The Role of Predictive Analytics in Strategic Planning
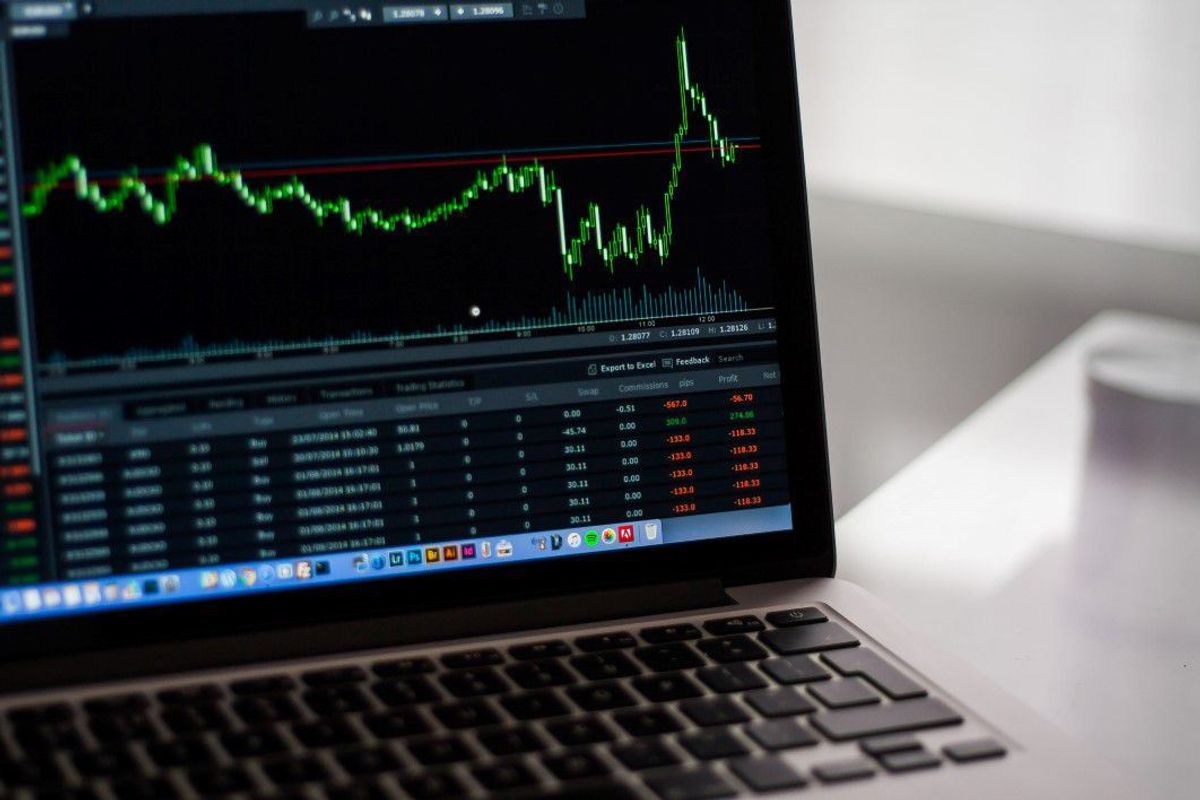
Anticipating Market Trends
In the realm of strategic planning, the ability to anticipate market trends is a game-changer. By leveraging predictive analytics, businesses can gain a competitive edge, making informed decisions that propel them forward. This foresight is not just about understanding current market dynamics but also about predicting future shifts and preparing for them.
Predictive insights play a crucial role in this process, enabling organizations to identify patterns and signals that suggest upcoming changes. For instance, advanced data analysis techniques can reveal consumer behavior trends, shifts in industry standards, or emerging technologies that may disrupt the market.
- Identifying business intelligence strategies to increase sales
- Analyzing customer behavior to predict demand
- Assessing the probability of success for new products
- Identifying and responding to competitive threats
By systematically analyzing data, companies can move from reactive to proactive strategies, ensuring they are always one step ahead in the market.
Ultimately, the integration of predictive analytics into business intelligence strategies is essential for any organization looking to thrive in an ever-evolving marketplace.
Improving Forecast Accuracy
The pursuit of improving forecast accuracy is a central goal of predictive analytics, as it directly influences the quality of decision-making. By leveraging historical data and advanced algorithms, organizations can significantly reduce the margin of error in their predictions. This precision is crucial in areas such as inventory management, financial planning, and demand forecasting.
Forecast accuracy is not just about having the right data; it's also about interpreting it correctly. It involves a continuous cycle of model training, testing, and refinement. Here are some steps to enhance forecast accuracy:
- Establish a baseline using historical data.
- Incorporate a variety of data sources to enrich the model.
- Apply different forecasting techniques and compare results.
- Continuously validate the model against actual outcomes.
By embedding a culture of data validation and model iteration, organizations can foster an environment where predictive analytics becomes more accurate and reliable over time. This iterative process is essential for adapting to market changes and maintaining a competitive edge.
It's important to measure the predictive power of a model by examining its ability to accurately forecast future outcomes. Metrics such as mean squared error or R-squared are commonly used to assess this. As new data becomes available, it's imperative to re-evaluate and refine models to ensure they remain effective in a dynamic business landscape.
Enhancing Competitive Intelligence
In the realm of strategic decision-making, enhancing competitive intelligence is pivotal. By leveraging analytics, organizations can dissect competitor strategies, understand market dynamics, and anticipate shifts that may affect their competitive stance. This proactive approach allows businesses to stay ahead, rather than reactively adapting to changes.
Competitive intelligence is not just about gathering data; it's about transforming that data into actionable insights that can drive strategic advantage.
To effectively enhance competitive intelligence, consider the following steps:
- Identifying key business intelligence strategies to boost sales
- Analyzing and predicting customer behavior patterns
- Conducting thorough competitor analysis
- Tracking organizational progress against benchmarks
- Assessing the likelihood of success for various strategic initiatives
By systematically addressing these areas, companies can construct a robust framework for competitive intelligence that informs strategic planning and operational adjustments.
Data-Driven Decision Making: Transforming Intuition into Insight
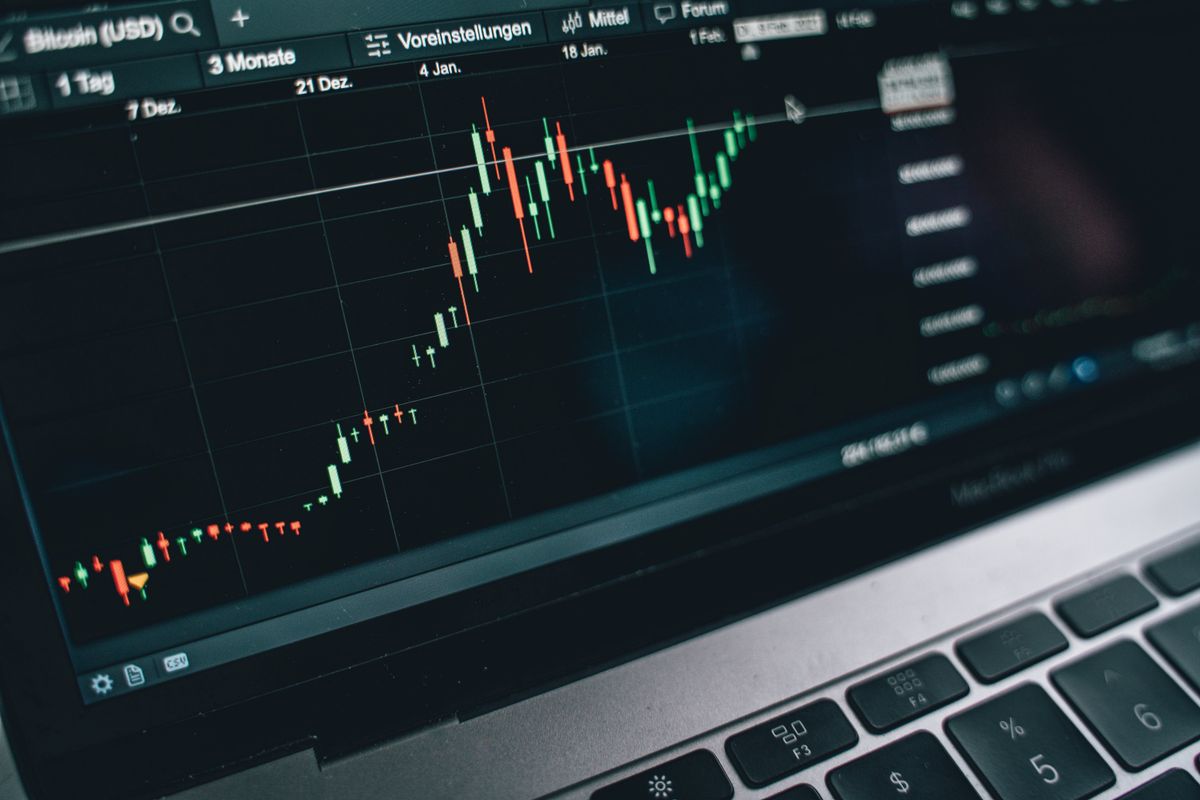
The Importance of Data Quality
At the heart of predictive analytics lies the undeniable truth that the quality of data determines the quality of insights. High-quality data is the cornerstone for accurate predictions and informed decision-making. Without it, even the most sophisticated algorithms can produce misleading results.
To maintain integrity and reliability, data quality management protocols must be rigorously followed from collection through to analysis. This ensures that the data is not only accurate but also relevant and timely for the decisions at hand.
- Establishing reliable data collection processes
- Ensuring adherence to data privacy regulations
- Maintaining data integrity and protecting sensitive information
The pursuit of data quality is a continuous process, involving regular checks and balances to safeguard against inaccuracies and inconsistencies.
Businesses that prioritize data quality can expect to see tangible benefits, such as improved decision-making, cost reduction, and enhanced customer satisfaction. These outcomes are not just theoretical; they are supported by numerous case studies across various industries.
Integrating Data into Decision Processes
Integrating data into decision processes is a critical step in ensuring that strategic choices are both informed and impactful. By leveraging predictive analytics, organizations can transform vast amounts of raw data into actionable insights. This integration allows for a more structured approach to decision-making, where intuition is supplemented with concrete evidence.
- Make informed decisions
- Optimize business processes
- Greater transparency
- Task sharing
The process of integrating data into decision-making can be distilled into several key steps:
- Define Objectives
- Identify and Collect Data
- Organize and Explore Data
- Perform Data Analysis
- Draw Conclusions
- Implement and Evaluate
Each step is crucial in building a robust data-driven strategy. For instance, defining clear objectives sets the stage for what data needs to be collected, while the subsequent analysis and evaluation ensure that the insights gained are effectively translated into business actions.
Ensuring that every decision is backed by data not only enhances the accuracy of predictions but also provides a solid foundation for continuous improvement and strategic agility.
In the context of predictive analytics, it is essential to maintain a balance between data-driven insights and domain expertise. While data provides the quantitative backing, the qualitative insights from experienced professionals are invaluable in interpreting the data correctly and making nuanced decisions.
Balancing Data with Expertise
In the realm of decision-making, the synergy between data analytics and human intuition is paramount. The interplay between analytical and intuitive decision making is about combining the two to create a more complete picture to inform strategy. While data provides a quantitative foundation, expertise adds qualitative nuances that data alone may overlook.
It's essential to recognize that data is not infallible. Experts bring to the table years of experience and contextual knowledge that can guide the interpretation of data. Here are some key considerations for effectively balancing the two:
- Acknowledge the strengths and limitations of both data and intuition.
- Encourage collaboration between data scientists and domain experts.
- Use data to inform decisions, but allow room for expert judgment.
By fostering a culture that values both data-driven insights and expert intuition, organizations can enhance their decision-making processes and achieve a more holistic approach.
Ultimately, the goal is to leverage the best of both worlds, ensuring that decisions are well-informed and consider all relevant factors. This balance can lead to more robust strategies and a competitive edge in the marketplace.
Best Practices for Implementing Predictive Analytics
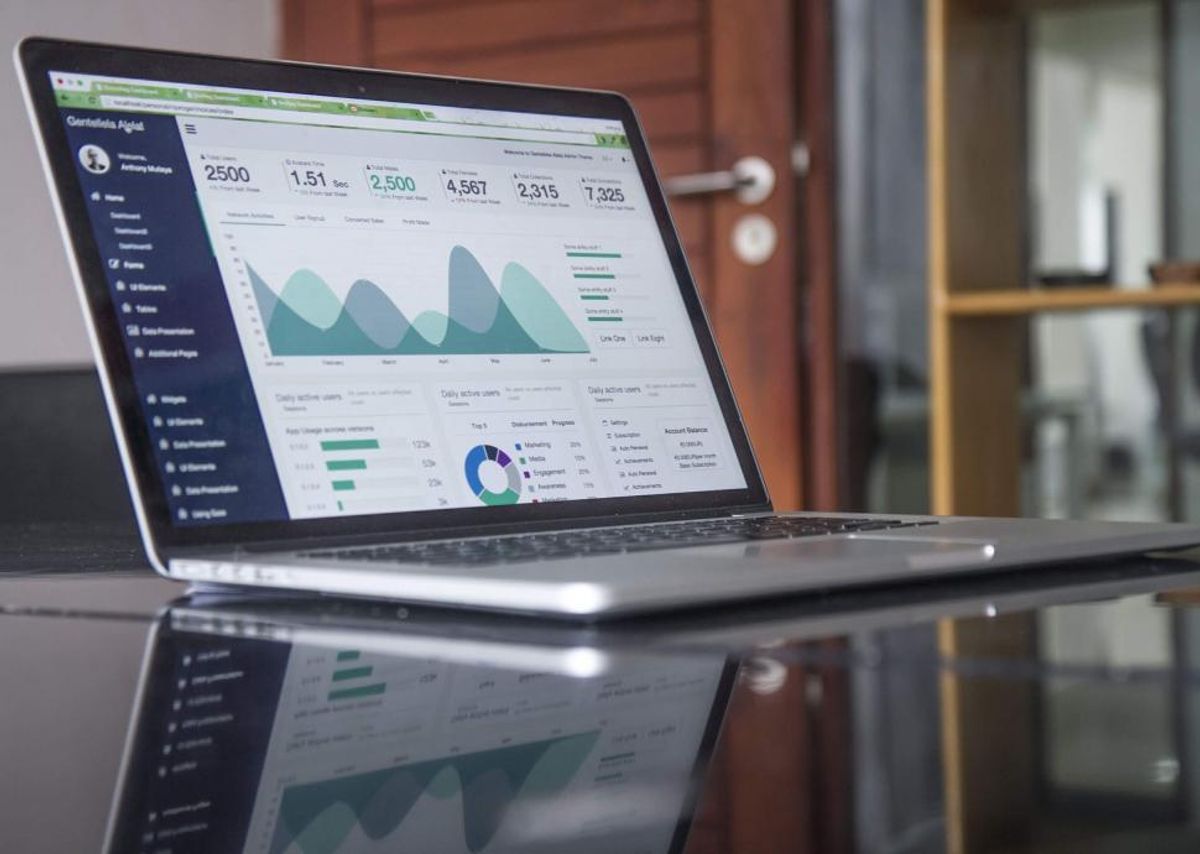
Defining Clear Objectives and Key Metrics
Before embarking on the journey of predictive analytics, it is essential to define clear objectives and pinpoint the key metrics that will serve as benchmarks for success. Consider the specific challenges or questions you aim to tackle with predictive analytics, ensuring that your efforts are in harmony with overarching business objectives.
Once objectives are set, the next step is to establish the metrics that will gauge the effectiveness of your predictive models. These metrics must adhere to the SMART criteria—specific, measurable, achievable, relevant, and time-bound. This strategic approach allows for a structured evaluation of your analytics initiatives and a clear path to measure their impact.
By setting clear objectives and key metrics, you create a foundation for success, enabling a focused and quantifiable assessment of your predictive analytics efforts.
Remember, the metrics you choose should reflect the progress towards your goals and provide actionable insights. Here's a simple list to guide you in selecting your metrics:
- Specificity: Choose metrics that are directly related to your objectives.
- Measurability: Ensure that you can quantify the progress made.
- Achievability: Set realistic targets that can be reached.
- Relevance: Align metrics with your strategic goals.
- Timeliness: Define the timeframe for achieving the targets.
Data Collection and Preparation
The process of data collection and preparation is a critical step in the journey towards unlocking the full potential of predictive analytics. It is during this phase that the groundwork for insightful analysis is laid. Accurate and high-quality data is the foundation of effective predictive analytics. Collecting relevant data from various sources is crucial in building robust predictive models. It is imperative to ensure that your data collection process is well-designed and comprehensive, capturing the necessary variables that can influence the outcome you are trying to predict.
Once collected, the data must undergo meticulous preparation. This includes cleaning the data, handling missing values, and addressing any inconsistencies or errors. Feature engineering techniques are pivotal in transforming and creating new variables that may enhance the predictive power of your models. Techniques such as normalization, feature scaling, and one-hot encoding are essential to ensure that the data is in a suitable format for analysis.
The success of predictive analytics is heavily dependent on the quality of data. Regular assessment and prompt addressing of data quality issues are non-negotiable practices.
Here is a brief overview of the steps involved in data collection and preparation:
- Determine the information relevant to your business goals.
- Establish clear metrics for data analysis.
- Categorize data to facilitate comparison and visualization.
- Share reports with relevant departments for collaborative insight generation.
Choosing the Right Models and Algorithms
Selecting the appropriate models and algorithms is a pivotal step in mastering predictive analytics. The choice hinges on the nature of the problem, the data at hand, and the need for model interpretability. For instance, linear regression offers simplicity and ease of understanding, while neural networks provide complex pattern recognition capabilities.
- Linear Regression: Simple and interpretable
- Decision Trees: Hierarchical and intuitive
- Random Forests: Robust and handles overfitting well
- Neural Networks: High capacity for complexity
It's essential to weigh the strengths and limitations of each option. A simpler model may be preferable when interpretability is paramount. Conversely, complex models might be the go-to for intricate patterns, despite being less transparent. The evaluation and comparison of algorithms is key to finding the one that delivers the best performance for your specific dataset.
By adhering to best practices, you empower your decision-making with models that yield accurate and effective insights. Continuous refinement and evaluation are crucial as new data emerges, ensuring your analytics initiatives stay relevant and impactful.
Optimizing Learning and Development with Predictive Insights
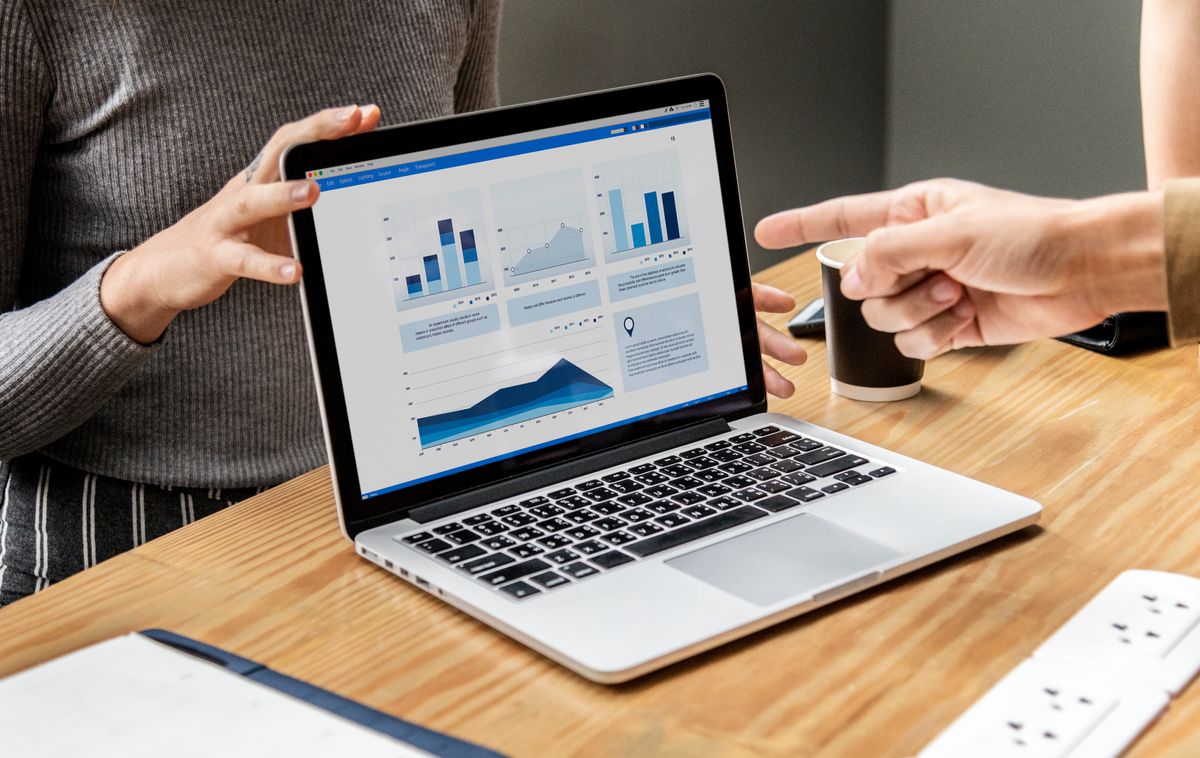
Identifying High-Potential Employees
Harnessing the power of predictive analytics in the realm of human resources enables organizations to identify high-potential employees with greater precision. By analyzing data on employee skills, performance, and potential, HR professionals can pinpoint those individuals who are most likely to succeed in advanced roles or leadership positions. This proactive approach not only aids in succession planning but also ensures that investments in employee development are strategically allocated.
Predictive analytics transforms the traditional approach to talent management by providing actionable insights that guide the nurturing of future leaders.
The following are key indicators often used to identify high-potential employees:
- Consistent top performance in current roles
- Demonstrated ability to learn and adapt quickly
- Strong leadership competencies and interpersonal skills
- Alignment with the company's core values and culture
By focusing on these indicators, organizations can create a robust pipeline of talent ready to step into critical roles, thereby securing their competitive edge in the market.
Predicting Training Needs
In the realm of learning and development (L&D), predictive analytics plays a crucial role in forecasting future skill gaps within an organization. By meticulously analyzing data on employee performance, training completion rates, and industry trends, L&D professionals can pinpoint areas that may require additional training or upskilling. This foresight not only enables proactive planning but also ensures that the organization remains competitive and adaptable.
Predictive analytics empowers organizations to transform reactive training into a strategic asset, aligning L&D initiatives with future business objectives.
Optimize learning interventions can be significantly enhanced through predictive analytics. By tailoring learning experiences to individual learner characteristics, past performance, and other relevant factors, the effectiveness of training programs is maximized, leading to improved outcomes and a more skilled workforce.
While the potential of predictive analytics in L&D is immense, it is not without its challenges. It is essential to consider the following:
- Ensuring the quality and relevance of data used for predictions.
- Balancing the insights from analytics with the wisdom and experience of L&D experts.
- Addressing privacy concerns and ethical considerations in data handling.
Optimizing Learning Interventions
Predictive analytics is revolutionizing the way learning and development (L&D) strategies are formed. By analyzing learner data, such as performance history and individual characteristics, L&D professionals can tailor learning interventions to meet the unique needs of each learner. This personalized approach not only enhances the learning experience but also maximizes the effectiveness of educational programs.
Integrating predictive analytics into strategic decision-making processes empowers businesses to anticipate market changes, optimize operations, and drive innovation for competitive edge and operational excellence.
To ensure content remains engaging and relevant, continuous analysis of learner feedback is crucial. This allows for the identification of areas where learning materials may require updates or improvements. Additionally, by tracking and measuring key metrics, the success of L&D initiatives can be evaluated, leading to ongoing refinement and improvement of learning strategies.
Here are some key steps to optimize learning interventions:
- Identify the most effective learning interventions for individual learners.
- Tailor learning experiences based on learner data and preferences.
- Continuously update and improve learning content to align with learner needs.
- Measure the impact of interventions and refine strategies accordingly.
The Impact of Real-Time Analytics on Decision-Making
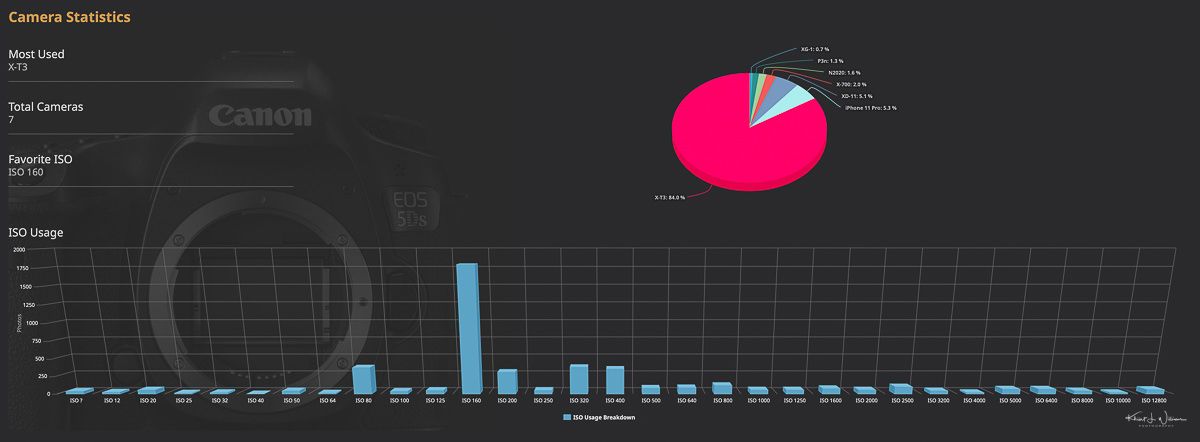
The Need for Up-to-Date Data
In the dynamic landscape of business, up-to-date data is the lifeblood of predictive analytics. It's not just about having data; it's about having the right data at the right time. Real-time data feeds into predictive models, ensuring that the insights generated are relevant and actionable.
Predictive analytics and real-time data drive organizational agility through informed decision-making, risk management, and strategic adaptability in response to market shifts.
The continuous influx of fresh data helps organizations to stay ahead of the curve, adapting to new market conditions and customer behaviors as they unfold. Here are some key considerations for maintaining up-to-date data:
- Regularly review and update data sources.
- Implement automated data collection and processing systems.
- Ensure data relevance by aligning it with current business objectives.
Without current data, businesses risk making decisions based on outdated information, which can lead to missed opportunities and strategic missteps.
Leveraging Real-Time Insights
The advent of real-time analytics has revolutionized the way organizations approach decision-making. With the ability to provide immediate insights, businesses can respond to changes and opportunities with unprecedented speed. This agility translates into a more dynamic and responsive customer experience, as well as more efficient risk and project management.
- Immediate insights lead to faster response times.
- Enhanced customer experience through dynamic interaction.
- Integration of AI improves risk and project management.
Real-time analytics not only supports operational efficiency but also offers a strategic edge in today's competitive market. The integration of AI into decision-making processes is pivotal for staying ahead.
The benefits of leveraging real-time insights are clear, yet they require a robust infrastructure that can handle the demands of continuous data analysis. Ensuring system performance and reliability is crucial for maintaining the flow of up-to-date information that drives strategic decisions.
Challenges in Real-Time Data Analysis
Real-time data analysis offers a competitive edge by providing immediate insights, but it also introduces several challenges. Scalability issues arise as the volume of data and number of users grow, demanding robust infrastructure that can handle the load without compromising performance. Ensuring control and security over this data is paramount, as real-time systems are often more vulnerable to unauthorized access.
Another significant challenge is aligning analytics with business goals. Real-time analytics must be designed to support strategic objectives, requiring a clear understanding of what information is most valuable to decision-makers. This alignment ensures that the analytics serve a purpose beyond just the collection of data.
The process of managing data quality is critical in real-time analysis. Without high-quality data, the insights derived can be misleading, leading to poor decision-making. It is essential to establish protocols for data quality management throughout the data lifecycle.
Lastly, the cost of implementing and maintaining real-time analytics can be substantial. Organizations must balance the benefits against the expenses to manage cost effectively.
Navigating the Challenges of Predictive Analytics
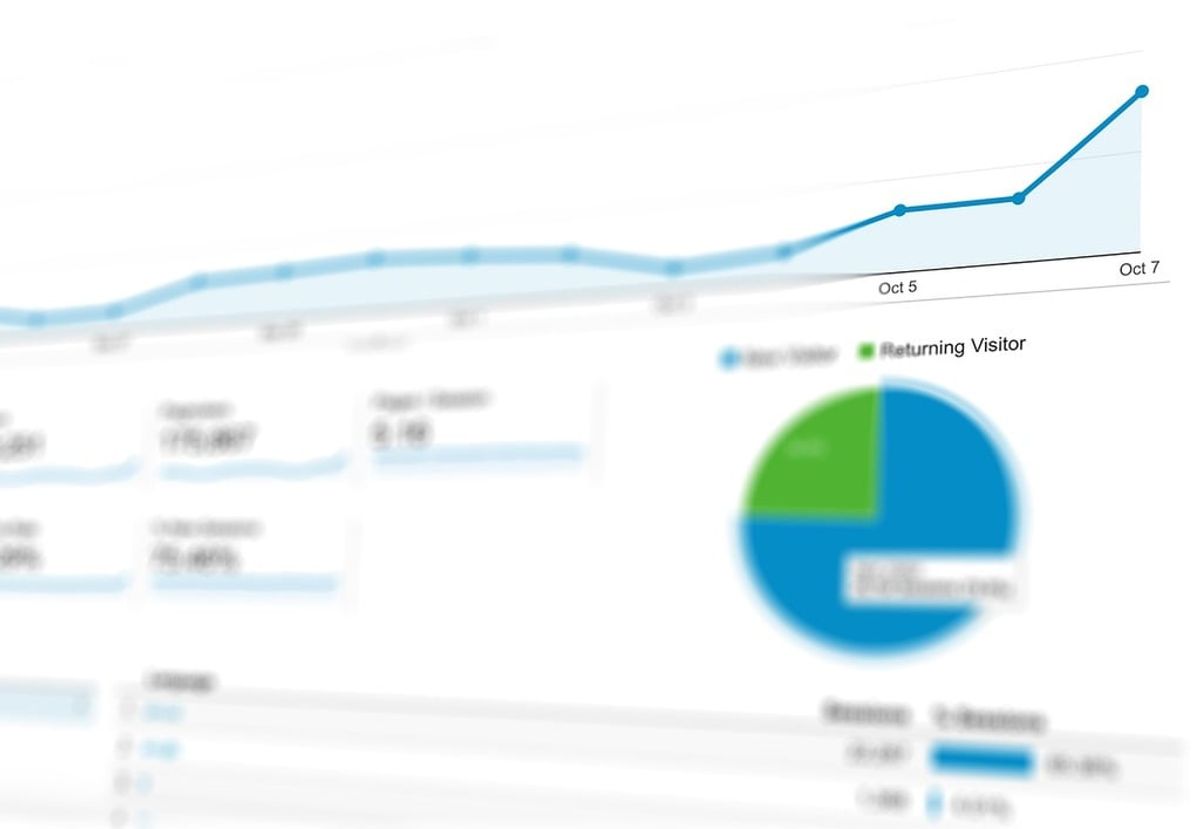
Ensuring Data Privacy and Security
In the realm of predictive analytics, the safeguarding of data privacy and security is paramount. High-quality, relevant data is the cornerstone of accurate predictions, and it is imperative for organizations to establish robust data collection processes that comply with data privacy regulations. Protecting sensitive information and maintaining data integrity are not just regulatory requirements but also critical for maintaining trust and the value of data.
Data security is a significant concern, especially given the rise in cyber-attacks affecting businesses of all sizes. Implementing security measures that address as many vulnerabilities as possible is essential. The importance of data security is a constant, extending well into the future, and is a key factor in the success of predictive analytics initiatives.
Ensuring secure access to data with robust security protocols is not only about compliance but also about preserving the integrity and value of the data itself.
The following list outlines the core aspects of data privacy and security in predictive analytics:
- Adherence to data privacy regulations
- Implementation of data quality management protocols
- Robust security protocols to prevent unauthorized access
- Continuous monitoring and updating of security measures
- Education and training on data security best practices
Overcoming Data Silos and Integration Issues
In the realm of predictive analytics, the integration of disparate data sources is paramount. Data silos present a significant barrier to the seamless flow of information, which is essential for generating accurate predictions and strategic insights. To address this, organizations must adopt a holistic approach to data management, ensuring that all relevant data is accessible and interoperable.
Collaboration across departments and the use of centralized data platforms can help break down these silos. Here are some steps to consider:
- Establishing a unified data governance framework
- Implementing data integration tools and platforms
- Encouraging cross-functional teams to share data and insights
By fostering an environment where data is shared and integrated, companies can leverage the full potential of predictive analytics to drive decision-making.
Adapting to new technologies such as AI and business intelligence tools is also crucial. These technologies not only facilitate the integration of data but also enhance the ability to analyze and visualize it for better decision-making. As the snippet suggests, managing data quality and literacy is essential for the successful application of predictive analytics in strategic planning.
Addressing the Skills Gap in Analytics
The integration of predictive analytics into Learning & Development (L&D) is a strategic move to forecast future skill gaps and ensure organizational agility. By analyzing trends in employee performance and industry dynamics, L&D professionals can proactively identify areas requiring additional training or upskilling.
Forecasting skill gaps with predictive analytics allows for a more targeted approach to talent development, aligning learning initiatives with the evolving needs of the business. This foresight is crucial in maintaining a competitive edge and fostering a culture of continuous learning.
By leveraging predictive analytics, organizations can transform reactive training into a strategic asset, optimizing learning outcomes and driving success.
However, the journey to effective analytics utilization is not without its challenges. It is essential to consider the following points:
- Ensuring the quality of data for accurate predictions
- Developing or acquiring the necessary analytical skills
- Integrating predictive insights into the broader L&D strategy
Addressing these considerations is key to unlocking the full potential of predictive analytics in bridging the skills gap.
The Evolution of Self-Service Business Analytics
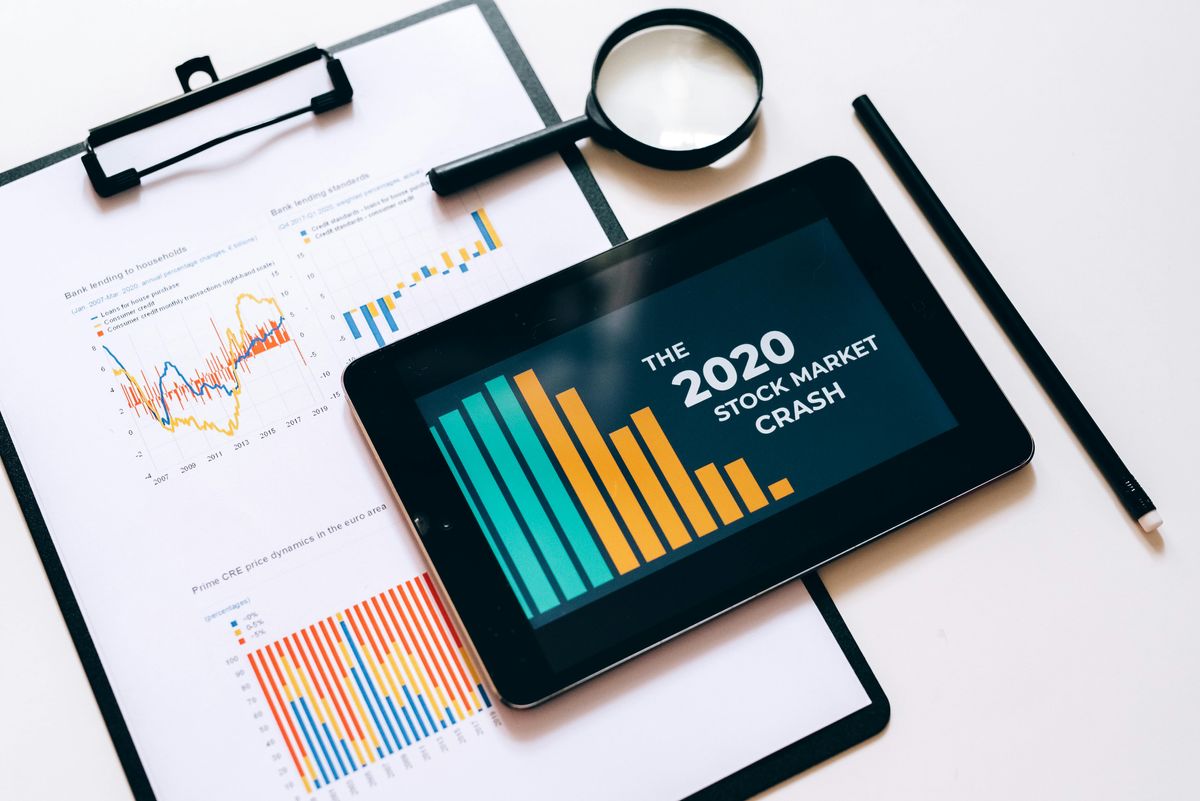
Empowering Non-Technical Users
The advent of self-service analytics has been a game-changer in democratizing data analysis. It empowers users throughout an organization to independently explore and analyze data, removing the traditional barriers of technical expertise and IT dependency. This shift not only accelerates decision-making but also fosters a culture of informed insights across various departments.
Key features of self-service analytics platforms include:
- Interactive dashboards and customized reports for visualizing information
- Advanced analytics capabilities, such as machine learning and data mining
- Tools for collaboration, allowing users to share insights and analyses
- Flexibility for mobile devices, ensuring access to data on-the-go
- Robust security protocols to maintain control and ensure data privacy
By tailoring the presentation of data and analytics tools to the audience's level of understanding, organizations can maximize the relevance and impact of insights for each user group.
The Power of Self-Service Analytics: Empowering Users - Akkio, highlights the transformative potential of these tools. They not only provide greater transparency in business processes but also enable task sharing and management of the overall situation, ensuring that no one is left clueless throughout the entire process.
The Role of Visualization Tools
In the realm of analytics, visualization tools are indispensable for translating complex data sets into clear, actionable insights. By employing charts, graphs, and interactive dashboards, these tools enable stakeholders to grasp intricate patterns and correlations at a glance. For instance, a well-designed bar chart can reveal sales trends over time, while a heat map might highlight geographical variations in consumer behavior.
Business intelligence tools not only facilitate the creation of these visual representations but also support data mining efforts to unearth underlying patterns within vast data repositories. The ability to visualize data democratizes the analytical process, empowering even non-technical users to engage with data and derive meaningful conclusions.
Visualization tools serve as a bridge between raw data and strategic action, allowing decision-makers to quickly identify and respond to emerging trends and anomalies.
The following table exemplifies how visualization tools can categorize data for better comprehension:
Metric | Q1 | Q2 | Q3 | Q4 |
---|---|---|---|---|
Revenue | $20M | $25M | $30M | $35M |
Customer Growth | 5% | 7% | 10% | 12% |
Market Share | 15% | 16% | 18% | 20% |
As the volume and complexity of data continue to grow, the role of visualization tools in making sense of this information becomes ever more critical. They are the lens through which data is transformed into strategic knowledge, guiding businesses towards informed decision-making.
Ensuring Data Governance in Self-Service Models
In the realm of self-service analytics, data governance is the cornerstone that ensures the integrity and security of data. Establishing a data governance committee is a pivotal step, tasked with the oversight of data quality, security, and compliance. This committee plays a critical role in maintaining the balance between user autonomy and control over the data ecosystem.
To effectively manage data governance, organizations must adhere to a set of principles:
- Control and Security: Implementing robust security protocols to secure access to data.
- Scalability: Ensuring systems can handle increasing data volumes and user numbers.
- Real-Time Analytics: Providing decision-makers with up-to-date data for timely insights.
- Predictive Analytics: Leveraging historical data to forecast future trends and inform decisions.
Data governance in self-service models is not just about control; it's about enabling users to make informed decisions while safeguarding the data landscape.
By integrating these governance measures, companies can achieve greater transparency and task sharing, allowing for a clear assignment of responsibilities within the organization. This ensures that self-service analytics tools are used effectively, responsibly, and in alignment with the company's strategic goals.
Scalability and Control in Analytics Infrastructure
Managing Increasing Data Volumes
As organizations amass larger quantities of data, the challenge of managing increasing data volumes becomes critical. Scalability is essential to accommodate the growth, ensuring systems can handle the influx without performance degradation. Scalability not only pertains to storage but also to the ability to process and analyze data efficiently.
To maintain a high level of data quality, it's important to implement best practices such as data profiling and dealing with missing values. These steps help in preserving the integrity of data, which is foundational for generating reliable insights. A structured approach to data management can include:
- Focusing on specific objectives
- Selecting high-quality data
- Utilizing tools like pivot tables for analysis
- Establishing protocols for data quality management
Ensuring secure access to data with robust security protocols is paramount. As data volumes grow, so does the risk of breaches, making control and security non-negotiable.
In the context of predictive analytics, handling large data sets is not just about storage but also about the capability to derive forecasts and trends that inform decision-making. The use of technologies like business intelligence and analytics platforms can provide greater transparency and facilitate task sharing, allowing for a more comprehensive management of the overall situation.
Maintaining Control with Robust Security Protocols
In the realm of analytics infrastructure, maintaining control is paramount, especially when it comes to security protocols. As businesses harness analytics for smarter decision-making, the need for robust security measures becomes non-negotiable to protect against cyber-attacks and unauthorized access.
Ensuring secure access to data is not just about technology; it's about safeguarding the trust and integrity that are the foundation of any data-driven organization.
To achieve this, organizations must implement a multi-layered security strategy that includes but is not limited to:
- Regular updates and patches to security software
- Strict access controls and authentication mechanisms
- Continuous monitoring for suspicious activities
- Employee training on security best practices
These steps help close vulnerabilities and maintain the market value of the company by preventing security breaches that could lead to exploitation or loss of data.
Ensuring System Performance and Reliability
In the realm of predictive analytics, system performance and reliability are not just technical requirements; they are foundational to the trust and confidence stakeholders place in the insights generated. Ensuring optimal system performance is a multifaceted challenge that involves not only the selection of robust hardware and software but also the design of scalable architectures that can handle growing data volumes and complex analytics workloads.
Scalability is a key consideration, as systems must be able to expand in capacity without compromising performance. This includes both vertical scaling (adding more power to an existing machine) and horizontal scaling (adding more machines to a system). Below is a list of critical factors to monitor to maintain system reliability:
- Continuous monitoring of system health
- Regular updates and maintenance schedules
- Load balancing to prevent any single point of failure
- Disaster recovery plans and backups
- Performance benchmarks and stress testing
By proactively addressing these factors, organizations can create a resilient analytics infrastructure that delivers consistent and reliable results, even under high demand. It is essential to not only establish these practices but to also regularly review and update them to adapt to new challenges and technological advancements.
The Art of Feature Selection and Engineering in Predictive Models
Identifying Predictive Features
To harness the Power of Predictive Analytics for informed decision-making, it's crucial to identify the features that significantly influence the predicted outcome. This process is not just about selecting the right data points but understanding their relevance and impact on the model's accuracy.
- Begin with a comprehensive analysis of the data to discern patterns and correlations.
- Eliminate redundant or non-informative features to streamline the model.
- Retain features that are critical for making accurate predictions.
By meticulously selecting features, you ensure the model's predictive insights are both relevant and reliable, providing a solid foundation for strategic decisions.
Remember, the goal is to provide predictive insights that are actionable and beneficial for the business. Continuous refinement and evaluation of the feature set are essential as new data emerges, maintaining the model's relevance in an ever-changing environment.
Techniques for Feature Engineering
Feature engineering is a critical step in the predictive analytics process, where data cleaning and preprocessing ensure the foundation for accurate predictions. By transforming raw data into a format that's more suitable for modeling, analysts can significantly enhance a model's accuracy and interpretability. Common techniques include:
- Normalization: Adjusting values to a common scale without distorting differences in the ranges of values.
- Standardization: Rescaling data to have a mean of zero and a standard deviation of one.
- Feature Interaction: Combining features to capture complex relationships, often through mathematical operations.
- Dimensionality Reduction: Techniques like PCA reduce feature space complexity while preserving the most informative components.
It's essential to remember that not all features contribute equally to a model's predictive power. Some may introduce noise rather than insight. Therefore, the art of feature engineering also involves the careful selection of features that are truly predictive. This process is not a one-time task but an iterative cycle of refinement, where each change's impact on the model's performance is assessed.
By applying these techniques, you can uncover hidden patterns in the data that are pivotal for forecasting future events. The iterative nature of this process ensures continuous improvement and adaptation to new insights.
The Importance of Domain Expertise
In the realm of predictive analytics, domain expertise is not just an asset; it's a necessity. Experts who understand the nuances of their field can tailor models to reflect the complexities of real-world scenarios. This deep knowledge is crucial for identifying which features of the data can predict outcomes with the greatest accuracy.
Domain expertise bridges the gap between raw data and actionable insights, ensuring that predictive models are both relevant and reliable.
For instance, in healthcare, a domain expert would discern which patient information is most indicative of health risks. In finance, they might pinpoint economic indicators that forecast market shifts. Below is a list of areas where domain expertise significantly enhances predictive analytics:
- Healthcare: Recognizing patterns in patient data for early diagnosis.
- Finance: Identifying market indicators for investment strategies.
- Retail: Understanding consumer behavior to optimize stock levels.
- Manufacturing: Predicting machinery maintenance to prevent downtime.
Collaboration between data scientists and domain experts ensures that the final predictive model is not only technically sound but also contextually informed. Without this collaboration, there's a risk of developing models that are technically accurate but practically irrelevant.
Measuring the Success of Predictive Analytics Initiatives
Key Performance Indicators for Analytics Projects
To gauge the efficacy of predictive analytics initiatives, it's essential to establish Key Performance Indicators (KPIs) that are in alignment with your strategic objectives. These KPIs should be SMART—specific, measurable, achievable, relevant, and time-bound. Regular tracking of these metrics is vital to understanding the impact and value of your analytics efforts.
Common KPIs include:
- Accuracy of predictions
- Reduction in cost due to optimized processes
- Increase in revenue from data-driven decisions
- Time saved in decision-making processes
- Customer satisfaction and retention rates
It is not enough to simply measure; one must also analyze the trends and patterns within these KPIs to optimize future strategies.
Remember, the selection of KPIs will vary based on the industry and the specific goals of the project. It is crucial to revisit and refine these indicators regularly to ensure they remain aligned with evolving business objectives and market conditions.
Continuous Evaluation and Refinement of Models
The journey of predictive analytics is ongoing, with models requiring regular evaluation and refinement to maintain their effectiveness. This iterative process is essential to adapt to changing conditions and improve the accuracy of predictions over time.
Continuous improvement is not just about tweaking algorithms; it involves a comprehensive review of the entire analytics process, from data collection to model deployment. It's crucial to monitor model performance using appropriate metrics, validate the accuracy of predictions, and adjust models in response to feedback and new data.
- Monitor model performance
- Validate prediction accuracy
- Adjust models as needed
Predictive analytics is a dynamic field that thrives on perpetual optimization. The balance between data-driven insights and human judgment is delicate, and achieving strategic alignment is paramount.
Harnessing tools like Power BI can provide enhanced insights and facilitate the ongoing process of model optimization. By staying vigilant and responsive, organizations can ensure that their predictive analytics systems remain robust and aligned with their strategic objectives.
Case Studies: Impact on Business Outcomes
The culmination of a predictive analytics initiative is often best illustrated through case studies that showcase tangible business outcomes. Walmart, for instance, has leveraged business intelligence strategies to enhance sales and customer behavior analysis, leading to improved decision-making and increased profitability.
Key Takeaways:
- Summarize main findings
- Highlight important points
- Contextualize results
The ability to translate data-driven insights into actionable strategies is the cornerstone of predictive analytics success.
Presenting findings effectively is crucial. It involves not only the clarity of data but also the ability to relate insights to business objectives, ensuring that stakeholders grasp the significance of the analytics efforts. The table below encapsulates the impact of predictive analytics on key business metrics:
Metric | Before Analytics | After Analytics |
---|---|---|
Sales Growth | 5% | 12% |
Customer Retention | 70% | 85% |
Operational Efficiency | 65% | 80% |
By continuously evaluating and refining models, businesses can sustain the momentum of their analytics projects, ensuring that the insights remain relevant and impactful.
Unlock the full potential of your data with OptimizDBA's cutting-edge predictive analytics initiatives. Our proven track record of database optimization and commitment to delivering faster data solutions sets us apart. Don't just take our word for it; experience the unparalleled transaction speeds and performance enhancements that our clients, like Radio-Canada Inc., rave about. Ready to measure success in real-time? Visit our website to learn how we can elevate your data strategy and ensure your analytics initiatives thrive.
Conclusion
In the journey through the transformative landscape of analytics, we have uncovered the pivotal role of predictive insights in shaping the future of decision-making. The power of analytics is not just in the data itself, but in the ability to interpret, understand, and act upon it to drive strategic outcomes. By integrating advanced analytics, AI, and machine learning into business intelligence, organizations can forecast trends, optimize operations, and anticipate the needs of tomorrow. As we have seen, the application of predictive analytics in Learning & Development is just one example of its vast potential. It is imperative for businesses to adopt best practices, ensure robust data management, and continuously refine their models to maintain relevance in a rapidly evolving market. The insights gleaned from predictive analytics are invaluable; they empower leaders to make informed, proactive decisions that can lead to sustainable growth and competitive advantage. As we embrace this data-driven era, the organizations that effectively harness the power of predictive analytics will be the ones to watch, as they will be poised to lead the charge in innovation and success.
Frequently Asked Questions
What is predictive analytics in the context of strategic planning?
Predictive analytics in strategic planning involves using statistical models and forecast techniques to anticipate market trends, improve forecast accuracy, and enhance competitive intelligence, thus aiding in informed decision-making.
How does data-driven decision-making differ from intuitive approaches?
Data-driven decision-making relies on empirical evidence and analytics to guide business decisions, whereas intuitive approaches are based on subjective judgment and experience. Data-driven methods aim to transform intuition into quantifiable insights.
What are some best practices for implementing predictive analytics?
Best practices include defining clear objectives and key metrics, thorough data collection and preparation, and choosing the right models and algorithms to ensure accurate and actionable insights.
How can predictive analytics optimize learning and development?
Predictive analytics can identify high-potential employees, predict training needs, and optimize learning interventions, thereby enhancing the effectiveness of L&D programs.
What is the impact of real-time analytics on business decision-making?
Real-time analytics provides up-to-date data, enabling decision-makers to leverage timely insights for immediate actions, although it presents challenges such as ensuring data accuracy and handling large data volumes.
What challenges are associated with predictive analytics?
Challenges include ensuring data privacy and security, overcoming data silos and integration issues, and addressing the skills gap in analytics to effectively interpret and utilize the data.
How does self-service business analytics empower users?
Self-service business analytics empowers non-technical users to access, analyze, and visualize data without deep expertise in data science, thus democratizing data analysis within an organization.
What role does feature selection and engineering play in predictive models?
Feature selection and engineering involve identifying the most predictive features and applying techniques to transform raw data into a format that enhances the predictive power of models, often requiring domain expertise.