The Evolution of Business Analytics
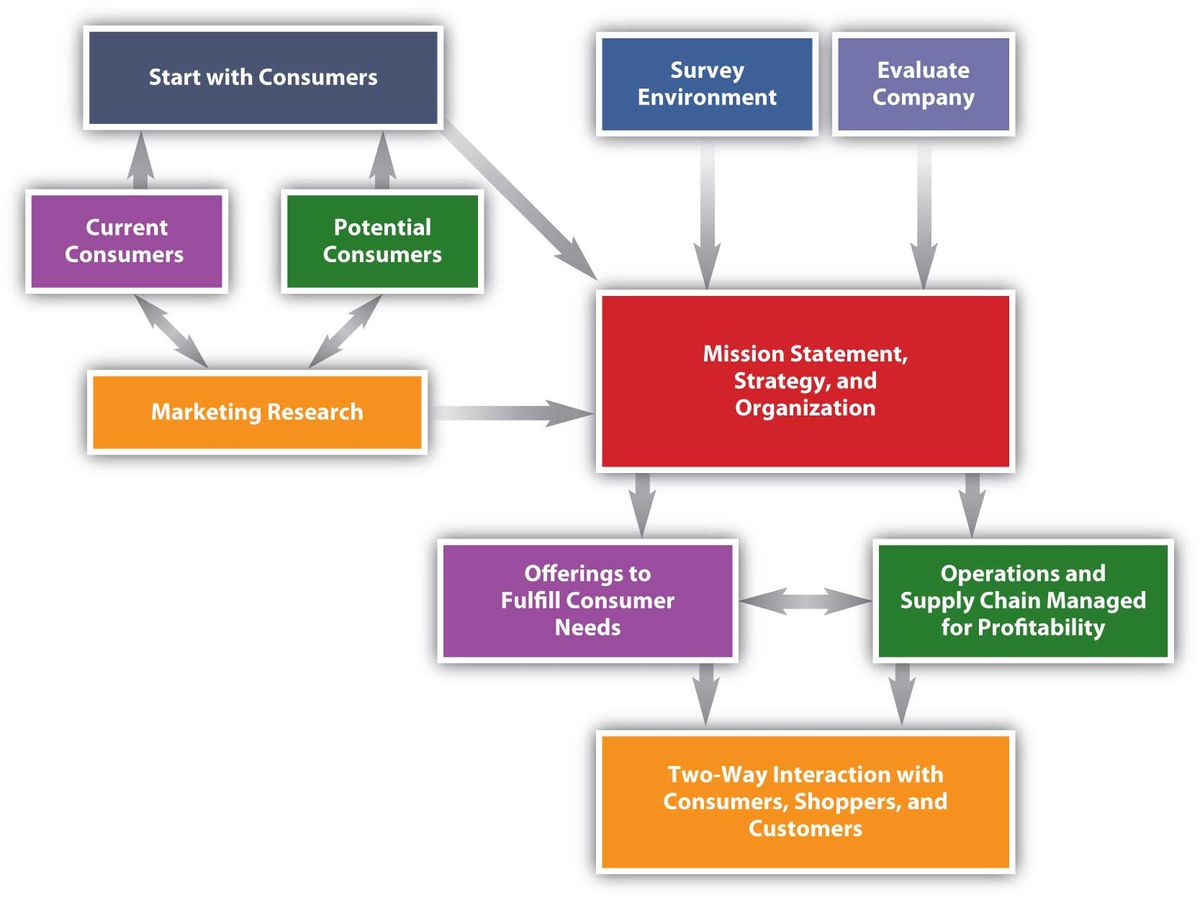
From Descriptive to Predictive and Prescriptive
The journey from descriptive to predictive and prescriptive analytics represents a significant leap in the ability of businesses to harness data for strategic advantage. Descriptive analytics provides a look at what has happened in the past, offering insights through data aggregation and mining. As we progress to predictive analytics, organizations gain the foresight to anticipate future trends and behaviors, enabling proactive decision-making.
Prescriptive analytics, the most advanced among the four pillars of analytics, goes a step further. It not only forecasts future scenarios but also recommends actions to achieve optimal outcomes. By employing sophisticated algorithms and decision frameworks, businesses can make informed choices that drive success and efficiency.
The evolution of analytics has transformed the landscape of business decision-making, shifting from a reactive to a strategic, forward-looking approach.
Here is a simple breakdown of the analytics types:
- Descriptive Analytics: What has happened?
- Diagnostic Analytics: Why did it happen?
- Predictive Analytics: What could happen?
- Prescriptive Analytics: What should we do about it?
The Rise of Big Data and Machine Learning
The advent of big data has revolutionized the landscape of business analytics, enabling companies to process and analyze vast datasets to uncover insights that were previously inaccessible. Machine learning algorithms have become integral in parsing through this data, learning from it, and identifying patterns that can inform strategic decisions.
Emerging trends in big data analytics include:
- Machine Learning as a Service (MLaaS)
- Data-Centric AI
- AI-Powered Analytics
- Edge Computing
- Deep Learning
With the proliferation of digital technologies and the Internet of Things (IoT), harnessing the power of big data analytics is becoming essential for organizations seeking to extract actionable insights.
The future of big data is promising, with predictions indicating an increased reliance on machine learning and AI to optimize analytics. As data volumes continue to grow, cloud storage and smart assistants that depend on big data analytics will become more prevalent, shaping the way businesses interact with data and make decisions.
Historical Milestones in Analytics Technology
The journey of analytics technology is marked by significant milestones that have revolutionized the way businesses operate. From the abacus to AI, the evolution of analytics has been driven by the quest for more accurate and actionable insights. The Ishango Bone, dating back to 18,000 BCE, is often cited as one of the earliest known tools for data representation.
As we progressed through the centuries, pivotal developments occurred:
- The 17th century saw the introduction of statistics as a tool for data analysis.
- The 20th century brought forth the computer revolution, enabling complex calculations and data storage.
- The turn of the 21st century witnessed the rise of big data and advanced analytics techniques.
The ability to not only understand but also predict and influence business outcomes has transformed analytics into a cornerstone of modern business strategy.
Today, organizations leverage a myriad of analytics tools to gain a competitive edge, from simple spreadsheets to sophisticated machine learning algorithms. The historical progression of analytics technology underscores its vital role in shaping the future of business decision-making.
Data-Driven Decision Making
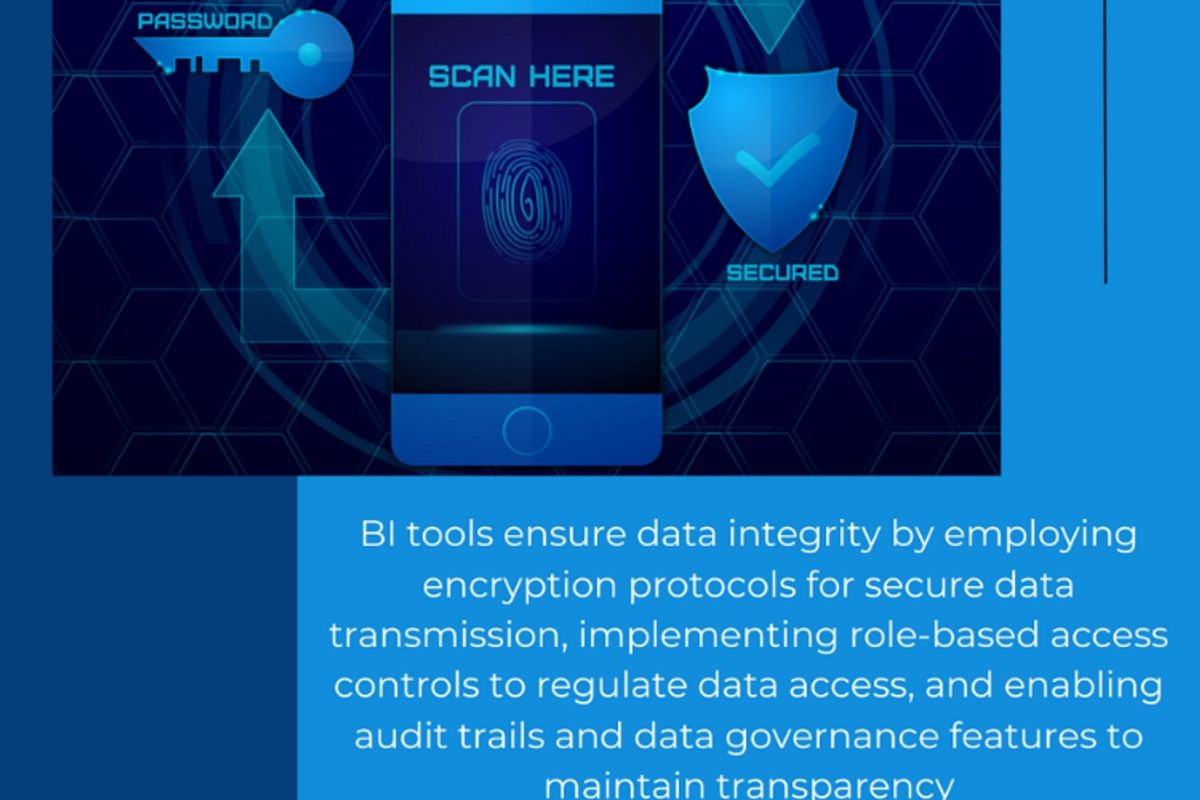
Integrating Analytics into Strategic Planning
The integration of analytics into strategic planning is a critical step for businesses aiming to stay competitive and agile in today's market. By aligning an analytics strategy with business use cases that drive value, such as gaining customer insights through a 360-degree view, organizations can significantly increase revenue.
To effectively manage insights from various touchpoints, consider the following steps:
- Invest in unified data platforms that integrate data from different sources, offering a consolidated view.
- Leverage technologies that allow for real-time data processing to respond swiftly to emerging patterns.
- Ensure that insights are shared across teams, promoting a holistic approach to strategy formulation.
The key to successful analytics integration lies in the ability to transform data into actionable insights that inform strategic decisions and foster business growth.
While the potential benefits of analytics are undeniable, organizations often face challenges such as data silos, integration issues, and talent shortages. Overcoming these obstacles is essential for harnessing the full potential of analytics to enhance operational efficiency and optimize performance.
Case Studies: Success Stories and Lessons Learned
The application of analytics in business has led to numerous success stories, where data-driven decisions have significantly improved performance and profitability. One notable example is a retail giant that optimized its supply chain through predictive analytics, resulting in reduced overhead costs and improved customer satisfaction.
Case studies not only highlight the triumphs but also shed light on the challenges faced during implementation. For instance, companies often struggle with data silos and the integration of analytics into existing workflows. The lessons learned from these experiences are invaluable for shaping future strategies:
- Ensuring data quality and consistency
- Building a skilled analytics team
- Fostering a culture that embraces data-driven decision making
By analyzing these case studies, businesses can anticipate potential obstacles and develop more robust analytics frameworks. This proactive approach can lead to more effective and efficient problem-solving, ultimately driving success in a competitive landscape.
Overcoming Challenges in Data Interpretation
While the potential benefits of analytics are undeniable, organizations often face challenges in harnessing its full potential. From data silos and integration issues to talent shortages and data privacy concerns, there are various obstacles that need to be overcome. Clear communication and alignment on goals and objectives are critical in navigating the analytics landscape.
Overcoming the obstacles of data-driven decision making begins with a strong leadership commitment.
To address these challenges, consider the following steps:
- Simplifying complex terms and explaining acronyms to ensure understanding across multidisciplinary teams.
- Maintaining open communication and accurate feedback loops to foster collaboration.
- Investing in high-quality communication tools to facilitate seamless information sharing.
- Providing support and training to ease the transition for those accustomed to traditional decision-making processes.
Resistance to change within an organization can be a significant barrier. Transitioning to a data-driven culture may encounter pushback from those accustomed to traditional decision-making processes. It is essential to communicate the benefits clearly and provide the necessary support and training to ease the transition.
The Role of Diagnostic Analytics in Business
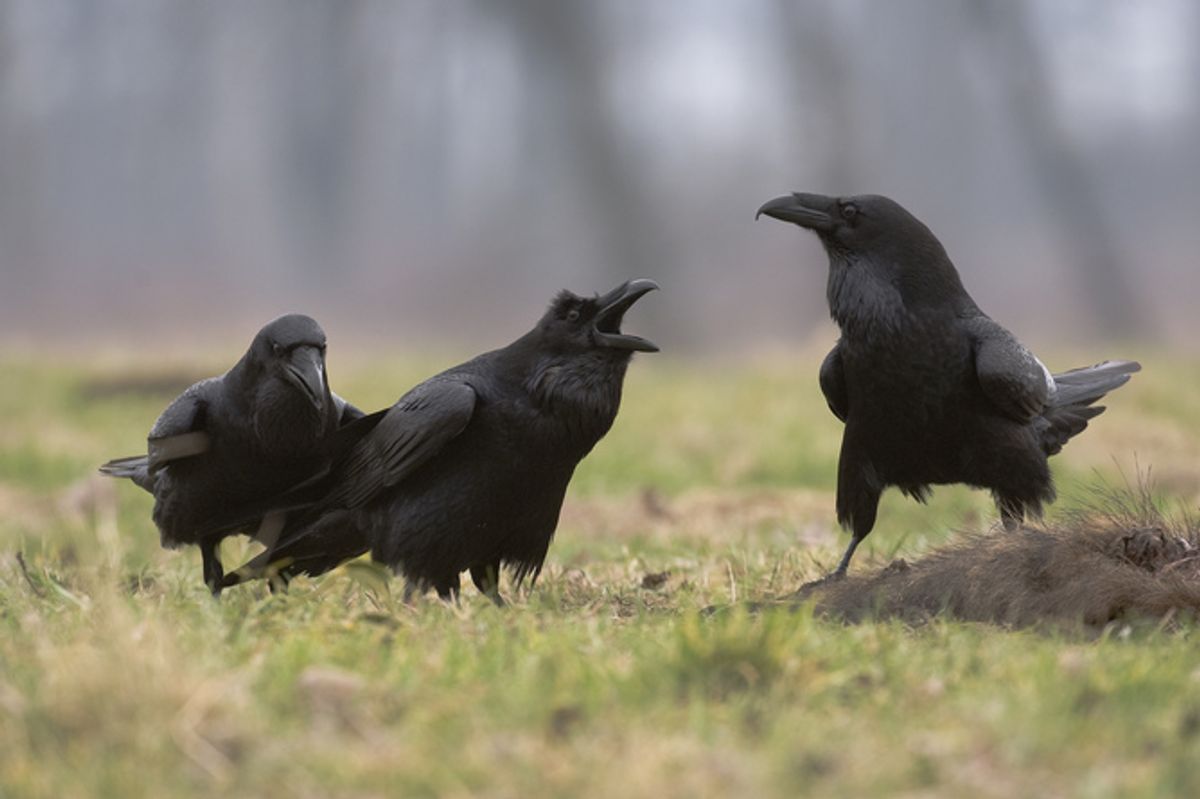
Identifying Patterns and Trends
In the realm of business analytics, identifying patterns and trends is a cornerstone for strategic foresight. Data analytics is essential for businesses to extract valuable insights, improve decision-making, and stay ahead of trends. Different types like descriptive, diagnostic, predictive, and prescriptive analytics play unique roles in transforming raw data into actionable strategies.
- Data can reveal patterns and trends that are not immediately apparent, allowing you to make predictions about future market behaviors and align your business accordingly.
- By analyzing customer behavior and preferences, businesses can tailor their products and services to meet their needs more effectively, leading to increased customer satisfaction and loyalty.
Effective data visualization not only enhances comprehension but also enables decision-makers to quickly identify trends, patterns, and outliers. Predictive analytics leverages historical data patterns to forecast future trends, demands, and events, allowing for proactive decision-making and resource alignment.
Root Cause Analysis for Performance Issues
In the realm of business analytics, diagnostic analytics plays a crucial role in uncovering the why behind performance issues. By using techniques such as data mining and statistical analysis, companies can identify underlying factors contributing to specific outcomes. This level of insight is essential for businesses to diagnose problems, identify opportunities, and make informed decisions to address underlying issues effectively.
Common challenges in diagnostic analytics include:
- The use of technical jargon that may not be clear to non-technical teams
- Poor communication, feedback, and evaluation across teams
- Lack of alignment on goals, scope, and objectives
To overcome these challenges, it is vital for businesses to foster clear communication, ensure alignment on analytics goals, and promote cross-functional collaboration.
Modern businesses rely on data for informed decision-making. Understanding data, leveraging it, and using advanced analytics tools are key for business success. Diagnostic analytics helps businesses implement targeted solutions that streamline processes and drive efficiency, ultimately leading to a more robust and informed decision-making process.
Implementing Targeted Solutions
Once patterns and root causes of performance issues are identified, businesses must pivot towards implementing targeted solutions. These solutions should be tailored to address the specific challenges uncovered by diagnostic analytics. For instance, simplifying complex terms and ensuring clear communication can enhance understanding across teams.
Investing in the right tools is crucial for effective implementation. A structured approach may include:
- Simplifying complex terms and explaining acronyms
- Maintaining open communication and accurate feedback across teams
- Investing in high-end communication tools
- Onboarding all involved parties to the goals and dynamics of the project
Embracing agility in implementation allows businesses to adapt to insights and refine strategies continuously.
Furthermore, integrating unified data platforms and leveraging real-time analysis are essential steps for managing insights from various touchpoints. This can be represented in a succinct table:
Step | Action |
---|---|
1 | Invest in unified data platforms |
2 | Leverage real-time analysis technologies |
3 | Promote team collaboration for holistic strategy |
By focusing on these targeted solutions, companies can optimize their operations, improve communication, and ultimately drive better business outcomes.
Harnessing Predictive Analytics for Competitive Advantage
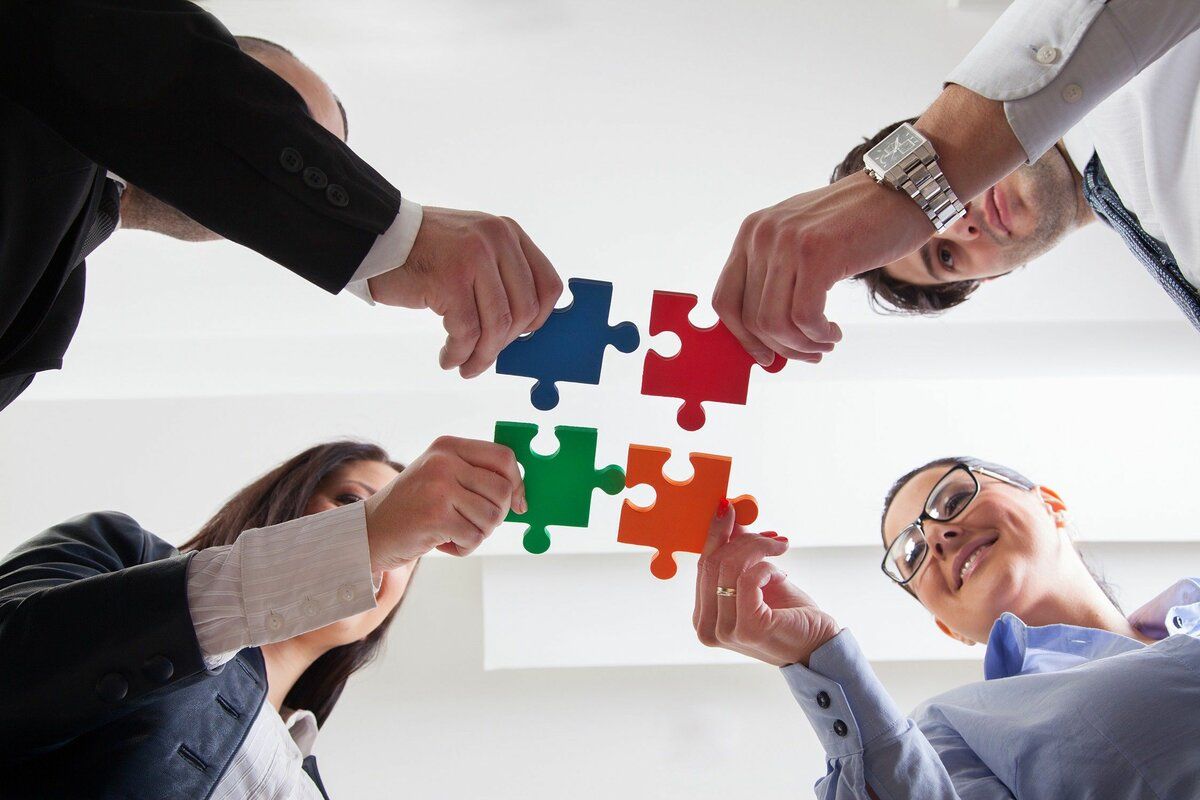
Forecasting Market Trends and Consumer Behavior
In the ever-changing landscape of consumer markets, forecasting trends and behavior is crucial for businesses aiming to stay ahead. By leveraging analytics, companies can gain insights into future consumer actions and preferences. This predictive power enables businesses to align their strategies with anticipated market shifts, ensuring they meet the evolving demands of their customer base.
Recent studies highlight key trends that are expected to shape consumer behavior in the coming year. For instance, a focus on cautious spending is predicted to be a significant factor in 2023. Understanding such trends is vital for companies to adapt their marketing and sales strategies effectively.
Data analytics not only helps in predicting market trends but also empowers businesses to understand their customers more deeply, fostering a customer-centric approach that can enhance satisfaction and loyalty.
Here are some of the top U.S. consumer trends to watch:
- Cautious spending
- Embracing data collection
These insights, derived from data analytics, are essential for retailers who aim to predict consumer behavior and find success in a potentially challenging 2024 market.
Risk Assessment and Management
In the realm of business analytics, risk assessment and management are critical components that leverage predictive analytics to safeguard the enterprise. By analyzing historical and real-time data, businesses can anticipate potential risks and devise strategies to mitigate them. This proactive approach is essential for maintaining a competitive edge and ensuring long-term sustainability.
Accruon's data analytics services empower businesses with BI for informed decisions, risk management, and revenue growth through predictive insights. The integration of cybersecurity measures into analytics is not just a precaution but a necessity in today's digital landscape. Regular security assessments and the implementation of AI for surveillance are key steps in protecting sensitive data from cyber threats.
With big data comes great responsibility. Ensuring data security and quality is paramount to the success of any analytics-driven risk management strategy.
Challenges such as data privacy and security, and organizational resistance to change, must be addressed to fully harness the power of analytics in risk assessment. The following points highlight the importance of a robust data infrastructure:
- Implementing robust security measures and adhering to data protection regulations.
- Conducting regular security assessments and vulnerability testing.
- Maintaining a continuous commitment to cybersecurity enhancements.
Enhancing Customer Experience with Predictive Insights
The advent of predictive analytics has revolutionized the way businesses approach customer experience. By harnessing historical data, companies can now forecast future customer behaviors and preferences, tailoring their services to meet the anticipated needs. This proactive stance ensures that customers feel understood and valued, leading to increased satisfaction and loyalty.
Personalization is at the heart of enhancing customer experience. Predictive models enable businesses to create individualized experiences that resonate with customers on a personal level. For example, e-commerce platforms use predictive analytics to suggest products that a customer is more likely to purchase, based on their browsing and buying history.
The strategic use of predictive insights can transform customer interactions from generic to exceptional, fostering a sense of exclusivity and connection.
The following table illustrates the impact of predictive analytics on key customer experience metrics:
Metric | Before Predictive Analytics | After Predictive Analytics |
---|---|---|
Customer Satisfaction Score | 75% | 85% |
Net Promoter Score (NPS) | +30 | +45 |
Customer Retention Rate | 80% | 90% |
By integrating predictive analytics into their operations, businesses not only enhance the customer experience but also achieve a significant competitive advantage. The ability to anticipate and meet customer needs is a game-changer in today's market, where customer centricity is paramount.
Prescriptive Analytics: Charting the Course of Action
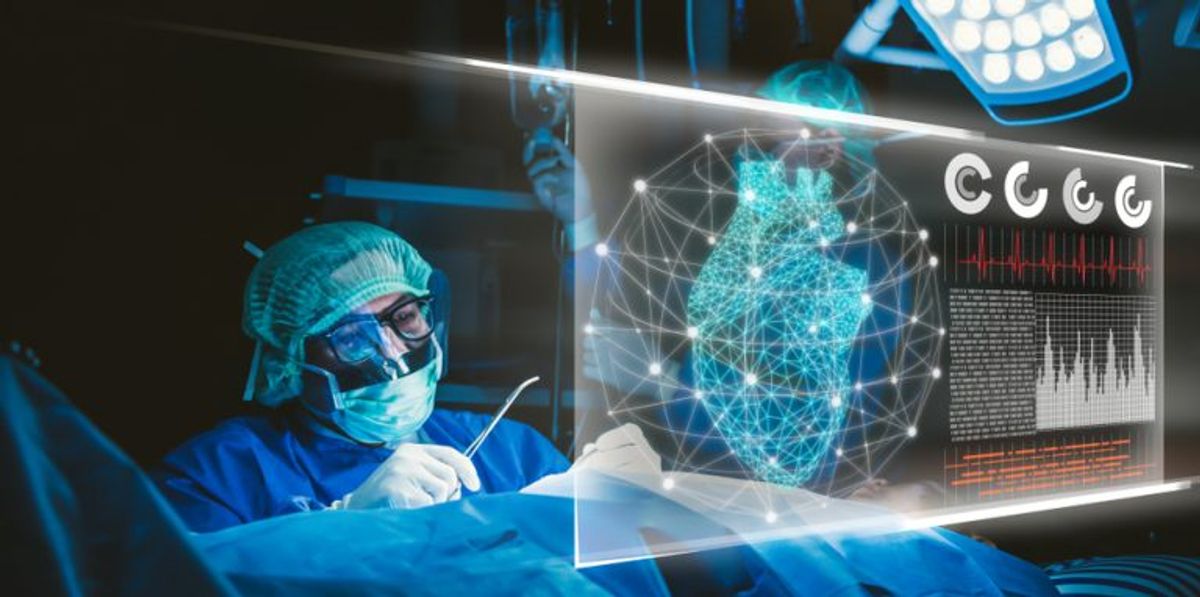
Optimizing Operations and Resource Allocation
In the realm of modern business, prescriptive analytics plays a pivotal role in enhancing operational efficiency. By scrutinizing operational data, companies can pinpoint inefficiencies, streamline workflows, and judiciously allocate resources. This analytical approach not only identifies bottlenecks but also paves the way for more effective supply chain management, inventory control, and workforce planning.
Operational efficiency is paramount for businesses striving for sustainable growth and a competitive edge. It involves the meticulous optimization of processes, resources, and technology to boost productivity and minimize waste. Ensuring that every facet of operations is fine-tuned leads to improved performance, reduced operational costs, and heightened customer satisfaction.
By integrating analytics into their strategic planning, organizations can transform data into actionable insights, driving operational efficiency through predictive and scenario modeling.
Here are some key benefits of optimizing operations through analytics:
- Enhanced performance and reduced operational costs
- Streamlined project management and improved delivery times
- Better resource allocation and workforce optimization
- Increased employee satisfaction and retention
Scenario Planning and Decision Analysis
In the realm of prescriptive analytics, scenario planning and decision analysis are pivotal for charting a successful course of action. By simulating various scenarios and evaluating potential impacts, businesses can identify the most effective strategies to achieve their goals. This analytical approach is not just about predicting outcomes, but about recommending the best actions to optimize performance.
Prescriptive analytics leverages optimization algorithms and decision-making frameworks to generate actionable recommendations. These recommendations are crucial for guiding strategic decisions and improving operational efficiency. For instance, a company might use scenario planning to decide whether to invest in new technology, enter a new market, or adjust its product portfolio.
By incorporating data-based insights into strategic planning, enterprises can enhance their overall performance and gain a competitive edge.
The following table illustrates the potential outcomes of different strategic decisions based on scenario analysis:
Scenario | Investment | Market Entry | Product Adjustment | Expected Outcome |
---|---|---|---|---|
A | High | New | Major | High Growth |
B | Low | Existing | Minor | Stable Growth |
C | Medium | New | None | Uncertain |
Each scenario presents a unique set of challenges and opportunities, and the right analytical tools can help decision-makers navigate these complexities with confidence.
Automating Decision Processes with AI
The integration of artificial intelligence (AI) in business analytics is transforming the landscape of decision-making. AI-powered automation is the new frontier, enabling companies to process vast amounts of data with unprecedented speed and accuracy. This shift not only enhances efficiency but also allows human talent to focus on more strategic and creative tasks.
- AI tools, like Improvado's AI Agent, facilitate seamless interaction with data, translating natural language queries into actionable insights.
- Python scripts and machine learning algorithms are employed to automate complex data processing, freeing up resources and generating significant ROI.
- The adoption of AI in analytics ensures that mundane tasks are handled by machines, while humans are empowered to innovate and drive growth.
The essence of automating decision processes with AI lies in its ability to redefine data analysis, offering predictive insights and smarter decision-making.
As businesses continue to embrace AI for automation, they witness a notable shift from manual, labor-intensive operations to streamlined, intelligent systems. The result is a more agile business environment where data-driven decisions are made swiftly, and strategic planning is informed by deep, analytical insights.
The Impact of Analytics on Marketing Strategies
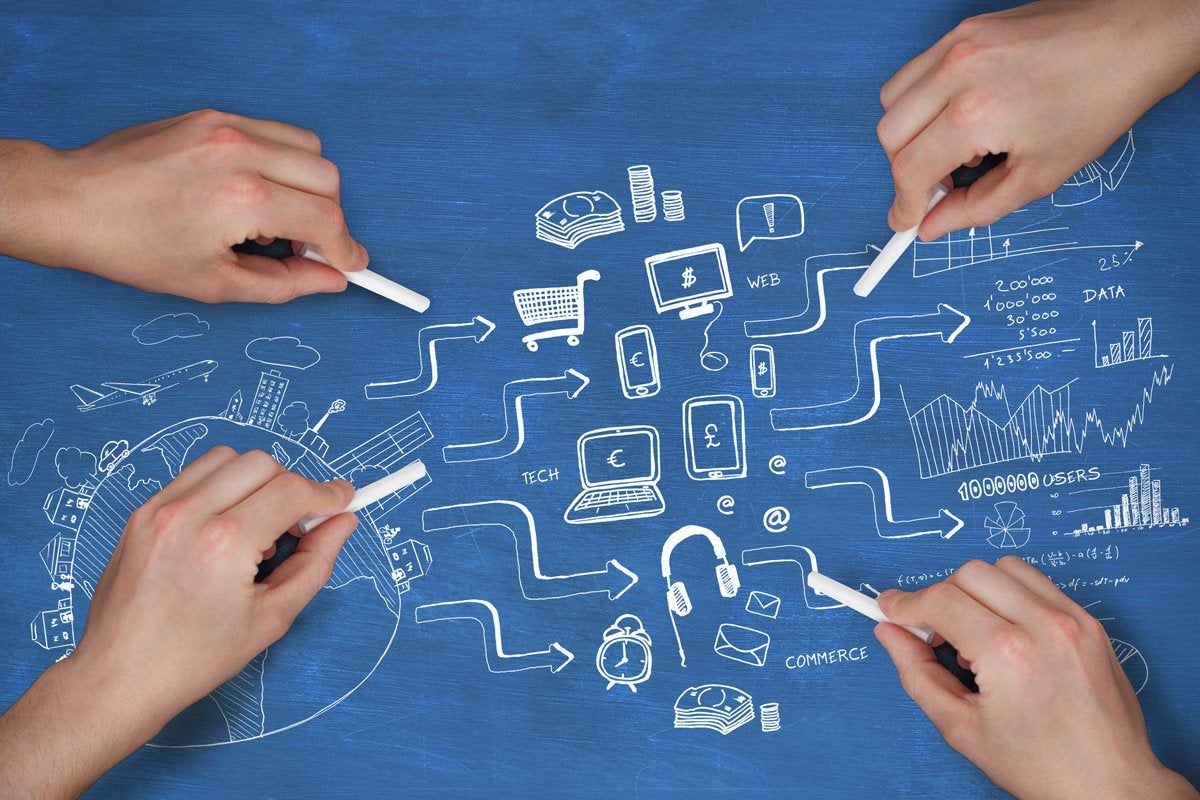
Personalization and Targeted Campaigns
In the realm of modern marketing, personalization has emerged as a cornerstone for engaging consumers effectively. By leveraging analytics, businesses can tailor their campaigns to meet the unique preferences and behaviors of individual customers. This granular approach not only enhances the customer experience but also drives significant improvements in campaign performance.
The integration of multi-touch attribution and predictive analytics has revolutionized how companies measure the efficacy of their marketing efforts. It allows for a nuanced understanding of the customer journey, attributing value to each interaction that leads to a conversion.
The table below highlights the benefits of personalized campaigns informed by analytics:
Benefit | Description |
---|---|
Increased Engagement | Tailored content resonates more deeply with consumers. |
Higher Conversion Rates | Personalized interactions often lead to better sales outcomes. |
Optimized Marketing Spend | Analytics enable more efficient allocation of resources. |
Embracing a data-driven approach to marketing not only addresses the complexities of consumer interactions but also ensures that resources are allocated effectively to maximize ROI. As the number of platforms and channels grows, the ability to manage and interpret this expanding web of data becomes a critical competitive advantage.
Measuring and Maximizing ROI
In the quest to maximize return on investment (ROI), businesses are increasingly turning to analytics to measure the effectiveness of their marketing strategies. Automated analytics platforms, like Improvado, are revolutionizing this process by streamlining data collection and transformation, leading to more informed decision-making.
To effectively manage insights from various touchpoints, consider the following steps:
- Identify new market opportunities through data analysis.
- Optimize marketing efforts by understanding customer interactions.
- Improve customer service by responding to data-driven feedback.
By setting data-based metrics for success, businesses can track progress and make iterative adjustments, ensuring agility and responsiveness to market conditions.
With the integration of multi-touch attribution and predictive analytics, companies can dissect the conversion funnel to understand the value of each consumer interaction. This nuanced approach aids in resource allocation and strategy refinement, driving a higher ROI.
Leveraging Social Media and Web Analytics
In the realm of modern marketing, social media analytics play a pivotal role in shaping strategies and enhancing user engagement. By meticulously gathering and analyzing data points from various social networks, businesses can gain invaluable insights into consumer behavior and preferences. This process not only informs a robust social media strategy but also optimizes engagement across platforms.
Emplifi highlights the importance of social media analytics as a cornerstone for informed decision-making. To harness the full potential of these analytics, consider the following steps:
- Invest in unified data platforms to integrate and analyze data from diverse sources.
- Embrace real-time analysis for swift response to emerging trends.
- Promote team collaboration to ensure insights fuel a comprehensive strategy.
Embracing the intricate layers of analytics, from automation to emotional analytics, is essential for capturing the full spectrum of consumer interactions. This approach paves the way for businesses to not only understand but also predict and influence customer behavior, driving growth and improving the customer experience.
Building a Data-Centric Organizational Culture
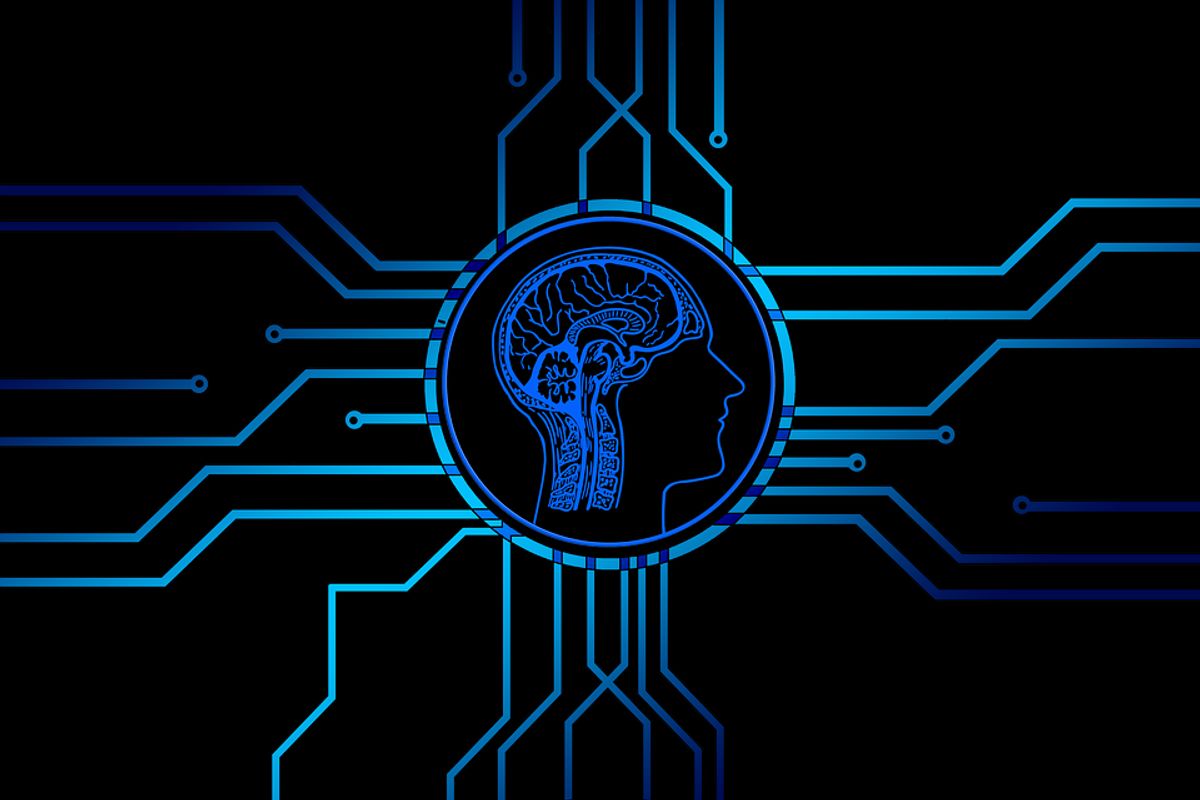
Fostering Data Literacy Among Employees
In the modern business landscape, data literacy is not just a valuable skill but a fundamental component of a thriving organization. Employees across all levels must understand the basic principles of data to make informed decisions and contribute to a data-driven culture.
- Leaders must champion the use of data and model the behavior they wish to see throughout the organization.
- Education and training are crucial in overcoming resistance and building confidence.
- Encouraging small-scale tests and pilots allows employees to see the value of data in action.
By promoting data literacy, businesses can unlock the full potential of data analytics and drive sustainable growth.
Investing in tools and platforms that enhance data accessibility, such as dashboards and centralized databases, empowers employees to retrieve real-time insights relevant to their roles. This democratization of information ensures that decision-makers at all levels are equipped with the same accurate data, fostering a culture of experimentation and innovation.
Creating a Collaborative Environment for Data Sharing
In the realm of modern business, encouraging collaborative data practices is crucial for aligning analytics with business objectives. A collaborative environment not only streamlines the collection and analysis of data but also fosters a culture of transparency and innovation. By sharing data across departments—from sales and marketing to customer service—organizations can leverage diverse perspectives to fuel strategic growth and informed decision-making.
A collaborative model facilitates the easy assemblage of data, which is then processed by analytics teams to provide valuable insights.
To overcome challenges in creating this environment, organizations can implement several strategies:
- Simplifying complex terms and jargon
- Maintaining open communication and accurate feedback
- Investing in high-quality communication tools
- Ensuring all team members understand the goals and scope of data initiatives
These steps help in building a data-centric culture where every employee has a basic understanding of data principles and is encouraged to participate in data-driven projects. Small-scale tests and pilots can demonstrate the value of data, building confidence and momentum for larger initiatives.
The Role of Leadership in Promoting Analytics
Leadership plays a pivotal role in the transition to a data-centric culture. Navigating non-relational data complexities and addressing the skills gap are key challenges that leaders must tackle to harness analytics for informed decision-making. By cultivating data-first leadership, organizations can ensure that analytics becomes an integral part of their strategic vision.
- Encouraging data literacy among employees
- Prioritizing data-driven initiatives
- Fostering a collaborative environment for data sharing
Leadership commitment to analytics is essential for driving a culture where data is valued as a critical asset for decision-making.
Investing in a data-driven culture is not just about adopting new technologies; it's about reshaping organizational attitudes and behaviors towards data. Leaders must champion the use of analytics and demonstrate its value in achieving business objectives.
Emerging Trends in Business Analytics
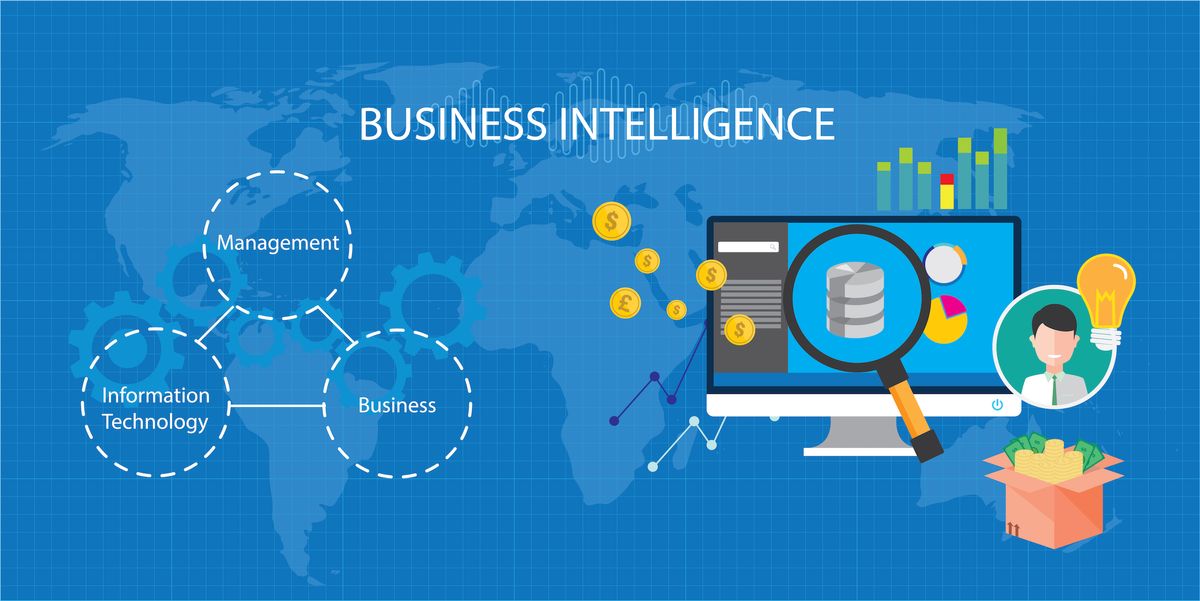
The Integration of IoT and Analytics
The convergence of the Internet of Things (IoT) and analytics is revolutionizing the way businesses operate. By connecting a myriad of devices and sensors, IoT generates a continuous stream of data that, when analyzed, can unlock profound insights into operations, customer behavior, and market trends. The real-time nature of IoT data combined with advanced analytics paves the way for more responsive and informed decision-making.
Integration challenges, however, remain a significant hurdle. To address these, businesses are adopting strategies such as:
- Investing in unified data platforms to consolidate IoT data sources
- Implementing real-time analysis for immediate insights
- Promoting team collaboration to ensure insights are shared and acted upon
Embracing IoT and analytics not only enhances operational efficiency but also drives innovation, offering a competitive edge in today's fast-paced market.
As IoT devices proliferate, the volume of data they produce becomes staggering. It is imperative for businesses to invest in robust analytics capabilities to sift through this data deluge and extract actionable intelligence. The table below illustrates the potential growth in IoT device connections, highlighting the escalating need for analytics integration.
Year | Estimated IoT Device Connections (in billions) |
---|---|
2021 | 10.07 |
2022 | 11.22 |
2023 | 12.44 |
2024 | 13.75 |
2025 | 15.09 |
The synergy between IoT and analytics is not just about handling the volume of data but also about transforming it into strategic assets that can guide businesses towards more innovative and efficient practices.
Advancements in Real-Time Data Processing
The growing importance of real-time data is a game-changer in the business world. As companies strive to make decisions based on up-to-the-minute information, the demand for advanced data processing technologies is at an all-time high. This shift is not just about speed; it's about the capacity to adapt quickly to market changes and unforeseen challenges, ensuring continuous project success.
Real-time analysis is a critical component of this trend. With the advent of automation and machine learning, data can now be processed as it streams in. This allows for immediate decision-making, a stark contrast to the traditional batch processing methods that could delay action.
The refinement of data into a more identifiable form for analysis is crucial. It involves organization, cleansing, integration, and transformation, all of which are essential for delivering insights at the speed of business.
The table below outlines the key aspects of data processing that are being enhanced to support real-time analytics:
Aspect | Description |
---|---|
Organization | Arranging data into categories for better analysis. |
Cleansing | Eliminating inconsistencies and flaws in data. |
Integration | Assembling data from various sources. |
Transformation | Presenting data in a consistent form across platforms. |
Ethical Considerations and Data Privacy
In the realm of business analytics, ethical considerations and data privacy are paramount. The advent of regulations like GDPR in Europe and HIPAA in the U.S. has set a new standard for data management. Non-compliance carries severe penalties, emphasizing the need for stringent data stewardship.
Organizations must navigate the complexities of data privacy and security, ensuring compliance with evolving regulations. This involves not only protecting sensitive information from unauthorized access but also respecting the privacy of individuals whose data is being analyzed.
To maintain the integrity of data privacy and security, businesses are turning to advanced solutions:
- Implementing robust data governance frameworks
- Deploying artificial intelligence solutions for data surveillance
- Regularly updating compliance measures in line with regulatory changes
The recent guidance update from the Health and Human Services (HHS) on the use of online tracking technologies like Google Analytics underscores the dynamic nature of data privacy regulations. Businesses must remain vigilant, adapting their strategies to safeguard sensitive information and uphold ethical standards.
In the dynamic world of business analytics, staying ahead means embracing the latest trends that drive efficiency and innovation. At OptimizDBA, we specialize in providing faster data solutions and database optimization consulting that can revolutionize your data management. Our proprietary methodologies have been proven to significantly increase performance, with transaction speeds often surpassing industry standards by 100 to 1000 times. Don't let your business fall behind; visit our website to learn how we can help you achieve unparalleled data processing speeds and take your analytics to the next level.
Conclusion
In the fast-paced world of modern business, the role of analytics has become central to decision-making processes. As we have explored throughout this article, the ability to turn vast amounts of data into actionable insights is a game-changer for organizations seeking to innovate, optimize, and compete. Analytics provides a lens through which businesses can view their operations, customer behaviors, and market trends with clarity and precision. By embracing the power of analytics, companies are not only making more informed decisions but are also positioning themselves to respond proactively to the ever-changing business landscape. The future of business is data-driven, and analytics is the key to unlocking that potential.
Frequently Asked Questions
How has business analytics evolved over time?
Business analytics has evolved from simple descriptive analytics to more complex predictive and prescriptive analytics. This evolution is driven by the rise of big data and machine learning, allowing businesses to not only understand past performance but also forecast future trends and prescribe actionable solutions.
What role does data analytics play in decision-making?
Data analytics plays a crucial role in decision-making by providing actionable insights from data mining and analysis. It enables businesses to make informed decisions based on data-driven evidence, identify successful strategies, and drive efficiency through understanding the root causes of performance issues.
What are some challenges in interpreting data for business decisions?
Challenges in interpreting data include dealing with large volumes of data, ensuring data quality and accuracy, overcoming biases in data analysis, and translating complex data insights into actionable business strategies.
How can predictive analytics provide a competitive advantage?
Predictive analytics can provide a competitive advantage by forecasting market trends and consumer behavior, assessing risks, and enhancing customer experiences with predictive insights, enabling businesses to make proactive decisions.
What is prescriptive analytics, and how does it guide business actions?
Prescriptive analytics is an advanced form of analytics that uses data to recommend actions that can lead to desired outcomes. It helps in optimizing operations, scenario planning, and automating decision processes through AI, guiding businesses toward the most effective strategies.
How does analytics impact marketing strategies?
Analytics significantly impacts marketing strategies by enabling personalization, targeted campaigns, and measurement of ROI. It also leverages social media and web analytics to refine marketing efforts and maximize their effectiveness.
Why is building a data-centric organizational culture important?
Building a data-centric organizational culture is important because it fosters data literacy among employees, encourages collaborative data sharing, and ensures that leadership promotes the use of analytics in strategic planning and decision-making.
What are some emerging trends in business analytics?
Emerging trends in business analytics include the integration of the Internet of Things (IoT) with analytics, advancements in real-time data processing, and a growing focus on ethical considerations and data privacy in the handling and analysis of data.