AI-Driven Optimization of Enterprise Operations
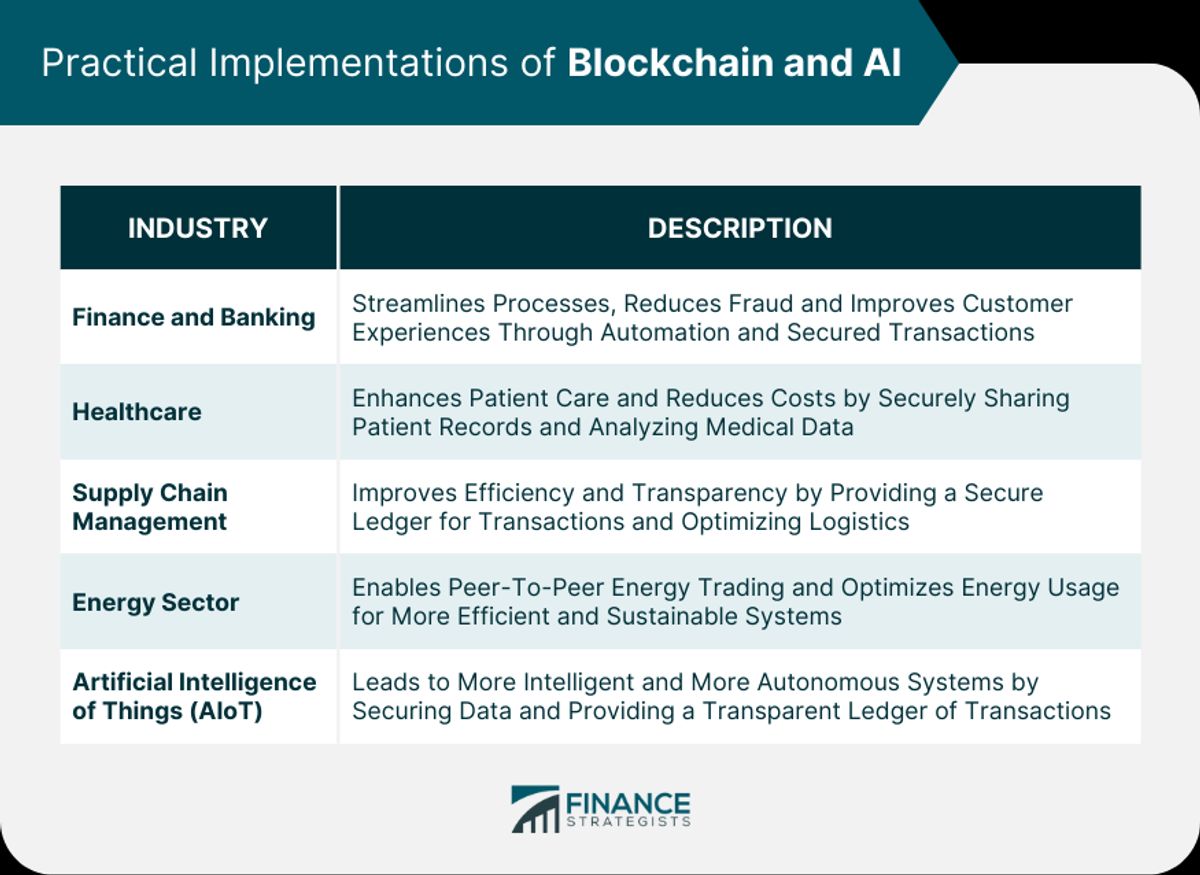
Manufacturing: Optimizing Production and Predictive Maintenance
In the realm of manufacturing, the integration of AI technology with IoT devices has revolutionized the way production lines operate. By leveraging AI for predictive maintenance, manufacturers can anticipate equipment failures, ensuring that maintenance schedules are optimized to minimize downtime. This not only enhances the efficiency of the production process but also leads to significant cost savings.
- AI and IoT for predictive maintenance
- Production optimization based on demand forecasts
- Reduced downtime and maintenance costs
Smart manufacturing also encompasses tasks such as defect detection and process optimization. However, the introduction of new product lines or changes in machinery can cause domain shifts, presenting challenges that require adaptive AI solutions. For example, different versions of the same product or changes in the operation pipeline can lead to these shifts, necessitating a flexible AI approach.
By proactively addressing common data change problems, enterprises can maintain a competitive edge in the fast-paced manufacturing sector. The goal is to ensure that AI-driven systems are not only reactive but also predictive, capable of handling variability and adapting to new conditions seamlessly.
Transportation and Logistics: Route Optimization and Fleet Management
In the realm of transportation and logistics, the integration of AI is revolutionizing how companies approach route optimization and fleet management. AI-powered logistics software is capable of processing vast amounts of data to determine the most efficient routes, taking into account variables such as traffic conditions, weather, and delivery schedules. This not only ensures faster deliveries but also contributes to significant cost savings and enhanced customer satisfaction.
Key Benefits of AI in Route Optimization and Fleet Management:
- Real-time route adjustments for optimal efficiency
- Reduced fuel consumption through intelligent planning
- Improved fleet utilization and reduced idle time
- Enhanced customer satisfaction with timely deliveries
By leveraging AI for route optimization, companies can expect a marked improvement in operational efficiency. The ability to adapt to real-time conditions and predict potential disruptions allows for a more agile and responsive logistics strategy.
The following table illustrates the potential cost savings associated with AI-driven route optimization:
Factor | Before AI | After AI |
---|---|---|
Fuel Consumption | High | Reduced |
Delivery Time | Longer | Shorter |
Fleet Utilization | Lower | Higher |
In conclusion, the strategic application of AI in transportation and logistics not only streamlines operations but also serves as a cornerstone for future growth and competitiveness in an increasingly dynamic market.
Strategies for Effective AI Integration
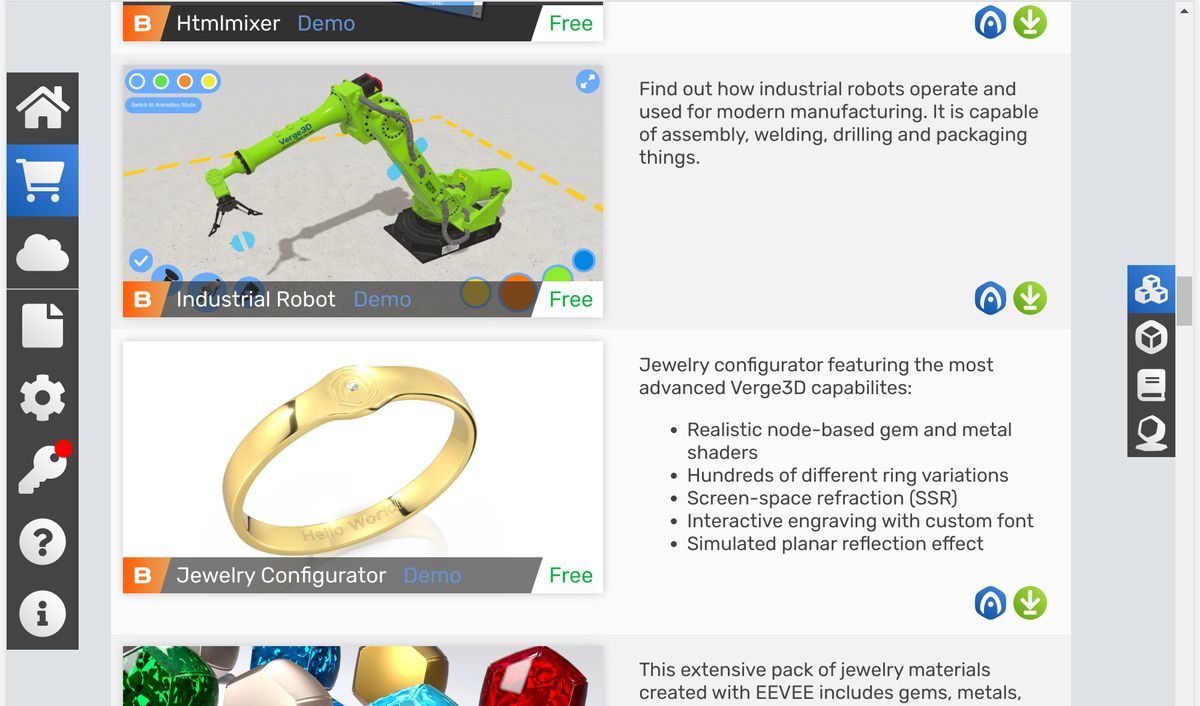
Aligning AI with Business Goals
To fully harness the potential of artificial intelligence, enterprises must ensure that AI initiatives are in lockstep with their core business objectives. Aligning AI with business goals is crucial for driving meaningful outcomes and ensuring that technology investments translate into real business value.
Strategic alignment involves setting clear, measurable objectives for AI projects. This ensures that AI solutions are not just technologically advanced but also directly contribute to the company's bottom line. For instance, an AI project aimed at improving customer service should have specific targets related to customer satisfaction scores or response times.
- Define specific AI goals that support business objectives
- Start with small-scale AI projects to demonstrate value
- Expand AI initiatives incrementally to align with business growth
A balanced approach to AI integration prioritizes strategic planning and incremental implementation, helping to mitigate investment risks and align with long-term business strategies.
Balancing Investment and Risk
Integrating AI into enterprise operations is a balancing act between the potential for transformative benefits and the need to manage associated risks. Businesses must navigate the complexities of significant investment requirements, data privacy concerns, and the necessity for skilled personnel to manage and interpret AI systems. A strategic, incremental approach is essential for mitigating these challenges and maximizing the value of AI investments.
Important considerations include:
- Understanding the risks to be managed
- Establishing governance policies for AI-augmented processes
- Becoming comfortable with AI modeling
A balanced approach emphasizes strategic planning and incremental implementation.
Collaboration with AI startups, research institutions, and technology partners is crucial. It fuels innovation and drives growth by tapping into external AI expertise. This synergy can lead to a more effective balance of investment and risk, ensuring that enterprises are not only adopting AI but are doing so in a way that aligns with their broader business objectives.
Addressing Data Privacy and Skill Gaps
In the era of data-driven decision-making, ensuring data privacy is paramount. Enterprises must navigate the delicate balance between leveraging data for AI advancements and maintaining the confidentiality of sensitive information. Techniques like Differential Privacy (DP) and Federated Learning (FL) offer promising solutions to protect individual privacy while still allowing for the collective benefit of shared insights.
To effectively address skill gaps, businesses must prioritize the development of AI literacy across their workforce. This involves not only hiring talent with the necessary technical skills but also investing in ongoing education and training programs. A multi-faceted approach is essential:
- Recognize the importance of quality data and its role in AI system effectiveness.
- Implement strict data protection regulations and robust security measures.
- Ensure human oversight remains a critical component in AI systems.
The journey towards AI integration in enterprise operations is continuous, with privacy and skill considerations evolving alongside technological advancements. It is crucial to foster an environment where learning and adaptation are constant, ensuring that both employees and AI systems are equipped to handle the challenges of tomorrow.
Scaling Growth Across Industries with AI
Enhanced Predictive Analytics for Decision-Making
The advent of AI-driven predictive analytics has been a game-changer for enterprises, enabling them to anticipate future trends and make informed decisions. By analyzing vast amounts of data, AI helps in identifying patterns and predicting outcomes with remarkable accuracy. This not only streamlines operational tasks but also equips businesses with the foresight to mitigate risks and tailor strategies to evolving market conditions.
Insight generation is a critical component of this process, as AI tools delve into large datasets to extract actionable insights. These insights are pivotal in shaping strategic decisions, from market trend analysis to risk assessment. For instance, in the realm of healthcare, machine learning algorithms can predict patient readmission risks, allowing for preemptive care plans that improve patient outcomes while reducing costs.
The integration of AI models into decision-making processes fundamentally transforms how enterprises respond to dynamic data landscapes.
Here's a snapshot of the benefits that enhanced predictive analytics bring to various aspects of enterprise operations:
- Operational Efficiency: Automation tools powered by AI reduce manual labor and minimize errors.
- Data Analysis: Businesses gain a deeper understanding of customer behavior, market trends, and performance metrics.
- Customization: Personalizing user experiences becomes more nuanced with AI's predictive capabilities.
- CI/CD Processes: AI's role in automating code integration and deployment significantly cuts down on errors.
Cost Savings and Resource Optimization
The integration of AI into enterprise software delivery is not just about innovation; it's about achieving tangible cost savings and resource optimization. By enhancing predictive analytics, AI enables smarter decision-making, which in turn leads to more efficient use of resources and significant cost reductions. For instance, AI-powered logistics software can optimize delivery routes in real-time, factoring in variables such as traffic and weather conditions. This not only speeds up deliveries but also minimizes fuel consumption and maximizes fleet utilization.
AI Technology | Example | Cost Savings |
---|---|---|
AI-powered logistics | Real-time route optimization | Fuel savings, improved fleet efficiency |
AI and IoT in manufacturing | Predictive maintenance, optimized production | Lower maintenance costs, reduced downtime |
By preemptively addressing potential issues and streamlining operations, businesses can avoid costly rework and downtime, ensuring a leaner and more agile operation.
The strategic allocation of AI resources can lead to a sustainable competitive advantage, with improved quality and efficiency directly impacting the bottom line. As AI continues to evolve, its role in driving cost-effective solutions across various industries becomes increasingly critical.
AI in Marketing and Sales Automation
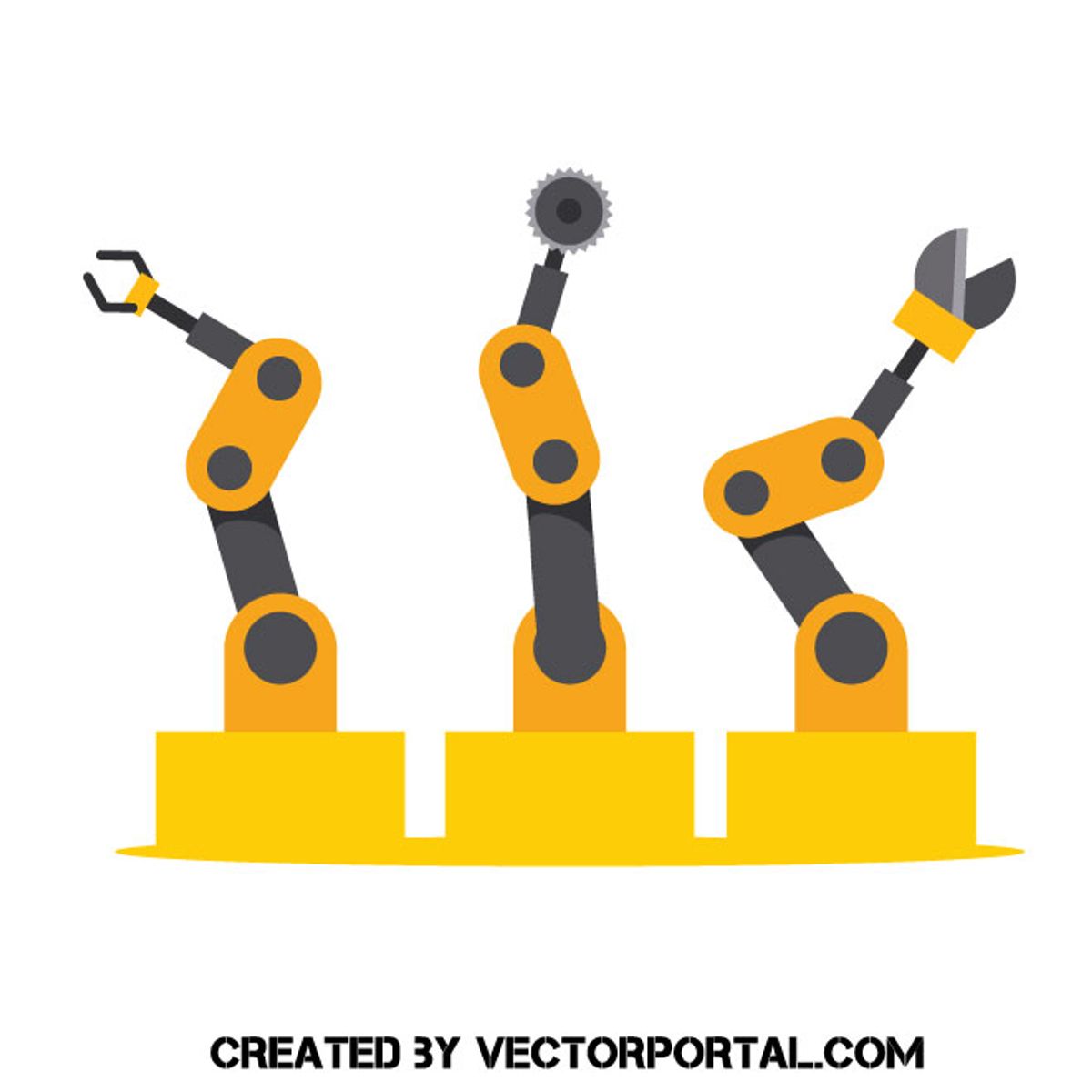
Targeted Marketing Campaigns
In the realm of marketing, AI has become a game-changer, particularly for targeted campaigns. AI-driven analytics enable a deep understanding of customer behavior and preferences, leading to highly personalized marketing strategies. These strategies are not only more engaging for the customer but also result in significantly higher conversion rates.
By leveraging AI, businesses can craft campaigns that resonate on an individual level, fostering a sense of connection and relevance.
The use of AI in advertising, as highlighted by the Marketing AI Institute, involves sophisticated tools that manage targeting and analyze ad campaigns for optimal performance. Here's how AI is revolutionizing marketing:
- Insight generation: AI tools sift through large datasets to uncover hidden patterns and insights.
- Market trend analysis: Predictive models monitor and anticipate market shifts, keeping businesses ahead.
- Customer Experience Enhancement: Technologies like chatbots and personalized recommendations create tailored experiences.
- Innovation and Market Adaptation: AI fosters a culture of innovation, enabling swift adaptation to market changes.
Sales Optimization
In the realm of enterprise software delivery, AI integration is pivotal for enhancing sales processes. By prioritizing sales leads and tailoring sales approaches, AI tools empower businesses to maximize their sales efficiency and effectiveness. This strategic application of AI not only drives revenue growth but also fosters market expansion and nurtures long-term client relationships.
AI Technology plays a crucial role in personalizing customer experiences, a factor that significantly boosts sales. For instance, AI-driven recommendation engines can analyze customer behavior to deliver personalized product suggestions, leading to increased conversion rates. Moreover, AI's capability to optimize inventory levels results in cost savings by reducing overstock and understock situations.
Embracing AI in sales optimization leads to a more competitive edge by enhancing customer experience through personalization, market segmentation, and automation.
Here's a glimpse of the impact of AI on sales optimization:
- Personalization: Tailoring customer interactions based on behavior analysis.
- Lead Prioritization: Using AI to identify and focus on high-value prospects.
- Inventory Management: Streamlining inventory with predictive analytics to match supply with demand.
Future-proofing Your Business with AI Innovation
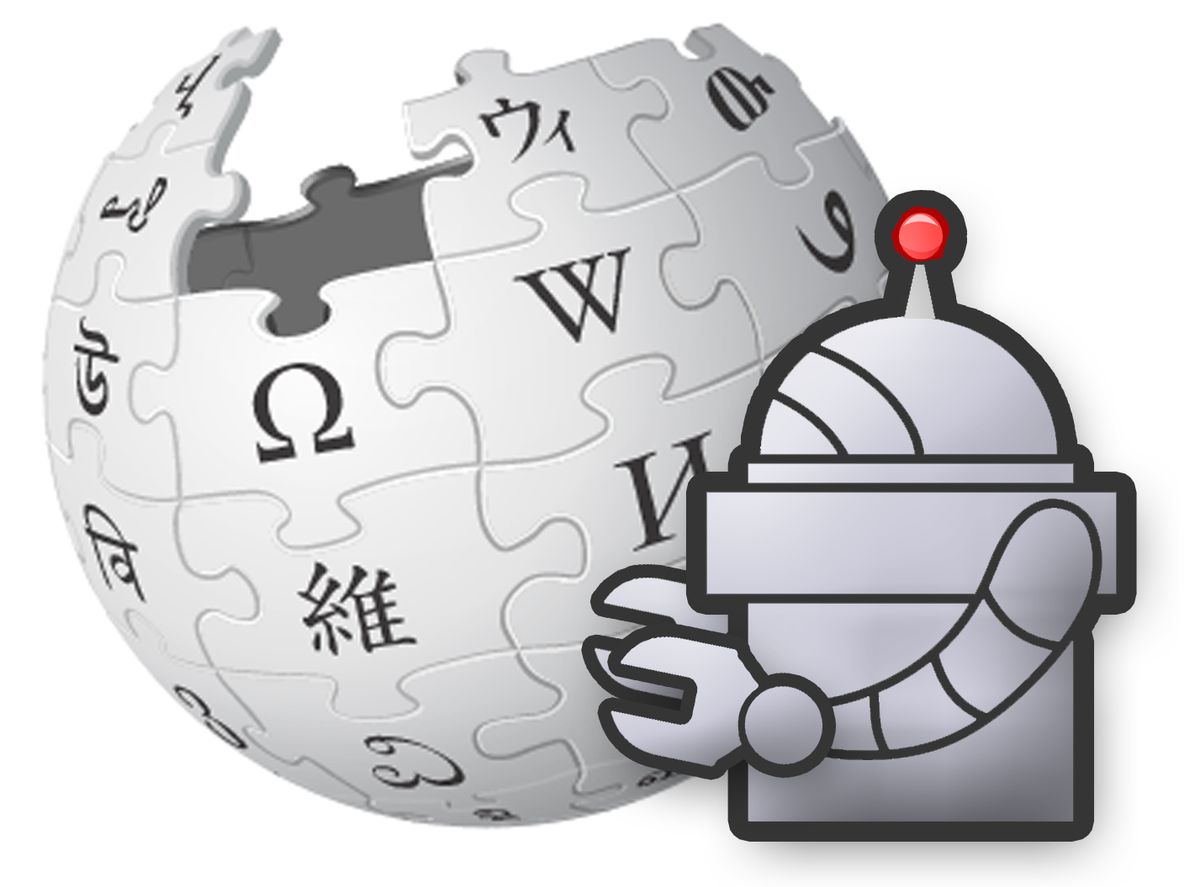
Continuous Learning and Adaptation
In the realm of AI-powered enterprise software, continuous learning and adaptation are not just beneficial; they are imperative for maintaining a competitive edge. As AI systems evolve, they must be capable of adapting to new data and changing environments without succumbing to catastrophic forgetting. This phenomenon, where new information overwrites previously learned knowledge, can be mitigated through techniques such as Neural Network with Dynamically Evolved Capacity (NADINE), which allows for partial updates and preserves essential knowledge.
The goal of continuous learning in AI is to enable systems to accumulate and refine knowledge over time, ensuring that they remain effective and relevant.
To achieve this, several strategies are employed:
- Utilizing effective data change detection methods to determine the best times for model updates.
- Leveraging self-supervised learning and continual learning techniques to generalize models for diverse data changes.
- Incorporating humans in the loop to enhance the learning process and ensure alignment with business goals.
Scalability of AI Solutions
The scalability of AI solutions is pivotal for businesses aiming to grow and adapt in the dynamic digital landscape. Scalable AI systems can handle increasing workloads and adapt to evolving business needs without compromising performance. This adaptability is essential for maintaining a competitive edge and ensuring long-term success.
Scalability involves more than just the ability to handle more data or transactions; it encompasses the integration of AI into various business processes and the seamless expansion of AI capabilities across different departments. To achieve this, businesses must focus on:
- Building flexible AI infrastructure that can grow with the company
- Ensuring AI systems are interoperable with existing technologies
- Developing AI solutions that can be easily updated and maintained
Scalability is not just a technical requirement; it's a strategic imperative that underpins the entire AI integration process.
While the initial investment in scalable AI may be significant, the long-term benefits can be substantial. Companies that prioritize scalability can expect to see improved efficiency, higher adaptability to market changes, and better overall performance of their AI-driven initiatives.
Practical Step-by-Step Guide for AI Implementation
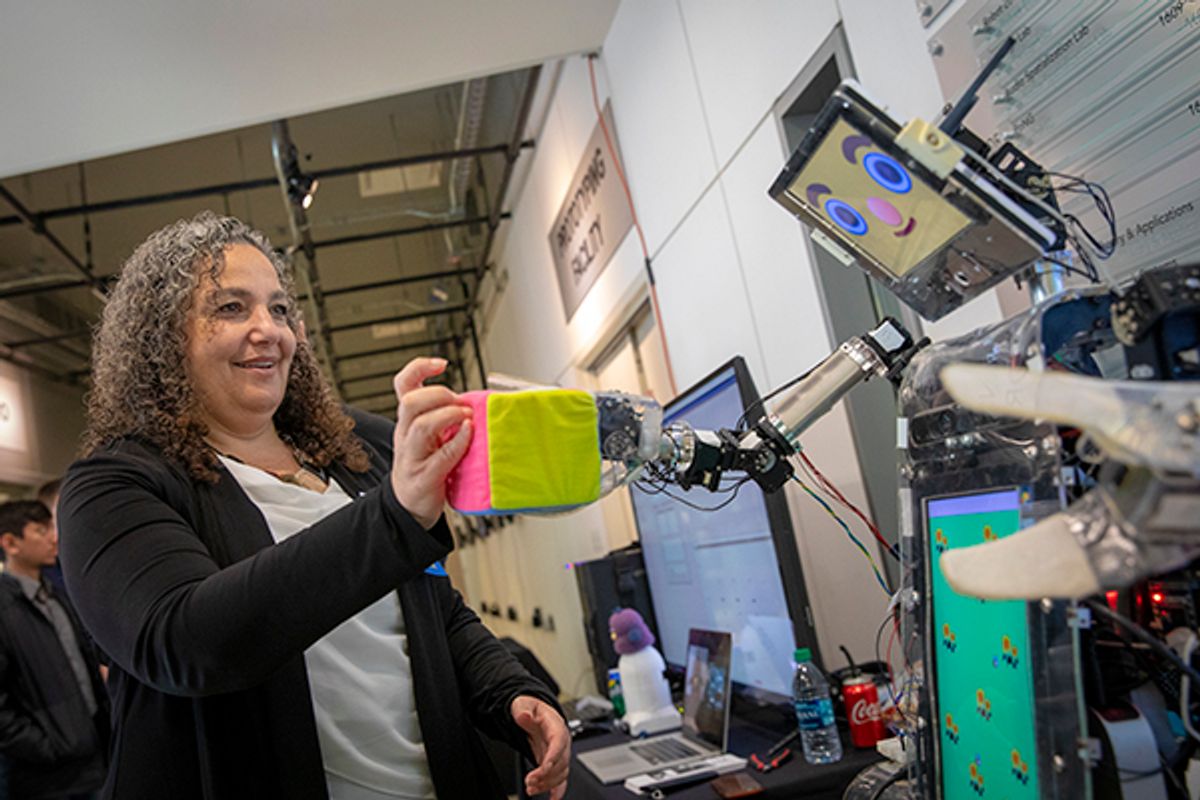
Step 1: Define Your Objectives
The foundation of a successful AI strategy begins with a clear definition of objectives. It's essential to identify the areas within your enterprise where AI can have the most significant impact, such as customer service, sales, or operations.
- Set specific, measurable goals that align with your overall business strategy.
- Determine the unique needs of your organization that AI can address.
- Establish benchmarks for success to guide development and measure progress.
By setting clear objectives, you create a roadmap for integrating AI technologies that can transform your enterprise operations. This step is crucial for ensuring that your AI initiatives are not only technologically sound but also strategically aligned with your business goals.
Remember, a well-defined objective serves as the north star for your AI journey, guiding every subsequent decision from technology selection to workforce development. As part of your blueprint, consider frameworks for implementation, data visualization tools, and strategies for enhancing your team's capabilities to support the transformation.
Step 2: Assess Your Data Infrastructure
Before diving into the world of AI, it's crucial to evaluate your existing data infrastructure. Ensure it is robust enough to handle the demands of AI technologies. This involves a thorough assessment of your data collection, storage, and management systems.
Identify any data gaps and strategize on how to fill them. The quality and quantity of your data are pivotal for the success of AI applications. Without sufficient data, even the most advanced AI algorithms can fail to deliver expected results.
A solid data foundation is not just about quantity; it's about the readiness of data to be utilized effectively by AI systems.
Consider the following checklist to guide your assessment:
- Review current data collection methods
- Evaluate data storage solutions
- Check data accessibility and security
- Analyze data quality and integrity
- Plan for data integration and scalability
Once you have a clear understanding of your data infrastructure's strengths and weaknesses, you can move forward with confidence, knowing that your AI initiatives are built on solid ground.
Step 3: Build or Enhance Your Team's Capabilities
To harness the full potential of AI, it's crucial to build or enhance your team's capabilities. Start by assessing your team's current expertise in AI and data science. Identify any skill gaps and consider investing in training programs or hiring new talent to address these needs. A team well-versed in AI can effectively manage and leverage AI technologies, driving innovation and efficiency.
- Assess current skill levels
- Invest in training or hiring
- Fill critical skill gaps
Ensuring your team is equipped with the necessary skills is not just about technical proficiency; it's about fostering a culture of continuous learning and innovation.
Remember, the goal is to create a robust team that can not only implement AI solutions but also adapt to the evolving AI landscape. This may involve a mix of upskilling current employees and recruiting new talent with specialized expertise.
Step 4: Select the Right AI Tools and Technologies
Selecting the appropriate AI tools and technologies is a pivotal step in the AI implementation journey. Research and evaluate various options that align with your specific objectives and the capabilities of your data infrastructure. Consider both off-the-shelf solutions and the potential for custom development. The decision should balance your needs, budget, and timeline for deployment.
- Ease of integration
- Scalability
- Support services
- User reviews
These factors are critical in determining the suitability of AI tools for your enterprise. It's essential to choose technologies that not only meet current requirements but also offer the flexibility to grow with your business.
Careful selection of AI tools and technologies is fundamental to the success of your AI initiatives. It ensures that your investment is aligned with your strategic goals and delivers tangible benefits.
Step 5: Pilot with a Proof of Concept
Embarking on the AI journey with a proof of concept (PoC) is a strategic move to validate the practicality of your AI solution. Start with a small-scale pilot to test the AI tools in a controlled environment. This step is crucial to identify potential issues and ensure that the solution fits within the existing ecosystem.
- Gather feedback from the pilot
- Iterate and refine the approach
- Ensure alignment with business processes
By adopting an incremental approach, you can demonstrate the value of the AI solution before scaling up. It's essential to implement data lineage, governance, and secure practices to enhance accuracy, compliance, and efficiency. The synergy between human expertise and AI capabilities is crucial for a successful implementation.
The PoC phase is not just about technology validation but also about gaining stakeholder buy-in and setting the stage for broader AI adoption across the enterprise.
Step 6: Implement AI Solutions
With a detailed implementation plan in hand, it's time to integrate the AI tools into your business processes. This step is crucial as it involves the actual deployment of AI within your enterprise, ensuring that the solutions work harmoniously with existing workflows and systems.
Ensure that all team members are clear on their roles and responsibilities to facilitate a smooth transition. Training and support should be provided to help staff adapt to the new technology.
It's essential to maintain open lines of communication during this phase to address any issues promptly and to make necessary adjustments.
Remember to document each stage of the implementation process:
- Initial setup and configuration
- Integration with existing systems
- User training and support
- Ongoing maintenance requirements
This documentation will be invaluable for future reference and for troubleshooting any potential issues that arise.
Step 7: Monitor and Optimize
After the implementation of AI solutions, it is crucial to establish a regimen of regular monitoring to ensure that the systems are performing as intended. This involves setting up key performance indicators (KPIs) that align with your business objectives and using them to measure the effectiveness of the AI.
Regularly monitor the performance of AI implementations, using predefined metrics to assess success against your objectives.
Continuous optimization is not just a one-time event but an ongoing process that adapts to new data, feedback, and changes in business requirements.
To effectively optimize AI systems, consider the following steps:
- Analyze performance data to identify areas for improvement.
- Implement changes based on insights from data and user feedback.
- Reassess KPIs and adjust them as necessary to reflect evolving business goals.
By embracing a cycle of monitoring and optimization, enterprises can maintain the relevance and efficiency of their AI systems, ensuring they continue to provide value in a dynamic business environment.
Step 8: Scale and Expand
After the initial success of AI implementation, it's time to scale and expand. Evaluate the impact and identify further opportunities where AI can add value. Gradually increase AI's footprint, ensuring each step is informed by previous learnings.
- Evaluate the impact of AI implementations
- Identify additional value-driven AI opportunities
- Scale AI use across business areas
- Leverage insights from initial experiences
Scaling AI initiatives is a transformative growth opportunity for organizations. It requires careful planning and execution to navigate the challenges effectively.
Remember to maintain a balance between speed and stability. Scalability is not just about growing quickly; it's about growing smartly. Ensure that your infrastructure, processes, and team are ready to support a larger scale of AI operations.
Step 9: Foster an AI-Driven Culture
To truly harness the power of artificial intelligence, it is essential to foster an AI-driven culture within the organization. This involves cultivating an environment where innovation is not just encouraged but is a fundamental aspect of the workplace ethos.
- Encourage employees to embrace AI opportunities and view them as tools for empowerment and growth.
- Promote collaboration between AI experts and various business units to ensure AI initiatives are well-integrated and support the company's strategic goals.
- Regularly review AI system performance to ensure optimal operation and alignment with expected outcomes, making adjustments as necessary.
By embedding AI into the organizational culture, businesses can create a dynamic and responsive environment that is primed for continuous improvement and technological advancement.
It is also crucial to integrate key strategies that focus on innovation, ethical AI use, and seamless integration of AI into business processes. This will not only prepare employees for the evolving landscape but also position the company at the forefront of AI adoption in its industry.
Step 10: Stay Informed and Adaptable
In the rapidly evolving landscape of artificial intelligence (AI), staying informed and adaptable is crucial for maintaining a competitive edge. Keep abreast of AI advancements and industry trends to ensure your strategies remain cutting-edge. This strategy should include a roadmap for AI adoption that is flexible and responsive to new developments.
Adaptability in AI initiatives is essential as new technologies emerge and business needs evolve. Be prepared to pivot and refine your approach in response to these changes.
To stay ahead, conduct thorough market research and evaluation. Regularly review and update your AI roadmap to reflect the latest trends and products. Here's a simple checklist to help maintain your AI initiative's relevance:
- Monitor key AI publications and forums
- Attend industry conferences and workshops
- Engage with AI thought leaders and communities
- Evaluate emerging AI technologies and tools
By committing to continuous learning and adaptation, you can ensure that your enterprise remains agile and innovative in the face of AI's constant progression.
Overcoming Common Scaling Mistakes with AI
Avoiding Over-ambition and Complexity
In the journey of integrating AI into enterprise systems, it's essential to avoid the pitfalls of over-ambition and unnecessary complexity. Scalability in AI requires strategic alignment, technical infrastructure, talent, governance, and financial planning. To ensure a smooth transition, companies should focus on incremental progress rather than attempting to overhaul systems all at once.
Collaboration across organizational roles is crucial for successful AI deployment. This involves clear communication and shared objectives among various departments to prevent siloed efforts and misaligned goals. Here are some common mistakes to avoid:
- Overlooking Data Privacy and Security
- Ignoring the Importance of Quality Data
- Underestimating the Need for Human Oversight
- Neglecting Employee Training and AI Integration
By taking a measured approach and prioritizing responsible AI implementation, enterprises can navigate the complexities of AI-augmented development to find sustainable success.
Ensuring Data Quality and Accessibility
The foundation of any successful AI initiative is the quality and accessibility of the data it utilizes. High-quality data standards are critical to ensure that AI systems have accurate and comprehensive information to work with. This involves regular data cleaning, validation, and enrichment processes that are essential for reliable AI outputs.
Data accessibility is equally important, allowing AI systems to integrate seamlessly while protecting sensitive information. However, it is imperative to maintain a balance between accessibility and security to safeguard against data breaches and ensure compliance with data protection regulations.
Ensuring data quality and accessibility is not a one-time task but an ongoing process that requires continuous attention and improvement.
Here are some key steps to maintain data integrity:
- Conduct regular data audits to identify and rectify issues.
- Implement robust data governance policies.
- Train staff on the importance of data quality and secure handling.
- Utilize advanced data management tools for better control and visibility.
By prioritizing data quality and accessibility, businesses can avoid common pitfalls that undermine the effectiveness of AI solutions and ensure a solid foundation for AI-driven growth.
Building a Skilled AI Workforce
The integration of AI into enterprise software delivery necessitates a workforce that is proficient in the latest technologies and methodologies. Investing in training or hiring new talent is essential to fill critical skill gaps and ensure that your team can effectively manage and leverage AI technologies. Upskilling and reskilling existing employees is a cost-effective strategy that can bridge the AI Talent Gap in software development.
To measure the impact of AI, companies will need to establish clear metrics, tracking development speed, code quality, and team satisfaction.
Fostering a culture of trust and collaboration is also crucial, as it prepares developers to work alongside their AI counterparts. This involves clear communication about the collaborative nature of AI augmentation, where AI amplifies human ability rather than replacing it. The following steps can guide you in building a skilled AI workforce:
- Assess your team's current skill levels in AI and data science.
- Identify and address any resistance to AI adoption among developers.
- Establish clear metrics for measuring the impact of AI on development.
By taking these steps, businesses can ensure they have a robust team capable of harnessing the full potential of AI.
The Evolution of AI-Augmented Software Development
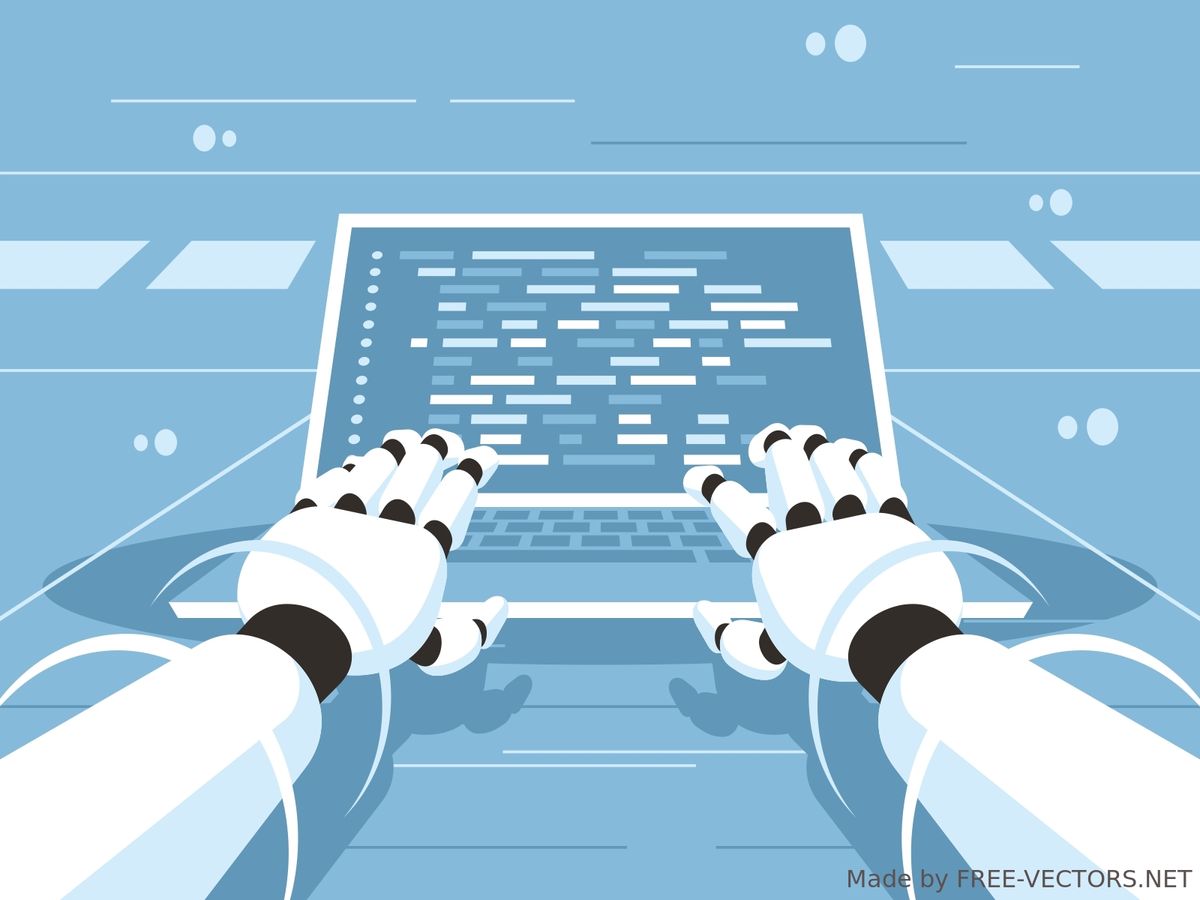
The Impact of AI on Development Lifecycles
The integration of AI into software development lifecycles marks a transformative era in enterprise software delivery. AI is not just a tool; it's a partner that enhances the capabilities of software engineers, enabling them to focus on innovation and high-quality output. By automating routine tasks and offering insights throughout the development process, AI helps to streamline the software development lifecycle, improve deployment processes, and enhance overall software quality.
AI-augmented development is about empowering developers to focus on quality and innovation while AI assists with the heavy lifting.
The benefits of AI-augmented development are manifold, including accelerated development cycles, improved code quality, and increased team satisfaction. However, the journey to AI-augmented development requires careful planning and a proactive approach. Training developers on AI's capabilities and limitations is crucial, as is establishing clear metrics to measure the impact of AI on development processes.
Challenges such as ensuring responsible AI implementation and fostering a culture of trust between developers and AI are not insurmountable. With a focus on responsible AI practices, companies can navigate these complexities and harness the full potential of AI-augmented development.
Innovative Growth Strategies Enabled by AI
The integration of AI into enterprise software delivery is not just about automation; it's about unleashing a new era of innovation. AI-augmented software development elevates the creation and maintenance of software, transforming projects for optimal results. By leveraging machine learning, businesses can anticipate market shifts and adapt their strategies accordingly, ensuring they remain competitive in a rapidly evolving digital landscape.
- AI for efficiency in operations and customer service
- Data analytics for informed decision-making
- AI-driven marketing for targeted campaigns
- Process automation to reduce manual workloads
- Fostering a culture of continuous AI exploration
AI's potential to revolutionize industries is immense, offering streamlined operations, enhanced customer experiences, and significant cost savings. As AI technology evolves, its impact across sectors deepens, paving the way for innovative growth strategies and operational excellence.
By embracing AI, businesses can scale operations, enhance customer experiences, and secure a competitive edge. The strategic application of AI services is crucial for steering clear of common pitfalls and achieving sustainable growth.
Navigating the AI Regulatory Landscape
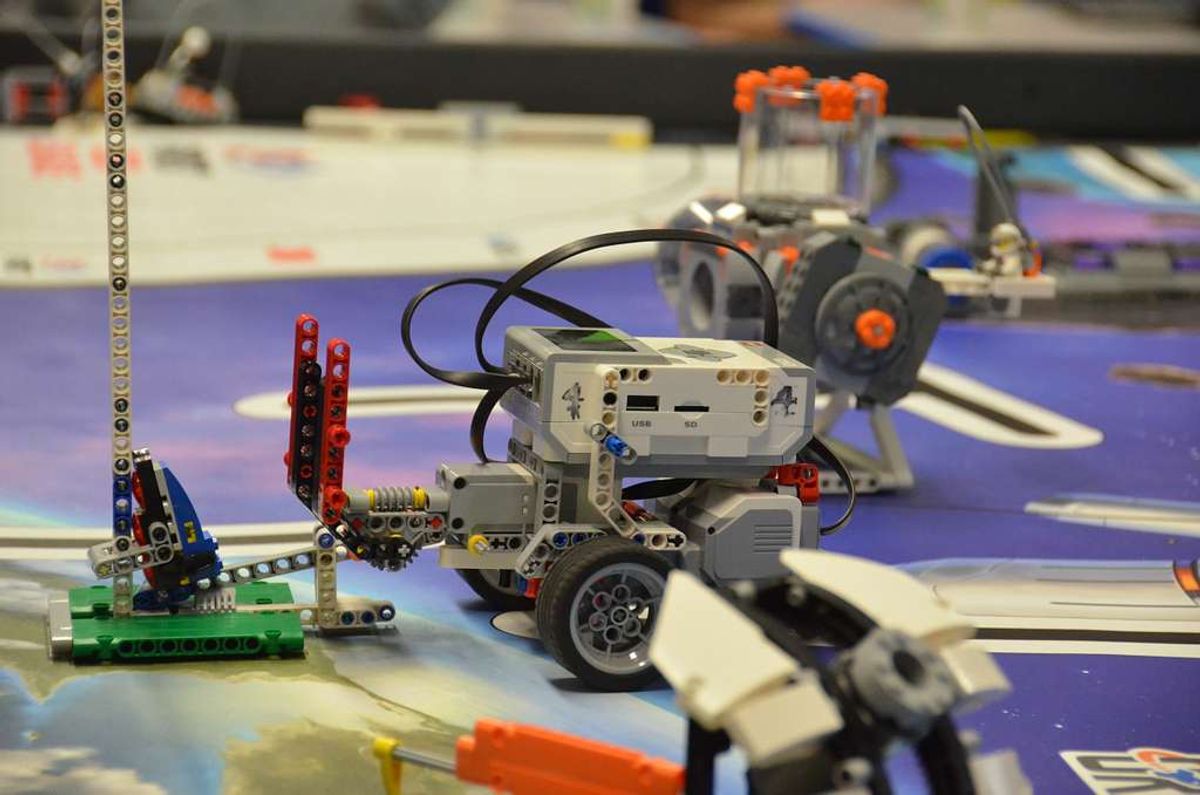
Understanding Compliance and Ethical Considerations
In the realm of AI, compliance and ethical considerations are paramount. Ensuring AI doesn’t perpetuate bias or create unfair outcomes is a critical ethical concern. Companies must balance the innovative potential of AI with the need for fairness and accountability.
Data privacy and security are non-negotiable, demanding robust security measures to protect sensitive information. AI tools must adhere to strict data protection regulations, safeguarding both customer and business data. Transparency and interpretability are also essential; AI-driven decisions must be easily understood and explained.
It is imperative that developers understand the 'why' behind AI suggestions, rather than blindly accepting them.
Here are some challenges and considerations:
- Understand what risks should be considered and managed.
- Make sure you have governance policies around managing AI-augmented business processes.
- Become comfortable with AI modeling, ensuring that protection and fairness awareness designs are integrated.
Developing AI Policies for Governance
In the realm of enterprise AI, developing robust governance policies is not just a regulatory requirement but a strategic imperative. Ensuring that AI systems operate within ethical and legal boundaries is crucial for maintaining trust and accountability. Key considerations include the management of risks, the regular review of AI performance, and the adaptability of AI models to changing business environments.
Ethical concerns, data privacy, and the need for transparency are at the forefront of AI governance. It is essential to balance these factors to prevent bias, protect sensitive information, and maintain clarity in AI-driven decisions. To this end, companies must:
- Understand and manage the risks associated with AI-augmented business processes.
- Regularly review and adjust AI system performance to optimize operations.
- Foster a culture of innovation and continuous learning among employees.
Developing AI policies for governance requires a proactive approach, focusing on continuous improvement and alignment with business values. It is not only about compliance but also about leveraging AI responsibly to drive growth and innovation.
Finally, training developers on AI capabilities and limitations is vital, as is establishing clear metrics to measure the impact of AI on development speed, code quality, and team satisfaction. This will ensure a future-proof strategy that harmonizes AI innovation with human expertise.
Measuring the ROI of AI in Enterprise Software Delivery
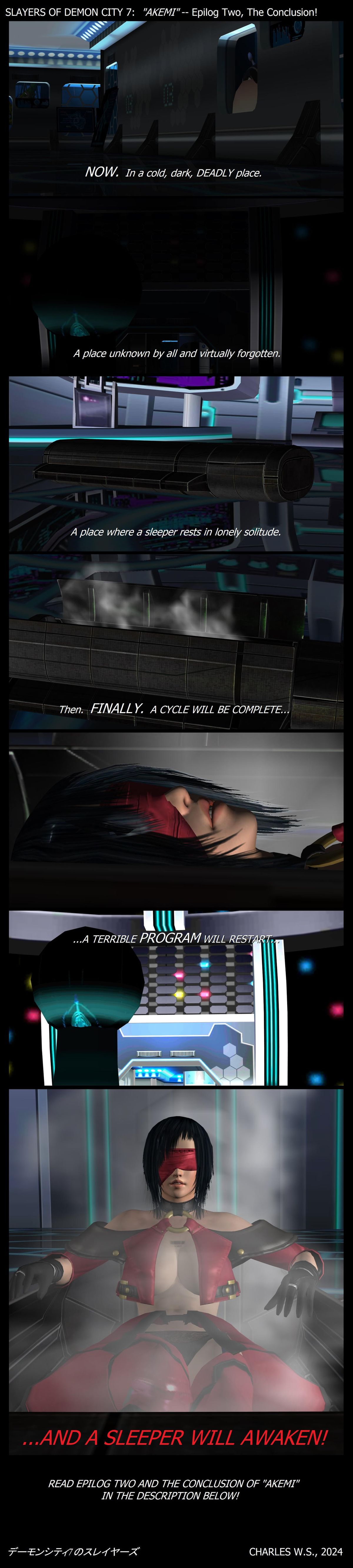
Quantifying Cost Savings and Efficiency Gains
In the realm of enterprise software delivery, the implementation of AI technologies has been pivotal in achieving significant cost savings and efficiency gains. AI-driven predictive analytics have been instrumental in enabling smarter decision-making, which in turn reduces the need for costly rework and optimizes resource allocation.
Operational efficiency is another area where AI has made a substantial impact. Automation tools powered by AI have streamlined a multitude of business operations, from inventory management to customer service, thereby reducing manual labor and minimizing errors.
The transformative power of AI is evident across various sectors, offering unique opportunities for growth and efficiency.
Here's a succinct overview of the cost savings and efficiency improvements reported in different industries:
- Healthcare: Enhancing patient care and operational efficiency.
- Manufacturing: Optimizing production and predictive maintenance.
- Transportation and Logistics: Achieving route optimization and fleet management.
By leveraging AI for data analytics, businesses can uncover insights from customer behavior, market trends, and operational performance, guiding strategic decisions that lead to measurable financial benefits.
Evaluating Long-term Strategic Benefits
When considering the integration of AI into enterprise software delivery, it is crucial to look beyond immediate cost savings and efficiency gains. Long-term strategic benefits are often the true measure of AI's value to a business. These benefits include sustained competitive advantage, agility in responding to market changes, and the continuous improvement of products and services.
Innovation is a key driver of long-term success, and AI is a catalyst for such innovation. For instance, in manufacturing, AI software can boost productivity, enhance quality control, and streamline supply chain management. This positions companies to not only improve current operations but also to explore new business models and revenue streams.
The strategic integration of AI can lead to a transformative impact on business models, enabling companies to adapt and thrive in an ever-evolving marketplace.
To effectively evaluate these long-term benefits, businesses should consider the following points:
- The ability of AI to drive innovation and create new market opportunities.
- The role of AI in enhancing customer experiences and building brand loyalty.
- The potential for AI to optimize resource allocation and open up new avenues for growth.
By focusing on these strategic aspects, enterprises can ensure that their investment in AI yields not just short-term gains, but a robust foundation for future success.
Unlock the full potential of your enterprise software with AI and measure its ROI with precision. At OptimizDBA, we specialize in database optimization and guarantee a significant performance increase. Our proprietary methodology has been trusted by over 500 clients since 2001. Don't just take our word for it; experience transaction speeds that are often 100 times faster. Ready to transform your data solutions? Visit our website and let's discuss how we can elevate your business to the next level.
Conclusion
In conclusion, leveraging AI to transform enterprise software delivery is not just a trend but a strategic imperative for future-proofing businesses. The integration of AI offers unparalleled benefits, including enhanced predictive analytics, cost savings, and the ability to make data-driven decisions. However, it requires a thoughtful approach that encompasses defining clear objectives, assessing data infrastructure, and fostering a culture of continuous learning and adaptability. By following a practical step-by-step guide and avoiding common pitfalls, enterprises can scale operations, improve customer experiences, and maintain a competitive edge. As we look to the future, the potential of AI-augmented development is vast, promising innovative growth strategies and operational excellence across industries.
Frequently Asked Questions
How does AI optimize manufacturing and predictive maintenance?
AI optimizes manufacturing by analyzing production data to improve efficiency and quality. It predicts maintenance needs to prevent downtime using machine learning algorithms that identify patterns indicating potential equipment failures.
What are the key strategies for effective AI integration in business?
Effective AI integration strategies include aligning AI with business goals, balancing investment with risk, addressing data privacy, and filling skill gaps. It's crucial to have a clear strategy that fits the business context and market needs.
Can AI help in scaling growth across different industries?
Yes, AI can scale growth across industries by providing enhanced predictive analytics for better decision-making and optimizing cost savings and resource allocation, leading to operational excellence and competitive advantages.
How does AI transform marketing and sales automation?
AI transforms marketing by analyzing customer behavior to create targeted campaigns that improve conversion rates. In sales, AI prioritizes leads and personalizes sales approaches, enhancing efficiency and effectiveness.
What does future-proofing your business with AI entail?
Future-proofing with AI involves fostering a culture of continuous learning and adaptation, as well as choosing scalable AI solutions that can grow with your business, ensuring long-term innovation and competitiveness.
What are the first steps to implementing AI in an enterprise?
The first steps to implementing AI include defining your objectives, assessing your data infrastructure, and building or enhancing your team's capabilities. This foundation is crucial for selecting the right AI tools and technologies.
What common mistakes should be avoided when scaling with AI?
Avoid over-ambition, complexity, and ensure data quality and accessibility. It's important to build a skilled AI workforce and to implement AI incrementally while focusing on strategic planning and continuous improvement.
What is the impact of AI on software development lifecycles?
AI impacts software development lifecycles by automating tasks, improving accuracy, and shortening development times. It enables innovative growth strategies by providing insights and efficiencies previously unattainable.