The Role of Predictive Analytics in Modern Business
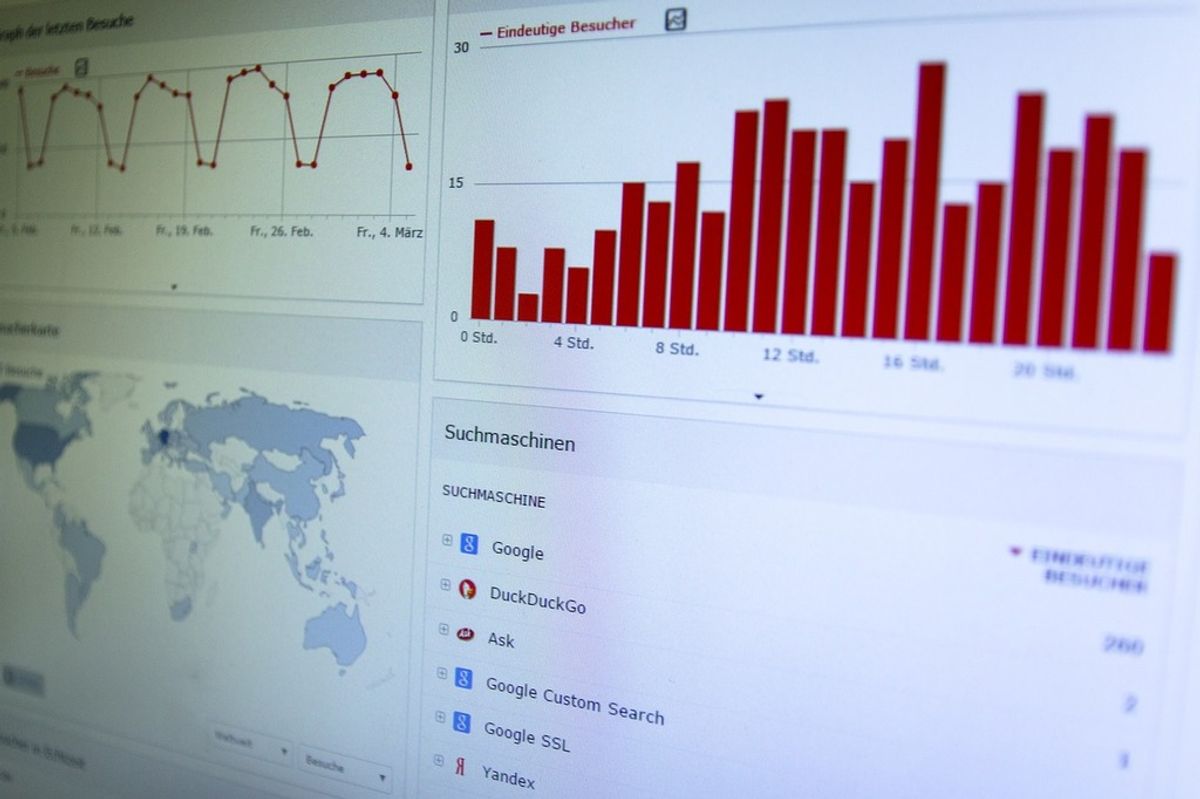
Forecasting Market Trends and Consumer Behavior
The power of predictive analytics lies in its capacity to anticipate market shifts and consumer preferences. By analyzing historical data, businesses can identify patterns that signal upcoming trends. This foresight enables companies to tailor their strategies, ensuring they remain agile and responsive to market demands.
Forecasting market trends and consumer behavior is crucial for staying ahead in a competitive landscape. It involves not just predicting what customers will want, but also when and how they will want it. This can lead to more informed decisions regarding product development, marketing campaigns, and inventory management.
Predictive analytics transforms raw data into actionable insights, empowering businesses to make data-driven decisions that can significantly impact their success.
The benefits of predictive analytics in forecasting include:
- Identification of emerging patterns and trends
- Accurate predictions of future consumer behavior
- Enhanced risk management and mitigation strategies
- Gaining a competitive edge through informed decision-making
For instance, retailers leverage predictive analytics to fine-tune inventory levels, reducing waste and ensuring product availability aligns with consumer demand. This strategic approach not only optimizes operations but also enhances customer satisfaction.
Enhancing Operational Efficiency and Risk Management
Predictive analytics is a game-changer for businesses seeking to enhance operational efficiency and manage risks effectively. By leveraging historical data and advanced algorithms, companies can anticipate future challenges and opportunities, leading to more strategic decision-making processes. Predictive analytics enhances decision-making by analyzing data to forecast outcomes, manage risks, and drive growth. Embrace data-driven strategies for informed decisions and competitive advantage.
This transformative capability enables organizations to make informed decisions swiftly, enhancing operational efficiency and competitive advantage.
Here are some ways predictive analytics contributes to operational efficiency and risk management:
- Automate Data Processing: Streamline data handling to reduce manual intervention and minimize errors.
- Identify Patterns and Anomalies: Detect complex patterns and anomalies, offering new insights into operations and customer behavior.
- Enhanced Risk Assessment: In finance, for example, machine learning models provide a more accurate assessment of loan default risks, leading to smarter lending decisions.
Incorporating predictive analytics into various aspects of business operations not only optimizes current processes but also fortifies the organization's ability to manage potential risks. It is a critical component in building a resilient and agile business model.
Facilitating Strategic Planning and Competitive Advantage
Predictive analytics serves as a cornerstone for strategic planning, providing insights that drive competitive advantage. Organizations that harness predictive analytics can anticipate market shifts and consumer needs more effectively than their competitors.
Gaining a competitive edge is not just about understanding current trends but also about predicting future ones. By leveraging historical data and predictive models, businesses can make proactive decisions that position them ahead of the curve.
- Assessment and Planning: Evaluate current data analysis processes and identify where predictive analytics can add value.
- Seamless Integration: Ensure the integration of predictive analytics is smooth, complementing existing workflows without disruption.
- Gaining a Competitive Edge: Use predictive insights to anticipate market changes and consumer preferences.
- Inventory Management: Employ predictive models to forecast demand and optimize inventory levels, reducing waste and costs.
The strategic use of predictive analytics transforms not only the decision-making process but also fortifies a company's position in the market. It's about creating a future-ready business that can adapt and thrive amidst changing conditions.
Integrating Predictive Analytics into Learning and Development
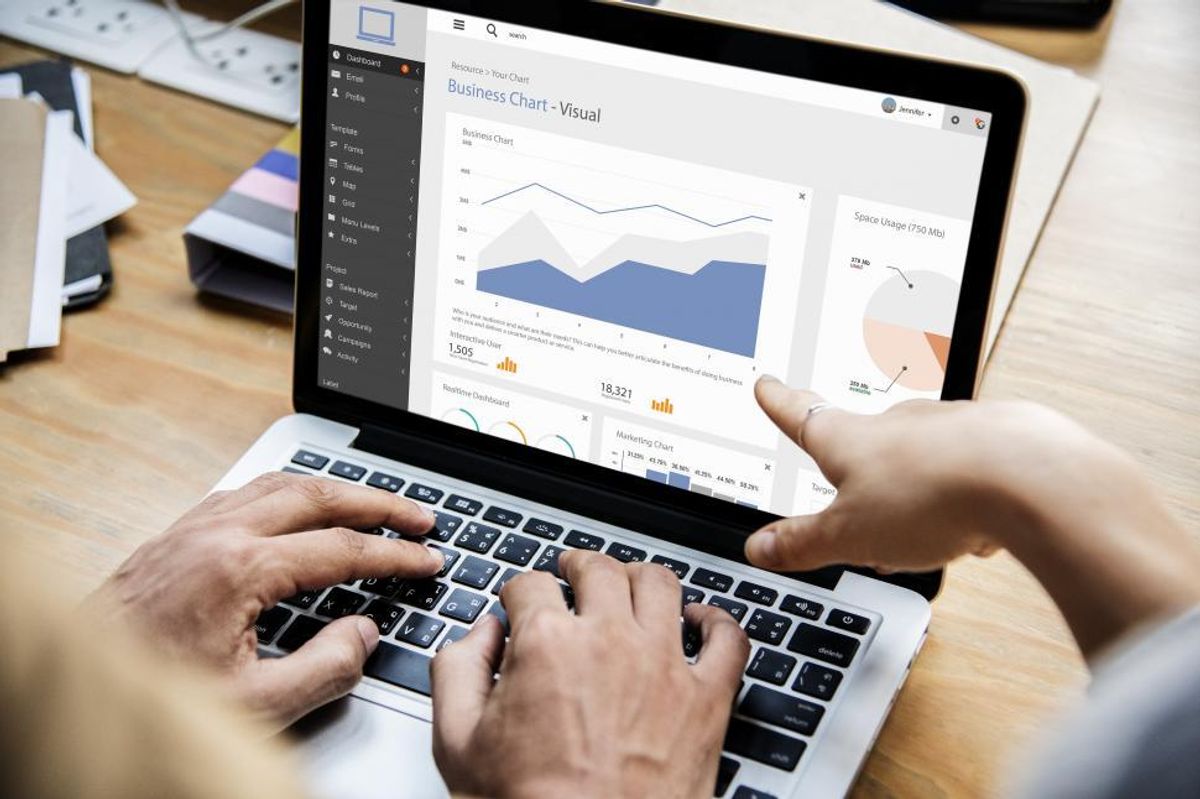
Identifying High-Potential Employees
Leveraging predictive analytics in the realm of Learning & Development (L&D) has become a game-changer for organizations aiming to nurture talent effectively. By analyzing patterns in employee performance data, companies can identify individuals with the potential to excel in more advanced roles or leadership positions. This proactive approach not only prepares a robust pipeline for succession planning but also aligns employee development with the strategic goals of the organization.
Predictive analytics tools empower proactive decision-making. Data-driven decision making enhances operational efficiency and strategic planning, leading to improved customer satisfaction and competitive edge.
The process of identifying high-potential employees typically involves several key steps:
- Assessing past and current performance metrics
- Analyzing employee engagement and behavioral data
- Predicting future performance based on historical trends
By comparing individual employee profiles against the traits of successful leaders within the company, L&D teams can tailor development programs that are both effective and efficient, ensuring that high-potential employees receive the support they need to thrive.
Predicting Training Needs and Outcomes
The integration of predictive analytics into learning and development (L&D) is transforming how organizations approach employee growth. By analyzing patterns in employee performance and industry trends, L&D professionals can proactively identify areas that require additional training or upskilling. This foresight not only keeps the workforce competitive but also aligns with the evolving demands of the market.
Optimizing learning interventions is another area where predictive analytics shines. Tailoring training programs to individual learner characteristics and past performance can significantly enhance the learning experience. This personalized approach not only increases engagement but also maximizes the likelihood of achieving desired outcomes.
Predictive analytics empowers organizations to make informed decisions about where to allocate resources for training, ensuring that investments in L&D yield the highest return on investment (ROI).
Challenges such as ensuring data privacy and addressing the varying needs of employees must be navigated carefully. However, the benefits of a data-driven L&D strategy are clear:
- Anticipating future skill requirements
- Personalizing learning paths
- Measuring the effectiveness of training interventions
- Aligning L&D initiatives with strategic business goals
Optimizing Learning Interventions and ROI
Predictive analytics is revolutionizing the way organizations approach learning and development (L&D). By leveraging data, L&D teams can tailor educational interventions to individual needs, ensuring that each employee receives the most relevant and effective training. This targeted approach not only enhances the learning experience but also maximizes the return on investment (ROI) for training programs.
Predictive analytics empowers organizations to identify high-potential employees, predict training needs, and anticipate market trends for strategic planning, enhancing decision-making and learning interventions. It's a powerful tool for optimizing content, as it provides insights into which materials are most effective and which require refinement to stay aligned with learner needs and market demands.
By continuously measuring and evaluating the success of learning interventions, organizations can make data-driven decisions that lead to improved performance and strategic growth.
Challenges such as ensuring the relevance and engagement of learning materials are addressed by predictive analytics, which can pinpoint areas for improvement. Moreover, the ability to measure and evaluate the success of L&D initiatives allows for ongoing refinement, contributing to a culture of continuous learning and development.
Here are some key considerations when optimizing learning interventions with predictive analytics:
- Personalizing learning experiences to match individual learner profiles.
- Analyzing learner data and feedback to update and improve content.
- Monitoring key metrics to evaluate the impact of learning interventions.
- Refining strategies based on data-driven insights to enhance overall effectiveness.
Best Practices for Implementing Predictive Analytics
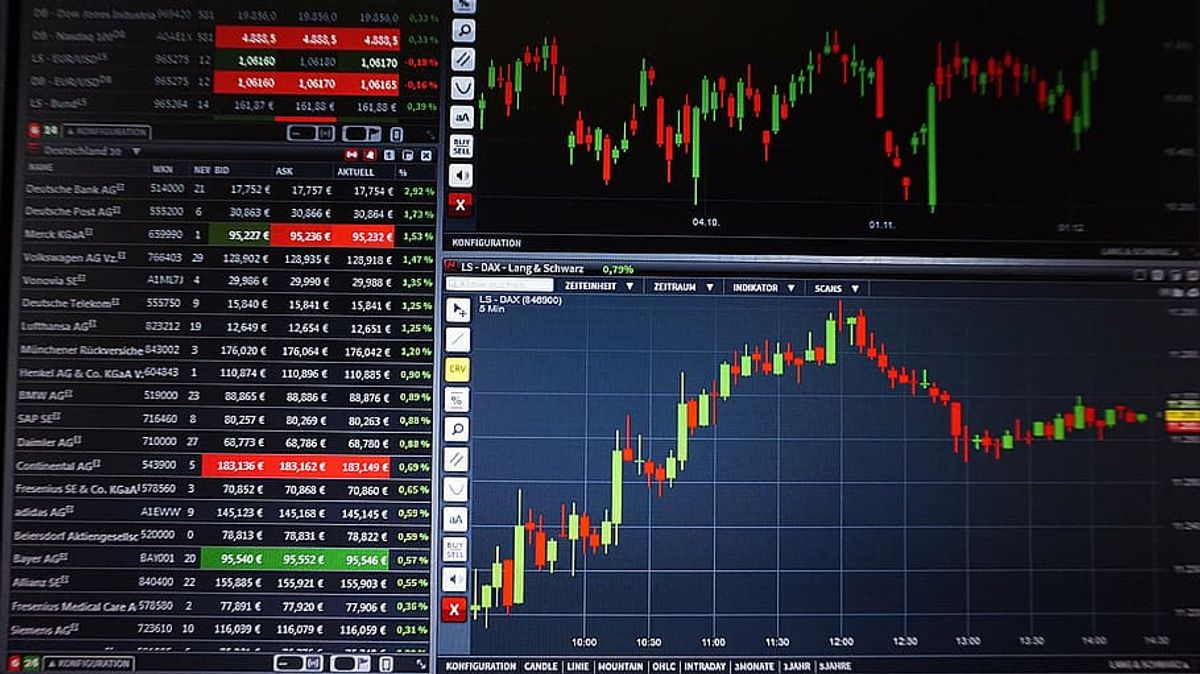
Collaborating Across Disciplines for Model Development
The development of predictive analytics models is a multidisciplinary endeavor that requires the expertise of data scientists, business analysts, and decision-makers. Collaboration across these roles is crucial to ensure that the models provide actionable insights and drive business outcomes.
Data scientists bring technical expertise, business analysts offer a deep understanding of market dynamics, and decision-makers contribute strategic oversight. Together, they form a robust framework for predictive model development. This synergy is not just beneficial but necessary for leveraging data and technology to enhance interdisciplinary collaboration.
By fostering an environment where knowledge and skills are shared, organizations can create models that are not only technically sound but also aligned with business objectives.
Here are some key steps to encourage collaboration:
- Establish clear communication channels among all stakeholders.
- Promote interdisciplinary education and training.
- Implement regular cross-functional meetings to review progress and share insights.
- Utilize collaborative tools and platforms to streamline the model development process.
Ensuring Data Quality and Governance
In the realm of predictive analytics, the adage 'garbage in, garbage out' holds particularly true. High-quality data is the cornerstone of any predictive model's accuracy and reliability. Ensuring data integrity by implementing robust data collection and management systems is not just a technical necessity; it's a strategic imperative.
- Ensure Data Integrity: Regularly clean and validate data sets.
- Compliance with Regulations: Adhere to data protection regulations like GDPR or CCPA.
- Secure Data Practices: Employ encryption and secure access controls.
- Regular Audits: Perform security audits to detect vulnerabilities.
Predictive analytics requires a balance of data insights and human judgment. Continuous improvement, data quality, and strategic alignment are key for success.
While technology can process vast amounts of data, it is the governance frameworks that ensure this data is used ethically and responsibly. Without proper governance, even the most sophisticated analytics can lead to flawed decisions and potential legal issues.
Continuous Model Evaluation and Refinement
The dynamic nature of business environments necessitates that predictive analytics models are not static entities; they require continuous evaluation and refinement. This iterative process ensures that models adapt to new data, trends, and underlying patterns, maintaining their relevance and accuracy over time.
Model performance metrics are essential tools in this process. They provide quantifiable measures of a model's predictive power and can highlight areas for improvement. Common metrics include accuracy, precision, recall, and the F1 score. Depending on the model's purpose, different metrics may be prioritized:
- Accuracy: Measures the proportion of true results among the total number of cases examined.
- Precision: Assesses the proportion of true positive identifications.
- Recall: Evaluates the proportion of actual positives that were correctly identified.
- F1 Score: Balances precision and recall in a single metric.
It is crucial to select the most appropriate evaluation metric based on the specific requirements of your learning and development (L&D) context. A mismatch between the metric and the model's intent can lead to misguided conclusions and actions.
Cross-validation techniques further enhance the robustness of model evaluation by testing the model's ability to perform on unseen data. This approach mitigates the risk of overfitting and provides a more generalizable assessment of model performance. Regularly revisiting and updating the model with new data and insights is a best practice that can significantly improve the outcomes of predictive analytics initiatives.
Technological Advancements in Predictive Analytics
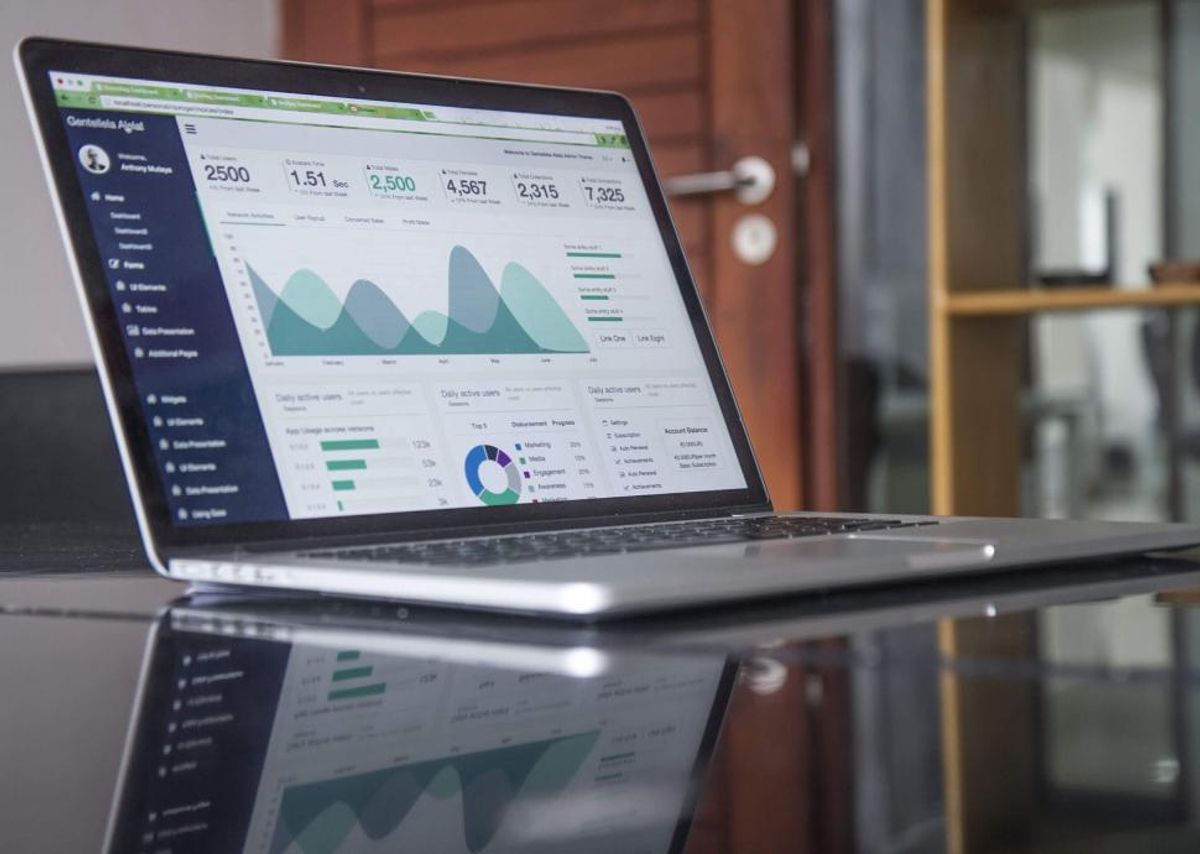
Leveraging Machine Learning and AI
The integration of Artificial Intelligence (AI) and Machine Learning (ML) into predictive analytics has been transformative, enabling businesses to process and analyze vast volumes of data with remarkable speed and accuracy. Machine learning algorithms excel at identifying patterns and trends, often uncovering insights that traditional analysis might miss.
- Autonomous Data Learning: Machines learn from historical data, improving predictions over time.
- Self-Improvement: Algorithms adapt to new data trends autonomously.
- Efficiency: Rapid processing of large datasets facilitates quicker decision-making.
The shift to AI-powered analytics marks a significant leap from manual processes to automated, strategic innovation. This evolution allows businesses to not only react to market changes but also to anticipate them, securing a competitive edge.
Machine learning's self-improvement capability is particularly valuable for adapting to changing market conditions and maintaining the relevance and accuracy of predictive models. As businesses continue to leverage these technologies, they gain new insights and enhance their decision-making processes, ensuring that they remain agile in an ever-evolving business landscape.
Incorporating Big Data and Real-Time Analytics
The integration of Big Data and real-time analytics into predictive analytics is a transformative move for businesses seeking to harness the full potential of their data. Predictive analytics looks at past and present data to make predictions, leveraging the power of AI, machine learning, and data mining to provide actionable insights.
The agility provided by real-time analytics, combined with the comprehensive view offered by Big Data, enables businesses to respond swiftly to market dynamics and customer preferences.
To fully embrace this integration, businesses should consider the following steps:
- Promote AI Literacy within the organization to ensure AI-generated insights are effectively utilized.
- Stay Agile to adapt quickly to real-time analytics that inform business strategies.
- Automate Data Processing to minimize errors and enhance efficiency.
- Identify Patterns and Anomalies to gain new insights into operations and customer behavior.
Emerging Technologies Shaping the Future of Analytics
The landscape of predictive analytics is being transformed by a suite of emerging technologies that promise to redefine how businesses analyze data and make decisions. At the forefront of this transformation is the integration of artificial intelligence (AI), which not only enhances existing analytical capabilities but also paves the way for new forms of strategic decision-making.
As technology continues to evolve, so does the potential of predictive analytics. The use of real-time data and advanced predictive modeling tools is setting a new standard for accuracy and efficiency.
To fully harness these advancements, organizations should consider the following steps:
- Promote AI literacy across all levels of the organization to ensure AI-generated insights are effectively utilized.
- Maintain agility to quickly adapt to real-time analytics that inform business strategies, keeping the organization resilient and competitive.
- Foster collaboration between data scientists, business analysts, and decision-makers to develop models that deliver actionable insights.
AI integration in business analytics tools has democratized access to predictive analytics, enabling businesses to anticipate market shifts and customer behavior with remarkable precision.
Overcoming Challenges to Data-Driven Decision Making
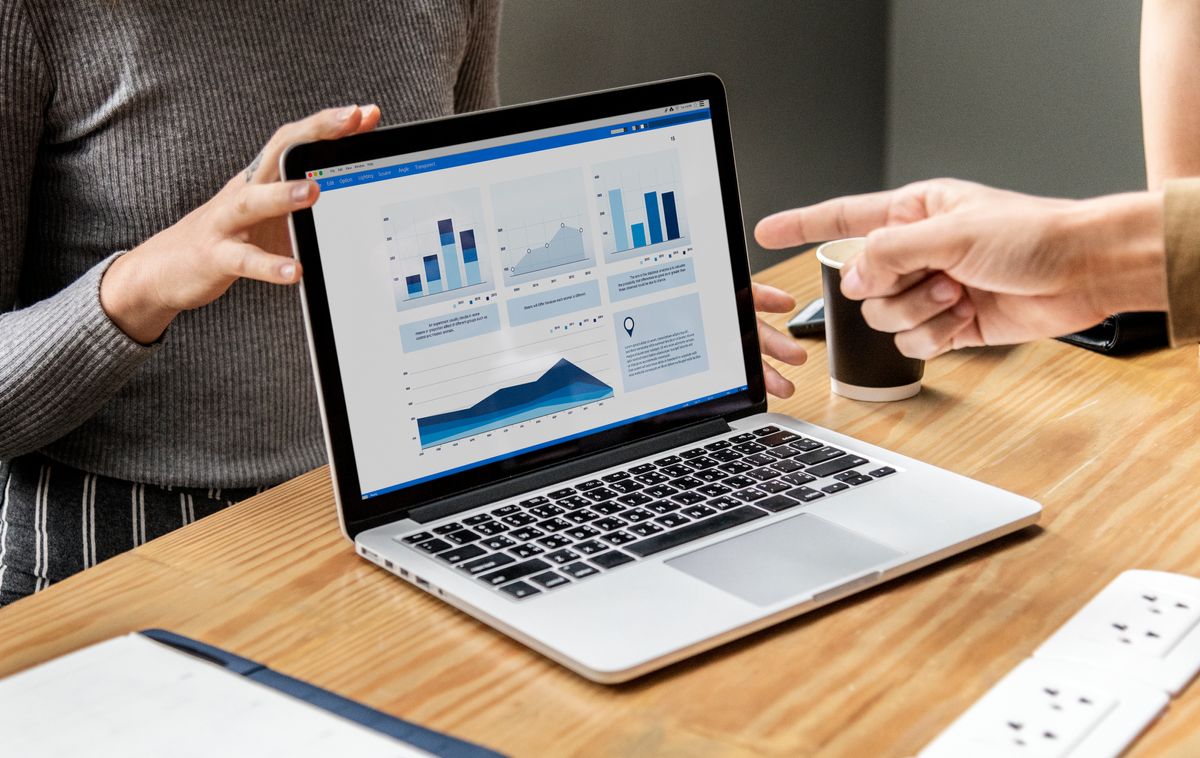
Cultivating a Data-Driven Culture within Organizations
To truly unlock the potential of predictive analytics, organizations must cultivate a data-driven culture. Fostering data-driven culture through data literacy, leadership vision, and organizational commitment leads to informed decision-making and competitive advantage. Resistance to data-driven approaches often stems from a lack of understanding. By promoting data literacy across all levels, employees become more adept at interpreting data and making evidence-based decisions.
Embracing iterative learning and focusing on long-term benefits are key to developing a data-driven culture. This approach encourages organizations to learn from past efforts and successes, rather than dwelling on failures.
Here are some steps to foster a data-driven culture:
- Encourage leadership to set a vision for data utilization.
- Invest in training programs to enhance data literacy.
- Implement policies that support data-driven practices.
- Recognize and reward data-informed achievements.
- Ensure transparent communication of data insights and their implications.
Addressing Data Privacy and Security Concerns
In the realm of predictive analytics, data privacy and security are paramount. Organizations must navigate the complex landscape of regulatory compliance while ensuring the protection of sensitive information. To use predictive analytics effectively, a proactive approach is essential, analyzing past security breaches to prepare for future threats. This strategy not only identifies vulnerabilities but also reinforces the organization's defense mechanisms.
Ensuring data privacy is not just a legal obligation but an ethical business practice. It involves allowing clients to control their information and sharing it only with authorized sources. Encryption and access controls are critical tools in safeguarding data against unauthorized access.
To maintain the integrity of data privacy and security, organizations should consider the following steps:
- Compliance with regulations such as GDPR or CCPA.
- Implementation of secure data practices, including encryption and secure access controls.
- Regular security audits to detect vulnerabilities and ensure continuous protection.
Bridging the Gap Between Data Insights and Business Strategy
To effectively bridge the gap between data insights and business strategy, a collaborative approach is paramount. Data scientists, business analysts, and decision-makers must work in unison to ensure that predictive models are not only statistically sound but also aligned with strategic business objectives. This synergy facilitates the translation of complex data into actionable strategies that can propel a business forward.
Collaboration is the cornerstone of integrating predictive analytics into the strategic planning process. It enables organizations to move beyond the mere collection of data to leveraging these insights for competitive advantage. The following points highlight key aspects of this integration:
- Ensuring that data insights are presented in a business-friendly manner that is easily understood by non-technical stakeholders.
- Aligning predictive models with the company's strategic goals to drive relevant and impactful outcomes.
- Establishing a feedback loop where business strategy informs the refinement of predictive models.
By fostering a culture that values data-driven decision making, organizations can overcome resistance and inertia, leading to a more agile and informed approach to business strategy.
The challenge lies not just in analyzing the data, but in making it accessible and actionable for all levels of decision-making within an organization. This requires a clear understanding of business intelligence and the ability to distill vast amounts of data into concise, strategic insights.
The Impact of Predictive Analytics on Industry Verticals
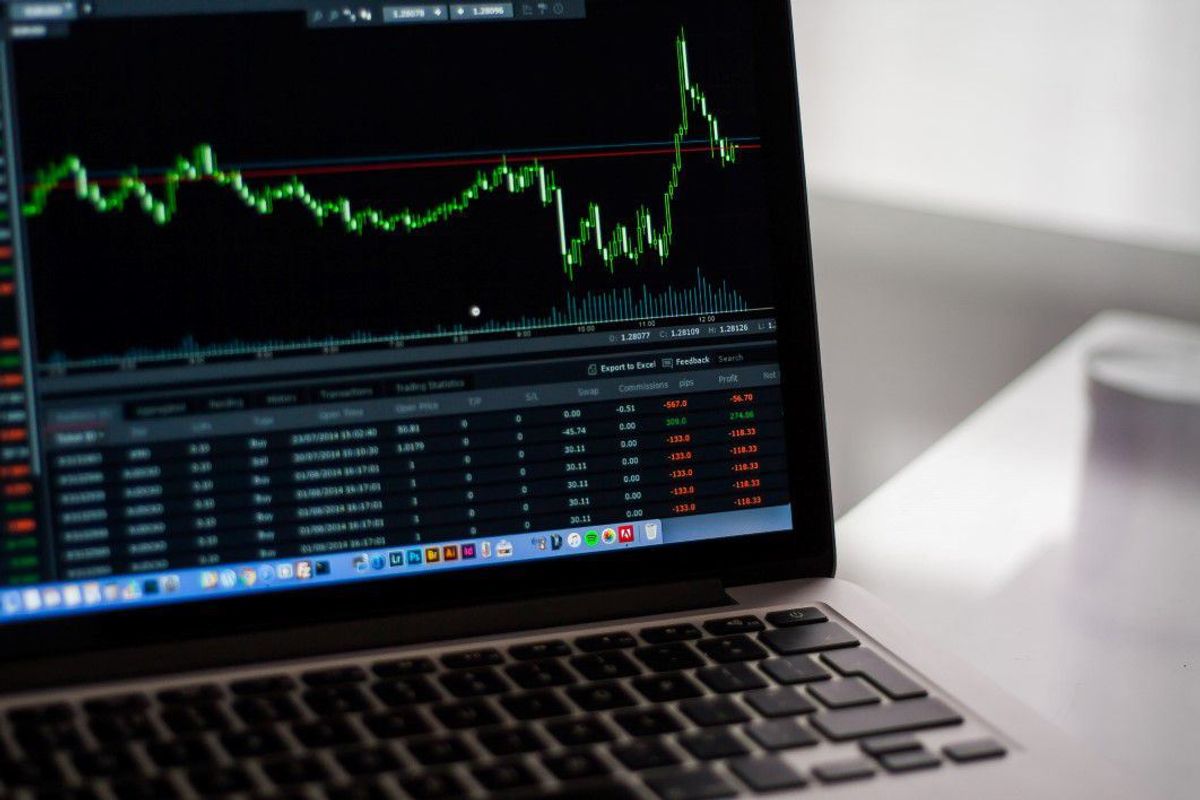
Transforming Healthcare with Predictive Diagnostics
The advent of predictive analytics in healthcare has marked a new era of proactive patient care. Predictive diagnostics are now instrumental in foreseeing health outcomes and tailoring treatment plans. By analyzing patterns within vast datasets, healthcare providers can identify at-risk patients, leading to more timely and effective interventions.
- Reduction in Hospital Readmissions: A case study revealed a 20% decrease in readmissions due to the implementation of AI-driven predictive tools.
- Disease Prediction and Resource Allocation: Predictive models are used to anticipate disease outbreaks and optimize the distribution of medical resources.
- Personalized Healthcare Strategies: Analytics enable the creation of personalized care plans, improving patient outcomes and satisfaction.
Predictive analytics in healthcare not only enhances the quality of care but also contributes to the sustainability of healthcare systems by optimizing resource utilization.
The integration of predictive analytics into healthcare management has demonstrated significant benefits, from disease prediction to patient outcome forecasting. This strategic application of data science supports a shift towards more personalized and efficient healthcare delivery.
Revolutionizing Retail with Personalized Marketing
The retail landscape is undergoing a profound transformation, largely driven by the power of predictive analytics. Predictive analytics empowers businesses to understand customer behavior, align with market demand, and optimize strategies for growth. A notable example is a leading fashion retailer that faced challenges with inventory management and customer satisfaction. By harnessing AI-driven data analysis for demand forecasting and customer sentiment analysis, they achieved a significant reduction in unsold inventory and a boost in customer satisfaction.
Personalized marketing campaigns, powered by predictive analytics, are not just about selling products but about creating value for customers. Retailers can now anticipate needs and offer tailored recommendations, which in turn fosters loyalty and increases sales. Consider the following outcomes from the implementation of predictive analytics in retail:
- 25% reduction in unsold inventory
- 15% increase in customer satisfaction scores
Cloud technology and pattern identification are key components that enhance decision-making and efficiency in retail. These advancements enable retailers to execute dynamic pricing models and targeted marketing campaigns that resonate with consumers. As a result, retailers are not only meeting but exceeding customer expectations, setting new standards for the industry.
Optimizing Supply Chain and Logistics
The integration of predictive analytics into supply chain and logistics has revolutionized the way businesses manage their operations. Implement data-driven strategies with clear objectives to achieve efficiency and cost savings. Advanced analytics and automation play a pivotal role in optimizing operations, from predictive maintenance to supply chain optimization.
For instance, a manufacturing company leveraging AI-driven analysis witnessed a significant reduction in unplanned downtime and an increase in production efficiency. This was achieved by analyzing operational data in real-time to identify bottlenecks and predict maintenance needs.
- Route optimization
- Dynamic pricing models
- Targeted marketing campaigns
These elements are crucial for streamlining supply chain processes. Moreover, companies like PepsiCo are utilizing Bluetooth-enabled sensors to ensure the freshness of food during transportation, thereby reducing waste and enhancing the overall supply chain.
By embracing predictive analytics, organizations can not only anticipate future demands but also tailor their inventory and offerings to meet consumer preferences more accurately, leading to improved customer satisfaction and reduced inventory costs.
Building Effective Predictive Models
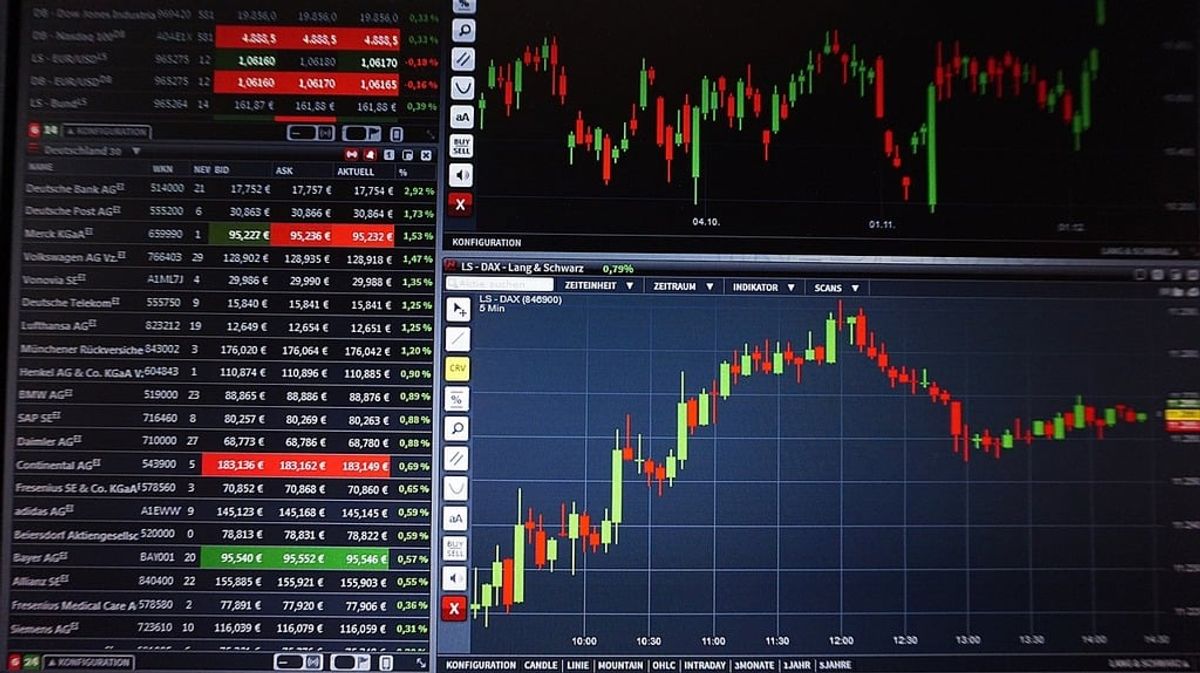
Feature Selection and Data Engineering
In predictive analytics, the art of feature selection and engineering is pivotal for the development of robust models. Feature selection involves discerning which data attributes are most predictive of the outcome you're interested in. This step is essential to enhance model accuracy and prevent overfitting. Feature engineering, on the other hand, is about creating new features from existing ones to uncover hidden patterns and insights.
By applying these techniques, you can enhance the predictive power of your model and uncover hidden patterns in the data.
Here are some common techniques used in feature engineering:
- Normalization: Adjusting values to a common scale without distorting differences in the ranges of values.
- Standardization: Rescaling data so it has a mean of 0 and a standard deviation of 1.
- One-hot encoding: Representing categorical variables as binary vectors.
- Feature scaling: Ensuring that the influence of a feature on the model is proportional to its predictive value.
It's crucial to iterate over these processes, evaluating their impact on the model's performance and refining your approach to achieve the best results.
Algorithm Selection and Model Training
Selecting the right algorithm is a pivotal step in predictive analytics. Common techniques include regression analysis, decision trees, and neural networks. Each model has its strengths and limitations, and the choice should align with the specific objectives of the project. For instance, linear regression is favored for its simplicity and interpretability, while random forests may be chosen for their robustness to outliers and ability to handle non-linear relationships.
The process of model training involves feeding data into the algorithm to uncover underlying patterns. This phase is critical as it determines the model's ability to generalize from the training data to unseen data.
It's essential to balance model complexity with interpretability. A simpler model can be more beneficial when ease of understanding is required. After selecting an algorithm, the model must be trained and tested to ensure it performs well. This involves splitting the dataset into training and testing sets, where the former is used to build the model and the latter to evaluate its predictive power.
- Evaluate model performance using metrics like accuracy, precision, recall, and F1 score.
- Refine the model by tuning hyperparameters to optimize performance.
- Validate the model using cross-validation techniques to prevent overfitting.
By adhering to these steps and continuously refining the model with new data, predictive analytics can yield powerful insights for data-driven decision-making.
Model Deployment and Real-World Application
Once a predictive model has been trained and tested, the next critical step is deployment into a real-world environment. This phase involves integrating the model into existing business processes and systems, ensuring that it can receive new data, perform predictions, and provide actionable insights.
Key considerations for successful deployment include:
- Ensuring compatibility with current IT infrastructure
- Establishing a seamless data pipeline for real-time or batch processing
- Defining clear protocols for model updates and maintenance
Successful model deployment requires meticulous planning and coordination with various stakeholders to ensure that the predictive insights are effectively translated into business value.
After deployment, it's crucial to monitor the model's performance continuously. This involves setting up mechanisms to track the accuracy of predictions and the model's impact on decision-making processes. Regularly reviewing and updating the model with new data helps maintain its relevance and accuracy over time.
In summary, the transition from a theoretical model to a practical tool is a complex but rewarding journey. It demands a collaborative effort across multiple departments and a commitment to ongoing evaluation and refinement. The ultimate goal is to create a sustainable predictive system that adapts to changing conditions and consistently delivers value.
Case Studies: Success Stories in Predictive Analytics
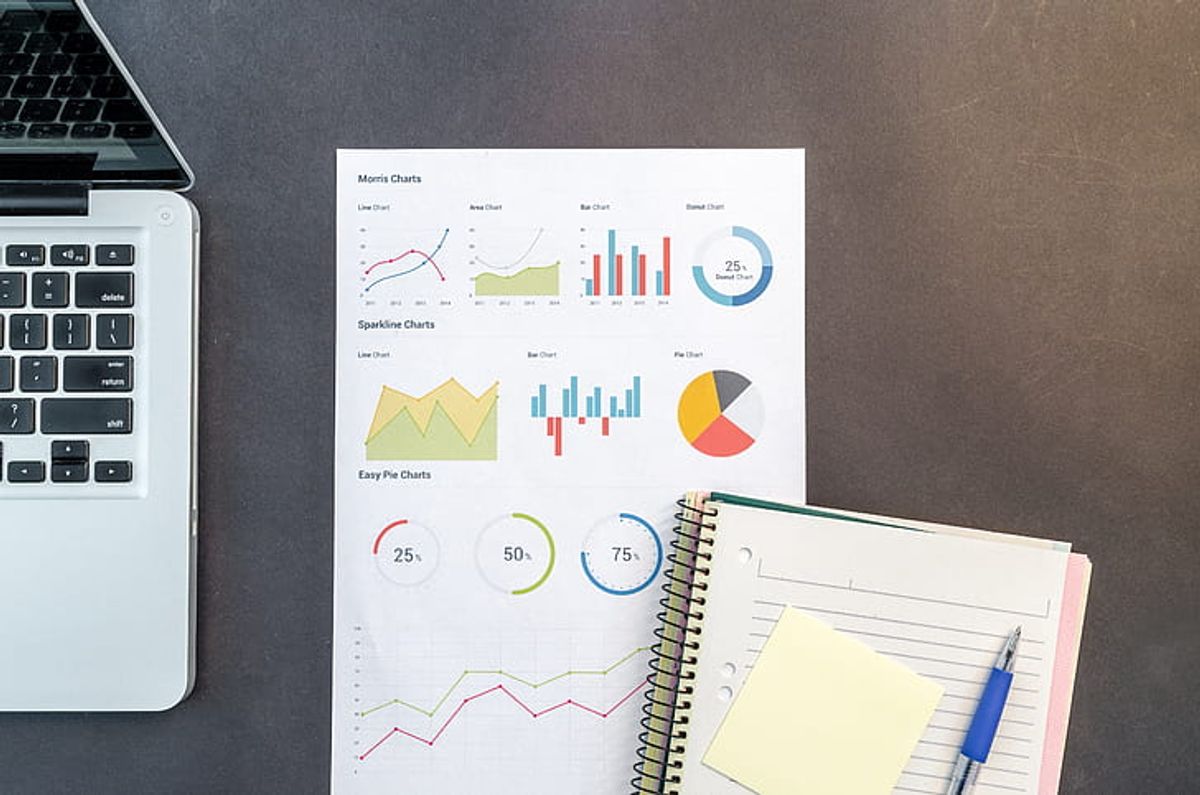
Financial Services: Fraud Detection and Credit Scoring
In the realm of financial services, predictive analytics has become a cornerstone for combating fraud and enhancing credit scoring mechanisms. A global financial institution witnessed a 30% reduction in fraud cases after integrating AI-driven data analysis into its fraud detection processes. The machine learning algorithms, trained on historical transaction data, not only saved millions of dollars annually but also fortified customer trust.
Enhanced Risk Assessment is another area where predictive analytics shines, particularly in finance. By analyzing customer transactions, machine learning models offer a more accurate assessment of loan default risks, leading to smarter lending decisions.
Predictive analytics in finance not only enhances risk management and fraud detection but also ensures regulatory compliance and strategic decision-making.
The integration of predictive analytics into financial services has led to significant advancements in customer relationship management (CRM) as well. Organizations are now able to understand customer behavior, anticipate needs, and personalize interactions, which in turn boosts customer satisfaction, retention, and loyalty.
Manufacturing: Predictive Maintenance and Quality Control
In the realm of manufacturing, the integration of predictive analytics has been a game-changer, particularly in the areas of predictive maintenance and quality control. By leveraging manufacturing analytics, companies have been able to predict machinery's future use and preemptively address potential failures. This foresight not only prevents costly downtime but also enhances the overall efficiency of production lines.
With the application of AI-driven analysis, manufacturers can optimize their processes in real-time. This includes identifying bottlenecks and forecasting maintenance needs, which, in one instance, led to a 40% reduction in unplanned downtime and a 10% increase in production efficiency.
The benefits of predictive analytics in manufacturing are further underscored by the following points:
- Automate Data Processing to minimize manual intervention and errors.
- Identify Patterns and Anomalies in operational data, offering new insights and improving decision-making.
These advancements underscore the critical role that predictive analytics plays in modern manufacturing, enabling businesses to stay ahead of the curve in a competitive landscape.
Telecommunications: Churn Prediction and Customer Segmentation
In the competitive landscape of telecommunications, predictive analytics plays a pivotal role in customer retention and targeted marketing. By analyzing call patterns, usage data, and customer feedback, telecom companies can predict which customers are at risk of churning and why. This insight allows for timely intervention with personalized offers and services to increase loyalty.
Churn prediction models harness historical data to identify the warning signs of customer dissatisfaction. Segmentation, on the other hand, groups customers based on behavior, value, and needs, enabling tailored communication strategies. Together, these predictive techniques transform raw data into actionable intelligence.
The synergy between churn prediction and customer segmentation creates a dynamic framework for sustaining growth and improving customer experience in the telecom sector.
The following table illustrates a simplified view of the outcomes from predictive analytics in a telecom context:
Metric | Before Predictive Analytics | After Predictive Analytics |
---|---|---|
Customer Retention Rate | 75% | 85% |
Average Revenue Per User (ARPU) | $45 | $50 |
Customer Satisfaction Index | 70% | 80% |
By leveraging predictive analytics, telecom companies not only enhance their operational efficiency but also gain a competitive edge through data-driven strategies.
The Ethical Considerations of Predictive Analytics
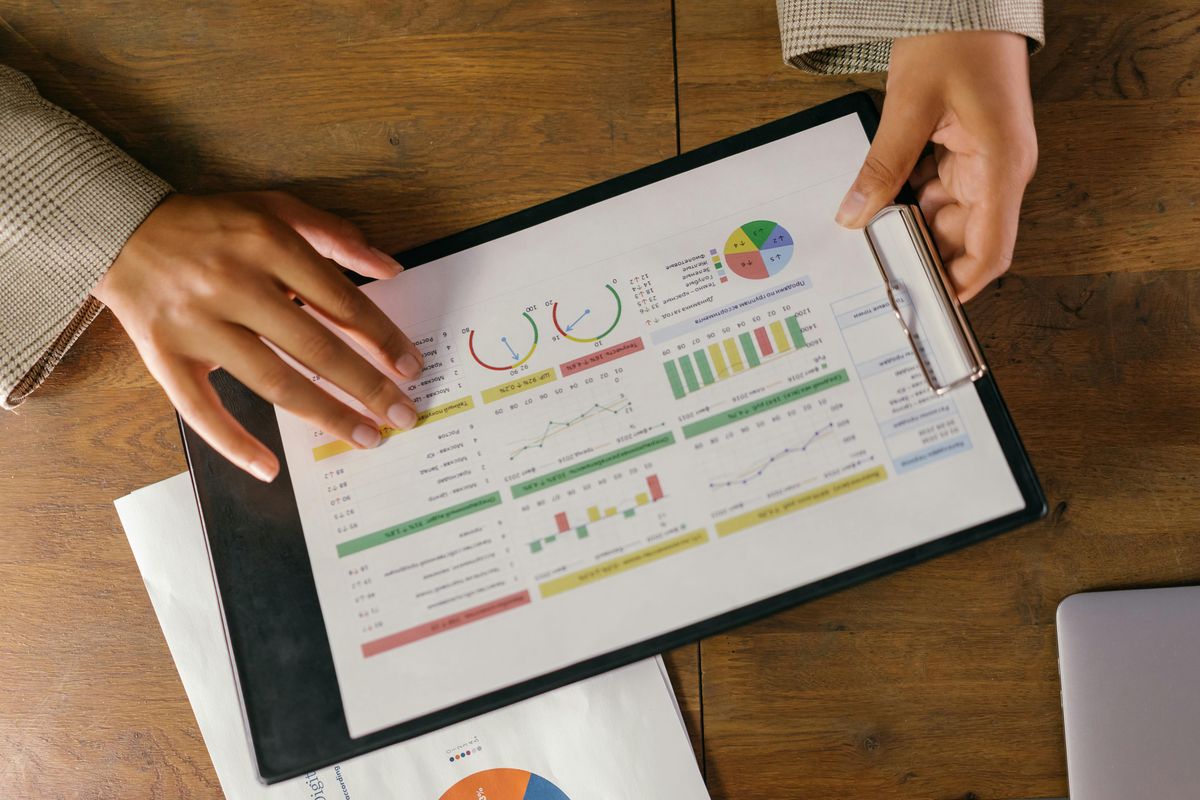
Avoiding Bias in Predictive Modeling
In the realm of predictive analytics, avoiding bias is paramount to ensure the integrity and fairness of the models. Bias can creep into models through various channels, such as skewed data sets, flawed algorithms, or even the unconscious prejudices of the modelers. To combat this, it is essential to adopt a rigorous approach to feature selection and data engineering, as these are critical steps in building a predictive model.
- Feature Selection: Carefully choose features that are relevant and have predictive power, while excluding those that could introduce bias.
- Data Engineering: Transform and preprocess data to reflect true patterns and relationships, without amplifying existing biases.
Predictive modeling offers strategic guidance by transforming data into actionable insights, reducing bias in decision-making through advanced analytics, and promoting equitable strategies in uncertain business environments.
It is also crucial to select the right models and algorithms that are appropriate for the data and the problem at hand. Techniques such as cross-validation and regularisation can help in detecting and mitigating bias. Continuous model evaluation and refinement are necessary to maintain the accuracy and fairness of predictive analytics over time.
Ensuring Transparency and Explainability
In the realm of predictive analytics, transparency is paramount. It is essential for users to comprehend the journey from input data to the final decision. This understanding fosters trust and facilitates the responsible use of AI. Explainability, on the other hand, delves into the intricacies of the decision-making process, ensuring that the outcomes are not just a black box but can be interpreted and justified.
Explainability and interpretability are at the heart of transparent artificial intelligence. They enable stakeholders to grasp how data are processed, which is crucial for accountability and ethical AI practices. To achieve this, organizations may adopt the following measures:
- Compliance with regulations to align with data protection laws like GDPR or CCPA.
- Implementation of secure data practices, including encryption and secure access controls.
- Regular security audits to identify vulnerabilities and ensure the effectiveness of privacy measures.
By prioritizing explainability, businesses not only adhere to ethical standards but also empower users to make informed decisions based on the insights provided by predictive models.
Regulatory Compliance and Ethical Use of Data
In the realm of predictive analytics, ensuring regulatory compliance and ethical use of data is paramount. Companies must navigate a complex landscape of data protection laws, such as the General Data Protection Regulation (GDPR) and the California Consumer Privacy Act (CCPA), to maintain trust and avoid legal repercussions.
Compliance with regulations is not just about avoiding fines; it's about respecting the privacy and rights of individuals. Secure data practices, including encryption and access controls, are essential to safeguard sensitive information from breaches. Regular security audits help identify vulnerabilities and reinforce data protection efforts.
Organizations should empower clients by allowing them control over their personal information, sharing it only with authorized entities.
The following list outlines key steps for maintaining ethical standards in data use:
- Ensure AI tools comply with all relevant data protection regulations.
- Implement secure data practices to protect sensitive information.
- Conduct regular security audits to maintain high standards of data privacy.
Future Directions in Predictive Analytics
The Role of Quantum Computing in Analytics
Quantum computing is poised to revolutionize the field of predictive analytics by offering unparalleled processing power and speed. Quantum computers are capable of analyzing and interpreting massive datasets far more efficiently than traditional computers. This quantum advantage stems from their ability to perform complex calculations at a fraction of the time, making them particularly suited for handling the voluminous and intricate data involved in predictive analytics.
The integration of quantum computing in data analytics can lead to high-speed detection and analysis of large scattered datasets. For businesses, this means the ability to integrate and diagnose data with unprecedented speed, offering a significant edge in a competitive market.
The synergy between quantum computing and predictive analytics could unlock new dimensions in data-driven decision making, enabling deeper insights and more accurate forecasts.
Quantum computing is not without its challenges, however, as it requires specialized knowledge and infrastructure. But as the technology matures, it is expected to become more accessible, leading to wider adoption across various industries.
Predictive Analytics in the Era of IoT
The Internet of Things (IoT) has ushered in a new frontier for predictive analytics, where interconnected devices provide a continuous stream of data. This data, when analyzed, can lead to unprecedented insights and proactive decision-making. The synergy between IoT and predictive analytics is transforming how businesses operate, maintain equipment, and interact with customers.
In the context of IoT, predictive analytics can be utilized to monitor the health of devices, predict failures, and schedule maintenance. This not only enhances operational efficiency but also extends the lifespan of equipment. For example, in the manufacturing sector, sensors on machinery can predict when parts are likely to fail and schedule repairs before breakdowns occur, minimizing downtime.
The integration of predictive analytics with IoT devices is not just about preventing failures; it's about optimizing the entire ecosystem for peak performance and cost savings.
Key Applications of Predictive Analytics in IoT:
- Real-time asset tracking and management
- Energy consumption optimization
- Predictive maintenance and failure prevention
- Enhanced customer experience through personalized services
By leveraging machine learning algorithms, predictive models can be trained on IoT-generated data to identify patterns that humans might miss. This leads to smarter, more efficient systems that can adapt over time. As IoT continues to expand, the potential for predictive analytics to provide deep, actionable insights will only grow, making it an essential tool for any data-driven organization.
The Convergence of Predictive Analytics and Blockchain Technology
The integration of blockchain technology with predictive analytics represents a groundbreaking shift in how data is secured and utilized for forecasting. Blockchain's inherent security and transparency features complement the predictive capabilities by providing a tamper-proof ledger for data storage and transactions. This synergy enhances the trustworthiness of predictive models, especially in sectors where data integrity is paramount.
- Ensuring data immutability and traceability
- Facilitating secure sharing of predictive insights
- Enhancing the verification of data sources
The convergence of these technologies not only fortifies the security of predictive analytics but also opens up new avenues for innovation in data-driven strategies.
Collaboration between data scientists and blockchain experts is crucial to harness the full potential of this convergence. As organizations strive for enhanced security solutions and proactive decision-making, the blend of predictive analytics and blockchain stands as a beacon of progress in the realm of data science.
As we look towards the horizon of predictive analytics, the potential for innovation and efficiency is boundless. The future promises even faster data solutions, and at OptimizDBA, we're at the forefront of this evolution. Our proprietary methodologies and unparalleled experience have consistently delivered performance increases that redefine industry standards. Don't let your data strategies fall behind; visit our website to discover how we can accelerate your database performance and transform your business's predictive analytics capabilities. It's time to experience the OptimizDBA difference and propel your organization into the future.
Conclusion
As we have explored throughout this article, predictive analytics stands as a cornerstone in the edifice of modern data-driven decision-making. By integrating advanced statistical algorithms, machine learning techniques, and a collaborative approach among data scientists, business analysts, and decision-makers, organizations can not only forecast future trends but also identify risks and optimize processes across various industries. The key to harnessing the full potential of predictive analytics lies in the implementation of best practices, continuous model refinement, and fostering a culture that embraces data-driven insights. As businesses continue to navigate an ever-evolving landscape, the ability to anticipate and proactively respond to challenges through predictive analytics will be a defining factor in achieving sustained success and competitive advantage.
Frequently Asked Questions
What is predictive analytics and how is it used in business?
Predictive analytics is a field within data science that uses statistical algorithms and machine learning techniques to forecast future trends, behaviors, and outcomes, allowing businesses to make informed decisions, mitigate risks, and capitalize on opportunities.
How does predictive analytics impact learning and development?
Predictive analytics can identify high-potential employees, predict training needs, and optimize learning interventions, thereby enhancing the effectiveness of L&D programs and driving organizational success.
What are some best practices for implementing predictive analytics?
Best practices include collaborating across disciplines for model development, ensuring data quality and governance, and continuously evaluating and refining models as new data becomes available.
What technological advancements are shaping predictive analytics?
Advancements such as machine learning, AI, big data, real-time analytics, and emerging technologies like IoT and blockchain are revolutionizing the predictive analytics landscape.
What challenges do organizations face with data-driven decision making?
Challenges include cultivating a data-driven culture, addressing data privacy and security concerns, and bridging the gap between data insights and business strategy.
How does predictive analytics transform industry verticals like healthcare and retail?
In healthcare, predictive analytics enables predictive diagnostics, while in retail, it allows for personalized marketing, significantly enhancing efficiency and customer experience.
What ethical considerations must be taken into account with predictive analytics?
Ethical considerations include avoiding bias in predictive modeling, ensuring transparency and explainability of models, and adhering to regulatory compliance and ethical data use.
What future directions is predictive analytics heading towards?
Predictive analytics is moving towards integration with quantum computing, the Internet of Things (IoT), and blockchain technology, promising even more sophisticated and powerful analytics capabilities.