In today's world, data is everywhere. Businesses use data to make smart choices and stay ahead. This guide will help you understand how to use Business Intelligence (BI) and analytics to turn data into useful information. Whether you're new to these concepts or looking to improve your skills, this guide has something for everyone.
Key Takeaways
- Business Intelligence helps companies make better decisions by turning data into insights.
- Big Data technologies allow businesses to handle large amounts of information effectively.
- Analytics helps in understanding past data and predicting future trends.
- Data visualization tools make it easier to understand complex data.
- A data-driven culture in a company leads to smarter and faster decision-making.
Understanding Business Intelligence
Business Intelligence (BI) is all about using data to make better decisions. It includes tools and techniques to turn raw data into useful information. BI helps companies understand their past performance and plan for the future.
Exploring Big Data
Big Data Technologies
Big data technologies have changed how businesses handle huge amounts of information. These tools help companies process and analyze data very quickly. Technologies like Hadoop and Spark allow for distributed computing, making it easier to manage large datasets. This is important for getting timely insights and staying competitive in today's fast-paced market.
Some of the key technologies used to handle Big Data include:
- Hadoop: An open-source framework for distributed storage and processing of large datasets across clusters of computers.
- Apache Spark: A fast and general-purpose cluster computing system for big data processing.
- NoSQL Databases: Non-relational databases designed to handle large amounts of unstructured and semi-structured data.
- Data Lakes: Centralized repositories that allow organizations to store and analyze large amounts of structured and unstructured data.
- Stream Processing: Technologies that enable real-time data processing and analytics.
Applications of Big Data
Big Data has many uses across different industries. In healthcare, it helps in predicting disease outbreaks and improving patient care. Retailers use big data to understand customer behavior and optimize inventory. In finance, it aids in fraud detection and risk management. Big Data's ability to provide deep insights makes it a valuable asset for any industry.
Challenges in Managing Big Data
Handling Big Data comes with its own set of challenges. One major issue is data quality. Ensuring that the data is accurate and reliable is crucial. Another challenge is data security. Protecting sensitive information from breaches is a top priority. Additionally, the sheer volume of data can be overwhelming, requiring specialized tools and technologies to manage effectively.
Managing Big Data is not just about having the right tools, but also about having the right strategies in place to ensure data quality and security.
Defining Analytics
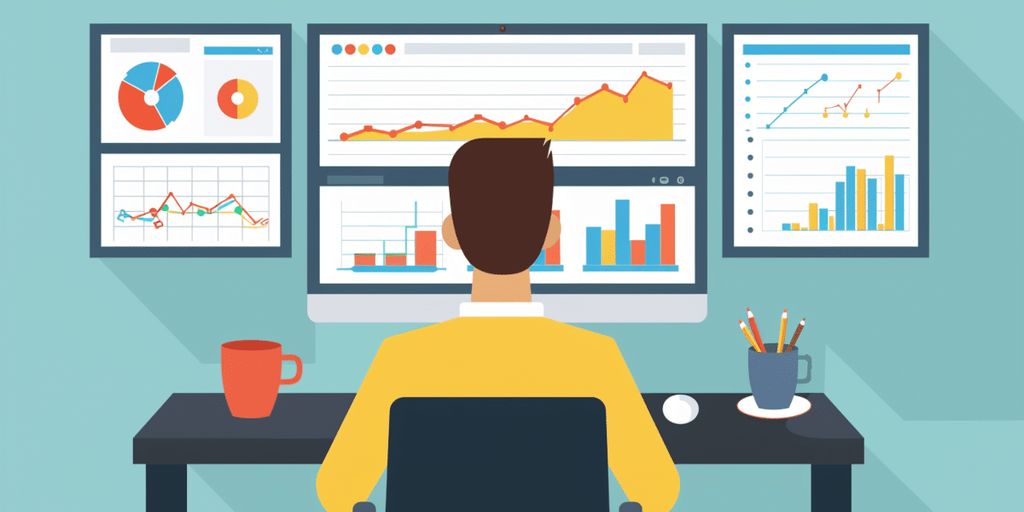
Analytics is the practice of using data, statistical methods, and quantitative techniques to gain insights and make smart choices. It can be used in many business areas like marketing, finance, operations, and human resources.
Types of Analytics
There are different kinds of analytics:
- Descriptive Analytics: This type looks at what happened in the past by studying old data. For example, checking sales data to find trends.
- Diagnostic Analytics: This type tries to understand why something happened by digging deeper into the data. For instance, looking at customer churn data to find out why customers leave.
- Predictive Analytics: This type forecasts what might happen in the future using historical data and models. For example, predicting future sales based on past trends.
- Prescriptive Analytics: This type suggests actions to take for the best outcomes. For instance, recommending marketing strategies based on data insights.
Tools for Data Analytics
Various tools help in data analytics, including:
- Spreadsheets: Simple and widely used for basic data analysis.
- Business Intelligence (BI) Tools: These tools help in visualizing data and making reports.
- Statistical Software: Programs like R and Python are used for advanced data analysis.
- Data Visualization Tools: Tools like Tableau and Power BI help in creating visual representations of data.
Common Pitfalls in Data Analytics
While data analytics is powerful, there are common mistakes to avoid:
- Ignoring Data Quality: Poor data quality can lead to wrong insights.
- Overlooking Context: Data should be analyzed within the right context to make sense.
- Misinterpreting Correlation and Causation: Just because two things happen together doesn't mean one caused the other.
- Lack of Clear Goals: Without clear goals, data analysis can become directionless and unproductive.
Analytics has become a key part of modern business, helping companies make data-driven decisions for a competitive edge.
The Relationship Between BI, Big Data, and Analytics
Integrating BI and Big Data
Integrating Business Intelligence (BI) with Big Data allows organizations to unlock the power of data: a comprehensive guide to business intelligence and analytics. BI tools help collect, integrate, and analyze large datasets from various sources. This integration enables businesses to gain deeper insights and make smarter decisions.
The Role of Analytics in BI
Analytics plays a crucial role in BI by applying statistical and quantitative methods to the data collected. This process helps uncover hidden patterns and trends, leading to informed decision-making. Analytics involves using data, statistical methods, and quantitative techniques to gain insights and make informed decisions.
Case Studies of Successful Integration
Several organizations have successfully integrated BI and Big Data to achieve remarkable results. For instance, a retail company used BI tools to analyze customer data, leading to personalized marketing strategies and increased sales. Another example is a healthcare provider that utilized Big Data and BI to improve patient care and operational efficiency.
By combining BI, Big Data, and Analytics, businesses can stay competitive and make data-driven decisions that drive growth and success.
Data-Driven Decision Making
Importance of Data-Driven Decisions
In today's world, making choices based on data is crucial. Unlocking the power of data helps businesses make smarter decisions. When companies use data, they can see patterns and trends that guide them in the right direction.
Steps to Implement Data-Driven Decision Making
- Collect Data: Gather data from various sources like sales, customer feedback, and market trends.
- Analyze Data: Use tools to find patterns and insights.
- Make Decisions: Use the insights to make informed choices.
- Measure Success: Track the results to see if the decisions were effective.
Real-World Examples
Many companies have successfully integrated data into their decision-making process. For instance, a retail company might use BI tools to analyze sales data from their point-of-sale systems, combine this with customer data from their CRM system, and then use predictive analytics to forecast future sales and optimize their inventory management.
A data-driven culture empowers employees to make informed decisions, leading to better outcomes for the business.
Data Visualization Techniques
Data visualization tools turn complex data into easy-to-understand visuals. This helps stakeholders quickly grasp insights and trends. Effective data visualization can lead to better decision-making. Tools like D3.js, Plotly, and Chart.js are popular for creating interactive and engaging visualizations.
Predictive Analytics in Business
Predictive analytics uses statistical models and machine learning techniques to forecast future outcomes based on historical data. Businesses use this type of analytics to anticipate trends, customer behaviors, and potential risks. Predictive models can help companies stay ahead of the competition by making proactive decisions.
Data Governance and Security
Importance of Data Governance
Data governance is essential for managing and using data effectively. It involves setting up rules and processes to ensure data is accurate, consistent, and used properly. Good data governance helps in building trust in the data and the insights derived from it. It also ensures that data is handled ethically and legally, which is crucial for any business.
Data Security Best Practices
Data security is about protecting data from unauthorized access and breaches. Businesses need to implement strong security measures to keep data safe. This includes using encryption, setting up firewalls, and regularly updating security protocols. It's also important to train employees on data security practices to prevent accidental breaches.
Regulatory Compliance
Organizations must follow various laws and regulations related to data, such as GDPR and HIPAA. Compliance ensures that data is handled legally and ethically, helping businesses avoid fines and legal issues. Companies should regularly review and update their policies to stay compliant with these regulations.
Good data governance and security practices not only protect sensitive information but also foster a culture of responsible data use within the organization.
Future Trends in Business Intelligence and Analytics
Artificial Intelligence (AI) and machine learning are becoming key parts of business intelligence. These technologies help in making more accurate predictions and speeding up data processing. AI integration allows businesses to automate routine tasks, making data analysis faster and more reliable.
Self-service analytics is on the rise, allowing users to generate their own reports without needing deep technical skills. This trend empowers employees at all levels to make data-driven decisions, enhancing overall business efficiency.
The future holds both challenges and opportunities for business intelligence and analytics. Companies will need to focus on data governance and security to protect sensitive information. At the same time, advancements in technology will offer new ways to leverage data for business success.
The future of BI and analytics includes AI integration, self-service analytics, and big data impact, crucial for business success.
Building a Data-Driven Culture
Encouraging Data Literacy
Creating a data-driven culture is key to unlocking the power of data. Encourage your team to use data in their daily decision-making. Provide training and resources to help them understand the importance of data. This will make your BI strategy more effective and sustainable.
A data-driven culture empowers employees to make informed decisions, leading to better outcomes for the business.
Overcoming Resistance to Change
Change can be hard, but it's necessary for growth. Address concerns and show the benefits of using data. Involve everyone in the process and celebrate small wins to build momentum.
Measuring the Impact of a Data-Driven Culture
Track progress to see how data-driven practices are improving your business. Use metrics like decision-making speed, accuracy, and business outcomes. Regularly review these metrics to ensure continuous improvement.
Case Studies of BI and Analytics Success
Retail Industry
In the retail sector, companies have been unlocking the power of data to enhance customer experiences and streamline operations. By integrating BI with business strategy, retailers can track inventory in real-time, predict trends, and personalize marketing efforts. This leads to increased sales and customer satisfaction.
Measuring the impact of BI is essential for continuous improvement and ensuring that the organization is on the right path to achieving its goals.
Healthcare Sector
Healthcare organizations use BI to improve patient care and operational efficiency. By analyzing patient data, hospitals can predict patient admissions, manage resources better, and improve treatment outcomes. This not only enhances patient satisfaction but also reduces costs.
Financial Services
In the financial sector, BI helps in risk management and fraud detection. By analyzing transaction data, financial institutions can identify suspicious activities and take preventive measures. Additionally, BI tools assist in customer segmentation, enabling personalized financial services and improving customer loyalty.
Conclusion
In today's world, data is like a treasure chest waiting to be unlocked. Business Intelligence and Analytics are the keys that open it. By using these tools, companies can turn raw numbers into clear insights. This guide has shown how important it is to use data wisely. With the right tools and approach, any business can make smart decisions that lead to success. So, start your data journey now and see the amazing things you can achieve!
Frequently Asked Questions
What is Business Intelligence (BI)?
Business Intelligence, or BI, includes tools and techniques for turning raw data into useful information for making better decisions.
How does Big Data differ from regular data?
Big Data refers to extremely large datasets that require special tools to process and analyze, unlike regular data which can be handled by standard software.
What are the benefits of using BI in a business?
BI helps businesses make smarter decisions, improve efficiency, and gain insights into customer behavior and market trends.
What is data analytics?
Data analytics is the process of examining data sets to draw conclusions and identify patterns, trends, and insights.
How are BI and analytics related?
BI focuses on using data to make informed decisions, while analytics involves examining data to find patterns and insights. Together, they help businesses optimize their operations.
What challenges might a business face when adopting BI?
Challenges include high costs, data quality issues, and the need for skilled personnel to manage and analyze the data.
Why is data visualization important?
Data visualization helps people understand complex data by presenting it in a visual format, making it easier to identify trends and patterns.
What is predictive analytics?
Predictive analytics uses historical data to make predictions about future events, helping businesses anticipate trends and make proactive decisions.