Understanding Predictive Analytics
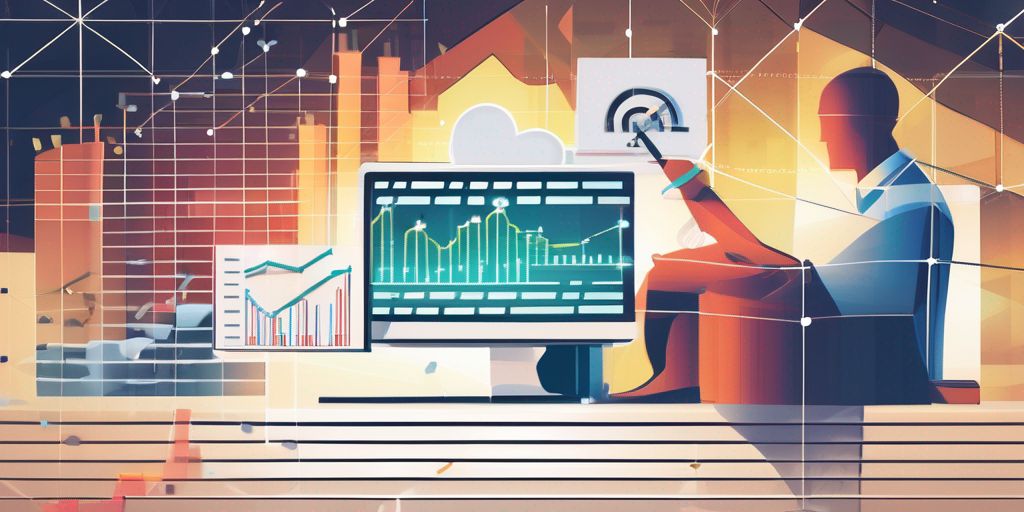
Defining Predictive Analytics
Predictive analytics uses data, statistical algorithms, AI, and machine learning techniques to identify the likelihood of future outcomes based on historical data. It's a bit like a weather forecast for your business, helping you predict sunny days and stormy periods. Predictive analytics helps businesses anticipate customer needs, manage risks, and tailor marketing campaigns so they hit the mark every time. But here's the catch: it's not foolproof. Like any forecast, it's based on probabilities. There's always a margin of error, but it's still much better
Applications in Business
Predictive analytics has become a cornerstone for driving business growth, enabling organizations to anticipate market trends, customer behaviors, and potential risks. By leveraging historical data, businesses can forecast future outcomes with a significant degree of accuracy, informing strategic decisions across various departments.
In the realm of business analytics (BA), predictive models are applied to a wide array of functions, including financial planning, HR management, and operational efficiency. For instance, predictive analytics can enhance financial forecasting, optimize HR processes like applicant tracking, and improve supply chain management through predictive maintenance.
Here are some key applications in business:
- Anticipating customer needs and preferences to tailor marketing strategies
- Identifying potential risks and fraud in financial transactions
- Streamlining operations to reduce costs and increase process efficiency
- Enhancing product development by predicting market acceptance
- Improving employee retention through predictive insights on turnover
Tip: Integrating predictive analytics into business processes requires a clear understanding of organizational goals and a commitment to data-driven decision-making.
Challenges and Limitations
While predictive analytics can be a powerful tool for driving business growth, it comes with its own set of challenges and limitations that organizations must address. One significant challenge is the data quality and completeness necessary for accurate predictions. Without high-quality data, the output of predictive models can be misleading, leading to poor decision-making.
Another limitation is the complexity of creating and maintaining predictive models. These models require expertise in data science and a deep understanding of the business context to be effective. Additionally, the integration of predictive analytics into existing business processes can be a daunting task, often requiring substantial changes to workflows and systems.
Lastly, ethical considerations such as data privacy, bias, and transparency cannot be overlooked. Organizations must ensure that their use of predictive analytics aligns with legal requirements and ethical standards to maintain trust and avoid potential backlash.
Tip: Start small and scale gradually. Begin with a pilot project to understand the intricacies of predictive analytics and to build the necessary infrastructure before expanding its use across the organization.
Implementing Predictive Analytics
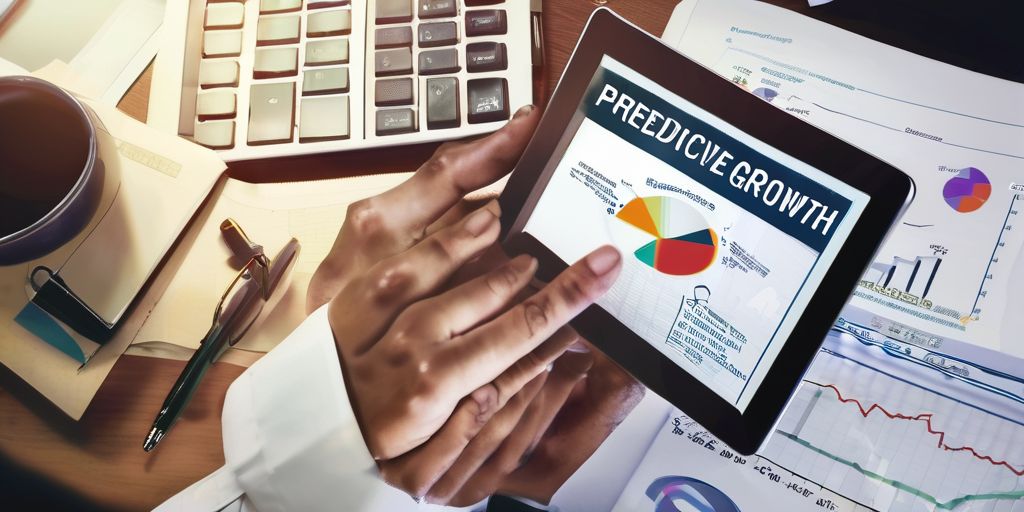
Data Collection and Preparation
The foundation of predictive analytics is the data that fuels it. The initial phase of data collection and preparation is akin to gathering ingredients for a recipe. Businesses must first identify the data sources—ranging from transaction records and customer demographics to social media interactions and sensor data.
Once the data is collected, the next critical step is cleaning and prepping it. This involves removing inconsistencies, correcting errors, and formatting the data uniformly. It's essential to select only the relevant data points that will influence the predictions. Here's a simple list to guide the process:
- Gather diverse data points to enrich the predictive model.
- Cleanse the data to eliminate errors and outliers.
- Transform and format data for consistency.
- Select relevant features that will drive accurate predictions.
Tip: Always question the relevance of each data point to the predictive outcome you seek. Irrelevant data can lead to misleading predictions and wasted resources.
Choosing the right tools and techniques for data preparation is also crucial. It sets the stage for developing a robust predictive model tailored to the specific needs of the business.
Model Development
Once the data is collected and prepared, the focus shifts to model development. This phase is critical as it involves selecting the right predictive model that aligns with the data characteristics and the specific predictions desired. For instance, regression models are often utilized for forecasting sales, while decision trees may be better suited for customer segmentation.
The development process includes training the model on a subset of the data, which allows it to learn and identify patterns. It is then tested on a separate data set to assess its performance. This step is crucial for validating the model's accuracy and ensuring it can generalize well to new data.
Different types of models serve various purposes:
- Classification Models: Sort objects into categories for targeted marketing or personalized experiences.
- Regression Models: Explore relationships between variables to predict outcomes.
- Clustering Models: Group similar entities to reveal customer segments.
- Time Series Models: Analyze data over time to forecast trends and patterns.
Tip: Always ensure that the model is not only accurate but also interpretable to stakeholders. The ability to explain a model's predictions is essential for trust and adoption within a business setting.
Integration with Business Processes
Predictive analytics plays a crucial role in integrating with business processes, enabling businesses to optimize efficiency, predict customer behavior, forecast sales, and mitigate risks to drive growth and success. It provides capabilities for critical thinking and obtaining insights to improve business activity monitoring, inter-departmental collaboration, process optimization, and workflow optimization. This leads to the identification of errors, weaknesses, and potential areas for improvement, as well as future scenario prediction (forecasting) and increased customer knowledge. Additionally, it aids in defining buyer personas and target customers, identifying business possibilities, and developing market initiatives. Implementing predictive analytics also contributes to increasing client satisfaction, optimizing existing markets, and reorienting business strategy to adapt to a volatile and unpredictable market, ultimately boosting return on investment.
Measuring Predictive Analytics Success
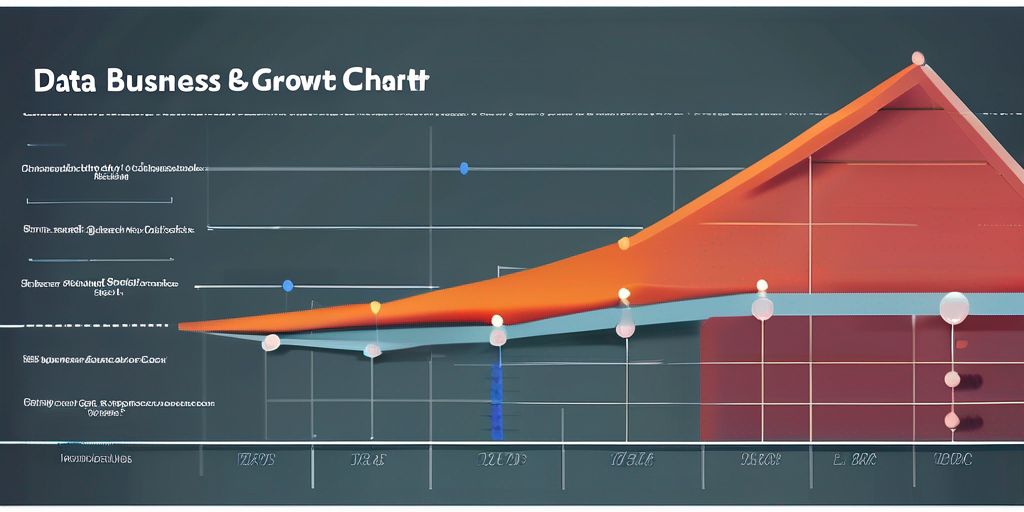
Key Performance Indicators
Predictive analytics success can be measured using a variety of key performance indicators (KPIs) that reflect the impact and effectiveness of predictive models. One important KPI is the accuracy of predictions, which can be measured using metrics such as precision, recall, and F1 score. Additionally, the business impact of predictive analytics can be evaluated through metrics like return on investment (ROI) and cost savings. To track the continuous improvement of predictive analytics, businesses can create a scorecard that includes KPIs and benchmarks for comparison over time. This structured approach allows for data-driven insights and informed decision-making.
Evaluating Business Impact
Predicting business impact is crucial for evaluating the success of predictive analytics. It involves aligning analytics goals with business objectives, establishing metrics for success, and collecting/analyzing relevant data. To effectively measure success, businesses can optimize marketing and sales efforts by tracking KPIs and tailoring products or services. Predictive risk analytics provides the capability to identify potential pitfalls and navigate them with solid data. In this context, a hypothetical health and wellness app's business case illustrates the importance of analyzing user retention rates and revenue to make informed decisions for business growth.
Continuous Improvement
The journey of continuous improvement in predictive analytics is an ongoing process that requires dedication and a strategic approach. It's not about transforming your business overnight but about making incremental changes that accumulate to significant advancements. To ensure success, start with small, manageable projects and gradually scale up.
Ongoing training and development are crucial for teams to effectively utilize predictive analytics tools and interpret results. This knowledge should be integrated into strategic decision-making, fostering a culture where data-driven insights are paramount.
Predictive models require regular reviews and adjustments to remain relevant. As your business and external conditions change, so should your models. This iterative process helps maintain accuracy and adaptability.
Always be mindful of privacy and consistently drive improvements, balancing efficiency with the flexibility to meet evolving customer demands and market conditions.
Ethical Considerations in Predictive Analytics
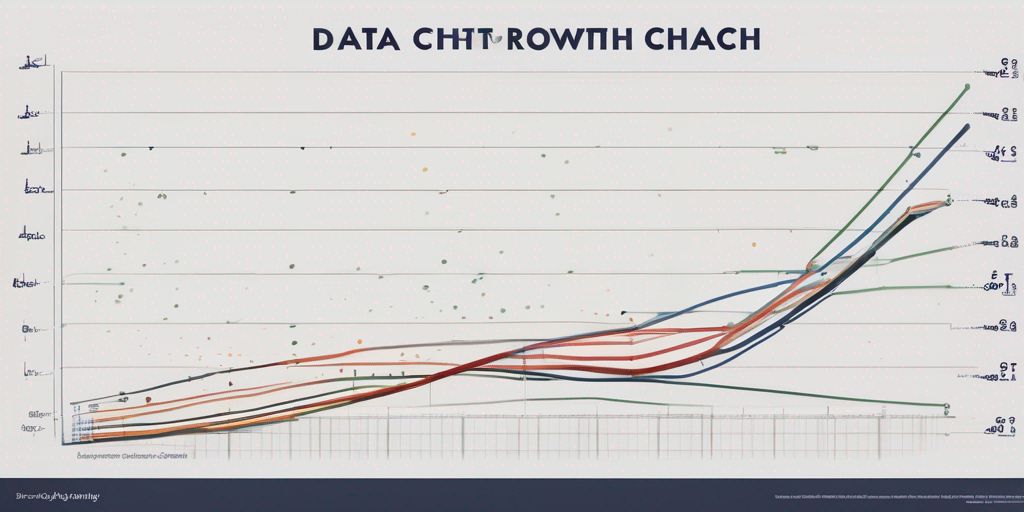
Data Privacy and Security
As new data emerges, businesses must prioritize data accuracy, governance, and ethical considerations. Use analytics for innovation and product optimization. Transparency and accountability are crucial for ethical data practices. Challenges of big data analytics include complying with industry regulations like the GDPR and CCPA and enforcing organization-level security protocols while managing vast volumes. Techniques like data anonymization and pseudonymization can help protect individual identities while making data available for analysis. Organizations need to be transparent about how they collect, use and store data. SageMaker, Alteryx, Dataiku, Spark and H2O.ai are some predictive analytics platforms. Refer to our Predictive Analytics
Bias and Fairness
Predictive analytics raises ethical concerns related to privacy, data protection, and fairness. For instance, using predictive models to make decisions about individuals' creditworthiness or job applications may lead to biased outcomes. Businesses need to ensure that they adhere to ethical guidelines and regulations while using predictive analytics and take steps to mitigate any potential biases.
In conclusion, while predictive analytics offers significant benefits for businesses, it is essential to address the challenges associated with its implementation. By overcoming data quality issues, building a skilled workforce, and considering ethical implications, businesses can harness the power of predictive analytics to gain valuable insights and drive growth.
- Implement a table for presenting structured, quantitative data. Ensure it's succinct and formatted correctly in Markdown.
- Use a bulleted or numbered list for less structured content, like steps, qualitative points, or a series of related items.
Businesses must prioritize ethical considerations and regulatory compliance when implementing predictive analytics to ensure fair and unbiased outcomes.
Transparency and Accountability
Transparent and responsible big data practices build trust and protect your business. Keep track of how predictive analytics impacts your business. This not only justifies the investment but also guides future initiatives. Before anything else, know what you want to achieve. Are you looking to improve customer retention, increase sales, optimize operations, or something else? The accuracy of your predictions hinges on the quality management of your data. Ensure you collect comprehensive, relevant, and high-quality data from various sources. There's a plethora of must navigate through various regulatory and legal frameworks that govern their operations. Compliance with corporate governance standards, financial reporting requirements, and competition laws becomes essential. Organizations need to invest in robust legal and compliance frameworks to ensure adherence to these regulations. Balancing Public Interests: Corporatization may lead to a shift in priorities, with a greater emphasis on financial performance and profitability. While this can enhance efficiency, it is essential to strike a balance between the organization's financial process optimization, and waste elimination. Increased Accountability: By allocating costs to specific activities or departments, organizations can hold individuals or teams accountable for their expenditure. This promotes a culture of responsibility and cost-consciousness. Challenges: Complexity: Cost assessment can be a complex process, especially in organizations with multiple cost centers, departments, or products. Determining the appropriate cost allocation methods, bases, and drivers requires careful analysis and consideration. Data Availability: Accurate
Predictive Analytics in Marketing
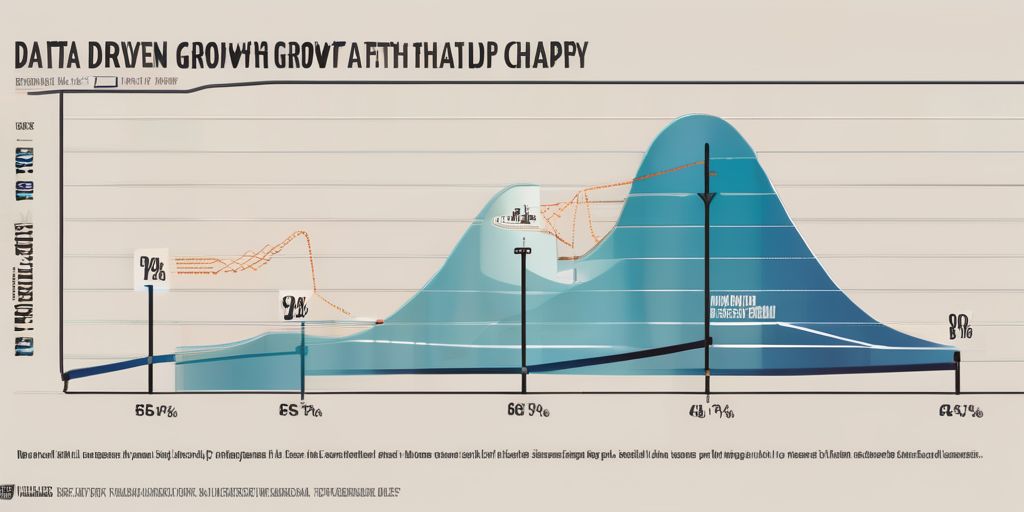
Customer Segmentation
In the realm of marketing, predictive analytics plays a pivotal role in the segmentation of customers. By analyzing patterns in customer behavior, preferences, and engagement, businesses can create highly targeted segments. This segmentation enables marketers to tailor their strategies and communications to meet the specific needs and interests of different customer groups, leading to enhanced personalization and increased conversion rates.
Customer segmentation is not just about grouping similar customers; it's about understanding the nuances that drive their decisions. For instance, predictive models can identify which customers are most likely to respond to a particular campaign, which are at risk of churning, and which may be more receptive to upselling or cross-selling opportunities.
Tip: Leveraging predictive analytics for customer segmentation can significantly improve the efficiency of marketing resources by focusing efforts where they are most likely to yield results.
Here's how predictive analytics can transform customer segmentation:
- Clustering: Grouping customers based on similar attributes and behaviors.
- Trend Analysis: Identifying patterns over time to predict future behaviors.
- Predictive Scoring: Assigning scores to customers based on their likelihood to take certain actions.
- Micro-Segmentation: Creating very specific segments for ultra-targeted marketing campaigns.
Personalization Strategies
Predictive Analytics for personalization strategies is about leveraging data to create a shopping experience as unique as your customers. It's a powerful tool to increase customer engagement, loyalty, and bottom line. It's selling savvy.
- Implement a table for presenting structured, quantitative data. Ensure it's succinct and formatted correctly in Markdown.
Predictive models can help understand and segment customers based on their behavior, preferences, and likelihood to engage or convert. This enables personalization at scale, improving the relevance of marketing efforts for each customer and leading to better engagement and conversion rates.
- Use a bulleted or numbered list for less structured content, like steps, qualitative points, or a series of related items.
By identifying the characteristics of customers who are likely to churn, predictive modeling allows for proactive customer retention strategies. This can significantly reduce churn rates.
- Optionally, include a Markdown blockquote for important tips or advice, avoiding citing sources.
Regarding sales strategies, predictive analytics is your ace in the hole. It helps forecast future sales trends, identify potential new markets, or suggest the most effective sales channels.
Campaign Optimization
AI plays a crucial role in content marketing by identifying trending topics, optimizing SEO, and predicting content performance. It also enhances cross-selling and upselling by analyzing real-time customer data to suggest relevant complementary products, thereby increasing the average purchase value and improving the customer shopping experience. Additionally, AI predicts ad performance, enabling marketers to optimize their campaigns effectively.
- Implement a table for presenting structured, quantitative data. Ensure it's succinct and formatted correctly in Markdown.
AI in Marketing | Benefits |
---|---|
Content Marketing | Identifying trending topics, optimizing SEO, predicting content performance |
Cross-selling and Upselling | Analyzing real-time customer data, suggesting complementary products |
Ad Performance Prediction | Predicting ad performance, optimizing campaigns |
- Use a bulleted or numbered list for less structured content, like steps, qualitative points, or a series of related items.
- AI aids in content marketing by identifying trending topics, optimizing SEO, and predicting content performance.
- AI-driven tools enhance cross-selling and upselling by analyzing real-time customer data to suggest relevant complementary products.
- AI predicts ad performance, enabling marketers to optimize their campaigns effectively.
AI-driven tools can generate content outlines and recommend distribution strategies, making content creation more efficient and effective.
AI in marketing harnesses historical and incoming data to predict market changes, eliminate high-risk strategies, and optimize marketing campaigns.
Predictive Analytics in Finance
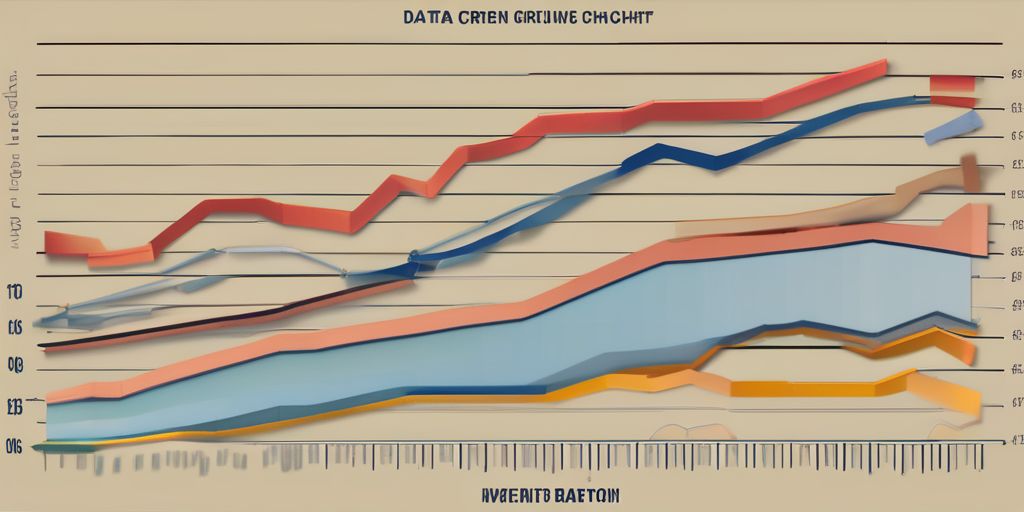
Risk Management
Financial services use predictive analytics in risk management to assess the creditworthiness of borrowers, reducing the risk of defaults. By identifying patterns that indicate fraudulent activity, businesses preemptively address these risks. Predictive analytics forecasts potential disruptions, allowing businesses to mitigate these risks in advance. Organizations need to carefully evaluate the regulatory landscape and ensure they have the necessary expertise to meet the requirements. Another challenge associated with risk management is the long-term commitment required. Risk transfer solutions, such as captive insurance or risk retention groups, allow businesses to tailor their coverage to specific risks and gain a more comprehensive protection plan. Cost reduction is another significant advantage of alternative risk transfer mechanisms, as organizations can eliminate unnecessary coverage and associated expenses. Interest rate risk management is crucial for banks to navigate the changing landscape and mitigate potential losses. Regulatory compliance is also a key consideration for financial institutions to ensure they meet the necessary requirements. Overall, predictive analytics plays a vital role in risk management by providing insights that enable businesses to make informed decisions and mitigate potential risks.
Fraud Detection
In the world of finance, AI is a game-changer for fraud detection. It analyzes transaction patterns in real-time to spot anomalies that could indicate fraud. This is crucial in an era where digital transactions are predominant, as it helps in quickly flagging and preventing fraudulent activities, thereby safeguarding both the institution and its customers. Credit scoring: AI improves credit scoring models by analyzing traditional and non-traditional data, enabling more accurate risk assessments. Investment strategies: AI assists in developing investment strategies by predicting market trends and identifying investment opportunities. Manufacturing Predictive maintenance: AI in manufacturing is primarily focused on predictive maintenance. By continuously monitoring equipment data like vibration, temperature, and operational metrics, AI can serve as an early warning system, alerting businesses to potential risks or deviations from expected expense patterns. This enables timely intervention and corrective actions to mitigate risks and prevent financial losses.
Financial Forecasting
Financial forecasting through predictive analytics offers a strategic advantage by providing foresight into potential financial scenarios. By analyzing historical data and current market trends, businesses can anticipate future revenue, costs, and other financial metrics with greater accuracy. This forward-looking approach enables companies to plan more effectively for growth, investments, and risk management.
For instance, consider the application of predictive analytics in anticipating inventory expenses. A retail company might use predictive models to determine which products will see increased demand, thereby optimizing inventory levels and reducing the risk of overstock. This not only ensures the availability of in-demand products but also contributes to more efficient capital allocation.
Predictive analytics also plays a crucial role in identifying potential financial risks before they materialize. By examining patterns and trends, companies can take preemptive measures to mitigate risks, such as adjusting credit policies or setting aside reserves for anticipated losses. The table below illustrates how predictive analytics can impact various financial forecasting aspects:
Aspect | Impact of Predictive Analytics |
---|---|
Revenue Forecasting | Enhances accuracy of future revenue predictions |
Expense Forecasting | Identifies potential cost-saving opportunities |
Risk Assessment | Aids in early detection and mitigation of financial risks |
Tip: Always cross-validate your predictive models with actual outcomes to refine their accuracy over time. This practice helps in continuously improving the reliability of your financial forecasts.
Predictive Analytics in Operations
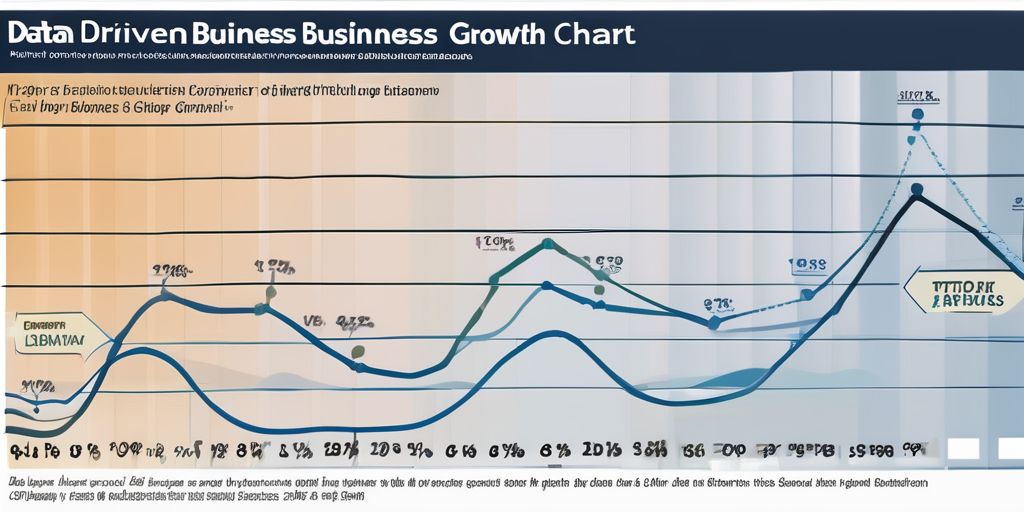
Supply Chain Optimization
Supply chain optimization is a critical aspect of business operations, ensuring efficient management of inventory levels and resource allocation. With predictive modeling, businesses can optimize their resource allocation by accurately forecasting their expenses, identifying areas for cost reduction, and streamlining operations. This ultimately leads to maximizing profitability. Retailers are using AI to elevate customer experience by analyzing consumer preferences and behaviors, offering personalized recommendations, and targeted promotions. Manufacturers with complex supply chains face challenges in streamlining processes and eliminating waste, requiring effective communication and collaboration. Lean principles emphasize the importance of continuous improvement, which requires a commitment to ongoing training and monitoring. Modern data tools provide the complete picture by combining data from various departments, leading to improved efficiency and shared insights. AI's adaptability and learning capabilities make it an invaluable tool in the modern business environment, aiding in strategic planning, risk management, and optimization of routes and inventory levels based on predictive demand.
Predictive Maintenance
Predictive maintenance is revolutionizing the way businesses approach equipment management. By leveraging advanced analytics, companies can anticipate equipment failures before they happen, scheduling maintenance proactively to avoid costly downtime. This not only enhances productivity but also extends the lifespan of machinery.
The process involves continuous monitoring of equipment data, such as vibration and temperature, to detect anomalies that may indicate impending issues. With predictive analytics, maintenance can be performed just in time, before problems escalate into major repairs or operational halts.
Tip: Regularly updating predictive models and ensuring data quality are key to maintaining the accuracy and effectiveness of predictive maintenance strategies.
The benefits of this approach are clear, with the predictive maintenance market experiencing significant growth. It's a testament to the value businesses place on operational efficiency and cost savings.
Process Efficiency
In the realm of operations, predictive analytics plays a pivotal role in enhancing process efficiency. By leveraging historical data and predictive models, businesses can anticipate future demands and adjust their processes accordingly. This proactive approach minimizes waste and optimizes resource allocation, leading to significant cost savings and improved operational performance.
For instance, predictive analytics can forecast production needs, allowing for just-in-time inventory management which reduces excess stock and associated holding costs. Similarly, in the service industry, it can predict peak periods, enabling better staff scheduling and customer service.
Key benefits of predictive analytics in process efficiency include:
- Reduction in operational costs
- Enhanced resource utilization
- Improved customer satisfaction through timely service delivery
- Increased agility in responding to market changes
Tip: Always validate predictive models with real-world data to ensure accuracy and relevance to your specific business context.
The Future of Predictive Analytics
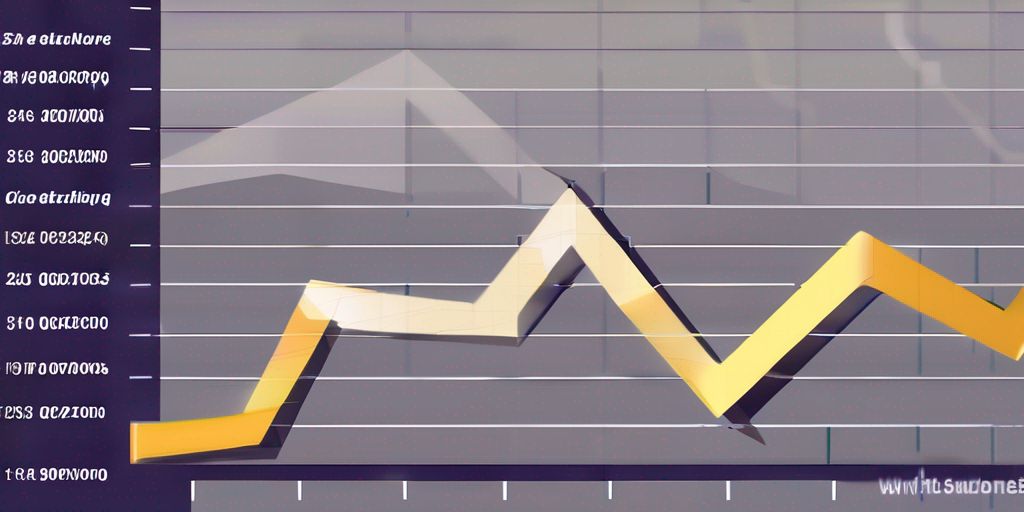
Emerging Technologies
The landscape of predictive analytics is continually reshaped by emerging technologies that enhance its capabilities and applications. Machine learning (ML) and artificial intelligence (AI) are at the forefront, driving the precision and efficiency of predictive models to new heights. The integration of these technologies allows for more nuanced and sophisticated analysis, enabling businesses to anticipate market trends and customer behaviors with greater accuracy.
Cloud computing has democratized access to powerful analytics tools, making them more accessible to businesses of all sizes. This shift is particularly beneficial for small and medium-sized enterprises (SMEs) that previously may have found the cost of advanced analytics prohibitive. Similarly, the proliferation of the Internet of Things (IoT) and edge computing is opening up new avenues for real-time data analysis and forecasting, critical for operations and strategic decision-making.
As decision intelligence becomes more prevalent, businesses will need to adapt to a landscape where real-time market responses and buyer-centric predictions are not just advantageous but expected.
The future of predictive analytics also points towards the importance of no-code/low-code platforms and self-service Business Intelligence (BI) tools. These advancements are set to empower a wider range of individuals to engage with data analytics, fostering a culture of evidence-based thinking and an analytics mindset across organizations.
Industry Trends
In an era where data is the new gold, businesses across the spectrum are turning to predictive analytics to not just survive but thrive. As decision intelligence comes into focus, real-time market response will drive business agility. AI-related data security concerns will overshadow the attempt to foster user trust with explainable AI. No-code/low-code platforms will enable more people to participate in data tasks. Self-service BI with automated data storytelling will make a formidable combination. Cloud services will offer access to powerful analytics tools, including forecasting systems. Multicloud deployments are widespread, with over 98% of public.
Impact on Business Strategy
The future of predictive analytics has a profound impact on business strategy, requiring organizations to adapt to a volatile and unpredictable market. This adaptation is crucial for boosting Return on Investment (ROI) and mitigating risks. Additionally, predictive analytics introduces change into an organization, assisting in the management of new business models. It also plays a key role in identifying and establishing business requirements, as well as efficiently communicating with company leaders or stakeholders. Furthermore, predictive analytics aids in defining business issues and developing organizational solutions, providing a comprehensive and dependable picture of the organization and all its aspects. Critical thinking capabilities are enhanced, leading to improved business activity monitoring, inter-departmental collaboration, process optimization, and workflow optimization. It also helps in identifying errors, weaknesses, and potential areas for improvement, as well as predicting future scenarios and increasing customer knowledge. These insights are essential for defining buyer personas, identifying business possibilities, and developing market initiatives. Ultimately, predictive analytics contributes to increasing client satisfaction and optimizing existing markets, thereby reorienting business strategy to meet the demands of the evolving market landscape.
The future of predictive analytics is an exciting and rapidly evolving field. As businesses continue to rely on data-driven decision-making, the role of predictive analytics becomes increasingly crucial. At OptimizDBA Database Optimization Consulting, we understand the importance of harnessing the power of predictive analytics to drive business success. Our expert team is dedicated to optimizing database performance and leveraging predictive analytics to achieve unparalleled transaction speeds. With our proven track record and industry-leading remote DBA services, we guarantee a significant increase in performance. Experience transaction speeds that are at least twice as fast as before, and often 100 times, 1000 times, or even higher! Join over 500 satisfied clients who have benefited from our trusted database optimization services since 2001. Take the next step towards maximizing your database performance and achieving unparalleled business success with OptimizDBA Database Optimization Consulting.
Conclusion
In conclusion, as companies march towards a digitized future, the strategic application of predictive analytics is becoming increasingly vital. By embracing the methodologies and tools that predictive analytics offers, businesses can gain a competitive edge, make informed decisions, and set themselves on a path of growth and innovation. As we witness more success stories across industries, it is clear that those who harness the power of predictive analytics effectively are the ones who will lead the way in the business world of tomorrow.
Frequently Asked Questions
What is predictive analytics and how does it work?
Predictive analytics is the use of data, statistical algorithms, and machine learning techniques to identify the likelihood of future outcomes based on historical data. It works by analyzing patterns and trends in data to make predictions about future events or behaviors.
What are the key applications of predictive analytics in business?
Predictive analytics is widely used in business for applications such as forecasting sales, market trends, and customer behavior, optimizing inventory levels, risk management, fraud detection, supply chain optimization, and process efficiency.
What are the main challenges and limitations of predictive analytics?
Some of the main challenges and limitations of predictive analytics include data privacy and security concerns, bias and fairness in predictive models, transparency and accountability in decision-making, and the complexity of integrating predictive analytics with existing business processes.
How is the success of predictive analytics measured in business?
The success of predictive analytics in business is measured through key performance indicators (KPIs) such as accuracy of predictions, improvement in decision-making, business impact evaluation, and continuous improvement in predictive models and processes.
What ethical considerations are important in the use of predictive analytics?
Important ethical considerations in the use of predictive analytics include ensuring data privacy and security, addressing bias and fairness in predictive models, and maintaining transparency and accountability in decision-making processes.
How is predictive analytics used in marketing?
Predictive analytics is used in marketing for customer segmentation, personalization strategies, campaign optimization, and targeted marketing based on predictive insights about consumer behavior and preferences.
What role does predictive analytics play in finance?
In finance, predictive analytics is used for risk management, fraud detection, financial forecasting, credit risk assessment, and optimizing financial processes based on predictive insights.
How does predictive analytics contribute to operational efficiency?
Predictive analytics contributes to operational efficiency by optimizing supply chain management, enabling predictive maintenance of equipment and assets, and improving process efficiency based on predictive insights and data-driven decision-making.