The Evolution of Data Storytelling in Business Intelligence
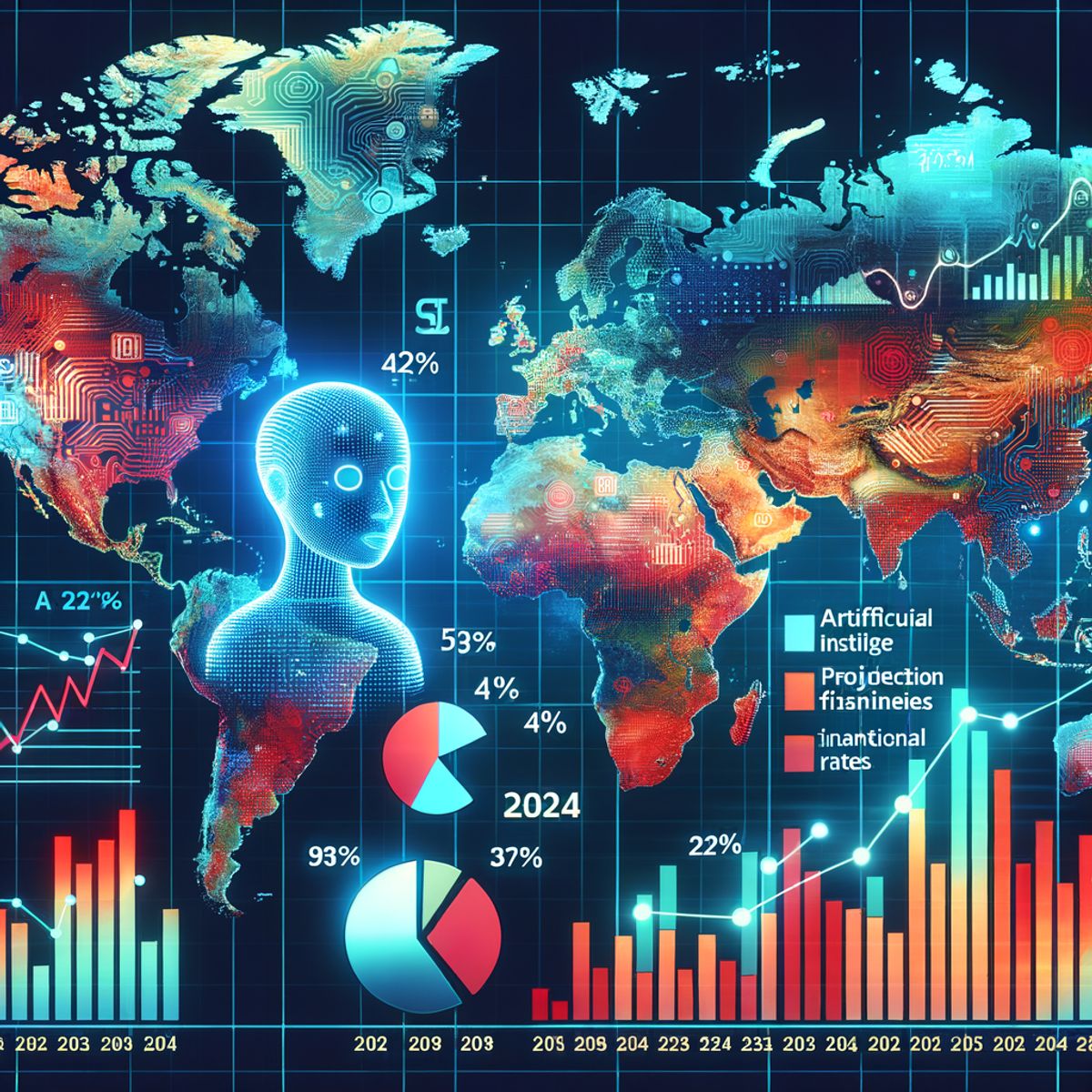
The Rise of Narrative Analytics
Narrative analytics is transforming the landscape of business intelligence by adding a layer of context to the raw numbers. Businesses are recognizing the power of stories to make data more relatable and actionable. Instead of merely presenting figures, narrative analytics weaves data into a compelling story, making it easier for stakeholders to understand and engage with the insights.
- Narrative analytics emphasizes the importance of context in data interpretation.
- It enables a more engaging and relatable presentation of data.
- Businesses are adopting storytelling to foster data-driven conversations.
Narrative analytics is not just about the data or the visuals; it's about crafting a story that resonates with the audience and drives action.
The adoption of narrative analytics is evident in the increasing number of businesses that are integrating storytelling principles into their BI processes. This trend is supported by data literacy campaigns that aim to make data understandable and useful for everyone within an organization.
Integrating Storytelling with BI Tools
Integrating storytelling with BI tools is more than just a trend; it's a transformative approach that turns raw data into compelling narratives. Business intelligence platforms are now expected to provide not only data but also the context and narrative that make the data meaningful. This integration allows businesses to communicate insights in a way that is both understandable and actionable for decision-makers.
Storytelling in BI tools is achieved through a combination of data visualization, narrative structures, and contextual frameworks. Here are some key aspects of this integration:
- Enhanced data visualizations that tell a story beyond the numbers
- Narrative frameworks that guide the user through the data discovery process
- Contextual layers that provide background and relevance to the data
By embedding storytelling into BI tools, organizations can ensure that their data is not just seen but also understood and acted upon.
As the integration of storytelling with BI tools evolves, it is crucial to monitor how this affects the analytical process and the way insights are communicated. The table below highlights the key takeaways of this trend:
Aspect | Impact on BI |
---|---|
Data Visualization | Makes complex data more accessible |
Narrative Frameworks | Guides interpretation and fosters engagement |
Contextual Relevance | Ensures insights are actionable and relevant |
Case Studies: Successful Data Storytelling Examples
The transformative power of data storytelling is evident in numerous organizations that have harnessed it to drive decision-making and strategic initiatives. One remarkable example is a retail giant that utilized narrative analytics to optimize its supply chain, resulting in a significant reduction in inventory costs. The company's analysts presented their findings through a compelling story that outlined the journey from data collection to actionable insights, which resonated with stakeholders and prompted swift action.
Another case study involves a financial services firm that integrated storytelling into its BI tools, enabling advisors to convey complex investment strategies through relatable scenarios. This approach not only improved client understanding but also fostered trust and engagement.
The essence of data storytelling lies in its ability to transform abstract numbers into relatable narratives, thereby enhancing comprehension and driving business value.
The following table summarizes key outcomes from these case studies:
Company Type | Challenge Addressed | Outcome Achieved |
---|---|---|
Retail | Supply Chain Optimization | Reduced Inventory Costs |
Financial Services | Client Engagement | Improved Trust and Understanding |
Advancements in Data Governance Strategies
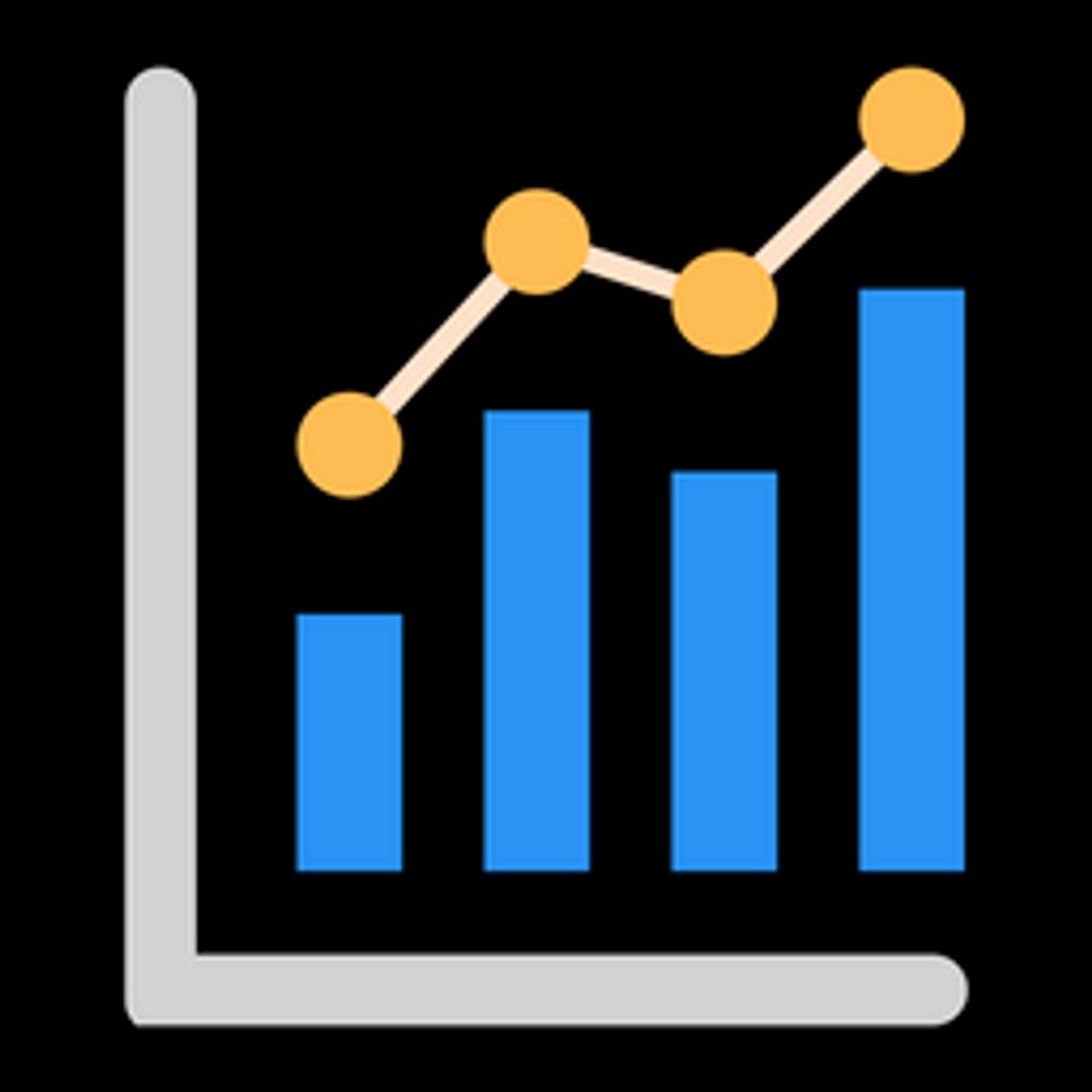
New Frameworks for Data Privacy and Security
As the digital landscape evolves, new frameworks for data privacy and security are becoming essential for businesses to maintain trust and compliance. The enactment of regulations like the General Data Protection Regulation (GDPR) has set a precedent, compelling companies to prioritize data governance and implement robust security measures.
- Access control systems are being enhanced to ensure that only authorized personnel have access to sensitive data.
- Data de-identification techniques are increasingly used to protect individual privacy.
- Investments in data security are growing, as businesses aim to fortify their defenses against cyberthreats.
Emphasizing data quality and security is not just a regulatory compliance issue but a cornerstone of ethical business practice.
With the rise of citizen data scientists and the focus on decision intelligence, organizations are now more than ever aware of the importance of securing their data assets. This trend is not only prevalent in sectors like healthcare and financial services but across all industries that handle consumer data.
The Role of AI in Data Governance
The integration of Artificial Intelligence (AI) in data governance is transforming how organizations manage and protect their data assets. AI is enhancing the efficiency and accuracy of data governance processes, from compliance monitoring to data quality management. With regulatory compliance becoming increasingly complex, AI's ability to interpret and act upon large datasets is proving indispensable.
- AI-driven systems can automatically classify and tag data, ensuring sensitive information is handled correctly.
- Machine learning algorithms can detect anomalies in data access patterns, potentially preventing breaches.
- Predictive analytics can forecast potential compliance risks, allowing for proactive measures.
The synergy between AI and data governance is creating a more dynamic and responsive approach to managing data risks and compliance.
As businesses navigate the evolving landscape of data privacy and security, the role of AI will continue to grow. The development of ethical frameworks and industry standards will be crucial in ensuring that AI's role in data governance remains responsible and transparent.
Best Practices for Data Stewardship
In the realm of business intelligence, data stewardship is pivotal for maintaining the integrity and value of data assets. Ensuring data accountability and reliability is a cornerstone of effective data management. To achieve this, organizations must adopt best practices that preserve the clarity and precision of data lineage, and enforce robust data governance policies.
- Access control is essential to limit dataset usage to authorized personnel through role-based access controls.
- Data de-identification helps in removing personal information, safeguarding privacy.
- Emphasizing data security measures is crucial to protect against cyberthreats and attacks.
Iterative learning from past efforts and successes, as well as mistakes, is key to continuous improvement in data stewardship.
By focusing on these practices, businesses can ensure the usability, accessibility, safety, and reliability of their data, while also addressing ethical considerations such as data privacy.
Voice-Activated Analytics: The New Frontier
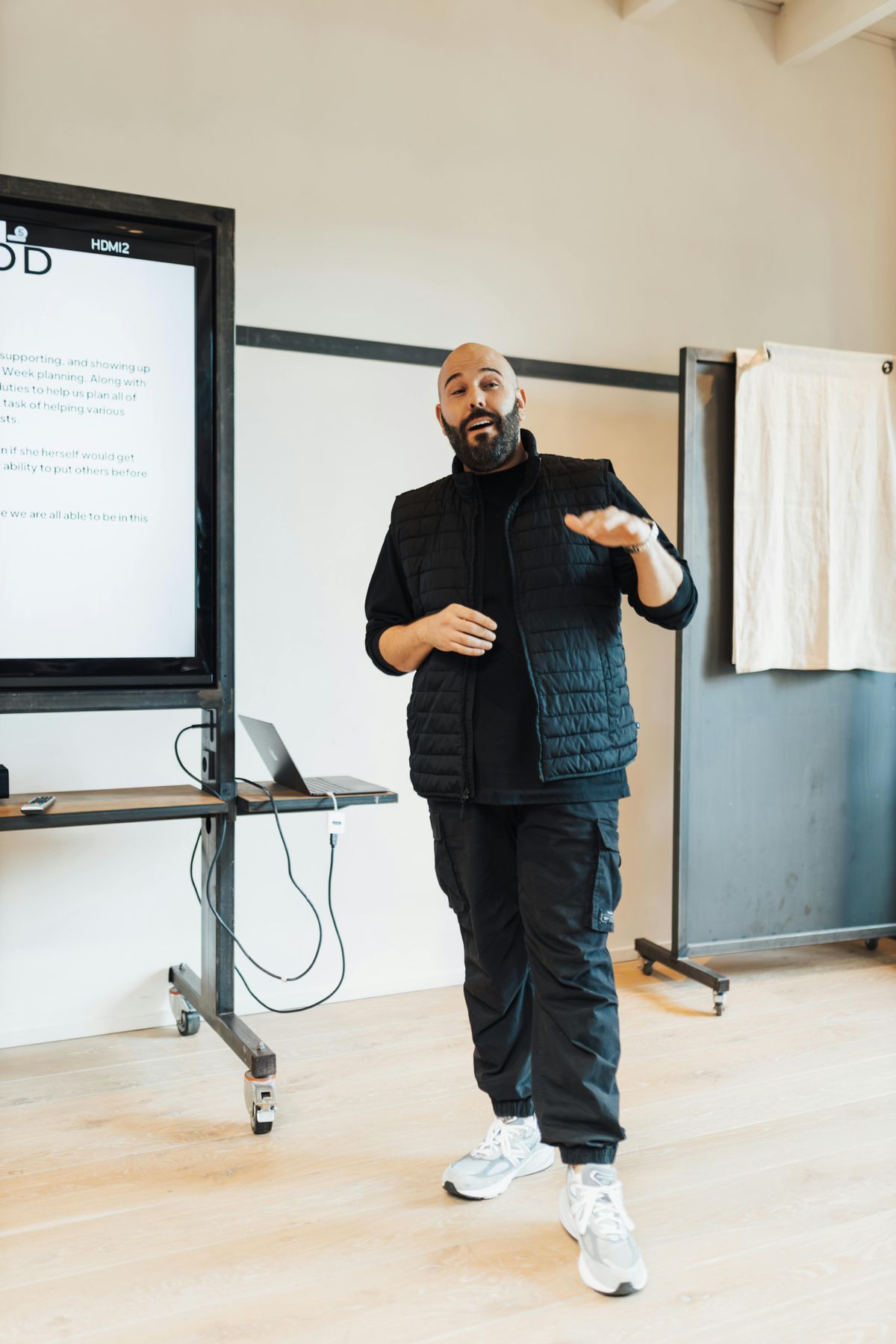
How Voice Commands are Changing Data Interactions
The integration of voice commands in business intelligence is revolutionizing the way we interact with data. With the advent of AI and Natural Language Processing (NLP), digital assistants are becoming more sophisticated, enabling a more natural and efficient way to access and analyze data.
Voice-activated assistants are particularly beneficial for small business owners who previously had to navigate complex reports and dashboards manually. Now, they can simply speak to their BI tools to get the insights they need, making data more accessible than ever before.
- With voice AI, businesses can transcribe spoken language into structured data.
- These digital assistants are streamlining tasks for data workers, allowing for quicker and more accurate data analysis.
The promise of voice-activated BI tools is to transform the way we extract insights from data, making it as simple as having a conversation.
The trend towards voice-enabled analytics is clear, and as these technologies continue to mature, we can expect them to become an integral part of the BI landscape.
Integrating Voice-Activated Assistants with BI Platforms
The integration of voice-activated assistants with BI platforms marks a significant shift in how businesses interact with data. Voice commands are simplifying the way users query and analyze data, making it more accessible to a broader range of users. With the rise of artificial intelligence (AI) and natural language processing (NLP), these digital assistants are becoming more adept at understanding and executing complex requests.
The seamless integration of voice-activated assistants into BI tools is transforming data interaction from a task-oriented process to a conversational experience.
This transformation is not just about convenience; it's about efficiency and inclusivity. By enabling voice commands, BI platforms can cater to users with varying levels of technical expertise. Here's a look at the current landscape:
- Over 3 billion AI voice assistants were in use in 2019.
- The number is expected to grow to over 6 billion by the end of 2023.
- These assistants are increasingly capable of transcribing voice and converting it into analyzable data.
The potential for growth in ROI through the use of voice assistants is substantial, as they offer a hands-free, intuitive way to access insights and drive data-driven decision-making.
Future Prospects of Voice-Enabled Analytics
The integration of voice-enabled analytics is poised to revolutionize the way businesses interact with data. The potential for voice search optimization and generative AI to streamline BI processes is immense. With the advent of digital assistants capable of transcribing spoken words into actionable data, the BI landscape is evolving rapidly.
The seamless interaction between users and BI tools through voice commands is expected to enhance user experience and accessibility, making data-driven decisions more intuitive and immediate.
However, the journey ahead is not without its challenges. Issues such as data privacy and the need for skilled personnel are critical considerations. Industries like healthcare, finance, retail, and technology are at the forefront of this transformation and will likely experience significant changes.
As we look to the future, continuous intelligence and evolving BI trends will shape the trajectory of voice-activated analytics. The key to success lies in the ability to adapt and embrace these advancements while maintaining a human-centric approach and ensuring data democratization.
The Integration of Connected Cloud Ecosystems
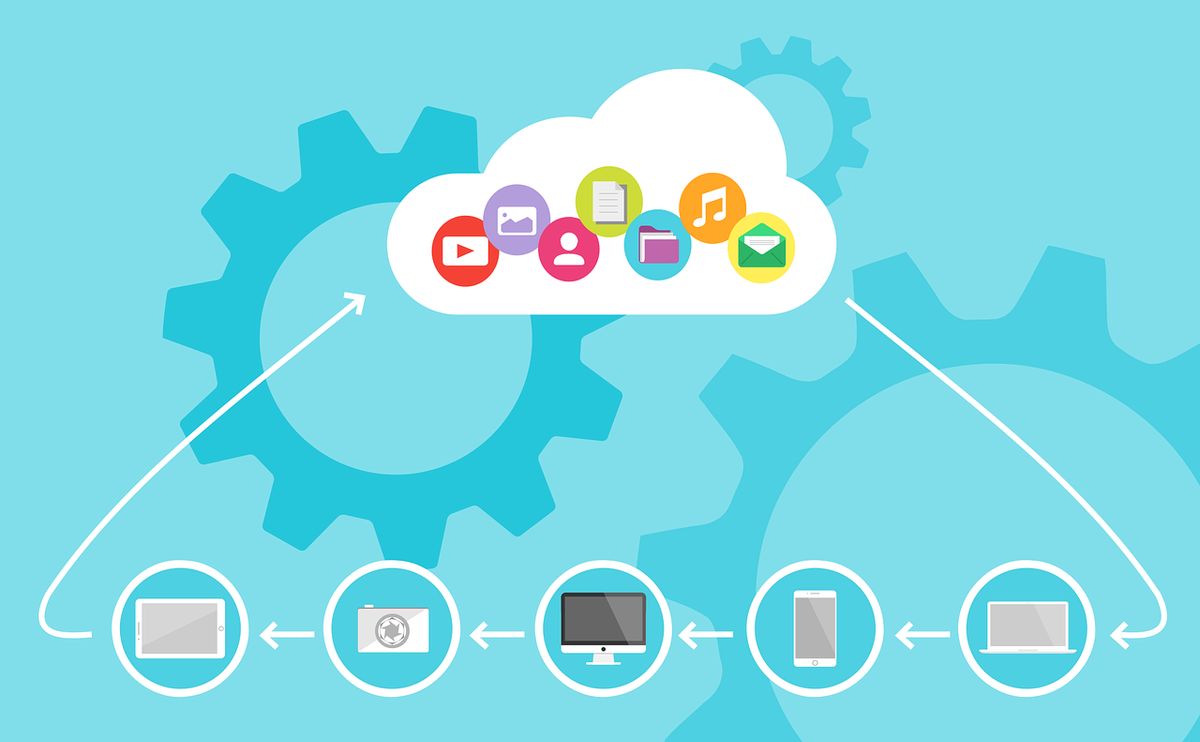
Benefits of a Unified Cloud Infrastructure
The adoption of a unified cloud infrastructure marks a significant shift in how businesses manage and utilize their data. A unified cloud infrastructure streamlines operations, offering a comprehensive view of data across the organization. This holistic approach breaks down data silos, enhances data quality, and embeds governance principles, leading to more informed decision-making.
Flexibility is a key advantage of a unified cloud infrastructure, allowing businesses to adapt quickly to changing data needs and market conditions. By facilitating effective data sharing and collaboration, organizations can leverage collective insights to drive innovation and growth.
- Improved data accessibility
- Enhanced collaboration across teams
- Streamlined governance and compliance
A unified cloud infrastructure not only simplifies data management but also paves the way for advanced analytics and business intelligence capabilities. It is a foundational element that supports the agility and responsiveness of modern enterprises.
Challenges in Cloud Integration and Solutions
While the connected cloud strategy offers a panacea for various risks and costs associated with data sources moving to the cloud, it is not without its challenges. Integrating disparate systems into a cohesive cloud environment can introduce complexity, risk, and additional costs. These factors make it difficult to find a one size fits all BI solution that caters to every business's unique needs.
- Flexibility is a key advantage of a connected cloud strategy, yet achieving it requires careful planning and execution.
- The risks involved in cloud analytics must be mitigated through robust security measures and compliance with regulatory standards.
- Cost management is crucial as businesses must balance the investment in cloud infrastructure with the expected ROI.
The challenges cloud migration and modernization solve for businesses often boil down to time and money, yet addressing these challenges is essential for successful cloud integration.
As cloud business intelligence becomes a dominant force, the future of business is undoubtedly in the cloud. However, navigating the transition requires a strategic approach to overcome the hurdles of cloud integration.
Case Studies: Companies Leveraging Connected Cloud
The shift towards connected cloud ecosystems has been pivotal for businesses seeking to streamline their operations and enhance their analytics capabilities. The transformative power of connected cloud strategies is evident in various case studies across industries. For instance, companies have been able to mitigate risks and reduce costs while gaining the flexibility to access and analyze data from anywhere.
Amazon Web Services (AWS), as reported by adopters, leads the pack as a cloud BI provider, with Microsoft Azure, Google Cloud, and IBM Bluemix also playing significant roles in the market. The preference for these providers underscores the importance of reliable and scalable cloud infrastructure in today's data-driven business landscape.
The connected cloud is not just a technological shift; it's a strategic move that aligns with the future of business intelligence.
While the benefits are clear, it's crucial to acknowledge the challenges in implementing a connected cloud strategy. These include ensuring data privacy, managing complex integrations, and maintaining consistent data quality across platforms. Addressing these challenges is essential for companies to fully harness the potential of the connected cloud.
The Emergence of Collaborative Business Intelligence
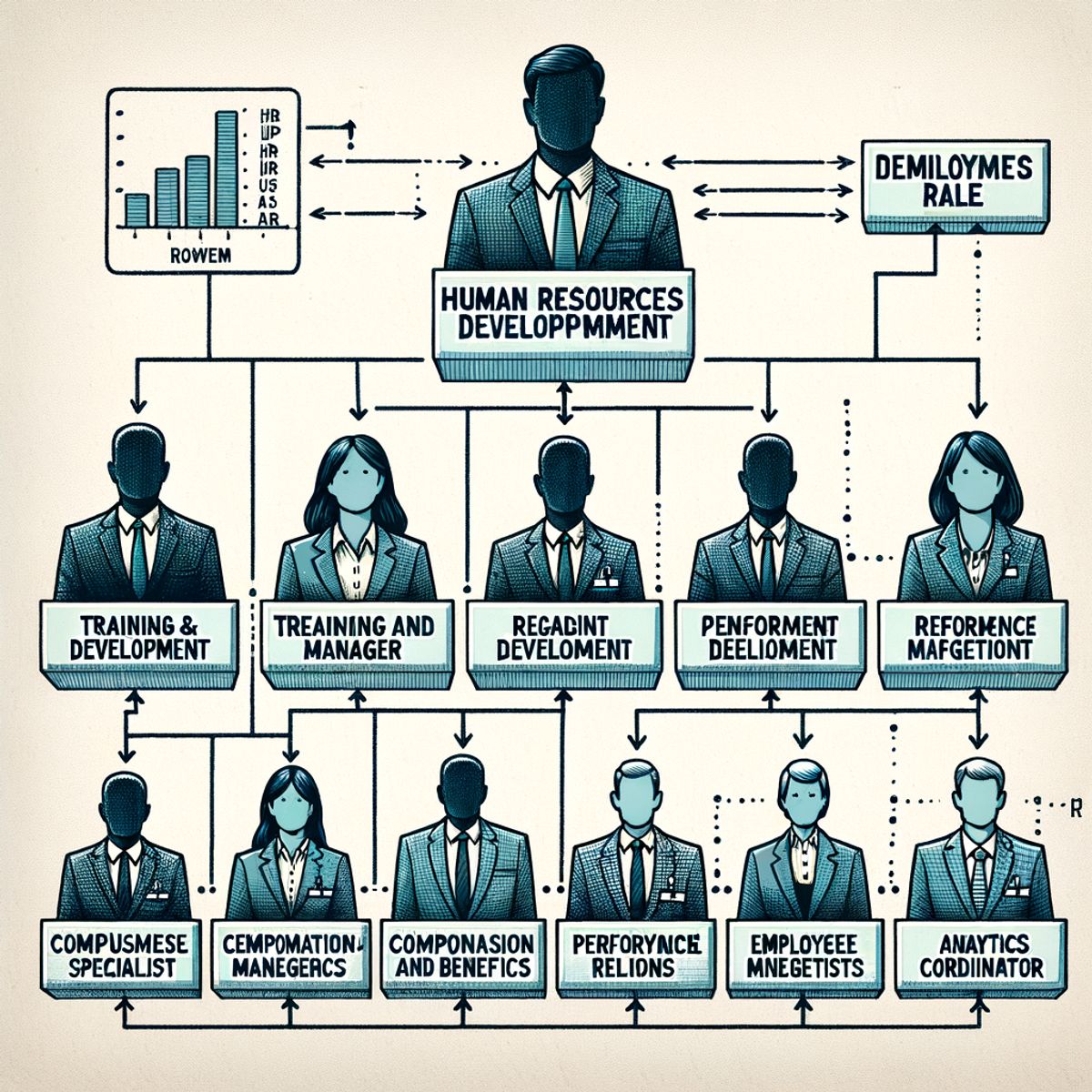
Tools and Technologies Enabling BI Collaboration
The landscape of Business Intelligence (BI) collaboration is rapidly evolving, with a focus on tools and technologies that facilitate seamless interaction and shared analytics. Collaborative BI tools are combining the power of BI with the best of collaboration software, including web 2.0 and social technologies, to enhance data-driven decision-making processes.
- Collaborative BI is redefining enterprise-wide analytics and reporting.
- It encourages collaboration and improves the efficiency of decision-making.
- Simplifies data access, analysis, and interpretation, especially when integrated with self-service BI interfaces.
Collaborative BI is creating an opportune setting for deliberation on analytics, streamlining decision-making, and fostering a culture of shared insights and collective problem-solving.
The integration of collaborative BI tools is not just about technology; it's about transforming the culture of an organization to one that values data-driven collaboration. As the BI tools market continues to grow, with Gartner predicting its expansion in 2022, the adoption of collaborative BI platforms is becoming a critical component for companies aiming to leverage data for competitive advantage.
Fostering a Culture of Data-Driven Collaboration
In the realm of business intelligence, fostering a culture of data-driven collaboration is pivotal for organizations aiming to harness the full potential of their data assets. A collaborative environment ensures that insights and analytics are not just the purview of data specialists but are integrated throughout the organization, supporting efficient decision-making.
To cultivate such a culture, businesses must address common challenges, including resistance to change and the complexity of managing vast data volumes. Embracing iterative learning and focusing on long-term benefits can lead to a gradual yet impactful transformation.
Organizations that prioritize data literacy and contextualize their data can engage all team members in the analytical conversation, leading to more informed decisions and a robust data-driven culture.
Key takeaways for building a successful data-driven culture include:
- Redefining enterprise-wide analytics and reporting to encourage collaboration.
- Streamlining decision-making processes by making analytics more accessible.
- Implementing data literacy campaigns to ensure all team members can contribute meaningfully.
Impact on Remote Work and Distributed Teams
The shift towards remote work and distributed teams has been significantly accelerated by the global pandemic, leading to an increased reliance on collaborative Business Intelligence (BI) tools. Collaborative BI has become a cornerstone for remote teams, ensuring that geographical dispersion does not hinder data-driven decision-making.
Italics are used to emphasize the importance of seamless integration of BI tools with communication platforms, which is essential for maintaining the flow of information across different time zones and work environments.
- Enhanced communication and collaboration features
- Real-time data sharing and analysis
- Centralized data repositories accessible from anywhere
The adoption of collaborative BI tools has not only facilitated a more inclusive and democratic approach to data analysis but also empowered teams to leverage collective intelligence effectively.
As organizations continue to navigate the complexities of remote work, the role of collaborative BI in bridging the physical divide cannot be overstated. The table below highlights the key benefits that collaborative BI tools offer to remote and distributed teams:
Benefit | Description |
---|---|
Accessibility | Enables team members to access BI resources from any location. |
Synchronization | Keeps data and insights in sync across all team members. |
Communication | Streamlines communication channels for data-related discussions. |
Productivity | Boosts productivity by facilitating quick and informed decision-making. |
Self-Service Analytics: Empowering End-Users
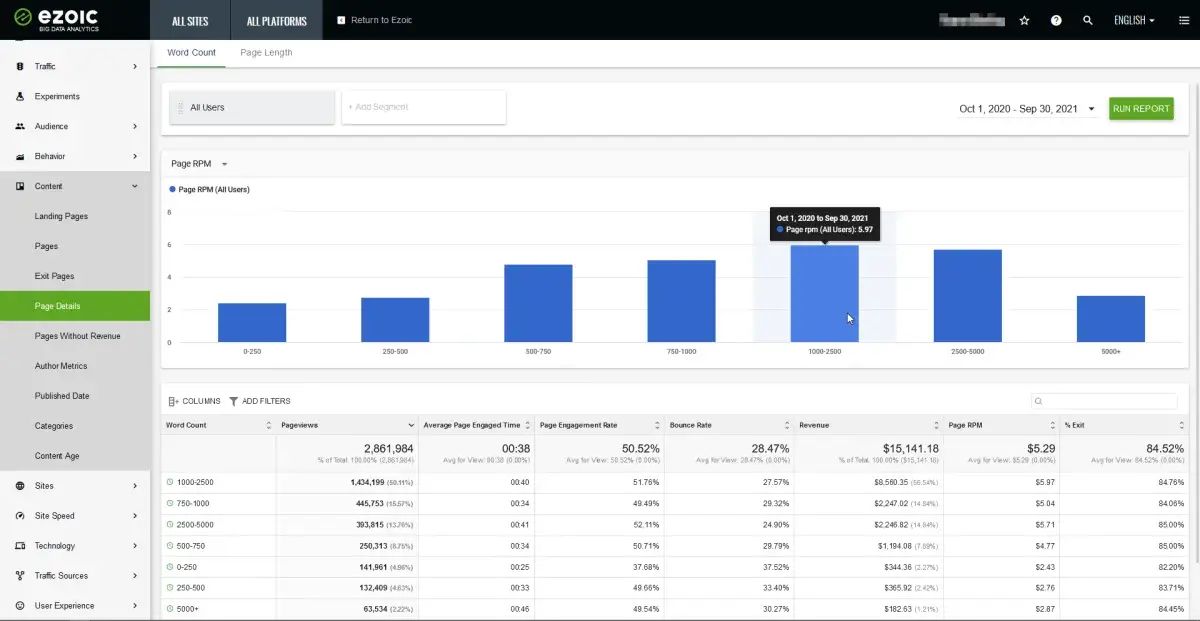
Trends in User-Friendly BI Interfaces
In the realm of Business Intelligence (BI), the user experience is paramount. Interactive dashboards have become the cornerstone of user-friendly BI interfaces, centralizing data into accessible web pages. These dashboards are not just about displaying data; they are about enabling users to interact with it through responsive features like filters and drill-down options.
Visualization techniques play a critical role in enhancing the user experience. By employing elements such as colors, fonts, and type sizes, analysts can create visual hierarchies that emphasize significant information and facilitate quicker insights.
The goal is to empower users to explore data intuitively, making complex information digestible at a glance.
Here's a glimpse into the elements that make BI interfaces user-friendly:
- Responsive design for various devices
- Intuitive navigation and layout
- Real-time data updates
- Customizable visual elements
- Accessibility features for diverse user needs
As BI tools evolve, they increasingly cater to the need for mobility and context-specific insights, ensuring that actionable analytics are available wherever the user may be.
Training and Support for Self-Service BI Adoption
The shift towards self-service business intelligence (BI) is a transformative trend in the BI landscape. Empowering non-technical users to perform analytics tasks without the need for data scientists is a key driver for this movement. As self-service BI gains momentum, the need for comprehensive training and support becomes critical to ensure successful adoption.
- User Training: Tailored training programs are essential to equip users with the necessary skills to utilize self-service BI tools effectively.
- Support Systems: Ongoing support, including help desks and user communities, provides a safety net for users as they navigate the BI platform.
- Feedback Loops: Establishing channels for user feedback helps in refining the tools and training materials based on real-world usage.
The democratization of data through self-service BI platforms is not just a trend; it's a strategic imperative for businesses aiming to foster a data-driven culture.
As organizations embrace self-service BI, they must also prioritize data security and governance to protect sensitive information. The balance between accessibility and security is delicate but essential for the sustainable growth of self-service BI capabilities.
Measuring the Impact of Self-Service on Business Outcomes
The advent of self-service business intelligence (SSBI) has marked a significant shift in how companies approach data analysis. Businesses are now regularly evaluating the impact of self-service analytics on key performance indicators. This evaluation often includes assessing improvements in decision-making speed and scrutinizing any increases in revenue, which are direct outcomes of democratizing data access.
Self-service BI has not only reduced the reliance on data scientists but also enabled a more agile and responsive approach to data analysis. The following table highlights some of the key metrics used to measure the impact of SSBI:
Metric | Description |
---|---|
Decision-Making Speed | Time taken from data access to decision |
Revenue Growth | Increase in earnings attributed to SSBI |
User Adoption Rate | Percentage of employees using SSBI tools |
Operational Efficiency | Reduction in time and resources for analysis |
Embracing SSBI has led to a more collaborative and empowered workforce, where every employee has the potential to contribute to data-driven decisions. This shift is not just about technology; it's about fostering a culture that values data accessibility and literacy.
As SSBI continues to evolve, it's crucial to measure its impact not just in terms of immediate business outcomes, but also in how it shapes the long-term strategic direction of a company. By doing so, organizations can ensure that their investment in self-service tools translates into tangible benefits.
Prioritizing Data Security in a BI Environment
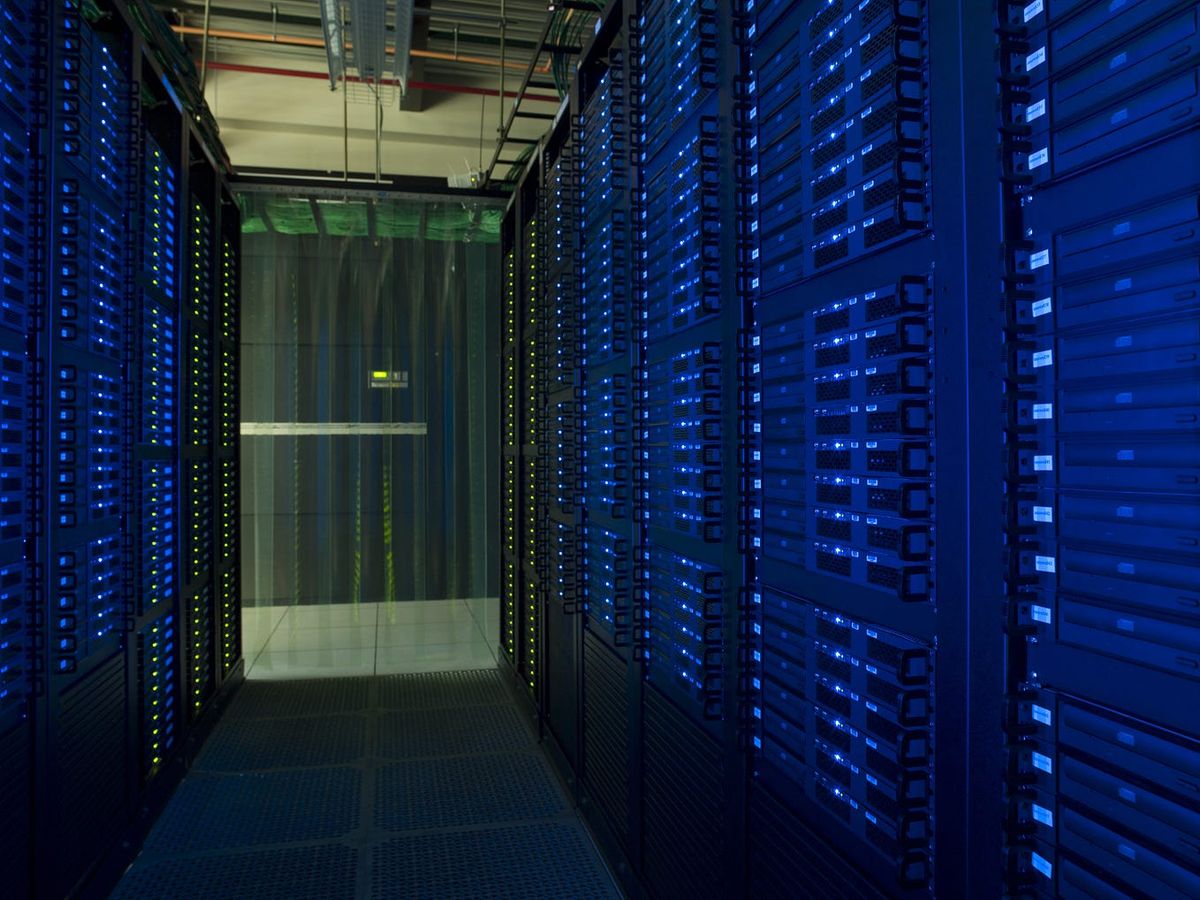
Emerging Threats and Security Measures
As the digital landscape evolves, so do the tactics of cyberattackers. There has been a wide range of major cybersecurity incidents in 2023, from sophisticated nation-state espionage campaigns to large-scale data breaches that have affected thousands of businesses. The Infosecurity Magazine reports a list of the top 10 cyber-attacks of the year, highlighting the critical need for robust security measures.
In response to these emerging threats, organizations are prioritizing database security. Consumers, now more aware of the value of their personal data, are increasingly cautious about sharing information online. This shift in consumer behavior underscores the importance of establishing impervious security layers to protect against successful attacks.
The integration of advanced technologies such as AI, natural language processing, and augmented analytics presents new opportunities but also requires heightened vigilance in data security and privacy.
To address these challenges, businesses are adopting regulatory frameworks and industry standards to ensure data protection and instill trust among consumers. The finance industry, for example, faces complex data sharing challenges with inherent security and privacy risks, necessitating specific technologies and governance steps for safe interoperability.
Regulatory Compliance and Data Protection Laws
In the wake of stringent regulations like the GDPR and the California Consumer Protection Act, businesses are compelled to prioritize data governance to avoid hefty fines and legal repercussions. The cost of non-compliance has become a significant motivator for companies to invest in robust data security measures.
Data privacy laws have not only increased operational costs but also reshaped marketing strategies, leading to a resurgence in membership-based platforms. As countries worldwide adopt similar regulations, the need for businesses to adapt is critical.
Businesses must navigate this complex regulatory environment, ensuring that their data practices are both ethical and compliant.
To effectively manage compliance, organizations can leverage prescriptive analytics, which utilizes historical compliance data, financial conditions, and predictive models to recommend bespoke strategies for risk mitigation and opportunity maximization.
Building a Security-Conscious Data Culture
In today's digital landscape, building a security-conscious data culture is essential for organizations to maintain trust and ensure the integrity of their business analytics. With a focus on data privacy, security, and compliance with regulations like GDPR and CCPA, businesses are increasingly prioritizing protective measures.
- Access control systems limit dataset usage to authorized personnel through role-based access controls.
- Data de-identification processes review datasets to remove any personal information.
- Implementation of robust data security measures to safeguard against cyberthreats and attacks.
Emphasizing data ethics and privacy is not just a regulatory requirement; it's a strategic business imperative that resonates with consumers' growing awareness of their personal information's value.
Advancements in BI tools and the rise of citizen data scientists are reshaping how decisions are made, ensuring that data security is not an afterthought but a foundational aspect of the decision-making process.
Artificial Intelligence's Role in Business Analytics
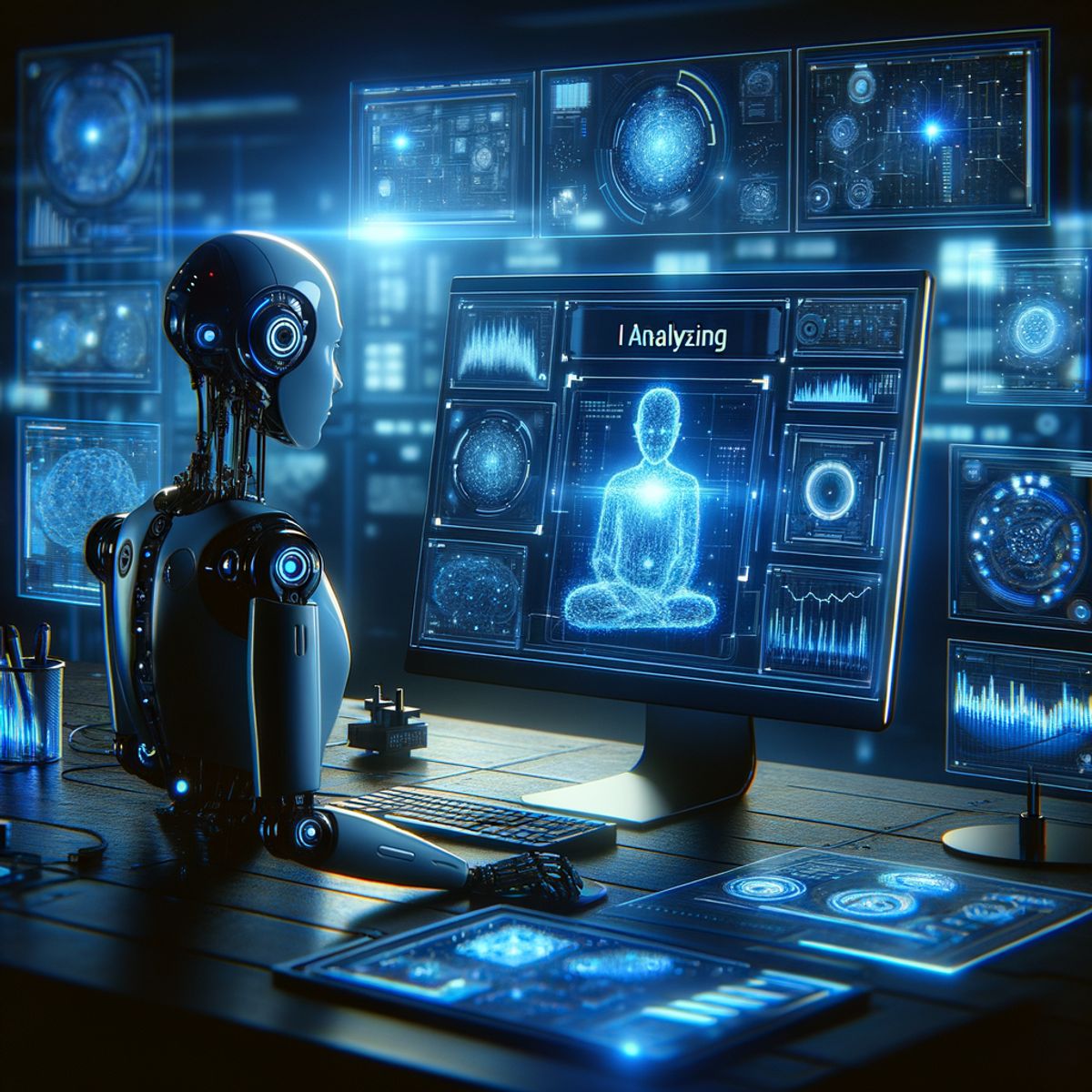
AI-Driven Predictive Analytics
The landscape of predictive analytics has undergone a significant evolution, becoming a cornerstone in the realm of business intelligence. By leveraging deep learning and machine learning (ML) models, these analytics have the power to sift through current and historical data, offering forecasts of future trends, behaviors, and outcomes with remarkable accuracy.
AI-driven algorithms have revolutionized the way organizations approach data analysis. These sophisticated models are capable of automating tedious manual processes, identifying intricate correlations, and generating insights from voluminous datasets. For example, ML models are adept at monitoring data to pinpoint trends and anomalies, subsequently alerting relevant teams in real-time—crucial in scenarios like cyber threat detection where immediate response is imperative.
AI's role extends beyond mere analysis; it empowers every individual within an organization to access vital insights and key metrics through a centralized, real-time analytics platform. Its prowess in uncovering patterns within both structured and unstructured data sets is unparalleled, often revealing trends that would remain obscured in traditional manual analysis.
The integration of AI and ML technologies into business processes has not only streamlined the extraction of actionable insights but has also brought about enhancements in automation, cost reduction, and overall efficiency within the BI services sector.
Machine Learning Models in BI
The integration of machine learning (ML) models into Business Intelligence (BI) systems has revolutionized the analytics landscape. Machine learning enables the discovery of hidden insights within large datasets, often revealing patterns and trends that are imperceptible to human analysts. This capability enhances the predictive power of BI tools, allowing businesses to anticipate market changes and customer behavior with greater accuracy.
ML models are now a staple in advanced BI platforms, providing a level of data analysis that goes beyond traditional statistical methods. These models can process and learn from data in real time, adapting to new information as it becomes available. This dynamic approach to data analysis is crucial for businesses that operate in fast-paced environments where timely and accurate insights can provide a competitive edge.
The use of machine learning in BI is not just about processing data; it's about transforming raw, unstructured information into actionable intelligence that drives strategic decision-making.
One of the most significant applications of ML in BI is in the realm of prescriptive analytics. By synthesizing descriptive and predictive analytics, ML algorithms can recommend strategic business decisions and optimize outcomes by considering various scenarios. This level of analysis is invaluable for companies looking to make data-driven decisions that align with their business objectives.
Ethical Considerations of AI in Analytics
As artificial intelligence (AI) becomes more integrated into business analytics, ethical considerations take center stage. The transparency of AI decision-making processes is a growing concern, with organizations striving to understand the 'logic' behind AI conclusions. This has led to the development of explainable AI, which aims to make the decision-making of AI systems more understandable to humans.
Responsible AI is not just a technical requirement but a business imperative. Companies are increasingly held accountable for the ethical use of data, emphasizing the need for robust regulatory frameworks and industry-wide standards.
The ethical use of data extends to ensuring data privacy and allowing clients to maintain control over their personal information. Businesses must implement encryption and access controls to safeguard sensitive data. Here are some key practices for ethical AI in analytics:
- Ensuring data privacy and client control over personal information
- Sharing data only with authorized sources
- Encrypting sensitive information to protect against security threats
- Adopting and complying with regulatory frameworks and industry standards
The necessity for ethical AI practices in analytics is clear, and businesses are aware of the risks involved. As AI continues to evolve, so too must the strategies for maintaining ethical standards in data analysis and usage.
Enhancing Data Quality Management (DQM)
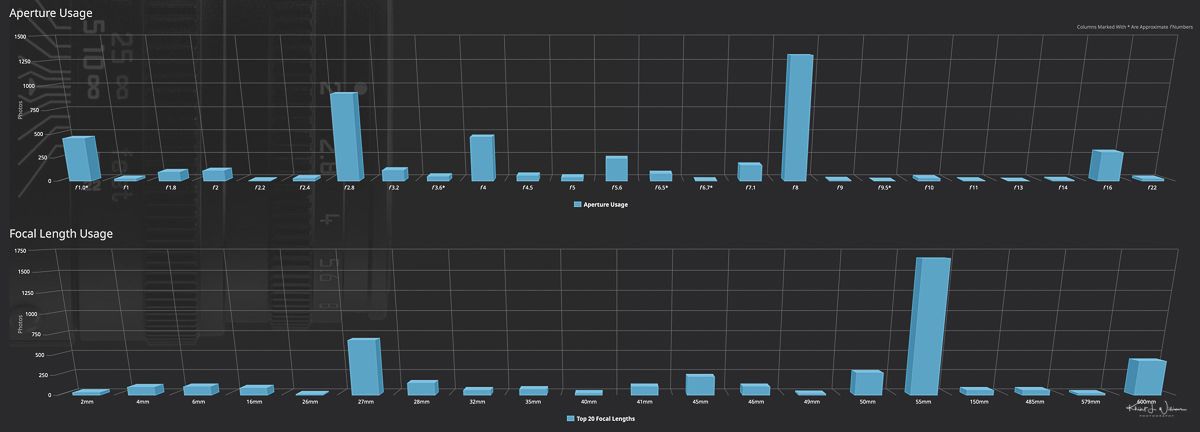
Techniques for Ensuring Data Accuracy and Consistency
In the realm of Business Intelligence (BI), the integrity of data is paramount. Robust data management practices are the cornerstone of ensuring data accuracy and consistency. These practices include standardization, which ensures uniformity across datasets; validation, to confirm that the data meets specific criteria and standards; and de-duplication, to eliminate redundant information.
Data quality management (DQM) is increasingly recognized as a central component of BI strategies. It is not only about maintaining the integrity of data but also about complying with stringent regulatory requirements. To achieve this, businesses are adopting various practices:
- Access control: Implementing role-based access to restrict data usage to authorized personnel.
- Data de-identification: Removing personal identifiers from datasets to protect individual privacy.
- Data security: Establishing robust security protocols to safeguard data against cyber threats.
By focusing on these techniques, organizations can ensure that the data they rely on for decision-making is both reliable and secure. This is crucial for businesses that must address data quality challenges, integrate AI in BI tools, and foster a data-driven culture to stay competitive.
The Impact of DQM on Decision-Making
The implementation of Data Quality Management (DQM) is pivotal for businesses seeking to harness the full potential of their data assets. High-quality data is the cornerstone of sound decision-making, providing clarity and confidence to business leaders as they navigate complex market dynamics. With DQM, organizations can ensure that the data they rely on is accurate, consistent, and reflective of the true state of affairs.
DQM not only enhances the reliability of business intelligence but also fortifies the foundation for predictive analytics. By establishing robust DQM practices, companies are better equipped to anticipate future trends and make proactive decisions. This strategic foresight is essential for maintaining a competitive edge in today's fast-paced business environment.
The integration of DQM into business processes is not merely a technical endeavor; it is a strategic initiative that permeates the entire organization, fostering a culture of data-driven excellence.
Here are some key takeaways regarding the role of DQM in decision-making:
- Ensures data accuracy and governance
- Enforces data standardization across the organization
- Facilitates clear and reliable insights into business operations
- Supports predictive analytics for proactive decision-making
- Propels the company forward with reliable insights and strategic foresight
Tools and Solutions for Data Quality Improvement
In the realm of Business Intelligence, the significance of Data Quality Management (DQM) cannot be overstated. As businesses increasingly rely on data to drive decisions, the demand for tools that can ensure data accuracy and consistency has surged. These tools are pivotal in rectifying issues such as formatting errors and typographical mistakes, which are common in data from diverse sources like social media, IoT devices, and databases.
To address these challenges, a variety of solutions have emerged:
- Data Profiling software to assess data quality and identify anomalies.
- Data Cleansing tools that correct errors and standardize data formats.
- Master Data Management (MDM) systems that provide a single source of truth.
- Data Governance platforms that enforce policies and ensure compliance.
Embracing these tools not only enhances the quality of data but also fortifies the data governance framework, leading to more reliable business insights.
The landscape of DQM tools is dynamic, with new and improved solutions being introduced regularly. Staying abreast of the latest offerings is crucial for businesses aiming to maintain a competitive edge through superior data quality.
Actionable Analytics: From Insight to Implementation
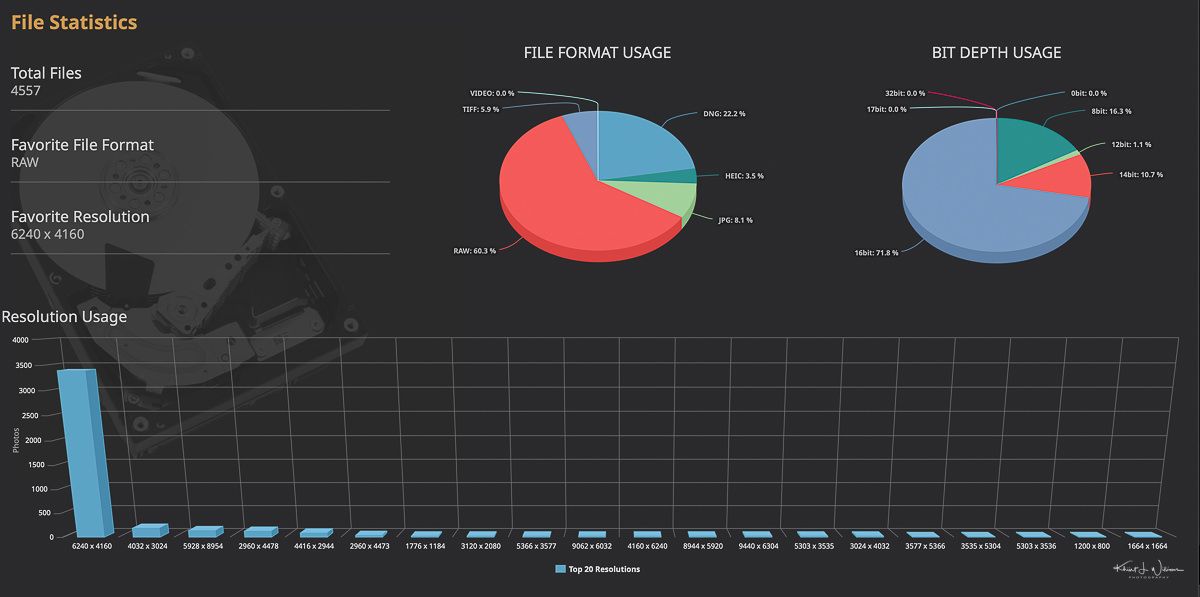
Bridging the Gap Between Data Analysis and Business Action
The future of Business Intelligence is being shaped by its ability to convert complex data into actionable insights. These insights are crucial for tailoring strategies to specific industries or lines of business. However, the challenge lies in making these insights accessible and actionable for business users.
- Business intelligence tools are now more capable than ever, with features like dashboard extensions, embedded analytics, APIs, and mobile analytics.
- These features enable a seamless transition from data analysis to business action, all within a single platform.
However, putting insights into action is easier said than done. It requires a clear process of data storytelling to convey information in a way that is both reasonable and actionable.
The tangible nexus between proficient data analytics and adept business strategy has never been more pronounced. In an era where data is the linchpin of strategic foresight, the ability to decipher data propels organizations to respond to current and future market trends effectively.
Real-Time Analytics and Decision Support Systems
In the realm of business intelligence, real-time analytics and decision support systems (DSS) are pivotal in translating data into immediate action. These systems enable organizations to respond swiftly to market changes, customer behavior, and operational challenges. By leveraging real-time analytics, businesses can gain a competitive edge through instantaneous insights and informed decision-making.
The integration of real-time analytics with DSS is transforming the landscape of data-driven strategies. For instance, in healthcare, decision support systems can help professionals navigate complex scenarios by utilizing real-time data and AI to extract actionable insights. This synergy is crucial for delivering timely and effective patient care.
The fusion of large datasets with statistical analysis is revolutionizing how decisions are made in the real world, reshaping industries from healthcare to retail.
Here are some key benefits of real-time analytics in decision support systems:
- Immediate identification of trends and anomalies
- Enhanced operational efficiency and productivity
- Faster reaction to customer needs and market demands
- Improved accuracy in forecasting and strategic planning
It is essential, however, to ensure that these insights are built on high-quality data. Without accurate, clean, and structured data, the analysis might mislead rather than guide. Therefore, robust data management practices are indispensable for the efficacy of real-time analytics and DSS.
Success Stories: Turning Insights into Business Value
The transformative power of business intelligence (BI) is best exemplified through success stories where companies have turned raw data into tangible business value. Amazon, for instance, has mastered the art of extracting actionable insights from customer behavior, leveraging purchasing history and feedback to refine their marketing strategies and enhance customer experiences.
Data storytelling is at the heart of this transformation, providing the necessary context to make numbers speak to decision-makers. It's not just about having access to data but being able to interpret and act upon it that counts. The journey from data to decision is a critical one, and it's paved with the skills of analysts who can narrate the story behind the data.
The key to actionable analytics lies in the ability to translate complex data into a compelling narrative that drives business decisions.
Here are a few examples of how insights have been turned into action:
- Amazon: Personalized marketing campaigns based on customer data analysis.
- A retail chain: Inventory optimization by predicting seasonal demand.
- A healthcare provider: Improved patient outcomes through data-driven treatment plans.
These cases illustrate the profound impact that well-executed BI strategies can have on an organization's bottom line and operational efficiency.
Unlock the full potential of your data with OptimizDBA's unparalleled expertise in database optimization. Our commitment to delivering faster data solutions sets us apart, ensuring your transactions are not just twice as fast, but often 100 times, 1000 times, or even more! Don't just take our word for it; experience the OptimizDBA difference that over 500 clients have trusted since 2001. Ready to revolutionize your data performance? Visit our website now to learn how we can transform your insights into powerful, actionable results.
Conclusion
As we navigate through 2023, the landscape of business intelligence and analytics continues to evolve, driven by the relentless pace of technological advancements and the growing demand for data-driven decision-making. This article has explored the top trends that are shaping the industry, from the rise of data storytelling and actionable analytics to the increasing importance of data governance and the integration of AI. Organizations that stay informed and adapt to these trends will not only enhance their decision-making processes but also maintain a competitive edge in an increasingly data-centric world. As the boundaries of BI and analytics expand, it is crucial for businesses to embrace these developments, ensuring they are equipped to harness the full potential of their data and thrive in the years to come.
Frequently Asked Questions
What is data storytelling in Business Intelligence?
Data storytelling is the practice of building a narrative around data analytics to communicate insights in a compelling and understandable manner, often integrating visualizations and narrative techniques to make complex data more accessible for decision-making.
How is AI impacting data governance in BI?
AI is playing a significant role in data governance by automating data quality checks, compliance monitoring, and risk management, thereby enhancing the effectiveness and efficiency of governance strategies.
What are the benefits of voice-activated analytics in BI?
Voice-activated analytics in BI allow users to interact with data using natural language, making data analysis more accessible, increasing efficiency, and enabling hands-free operation, which can be particularly useful in multitasking environments.
What challenges do businesses face with cloud integration in BI?
Businesses face challenges such as data security, compliance with regulations, managing multi-cloud environments, and ensuring seamless data integration across different cloud services and on-premise systems.
How does collaborative BI enhance business operations?
Collaborative BI enables teams to share insights, work together on data analysis, and make decisions based on collective knowledge, which can lead to improved outcomes, especially in remote work and distributed team environments.
What is self-service analytics, and why is it important?
Self-service analytics empower end-users to perform data analysis without needing specialized technical skills, fostering a data-driven culture and enabling faster, more informed decision-making across the organization.
Why is data security crucial in a BI environment?
Data security is critical in a BI environment to protect sensitive information from unauthorized access, breaches, and other cyber threats, ensuring trust and compliance with data protection laws.
What role does actionable analytics play in business strategy?
Actionable analytics provide businesses with practical insights that can be directly applied to improve processes, enhance customer experiences, and drive strategic initiatives, bridging the gap between data analysis and implementation.