumen, and technological prowess is crucial for staying ahead in competitive markets.
The Future of Analytics: Trends and Predictions
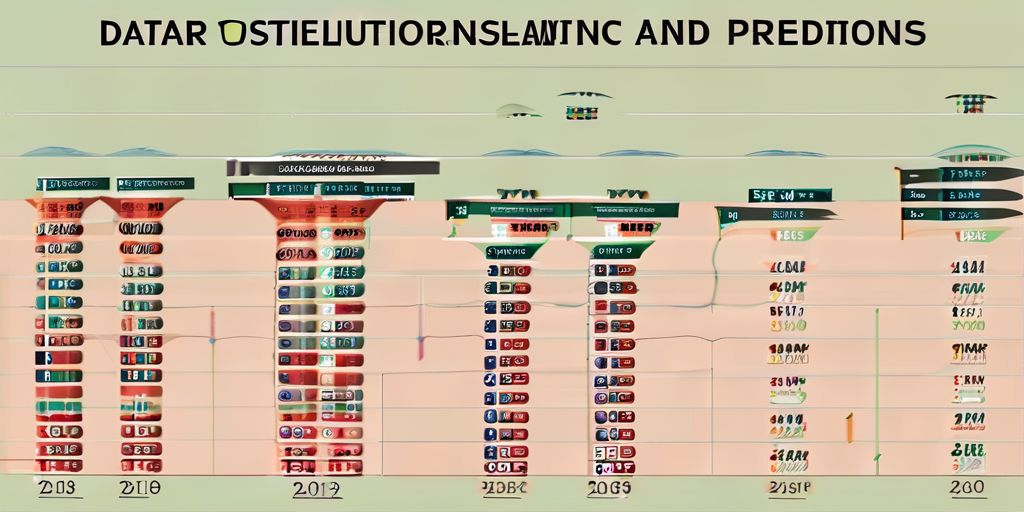
The Evolving Landscape of Analytics Technology
In 2024, there is an unprecedented emphasis on leveraging data to make informed decisions across diverse sectors. Predictive Analytics takes center stage, offering a glimpse into the future of data-driven decision-making. This transformative approach converges data management and analytics to create a tapestry of possibilities. Companies and organizations are utilizing data analytics to assess performance, understand customer motivation, and drive engagement and sales. The relentless pursuit of 'why' has dominated the analytics landscape, but now the focus shifts to the 'how' in analytics, paving the way for strategic foresight and sustained success. Join us on a journey where data transforms from a resource into a strategic asset.Embark on a transformative experience with SG Analytics’ Predictive Analytics Solutions. Elevate your business intelligence, embrace foresight, and chart a course towards sustained success in the digital era.
Predictive Analytics and the Internet of Things (IoT)
Predictive analytics plays a pivotal role in software design and project management, leveraging AI algorithms to optimize code, anticipate user needs, and make data-driven decisions for better resource allocation. This forward-looking approach enables businesses to identify trends, mitigate risks, and capitalize on opportunities with precision. Leveraging IoT data in predictive analytics enhances the ability to forecast outcomes and make proactive decisions. By integrating IoT data streams, businesses can gain real-time insights into operational efficiency, asset performance, and customer behavior. This convergence of predictive analytics and IoT opens up new possibilities for informed decision-making and strategic planning. Implementing a table to showcase the impact of IoT data on predictive analytics performance would provide a structured view of the benefits. Additionally, a bulleted list can highlight the key steps for integrating IoT data into predictive analytics processes. It's important to note that ethical considerations and data privacy should be prioritized when leveraging IoT data for predictive analytics. As businesses embrace the power of predictive analytics and IoT, it's essential to maintain a balance between innovation and ethical data usage. This balance ensures that the transformative insights gained from predictive analytics contribute to sustainable business growth and positive societal impact.
The Role of Analytics in Shaping Public Policy
In shaping public policy, analytics plays a crucial role in providing evidence-based insights for decision-making. By leveraging large-scale data analysis, policymakers can gain valuable insights into social trends, economic patterns, and public sentiment. This enables them to make informed decisions that have a positive impact on society. Additionally, analytics can help in identifying areas for policy improvement and resource allocation, leading to more effective governance and public service delivery. Furthermore, it facilitates the monitoring and evaluation of policy outcomes, ensuring accountability and transparency in governance.
Moreover, analytics in public policy can be used to:
- Analyze the impact of policy interventions on key societal indicators.
- Identify areas for targeted intervention and resource allocation.
- Forecast future scenarios based on current trends and patterns.
- Evaluate the effectiveness of public programs and initiatives.
By harnessing the power of analytics, policymakers can make data-driven decisions that address the complex challenges of modern governance and contribute to the well-being of the public.
Data-driven insights enable proactive policy-making and effective governance, fostering positive societal outcomes.
The future of analytics is an exciting and rapidly evolving landscape. As businesses continue to rely on data-driven insights, the demand for advanced analytics tools and techniques is on the rise. From machine learning and AI-powered analytics to real-time data processing and predictive modeling, the possibilities are endless. At OptimizDBA Database Optimization Consulting, we are at the forefront of these trends, helping businesses harness the power of analytics to drive growth and innovation. With our proven track record of delivering significant performance improvements, we guarantee a transformative experience for your database optimization needs. Experience the future of analytics with OptimizDBA and unlock the full potential of your data-driven strategy today!
The Power of Analytics: Transforming Data into Actionable Insights
In conclusion, the transformative potential of data analytics and predictive analytics is undeniable. By leveraging advanced analytics techniques and tools, businesses can gain valuable insights, forecast future scenarios, and optimize operations for maximum efficiency and profitability. The convergence of data management and analytics creates a tapestry of possibilities, illuminating the marketplace and propelling sales to unprecedented heights. Let's embark on this journey and transform raw data into lucrative opportunities!
Frequently Asked Questions
What is the role of analytics in business decision-making?
Analytics plays a crucial role in business decision-making by providing valuable insights and data-driven recommendations for strategic planning and operational improvements.
How does big data contribute to advanced analytics?
Big data contributes to advanced analytics by providing large and diverse datasets that enable the application of complex analytical models and algorithms for predictive and prescriptive analysis.
What are the key challenges in big data analytics?
Key challenges in big data analytics include data integration, data quality management, scalability of infrastructure, and ensuring data security and privacy.
Why is data visualization important in analytics?
Data visualization is important in analytics because it enables the clear and intuitive presentation of complex data, making it easier for stakeholders to understand and interpret the insights derived from analytical processes.
What skills are essential for a career in data analytics?
Essential skills for a career in data analytics include proficiency in statistical analysis, programming languages (such as Python or R), data visualization tools, and domain knowledge in specific industries.
How does predictive analytics benefit businesses?
Predictive analytics benefits businesses by forecasting future trends, identifying potential risks and opportunities, optimizing resource allocation, and enhancing decision-making processes based on data-driven predictions.
What ethical considerations are important in data analysis and interpretation?
Important ethical considerations in data analysis and interpretation include ensuring data privacy and confidentiality, avoiding bias in analytical models, and transparently communicating the implications of analytical insights to stakeholders.
What are the emerging trends in analytics technology?
Emerging trends in analytics technology include the integration of artificial intelligence and machine learning, the adoption of edge analytics for real-time processing, and the convergence of analytics with Internet of Things (IoT) devices for data collection and analysis.